·
Engenharia Industrial Madeireira ·
Estatística Experimental
Envie sua pergunta para a IA e receba a resposta na hora
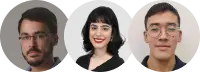
Prefere sua atividade resolvida por um tutor especialista?
- Receba resolvida até o seu prazo
- Converse com o tutor pelo chat
- Garantia de 7 dias contra erros
Recomendado para você
7
Exercícios Estatística Exp 2021 2
Estatística Experimental
UFES
80
Slide - Testes de Hipóteses - 2023-1
Estatística Experimental
UFES
34
Slide - Análise de Regressão - 2023-1
Estatística Experimental
UFES
6
Lista - Fatorial Parcelas Subdivididas Correlação e Regressão - Estatística Experimental 2023-1
Estatística Experimental
UFES
68
Slide - Regressão Linear Múltipla - 2023-1
Estatística Experimental
UFES
3
Lista 6 - Métodos Estatísticos 1 - 2023-1
Estatística Experimental
UFES
Texto de pré-visualização
CAPÍTULO VII – ANÁLISE DE REGRESSÃO Prof. Gilson Fernandes da Silva Departamento de Ciências Florestais e da Madeira (DCFM) Programa de Pós-graduação em Ciências Florestais (PGCF) Universidade Federal Espírito Santo (UFES) 8. INTRODUÇÃO À ANÁLISE DE REGRESSÃO O modelo de regressão definido como um modelo estatístico, difere em conceito de um modelo matemático por apresentar um termo denominado erro aleatório. Modelo Matemático Modelo de regressão X Y 1 0 X Y 1 0 0 1 2 3 4 5 6 7 0 2 4 6 8 X Y 0 2 4 6 8 10 12 0 2 4 6 8 X Y Modelo Matemático Modelo de regressão X Y 1 0 X Y 1 0 9. REGRESSÃO LINEAR SIMPLES Dados n pares de valores de duas variáveis Xi e Yi (i = 1, 2, ..., n), admitindo que Y é função linear de X, pode-se estabelecer uma regressão linear simples, cujo modelo estatístico é: em que β0 e β1 são os parâmetros do modelo e εi e são os erros aleatórios. O coeficiente angular da reta (β1) é também denominado coeficiente de regressão e o coeficiente linear da reta (β0) é também conhecido como termo constante da equação de regressão. i i i X Y 1 0 Pressuposições do modelo de Regressão linear simples 1) A relação entre X e Y é linear nos parâmetros. 2) Os valores de X são fixos, isto é, X é não estocástico. 3) A média do erro é nula, isto é, E(εi) = 0. 4) Para um dado valor de X, a variância do erro é sempre σ2, denominada variância residual, isto é, V(εi) = σ2. Pode-se dizer então que o erro é homocedástico ou que há homocedasticia (do erro ou da variável dependente). 𝑬 𝜺𝒊 𝟐 = 𝝈𝟐 ou E[Yi - E(Yi / Xi )]2 = σ2 5) O erro de uma observação é independente do erro em outra observação, isto é, E(εi εj) = 0 para i j. 6) Os erro têm distribuição normal. Combinando as pressuposições 3), 4), 5) e 6) tem- se que: εi ~ NID(0, σ2), em que NID significa Normal e Independentemente Distribuído. Deve-se verificar, entretanto, se o número de observações disponíveis é maior do que o número de parâmetros da equação de regressão. Para se ajustar uma regressão linear simples é preciso ter no mínimo 3 observações. Caso se disponha apenas de dua observações, a determinação da reta é um problema de geometria analítica, não é possível, neste caso, fazer nenhuma análise estatística. O método de Mínimos Quadrados Ordinários (MQO) Este é um método de estimação muito empregado para estimar parâmetros de modelos de regressão. Seu objetivo principal consiste em minimizar a soma de quadrados dos erros, tal como será demonstrado a seguir: Yi = 0 + 1Xi + i (1) i = Yi - 0 - 1Xi (2) (3) n i i i n i i X Y 1 2 1 0 1 2 0 1 2 1 1 0 0 1 2 n i i i n i i X Y 0 2 1 1 0 1 1 2 i n i i i n i i X X Y (4) (5) Dividindo-se (4) e (5) por -2, tem-se: n i i i i n i i i X X Y X Y 1 1 0 1 1 0 0 ˆ ˆ 0 ˆ ˆ n i n i i n i i i i n i n i i i X X Y X X n Y 1 1 2 1 1 0 1 1 1 0 0 ˆ 0 ˆ ˆ n i n i i n i i i i n i n i i i X X Y X X n Y 1 1 2 1 1 0 1 1 1 0 0 ˆ 0 ˆ ˆ (6) (7) n X n Y n i i n i i 1 1 1 0 ˆ ˆ X Y 1 0 ˆ ˆ (8) Sistema de Equações Normais (SEN) Substituindo (8) em (7) e desenvolvendo a expressão, tem-se: n X X n Y X Y X n i i n i i n i i n i i n i i i 2 1 1 2 1 1 1 1ˆ (9) X Y 1 0 ˆ ˆ ˆ e Duas relações muito úteis podem ser obtidas a partir do SEN. Para se desenvolver a primeira é preciso lembrar que: (10) i i i Y Y e ˆ i i X Y 1 0 ˆ ˆ ˆ n i i i n i i X Y e 1 1 0 1 ˆ ˆ n i i n i i n i i X n Y e 1 1 0 1 1 ˆ ˆ n i i n i i n i i n i i n i i X n X n Y n Y e 1 1 1 1 1 1 1 ˆ ˆ 0 ˆ ˆ 1 1 1 1 1 1 1 n i i n i i n i i n i i n i i X X Y Y e De modo que: A segunda relação diz respeito a: 0 ˆ ˆ 1 1 0 n i i i i X X Y 0 ˆ ˆ 1 2 1 1 0 1 n i i n i i n i i i X X X Y 0 ˆ ˆ 1 2 1 1 1 1 1 1 n i i n i i n i i n i i n i i i X X n X n Y X Y 0 ˆ ˆ 1 2 1 2 1 1 1 1 1 n i i n i i n i i n i i n i i i X n X n Y X X Y (11) 0 ˆ 1 2 1 2 1 n i n i i i XY n X X SPD 0 ˆ ˆ 1 1 1 0 X X XY XY n i i i n i i i i SQD SQD SPD SPD X e X X Y 0 1 n i iei X Concluindo-se que: Pode-se demonstrar também que: Como já demonstrado em (10) e (11): (12) 0 ˆ ˆ ˆ 1 1 0 1 n i i i n i i i X e Ye 0 ˆ ˆ ˆ 1 1 1 0 1 n i i i n i i n i i i X e e Ye 0 1 n i ie e 0 1 n i iei X De onde se deduz que: 0 ˆ 1 n i Yiei Outra relação útil pode ser derivada como se segue: Como demonstrado em (10), a soma dos erros é igual a zero. Então, temos: i i i e X Y 1 0 ˆ ˆ i i i e Y Y ˆ n i i n i i n i i i n i i e Y e Y Y 1 1 1 1 ˆ ˆ n i i n i i Y Y 1 1 ˆ ou Y Y ˆ Exemplo de aplicação: (Soares et al, 2007) Árv D(cm) H(m) v(m3) D2H = X X2 XY Y2 1 8,0 9,7 0,0274 620,8 385392,6 17,0 0,0008 2 27,7 27,6 0,7159 21177,2 448473969,3 15160,8 0,5125 3 23,2 26,5 0,5505 14263,4 203443438,5 7852,0 0,3031 4 17,7 17,4 0,178 5451,2 29716083,0 970,3 0,0317 5 13,8 12,9 0,1003 2456,7 6035257,0 246,4 0,0101 6 17,0 16,5 0,1852 4768,5 22738592,3 883,1 0,0343 7 18,8 20,3 0,2423 7174,8 51478214,2 1738,5 0,0587 8 8,0 11,6 0,0327 742,4 551157,8 24,3 0,0011 9 15,0 16,7 0,1292 3757,5 14118806,3 485,5 0,0167 10 21,6 21,2 0,3542 9891,1 97833305,3 3503,4 0,1255 11 11,0 12,8 0,0608 1548,8 2398781,4 94,2 0,0037 12 24,2 24,7 0,4368 14465,3 209245135,5 6318,4 0,1908 Soma 3,0133 86317,7 1086418133,1 37293,8 1,2888 Exemplo de ajuste do modelo de Spurr (1952) D H v 2 1 0 X Y 1 0 n X X n Y X Y X n i i n i i n i i n i i n i i i 2 1 1 2 1 1 1 1ˆ 0000336 ,0 12 86317 7, 1, 1086418133 12 ,3 0133 86317 7, 8, 37293 ˆ 2 1 X Y 1 0 ˆ ˆ ,0 0097713 ,0 0000336 71931, ,0 2511 ˆ 0 D H v 2 ,0 0000336 ,0 0097713 ˆ ANÁLISE DE VARIÂNCIA DA REGRESSÃO (ANOVA) FV GL SQ QM Fcalc Regressão 1 0,52402 0,52402 647,94** Resíduo 10 0,00809 0,00081 Total 11 0,53211 10,04 1 %; 1 1 0 gl e Ftab O modelo simplificado Uma simplificação conveniente dos cálculos é obtida quando se usa a variável centrada, isto é: 𝑥𝑖 = 𝑋𝑖 − 𝑋 Na representação gráfica, isso corresponde a tomar a média da variável Xi como origem do eixo das abscissas. Substituindo a variável independente original pela variável centrada, o modelo estatístico fica: i i i x Y 1 0 (13) (14) Admitindo que , o SEN agora ficará: 0 1 n i ix n i n i i n i i i i n i n i i i x x Y x x n Y 1 1 2 1 1 0 1 1 1 0 0 ˆ ˆ 0 ˆ ˆ n i n i i i i n i i x Y x n Y 1 1 2 1 1 0 ˆ ˆ n i i n i i i x Y x 1 2 1 1ˆ n Y n i i 1 0ˆ (15) (16) i i i i x y ou x Y Y 1 1 ˆ ˆ ˆ ˆ A equação estimada para o modelo simplificado será então: Propriedades dos estimadores do modelo de regressão Os estimadores obtidos pelo método de Mínimos Quadrados são não tendenciosos. Para que isso seja verdade, é necessário demonstrar que: 1 0 ˆ ˆ e 1 1 0 0 ˆ ˆ e E E O caso de 1ˆ Como foi demonstrado por meio do SEN para a variável centrada na média (xi), temos que: Vamos admitir que: De onde vem que: n i iYi k 1 1ˆ n n n n n n i i n i i i Y x x x x Y x x x x Y x x x x x Y x 2 2 1 2 2 2 1 2 1 2 2 1 1 1 2 1 1ˆ 2 2 1 2 2 1 2 2 2 1 1 1 2 n n n n n i i i i x x x x x x x x x x x x x x k (17) Admitindo que os valores de Xi são fixos (pressuposição 2), temos: Como i i i X Y 1 0 tem-se que: n i i i i n i i i X k Y k 1 1 0 1 1ˆ n i i i n i i i n i i k k X k 1 1 1 1 0 1ˆ n i i i n i i i n i i k k X k E E 1 1 1 1 0 1ˆ n i i i n i i i n i i k E k X k E 1 1 1 1 0 1ˆ (19) (18) Entretanto, sabe-se que: (21) 0 1 2 1 1 n i i n i i n i i x x k Admitindo que E(i) = 0, temos: n i i i n i i k X k E 1 1 1 0 1ˆ (20) Prova: nX X X X x n i n i i i n i i 1 1 1 Então: nX X n X X n i i n i i 1 1 mas, 0 1 n i ix n i i n i n i i i n i i n i i i i n i i n i i n i i i x X X X x X X X X x x X k 1 2 1 1 2 1 2 1 1 2 1 1 (22) Do mesmo modo que: n X X X n X X X X X n i i n i i n i i n i i n i i n i i n i i 2 1 1 2 1 1 1 2 1 1 2 Mas, (23) Contudo, n i i i n i i n i i X X X X X X x 1 2 2 1 2 1 2 2 n i n i i i n i i nX X X X x 1 2 1 2 1 2 2 n i n i i n i i i n i i n X n n X X x 1 2 2 1 2 1 2 1 2 2 n i n i i i n i i n i i n X X X X x 1 2 1 2 1 2 1 2 (24) A expressão (19) permite concluir que o estimador é um estimador não tendencioso do parâmetro β1 . 1 1ˆ E (26) Substituindo (25) e (21) em (20), temos: Comparando as expressões (23) e (24) e sua relação com a expressão (22), concluímos que: 1 1 n i kiXi (25) O caso de 0ˆ X Y 1 0 ˆ ˆ 1 1 0 ˆ ˆ ˆ XE E Y X E Y E Admitindo que X é uma variável fixa, temos: Mas, foi demonstrado que: 1 1ˆ E Então, temos que: 1 1 0 1 1 ˆ X E Y n X Y n E E n i i n i i Como i i i X Y 1 0 , tem-se que: 1 1 1 0 0 1 ˆ X X E n E n i i i 1 1 1 0 0 1 1 ˆ X X n n n E n i i 1 1 1 0 0 1 ˆ X E X n E n i i i 0 1 0 0ˆ n X n X E n i i n i i (27) 0 0ˆ E A expressão (27) permite concluir que o estimador é um estimador não tendencioso do parâmetro β0, Isto é: 0ˆ Variâncias dos estimadores dos parâmetros A variância de pode ser definida como: 1ˆ Foi demonstrado que: n i i i n i i i n i i k k X k 1 1 1 1 0 1ˆ (veja expressão 25) 0 1 n i ik 1 1 n i iXi k (veja expressão 18) (veja expressão 21) (28) 2 1 1 1 ˆ ˆ ˆ E E V 2 1 1 1 ˆ ˆ E V Substituindo (21) e (25) em (18), temos: n i i ik 1 1 1ˆ Mas, também foi definido( expressão 17) que: n i i i i x x k 1 2 e, portanto: n i i n i i i x x 1 2 1 1 1ˆ (29) Substituindo a expressão (29) na (28), vem que: 2 1 2 2 1 1ˆ n i i n i i i x x E V Mas, por meio de produtos notáveis, pode-se demonstrar que: 1 1 1 1 2 1 2 1 2 2 2 2 2 1 2 1 2 1 2 2 2 n n n n n i i i x x x x x x x E x x E Expressão (30) Sabe-se, contudo, que, de acordo com as pressuposições (2), (4) e (5), temos que: - Pressuposição (2): é fixo. - Pressuposição (4): - Pressuposição (5): E(εiεj) = 0 A expressão (30), de acordo com estas pressuposições, pode agora ser reescrita como: n i i n n i i i x x x x x E 1 2 2 2 2 2 2 2 2 2 1 2 1 2 ix 𝐸 𝜀𝑖 2 = 𝜎2 (31) Finalmente, pode-se concluir que: n i i n i i n i i x x x V 1 2 2 2 1 2 1 2 2 1ˆ ou n i ix s V 1 2 2 1ˆ ˆ Em que: s2 = Quadrado médio do resíduo da ANOVA da regressão (assista as próximas aulas!!!). Mas, sabe-se que (expressões 8 e 16): 2 0 0 0 ˆ ˆ ˆ E E V 2 0 0 0 ˆ ˆ E V A variância de pode ser definida como: 0ˆ (32) X x Y x n Y X Y n i i n i i i n i i 1 2 1 1 1 0 ˆ ˆ i n i n i i i Y X x x n 1 1 2 0 1 ˆ De modo que pode ser escrito como: 0ˆ Como i i i X Y 1 0 tem-se que: i i n i n i i i X X x x n 1 0 1 1 2 0 1 ˆ n i i n i i i n i i n i i i n i i n i i n i i n i i n i x x X x X x X x x X n n X n 1 2 1 1 2 1 1 1 2 1 0 1 1 1 1 0 0ˆ Na demonstração da expressão (21), tem-se que: O que simplifica a expressão anterior para: (33) 0 1 n i ix n i i n i i i n i i n i i i n i i x x X x X x X n X 1 2 1 1 2 1 1 1 1 0 0ˆ Por outro lado, pode-se demonstrar que: n i n i i i n i i i i n i i X X X X X X X X X 1 1 2 1 2 1 n i n i i i i n i i n X X X x 1 2 1 2 1 n i n i i n i i i i n i i X n X X X X X 1 1 1 2 1 i n i i i n i i X X X x X 1 1 (34) De acordo com o demonstrado para a expressão (24): n i n i i i n i i n X X x 1 2 1 2 1 2 De modo que substituindo as expressões (24) e (34) na expressão (33), concluímos que: n i i i n i i x X x 1 2 1 e, portanto, n i i n i i i n i i x x X X n X 1 2 1 1 1 1 0 0ˆ n i i n i i i n i i x x X n 1 2 1 1 0 0ˆ Mas, de acordo com a expressão 17, temos: n i i i i x x k 1 2 n i i i n i i k X n 1 1 0 0ˆ (35) Substituindo a expressão (35) na (32), temos: n i i i n i i i Xk n E Xk n E V 1 2 2 2 0 1 0 0 1 1 ˆ i n i X ik n 1 0 0 1 ˆ n i i i E Xk n V 1 2 2 0 1 ˆ (36) n i i i i n Xk X k n Xk n 1 2 2 2 2 2 1 1 (37) Substituindo a expressão (37) na (36), temos: n i i i i E n Xk X k n V 1 2 2 2 2 0 2 1 ˆ 𝐸 𝜀𝑖 2 = 𝜎2 De acordo com a pressuposição (4), temos: Então: 2 2 2 2 2 2 2 2 2 2 2 1 2 1 2 2 0 2 1 2 1 2 1 ˆ n Xk X k n n Xk X k n n Xk X k n V n n n i i i n i i i n Xk X k n n Xk X k n V 1 2 2 2 2 2 2 1 2 2 2 2 0 2 2 1 ˆ n k X k X n V n i i n i i 1 2 1 2 2 2 2 0 2 ˆ 0 1 2 1 1 n i i n i i n i i x x k n i ik X n V 1 2 2 2 2 0ˆ Mas, de acordo com a expressão (21), sabe-se que: E assim temos: (38) A expressão (38) pode ser reescrita como: n i i n i i k X n k X n V 1 2 2 2 1 2 2 2 2 0 1 ˆ n i i n i i k X n n k X n n V 1 2 2 2 1 2 2 2 0 1 1 ˆ n i i i i x x k 1 2 Sendo (expressão (17) 2 1 2 2 2 n i i i i x x k (39) n i i n i i n i i n i i x x x k 1 2 2 1 2 1 2 1 2 1 (40) Substituindo a expressão (40) na (39), temos: n i ix X n V 1 2 2 2 0 1 ˆ Mas, pode-se demonstrar que: (41) n i i n i i n i i x n nX x x X n 1 2 2 1 2 1 2 2 1 Mas, segundo a expressão (24): n i n i i i n i i n X X x 1 2 1 2 1 2 De onde vem que: n i i n i i n i i n i i n i i n i i n i i x n X x n n X n n X X x X n 1 2 1 2 1 2 2 2 1 2 1 1 2 1 2 2 1 (42) Assim, tem-se: 2 1 2 1 2 0ˆ n i i n i i x n X V ou 2 1 2 1 2 0ˆ ˆ s x n X V n i i n i i Substituindo a expressão (41) na (40), temos: (43) Covariância das estimativas dos parâmetros A covariância entre entre as estimativas dos parâmetros pode ser definida como: Do SEN pode-se obter que: X Y 1 0 ˆ ˆ Substituindo esta expressão na anterior, vem que: 1 0 1 1 1 0 ˆ ˆ cov ˆ , ˆ X Y E 1 0 1 0 1 1 0 0 1 0 ˆ ˆ ˆ ˆ ˆ ˆ ˆ ˆ cov ˆ , ˆ E E E E E E Contudo, sabe-se que: 1 0 2 1 1 1 0 1 1 1 0 ˆ ˆ ˆ ˆ cov ˆ , ˆ X E Y X Y E 1 0 2 1 1 1 0 ˆ ˆ cov ˆ , ˆ XE Y E n Y Y n i i 1 e 2 1 1 2 1 2 1 2 1 1 ˆ ˆ ˆ ˆ ˆ ˆ E V E E E V Assim, temos que: 1 0 2 1 1 1 1 1 0 ˆ ˆ ˆ 1 cov ˆ , ˆ E X V Y n E n i i 1 0 2 1 1 2 2 1 1 1 0 1 0 ˆ 1 cov ˆ , ˆ n i i n i i i X X X X E n 1 0 2 1 1 2 2 1 1 1 1 1 0 1 0 ˆ ˆ ˆ 1 cov ˆ , ˆ X X X X X E n n i i n i i i 1 0 2 1 1 2 2 1 1 2 1 1 0 1 0 ˆ 1 cov ˆ , ˆ X X X X E X n n i i n i i i 1 0 2 1 1 2 2 1 1 2 1 1 0 1 0 ˆ 1 cov ˆ , ˆ X X X X E n X n i i n i i n i i n i i X X X E n 1 2 2 1 1 1 0 ˆ 1 cov ˆ , ˆ (44) Nesse momento, vamos analisar a expressão . Primeiro é preciso relembrar a expressão (16), ou seja: n i i n i i i x Y x 1 2 1 1ˆ i 1ˆ E i n i i n i i i i x Y x 1 2 1 1ˆ Considerando e desenvolvendo os somatórios, temos: i i i X Y 1 0 Note que ao se fazer o produto entre o i-ésimo erro e o estimador de β1, teremos produtos cruzados entre os erros. Para distinguir estes cruzamentos, vamos denominar o índice relativo ao estimador de β1 de j e o índice relativo a ε de i. Assim, o somatório desdobrado pode ser reescrito como: n n n n n n n n n i n i i n i i i Y x x x x Y x x x x Y x x x x Y x x x x x Y x 2 2 1 1 2 2 1 1 1 2 2 1 1 2 2 1 1 1 2 1 1ˆ 2 2 1 1 0 1 2 2 1 1 1 1 0 1 2 2 1 1 1 0 2 2 1 1 1 1 1 0 1 1 2 1 1ˆ n n n n n n n n n n n i n i i n i i i x x x X x x x x X x x x x X x x x x X x x Y x i n i j n i j j j i x X x 1 2 1 1 0 1ˆ Mas, da expressão (21) demonstrou-se que: i j j n i i n i j i X x x E E 1 0 1 2 1 1ˆ j i j i i n i i n i j i X E x x E 1 0 1 2 1 1ˆ (45) 0 1 1 n i j n i i x x Assim, de acordo com a expressão (45), não importa se i = j ou i j, temos que: 0 ˆ 1 i E Substituindo esta identidade na expressão (44) , temos que: n i i n i i x X X X X 1 2 2 1 2 2 0 ˆ1 cov ˆ , ou RESUMO 2 1 2 1 2 0ˆ ˆ s x n X V n i i n i i n i ix s V 1 2 2 ˆ 1ˆ DECOMPOSIÇÃO DA SOMA DE QUADRADOS Considere a seguinte identidade: i i i i Y Y Y Y Y Y ˆ ˆ n i i n i i n i i e y y 1 2 1 2 1 2 ˆ Pode-se demonstrar que: n i i i n i i n i i Y Y Y Y Y Y 1 2 1 2 1 2 ˆ ˆ ou Da identidade anterior, pode-se concluir que a soma de quadrados do total (SQTot) é igual a soma de quadrados da regressão (SQReg) mais a soma de quadrados do erro, do resíduo ou dos desvios (SQRes). n i i i i n i i Y Y Y Y Y Y 1 2 2 1 ˆ ˆ i i n i i n i i i n i i n i i Y Y Y Y Y Y Y Y Y Y ˆ ˆ 2 ˆ ˆ 1 1 2 1 2 2 1 i n i i n i i i n i i n i i Y e Y Y Y Y Y Y Y 1 1 2 1 2 2 1 ˆ 2 ˆ ˆ n i i i n i i n i i Y Y Y Y Y Y 1 2 1 2 1 2 ˆ ˆ n i n i i i i n i i i n i i n i i e Y Y e Y Y Y Y Y Y 1 1 1 2 1 2 1 2 ˆ 2 ˆ ˆ Isto é, SQTot = SQReg + SQRes . 1 2 1 2 2 1 SQTot n Y Y Y Y n i n i i i n i i C n Y n i i 2 1 Correção para a média Tomando-se como base as expressões (10) e (12), vem que: n i i n i i Y X Y Y g SQ 1 2 1 0 1 2 ˆ ˆ ˆ Re n i i n i i X X Y X X Y g SQ 1 2 1 1 1 2 1 1 ˆ ˆ ˆ ˆ Re X n i i n i i SQD x X X g SQ 2 1 1 2 2 1 1 2 12 ˆ ˆ ˆ Re A SOMA DE QUADRADOS DA REGRESSÃO ou X XY n i n i i i n i i n i i n i i i SQD SPD n X X n Y X Y X g SQ 2 1 2 1 2 2 1 1 1 Re n i i n i i n i i i n i i n i i n i i i n i i x x y x x x y x x g SQ 1 2 2 1 2 2 1 1 2 2 1 2 1 1 2 1ˆ2 Re ou XY n i i i n i i i n i i n i i i SPD x y y x x y x g SQ 1 1 1 1 1 2 1 ˆ ˆ Re Em resumo, temos: X XY SQD SPD g SQ 2 Re ou SQDX g SQ 1ˆ2 Re SPDXY g SQ 1ˆ Re ou A soma de quadrados do resíduo usualmente é obtida por diferença, isto é: SQRes = SQTot - SQReg O COEFICIENTE DE DETERMINAÇÃO (r2) O coeficiente de determinação pode ser interpretado como: • A proporção da variação de Y que é “explicada” pela regressão ou • Quanto da SQTot é “explicada”pela regressão. Y X XY SQD SQD SPD r Y X XY SQD SQD SPD r 2 2 Mas, foi demonstrado que: X XY SQD SPD g SQ 2 Re e SQDY SQTot Portanto: SQTot g SQ r Re 2 ESPERANÇAS DAS SOMAS DE QUADRADOS Vamos começar pela esperança da soma de quadrados da regressão. Sabe-se que: i i i X Y 1 0 n Y Y n i i 1 e Portanto, X n X Y n i i i 1 0 1 1 0 (46) Subtraindo a expressão (46) de Yi, temos que: X X Y Y i i i 1 0 1 0 i i i x y 1 2 1 1 1 2 2 1 1 2 1 1 Re n i i i i n i i n i i i n i i x x x y x x g SQ 2 1 1 1 2 1 1 2 1 Re n i i n i i i n i i n i i x x x x g SQ (48) (47) Mas, de acordo com o demonstrado para a expressão (21), temos que: 0 1 n i ix O que reduz a expressão (48) a: 2 1 1 2 1 1 2 1 Re n i i i n i i n i i x x x g SQ Desenvolvendo o produto notável, temos: n i n i i i i n i i i n i i n i i x x x x x g SQ 1 1 2 1 2 1 2 1 2 2 1 1 2 2 1 Re (49) Assumindo que x é uma variável fixa e aplicando esperança a expressão (49), teremos: n i i i n i i n i i i n i i n i i x E x x E x x g SQ E 1 1 2 2 1 1 2 1 2 2 1 1 1 Re Como já demonstrado (veja expressão 31): n i i n i i i x x E 1 2 2 2 1 (50) Do mesmo modo que: 0 2 2 1 1 1 1 n n n i i i n i i i x E x E x E E x x E De modo que: fixa E(i) = 0, pressuposição (3) 2 1 2 12 Re n i ix g SQ E (51) A seguir será demonstrado como se obter a esperança da soma de quadrados do total. n i i n i i y Y Y SQTot 1 2 2 1 i i i x y 1 De acordo com a expressão (50): n i i x SQTot 1 2 1 1 n i i i i i x x SQTot 1 1 2 2 12 2 n i i i n i i n i i x x SQTot 1 1 1 2 1 2 12 2 Aplicando esperança a SQTot, teremos: (52) n i i i i n i i n i i x x E E x SQTot E 1 1 1 2 1 2 12 2 Mas, pode-se demonstrar que: 0 1 1 1 n i i n i i i n i i i i x x E x x E Pois, de acordo com as expressões (21) e (51), temos: 0 1 n i ix e 0 1 n i ix i E (53) mas, 1 2 2 2 2 1 1 2 1 2 n n n E E n i i n i i n i i Assim, a expressão (53) pode ser reescrita como: n i i n i i E x SQTot E 1 2 1 2 12 Assim, temos que: 2 1 2 12 1 n x SQTot E n i i n E E n E n i i n i i n i i n i i 2 1 1 2 2 1 1 2 Para compreender melhor a dedução anterior, é interessante notar que: 2 2 2 1 2 1 2 1 2 n n i i n i i E E E E E De acordo com a pressuposição (4), 2 2 i E Então, 2 2 2 2 1 2 n E n i i Por outro lado, 2 2 1 2 1 2 1 1 1 n n i i n i i n E n E n E 1 1 1 2 1 2 2 2 2 1 2 2 1 2 2 2 2 1 1 n n n n n n n E n E 2 2 i E Mas, de acordo com as pressuposições (4) e (5), (4) e 0 i j E 2 2 2 1 1 1 n n E n n i i (5) SQRes = SQTot - SQReg Falta obter a esperança da soma de quadrados de resíduos. E[SQRes] = E[SQTot] – E[SQReg] 2 1 2 2 1 2 1 2 12 1 Re n i i n i i x n x s SQ E 2 2 Re s n E SQ Por definição, os quadrados médios são obtidos dividindo-se as somas de quadrados pelos respectivos graus de liberdade, isto é: 2 Re Re n s SQ s QM g SQ g QM Re Re e e 2 2 2 2 1 2 Re Re n n n s E SQ s QM E 2 1 2 12 Re Re n i ix g E SQ g E QM FV GL SQ QM Fcalc Regressão 1 SQReg (V1) V1/V2 Resíduo n - 2 SQRes /(n -2) (V2) Total n - 1 SPDXY 1ˆ XY n i i SPD y 1 1 ˆ n i iy 1 ANOVA do modelo de Regressão Linear Simples 2 1 Re Re . V V s QM g QM Fcalc H0 : β1 = 0 Ha : β1 0 Ftab ; 1,GLRes. Continuando o exercício ... n i n i i i n Y Y SQTot 1 2 1 2 ,0 53211 12 ,3 0133 2888 ,1 2 SQTot SQDX g SQ 1ˆ2 Re SPDXY g SQ 1ˆ Re ,0 52402 ,0 0000336 Re 2 465522717,4 g SQ ou ,0 52402 ,0 0000336 Re 1086418133,1 g SQ ANÁLISE DE VARIÂNCIA DA REGRESSÃO (ANOVA) FV GL SQ QM Fcalc Regressão 1 0,52402 0,52402 647,94** Resíduo 10 0,00809 0,00081 Total 11 0,53211 10,04 1 %; 1 1 0 gl e Ftab SQRes = SQTot - SQReg SQRes = 0,53211 – 0,52402 = 0,00809 - O teste F: Interpretação: De acordo com este teste, rejeita- se H0, isto é, as variações ocorridas no volume podem ser explicadas pela variável combinada D2H, em nível de 1% de probabilidade. - Coeficiente de Determinação (r2): 98,48 0,53211100 0,52402 2 % r Interpretação: A equação ajustada explica 98,48% das variações ocorridas no volume. Interpretação: o erro médio associado ao uso da equação ajustada é de 11,33%. - Erro padrão das estimativa (Sy.x): Interpretação: o erro médio associado ao uso da equação ajustada é de 0,02844 m3. m3 0,00081 0,02844 QMResíduo SY.X - Erro padrão relativo(Sy.x(%)): 11,33 ,0 25111100 ,0 02844 % . SY X O cálculo das variâncias e covariâncias ... 2 1 2 1 2 0ˆ ˆ s x n X V n i i n i i n i ix s V 1 2 2 1ˆ ˆ 4, 465522717 1 2 n i ix 1, 1086418133 1 2 n i i X ,0 00081 Re 2 s QM s X 7193,14 ,0 0001575288 ,0 00081 *465522717 4, 12 10864181331, ˆ ˆ 0 V ,0 0000000000017 4, 465522717 ,0 00081 ˆ ˆ 1 V 𝑐𝑜𝑣 𝛽 0, 𝛽 1 = − 7193,14 ∗ 0,00081 465522717,4 = − 0,0000000125 𝑣𝑎𝑟 − 𝑐𝑜𝑣 = 0,0001575288 − 0,0000000125 − 0,0000000125 0,0000000000017
Envie sua pergunta para a IA e receba a resposta na hora
Recomendado para você
7
Exercícios Estatística Exp 2021 2
Estatística Experimental
UFES
80
Slide - Testes de Hipóteses - 2023-1
Estatística Experimental
UFES
34
Slide - Análise de Regressão - 2023-1
Estatística Experimental
UFES
6
Lista - Fatorial Parcelas Subdivididas Correlação e Regressão - Estatística Experimental 2023-1
Estatística Experimental
UFES
68
Slide - Regressão Linear Múltipla - 2023-1
Estatística Experimental
UFES
3
Lista 6 - Métodos Estatísticos 1 - 2023-1
Estatística Experimental
UFES
Texto de pré-visualização
CAPÍTULO VII – ANÁLISE DE REGRESSÃO Prof. Gilson Fernandes da Silva Departamento de Ciências Florestais e da Madeira (DCFM) Programa de Pós-graduação em Ciências Florestais (PGCF) Universidade Federal Espírito Santo (UFES) 8. INTRODUÇÃO À ANÁLISE DE REGRESSÃO O modelo de regressão definido como um modelo estatístico, difere em conceito de um modelo matemático por apresentar um termo denominado erro aleatório. Modelo Matemático Modelo de regressão X Y 1 0 X Y 1 0 0 1 2 3 4 5 6 7 0 2 4 6 8 X Y 0 2 4 6 8 10 12 0 2 4 6 8 X Y Modelo Matemático Modelo de regressão X Y 1 0 X Y 1 0 9. REGRESSÃO LINEAR SIMPLES Dados n pares de valores de duas variáveis Xi e Yi (i = 1, 2, ..., n), admitindo que Y é função linear de X, pode-se estabelecer uma regressão linear simples, cujo modelo estatístico é: em que β0 e β1 são os parâmetros do modelo e εi e são os erros aleatórios. O coeficiente angular da reta (β1) é também denominado coeficiente de regressão e o coeficiente linear da reta (β0) é também conhecido como termo constante da equação de regressão. i i i X Y 1 0 Pressuposições do modelo de Regressão linear simples 1) A relação entre X e Y é linear nos parâmetros. 2) Os valores de X são fixos, isto é, X é não estocástico. 3) A média do erro é nula, isto é, E(εi) = 0. 4) Para um dado valor de X, a variância do erro é sempre σ2, denominada variância residual, isto é, V(εi) = σ2. Pode-se dizer então que o erro é homocedástico ou que há homocedasticia (do erro ou da variável dependente). 𝑬 𝜺𝒊 𝟐 = 𝝈𝟐 ou E[Yi - E(Yi / Xi )]2 = σ2 5) O erro de uma observação é independente do erro em outra observação, isto é, E(εi εj) = 0 para i j. 6) Os erro têm distribuição normal. Combinando as pressuposições 3), 4), 5) e 6) tem- se que: εi ~ NID(0, σ2), em que NID significa Normal e Independentemente Distribuído. Deve-se verificar, entretanto, se o número de observações disponíveis é maior do que o número de parâmetros da equação de regressão. Para se ajustar uma regressão linear simples é preciso ter no mínimo 3 observações. Caso se disponha apenas de dua observações, a determinação da reta é um problema de geometria analítica, não é possível, neste caso, fazer nenhuma análise estatística. O método de Mínimos Quadrados Ordinários (MQO) Este é um método de estimação muito empregado para estimar parâmetros de modelos de regressão. Seu objetivo principal consiste em minimizar a soma de quadrados dos erros, tal como será demonstrado a seguir: Yi = 0 + 1Xi + i (1) i = Yi - 0 - 1Xi (2) (3) n i i i n i i X Y 1 2 1 0 1 2 0 1 2 1 1 0 0 1 2 n i i i n i i X Y 0 2 1 1 0 1 1 2 i n i i i n i i X X Y (4) (5) Dividindo-se (4) e (5) por -2, tem-se: n i i i i n i i i X X Y X Y 1 1 0 1 1 0 0 ˆ ˆ 0 ˆ ˆ n i n i i n i i i i n i n i i i X X Y X X n Y 1 1 2 1 1 0 1 1 1 0 0 ˆ 0 ˆ ˆ n i n i i n i i i i n i n i i i X X Y X X n Y 1 1 2 1 1 0 1 1 1 0 0 ˆ 0 ˆ ˆ (6) (7) n X n Y n i i n i i 1 1 1 0 ˆ ˆ X Y 1 0 ˆ ˆ (8) Sistema de Equações Normais (SEN) Substituindo (8) em (7) e desenvolvendo a expressão, tem-se: n X X n Y X Y X n i i n i i n i i n i i n i i i 2 1 1 2 1 1 1 1ˆ (9) X Y 1 0 ˆ ˆ ˆ e Duas relações muito úteis podem ser obtidas a partir do SEN. Para se desenvolver a primeira é preciso lembrar que: (10) i i i Y Y e ˆ i i X Y 1 0 ˆ ˆ ˆ n i i i n i i X Y e 1 1 0 1 ˆ ˆ n i i n i i n i i X n Y e 1 1 0 1 1 ˆ ˆ n i i n i i n i i n i i n i i X n X n Y n Y e 1 1 1 1 1 1 1 ˆ ˆ 0 ˆ ˆ 1 1 1 1 1 1 1 n i i n i i n i i n i i n i i X X Y Y e De modo que: A segunda relação diz respeito a: 0 ˆ ˆ 1 1 0 n i i i i X X Y 0 ˆ ˆ 1 2 1 1 0 1 n i i n i i n i i i X X X Y 0 ˆ ˆ 1 2 1 1 1 1 1 1 n i i n i i n i i n i i n i i i X X n X n Y X Y 0 ˆ ˆ 1 2 1 2 1 1 1 1 1 n i i n i i n i i n i i n i i i X n X n Y X X Y (11) 0 ˆ 1 2 1 2 1 n i n i i i XY n X X SPD 0 ˆ ˆ 1 1 1 0 X X XY XY n i i i n i i i i SQD SQD SPD SPD X e X X Y 0 1 n i iei X Concluindo-se que: Pode-se demonstrar também que: Como já demonstrado em (10) e (11): (12) 0 ˆ ˆ ˆ 1 1 0 1 n i i i n i i i X e Ye 0 ˆ ˆ ˆ 1 1 1 0 1 n i i i n i i n i i i X e e Ye 0 1 n i ie e 0 1 n i iei X De onde se deduz que: 0 ˆ 1 n i Yiei Outra relação útil pode ser derivada como se segue: Como demonstrado em (10), a soma dos erros é igual a zero. Então, temos: i i i e X Y 1 0 ˆ ˆ i i i e Y Y ˆ n i i n i i n i i i n i i e Y e Y Y 1 1 1 1 ˆ ˆ n i i n i i Y Y 1 1 ˆ ou Y Y ˆ Exemplo de aplicação: (Soares et al, 2007) Árv D(cm) H(m) v(m3) D2H = X X2 XY Y2 1 8,0 9,7 0,0274 620,8 385392,6 17,0 0,0008 2 27,7 27,6 0,7159 21177,2 448473969,3 15160,8 0,5125 3 23,2 26,5 0,5505 14263,4 203443438,5 7852,0 0,3031 4 17,7 17,4 0,178 5451,2 29716083,0 970,3 0,0317 5 13,8 12,9 0,1003 2456,7 6035257,0 246,4 0,0101 6 17,0 16,5 0,1852 4768,5 22738592,3 883,1 0,0343 7 18,8 20,3 0,2423 7174,8 51478214,2 1738,5 0,0587 8 8,0 11,6 0,0327 742,4 551157,8 24,3 0,0011 9 15,0 16,7 0,1292 3757,5 14118806,3 485,5 0,0167 10 21,6 21,2 0,3542 9891,1 97833305,3 3503,4 0,1255 11 11,0 12,8 0,0608 1548,8 2398781,4 94,2 0,0037 12 24,2 24,7 0,4368 14465,3 209245135,5 6318,4 0,1908 Soma 3,0133 86317,7 1086418133,1 37293,8 1,2888 Exemplo de ajuste do modelo de Spurr (1952) D H v 2 1 0 X Y 1 0 n X X n Y X Y X n i i n i i n i i n i i n i i i 2 1 1 2 1 1 1 1ˆ 0000336 ,0 12 86317 7, 1, 1086418133 12 ,3 0133 86317 7, 8, 37293 ˆ 2 1 X Y 1 0 ˆ ˆ ,0 0097713 ,0 0000336 71931, ,0 2511 ˆ 0 D H v 2 ,0 0000336 ,0 0097713 ˆ ANÁLISE DE VARIÂNCIA DA REGRESSÃO (ANOVA) FV GL SQ QM Fcalc Regressão 1 0,52402 0,52402 647,94** Resíduo 10 0,00809 0,00081 Total 11 0,53211 10,04 1 %; 1 1 0 gl e Ftab O modelo simplificado Uma simplificação conveniente dos cálculos é obtida quando se usa a variável centrada, isto é: 𝑥𝑖 = 𝑋𝑖 − 𝑋 Na representação gráfica, isso corresponde a tomar a média da variável Xi como origem do eixo das abscissas. Substituindo a variável independente original pela variável centrada, o modelo estatístico fica: i i i x Y 1 0 (13) (14) Admitindo que , o SEN agora ficará: 0 1 n i ix n i n i i n i i i i n i n i i i x x Y x x n Y 1 1 2 1 1 0 1 1 1 0 0 ˆ ˆ 0 ˆ ˆ n i n i i i i n i i x Y x n Y 1 1 2 1 1 0 ˆ ˆ n i i n i i i x Y x 1 2 1 1ˆ n Y n i i 1 0ˆ (15) (16) i i i i x y ou x Y Y 1 1 ˆ ˆ ˆ ˆ A equação estimada para o modelo simplificado será então: Propriedades dos estimadores do modelo de regressão Os estimadores obtidos pelo método de Mínimos Quadrados são não tendenciosos. Para que isso seja verdade, é necessário demonstrar que: 1 0 ˆ ˆ e 1 1 0 0 ˆ ˆ e E E O caso de 1ˆ Como foi demonstrado por meio do SEN para a variável centrada na média (xi), temos que: Vamos admitir que: De onde vem que: n i iYi k 1 1ˆ n n n n n n i i n i i i Y x x x x Y x x x x Y x x x x x Y x 2 2 1 2 2 2 1 2 1 2 2 1 1 1 2 1 1ˆ 2 2 1 2 2 1 2 2 2 1 1 1 2 n n n n n i i i i x x x x x x x x x x x x x x k (17) Admitindo que os valores de Xi são fixos (pressuposição 2), temos: Como i i i X Y 1 0 tem-se que: n i i i i n i i i X k Y k 1 1 0 1 1ˆ n i i i n i i i n i i k k X k 1 1 1 1 0 1ˆ n i i i n i i i n i i k k X k E E 1 1 1 1 0 1ˆ n i i i n i i i n i i k E k X k E 1 1 1 1 0 1ˆ (19) (18) Entretanto, sabe-se que: (21) 0 1 2 1 1 n i i n i i n i i x x k Admitindo que E(i) = 0, temos: n i i i n i i k X k E 1 1 1 0 1ˆ (20) Prova: nX X X X x n i n i i i n i i 1 1 1 Então: nX X n X X n i i n i i 1 1 mas, 0 1 n i ix n i i n i n i i i n i i n i i i i n i i n i i n i i i x X X X x X X X X x x X k 1 2 1 1 2 1 2 1 1 2 1 1 (22) Do mesmo modo que: n X X X n X X X X X n i i n i i n i i n i i n i i n i i n i i 2 1 1 2 1 1 1 2 1 1 2 Mas, (23) Contudo, n i i i n i i n i i X X X X X X x 1 2 2 1 2 1 2 2 n i n i i i n i i nX X X X x 1 2 1 2 1 2 2 n i n i i n i i i n i i n X n n X X x 1 2 2 1 2 1 2 1 2 2 n i n i i i n i i n i i n X X X X x 1 2 1 2 1 2 1 2 (24) A expressão (19) permite concluir que o estimador é um estimador não tendencioso do parâmetro β1 . 1 1ˆ E (26) Substituindo (25) e (21) em (20), temos: Comparando as expressões (23) e (24) e sua relação com a expressão (22), concluímos que: 1 1 n i kiXi (25) O caso de 0ˆ X Y 1 0 ˆ ˆ 1 1 0 ˆ ˆ ˆ XE E Y X E Y E Admitindo que X é uma variável fixa, temos: Mas, foi demonstrado que: 1 1ˆ E Então, temos que: 1 1 0 1 1 ˆ X E Y n X Y n E E n i i n i i Como i i i X Y 1 0 , tem-se que: 1 1 1 0 0 1 ˆ X X E n E n i i i 1 1 1 0 0 1 1 ˆ X X n n n E n i i 1 1 1 0 0 1 ˆ X E X n E n i i i 0 1 0 0ˆ n X n X E n i i n i i (27) 0 0ˆ E A expressão (27) permite concluir que o estimador é um estimador não tendencioso do parâmetro β0, Isto é: 0ˆ Variâncias dos estimadores dos parâmetros A variância de pode ser definida como: 1ˆ Foi demonstrado que: n i i i n i i i n i i k k X k 1 1 1 1 0 1ˆ (veja expressão 25) 0 1 n i ik 1 1 n i iXi k (veja expressão 18) (veja expressão 21) (28) 2 1 1 1 ˆ ˆ ˆ E E V 2 1 1 1 ˆ ˆ E V Substituindo (21) e (25) em (18), temos: n i i ik 1 1 1ˆ Mas, também foi definido( expressão 17) que: n i i i i x x k 1 2 e, portanto: n i i n i i i x x 1 2 1 1 1ˆ (29) Substituindo a expressão (29) na (28), vem que: 2 1 2 2 1 1ˆ n i i n i i i x x E V Mas, por meio de produtos notáveis, pode-se demonstrar que: 1 1 1 1 2 1 2 1 2 2 2 2 2 1 2 1 2 1 2 2 2 n n n n n i i i x x x x x x x E x x E Expressão (30) Sabe-se, contudo, que, de acordo com as pressuposições (2), (4) e (5), temos que: - Pressuposição (2): é fixo. - Pressuposição (4): - Pressuposição (5): E(εiεj) = 0 A expressão (30), de acordo com estas pressuposições, pode agora ser reescrita como: n i i n n i i i x x x x x E 1 2 2 2 2 2 2 2 2 2 1 2 1 2 ix 𝐸 𝜀𝑖 2 = 𝜎2 (31) Finalmente, pode-se concluir que: n i i n i i n i i x x x V 1 2 2 2 1 2 1 2 2 1ˆ ou n i ix s V 1 2 2 1ˆ ˆ Em que: s2 = Quadrado médio do resíduo da ANOVA da regressão (assista as próximas aulas!!!). Mas, sabe-se que (expressões 8 e 16): 2 0 0 0 ˆ ˆ ˆ E E V 2 0 0 0 ˆ ˆ E V A variância de pode ser definida como: 0ˆ (32) X x Y x n Y X Y n i i n i i i n i i 1 2 1 1 1 0 ˆ ˆ i n i n i i i Y X x x n 1 1 2 0 1 ˆ De modo que pode ser escrito como: 0ˆ Como i i i X Y 1 0 tem-se que: i i n i n i i i X X x x n 1 0 1 1 2 0 1 ˆ n i i n i i i n i i n i i i n i i n i i n i i n i i n i x x X x X x X x x X n n X n 1 2 1 1 2 1 1 1 2 1 0 1 1 1 1 0 0ˆ Na demonstração da expressão (21), tem-se que: O que simplifica a expressão anterior para: (33) 0 1 n i ix n i i n i i i n i i n i i i n i i x x X x X x X n X 1 2 1 1 2 1 1 1 1 0 0ˆ Por outro lado, pode-se demonstrar que: n i n i i i n i i i i n i i X X X X X X X X X 1 1 2 1 2 1 n i n i i i i n i i n X X X x 1 2 1 2 1 n i n i i n i i i i n i i X n X X X X X 1 1 1 2 1 i n i i i n i i X X X x X 1 1 (34) De acordo com o demonstrado para a expressão (24): n i n i i i n i i n X X x 1 2 1 2 1 2 De modo que substituindo as expressões (24) e (34) na expressão (33), concluímos que: n i i i n i i x X x 1 2 1 e, portanto, n i i n i i i n i i x x X X n X 1 2 1 1 1 1 0 0ˆ n i i n i i i n i i x x X n 1 2 1 1 0 0ˆ Mas, de acordo com a expressão 17, temos: n i i i i x x k 1 2 n i i i n i i k X n 1 1 0 0ˆ (35) Substituindo a expressão (35) na (32), temos: n i i i n i i i Xk n E Xk n E V 1 2 2 2 0 1 0 0 1 1 ˆ i n i X ik n 1 0 0 1 ˆ n i i i E Xk n V 1 2 2 0 1 ˆ (36) n i i i i n Xk X k n Xk n 1 2 2 2 2 2 1 1 (37) Substituindo a expressão (37) na (36), temos: n i i i i E n Xk X k n V 1 2 2 2 2 0 2 1 ˆ 𝐸 𝜀𝑖 2 = 𝜎2 De acordo com a pressuposição (4), temos: Então: 2 2 2 2 2 2 2 2 2 2 2 1 2 1 2 2 0 2 1 2 1 2 1 ˆ n Xk X k n n Xk X k n n Xk X k n V n n n i i i n i i i n Xk X k n n Xk X k n V 1 2 2 2 2 2 2 1 2 2 2 2 0 2 2 1 ˆ n k X k X n V n i i n i i 1 2 1 2 2 2 2 0 2 ˆ 0 1 2 1 1 n i i n i i n i i x x k n i ik X n V 1 2 2 2 2 0ˆ Mas, de acordo com a expressão (21), sabe-se que: E assim temos: (38) A expressão (38) pode ser reescrita como: n i i n i i k X n k X n V 1 2 2 2 1 2 2 2 2 0 1 ˆ n i i n i i k X n n k X n n V 1 2 2 2 1 2 2 2 0 1 1 ˆ n i i i i x x k 1 2 Sendo (expressão (17) 2 1 2 2 2 n i i i i x x k (39) n i i n i i n i i n i i x x x k 1 2 2 1 2 1 2 1 2 1 (40) Substituindo a expressão (40) na (39), temos: n i ix X n V 1 2 2 2 0 1 ˆ Mas, pode-se demonstrar que: (41) n i i n i i n i i x n nX x x X n 1 2 2 1 2 1 2 2 1 Mas, segundo a expressão (24): n i n i i i n i i n X X x 1 2 1 2 1 2 De onde vem que: n i i n i i n i i n i i n i i n i i n i i x n X x n n X n n X X x X n 1 2 1 2 1 2 2 2 1 2 1 1 2 1 2 2 1 (42) Assim, tem-se: 2 1 2 1 2 0ˆ n i i n i i x n X V ou 2 1 2 1 2 0ˆ ˆ s x n X V n i i n i i Substituindo a expressão (41) na (40), temos: (43) Covariância das estimativas dos parâmetros A covariância entre entre as estimativas dos parâmetros pode ser definida como: Do SEN pode-se obter que: X Y 1 0 ˆ ˆ Substituindo esta expressão na anterior, vem que: 1 0 1 1 1 0 ˆ ˆ cov ˆ , ˆ X Y E 1 0 1 0 1 1 0 0 1 0 ˆ ˆ ˆ ˆ ˆ ˆ ˆ ˆ cov ˆ , ˆ E E E E E E Contudo, sabe-se que: 1 0 2 1 1 1 0 1 1 1 0 ˆ ˆ ˆ ˆ cov ˆ , ˆ X E Y X Y E 1 0 2 1 1 1 0 ˆ ˆ cov ˆ , ˆ XE Y E n Y Y n i i 1 e 2 1 1 2 1 2 1 2 1 1 ˆ ˆ ˆ ˆ ˆ ˆ E V E E E V Assim, temos que: 1 0 2 1 1 1 1 1 0 ˆ ˆ ˆ 1 cov ˆ , ˆ E X V Y n E n i i 1 0 2 1 1 2 2 1 1 1 0 1 0 ˆ 1 cov ˆ , ˆ n i i n i i i X X X X E n 1 0 2 1 1 2 2 1 1 1 1 1 0 1 0 ˆ ˆ ˆ 1 cov ˆ , ˆ X X X X X E n n i i n i i i 1 0 2 1 1 2 2 1 1 2 1 1 0 1 0 ˆ 1 cov ˆ , ˆ X X X X E X n n i i n i i i 1 0 2 1 1 2 2 1 1 2 1 1 0 1 0 ˆ 1 cov ˆ , ˆ X X X X E n X n i i n i i n i i n i i X X X E n 1 2 2 1 1 1 0 ˆ 1 cov ˆ , ˆ (44) Nesse momento, vamos analisar a expressão . Primeiro é preciso relembrar a expressão (16), ou seja: n i i n i i i x Y x 1 2 1 1ˆ i 1ˆ E i n i i n i i i i x Y x 1 2 1 1ˆ Considerando e desenvolvendo os somatórios, temos: i i i X Y 1 0 Note que ao se fazer o produto entre o i-ésimo erro e o estimador de β1, teremos produtos cruzados entre os erros. Para distinguir estes cruzamentos, vamos denominar o índice relativo ao estimador de β1 de j e o índice relativo a ε de i. Assim, o somatório desdobrado pode ser reescrito como: n n n n n n n n n i n i i n i i i Y x x x x Y x x x x Y x x x x Y x x x x x Y x 2 2 1 1 2 2 1 1 1 2 2 1 1 2 2 1 1 1 2 1 1ˆ 2 2 1 1 0 1 2 2 1 1 1 1 0 1 2 2 1 1 1 0 2 2 1 1 1 1 1 0 1 1 2 1 1ˆ n n n n n n n n n n n i n i i n i i i x x x X x x x x X x x x x X x x x x X x x Y x i n i j n i j j j i x X x 1 2 1 1 0 1ˆ Mas, da expressão (21) demonstrou-se que: i j j n i i n i j i X x x E E 1 0 1 2 1 1ˆ j i j i i n i i n i j i X E x x E 1 0 1 2 1 1ˆ (45) 0 1 1 n i j n i i x x Assim, de acordo com a expressão (45), não importa se i = j ou i j, temos que: 0 ˆ 1 i E Substituindo esta identidade na expressão (44) , temos que: n i i n i i x X X X X 1 2 2 1 2 2 0 ˆ1 cov ˆ , ou RESUMO 2 1 2 1 2 0ˆ ˆ s x n X V n i i n i i n i ix s V 1 2 2 ˆ 1ˆ DECOMPOSIÇÃO DA SOMA DE QUADRADOS Considere a seguinte identidade: i i i i Y Y Y Y Y Y ˆ ˆ n i i n i i n i i e y y 1 2 1 2 1 2 ˆ Pode-se demonstrar que: n i i i n i i n i i Y Y Y Y Y Y 1 2 1 2 1 2 ˆ ˆ ou Da identidade anterior, pode-se concluir que a soma de quadrados do total (SQTot) é igual a soma de quadrados da regressão (SQReg) mais a soma de quadrados do erro, do resíduo ou dos desvios (SQRes). n i i i i n i i Y Y Y Y Y Y 1 2 2 1 ˆ ˆ i i n i i n i i i n i i n i i Y Y Y Y Y Y Y Y Y Y ˆ ˆ 2 ˆ ˆ 1 1 2 1 2 2 1 i n i i n i i i n i i n i i Y e Y Y Y Y Y Y Y 1 1 2 1 2 2 1 ˆ 2 ˆ ˆ n i i i n i i n i i Y Y Y Y Y Y 1 2 1 2 1 2 ˆ ˆ n i n i i i i n i i i n i i n i i e Y Y e Y Y Y Y Y Y 1 1 1 2 1 2 1 2 ˆ 2 ˆ ˆ Isto é, SQTot = SQReg + SQRes . 1 2 1 2 2 1 SQTot n Y Y Y Y n i n i i i n i i C n Y n i i 2 1 Correção para a média Tomando-se como base as expressões (10) e (12), vem que: n i i n i i Y X Y Y g SQ 1 2 1 0 1 2 ˆ ˆ ˆ Re n i i n i i X X Y X X Y g SQ 1 2 1 1 1 2 1 1 ˆ ˆ ˆ ˆ Re X n i i n i i SQD x X X g SQ 2 1 1 2 2 1 1 2 12 ˆ ˆ ˆ Re A SOMA DE QUADRADOS DA REGRESSÃO ou X XY n i n i i i n i i n i i n i i i SQD SPD n X X n Y X Y X g SQ 2 1 2 1 2 2 1 1 1 Re n i i n i i n i i i n i i n i i n i i i n i i x x y x x x y x x g SQ 1 2 2 1 2 2 1 1 2 2 1 2 1 1 2 1ˆ2 Re ou XY n i i i n i i i n i i n i i i SPD x y y x x y x g SQ 1 1 1 1 1 2 1 ˆ ˆ Re Em resumo, temos: X XY SQD SPD g SQ 2 Re ou SQDX g SQ 1ˆ2 Re SPDXY g SQ 1ˆ Re ou A soma de quadrados do resíduo usualmente é obtida por diferença, isto é: SQRes = SQTot - SQReg O COEFICIENTE DE DETERMINAÇÃO (r2) O coeficiente de determinação pode ser interpretado como: • A proporção da variação de Y que é “explicada” pela regressão ou • Quanto da SQTot é “explicada”pela regressão. Y X XY SQD SQD SPD r Y X XY SQD SQD SPD r 2 2 Mas, foi demonstrado que: X XY SQD SPD g SQ 2 Re e SQDY SQTot Portanto: SQTot g SQ r Re 2 ESPERANÇAS DAS SOMAS DE QUADRADOS Vamos começar pela esperança da soma de quadrados da regressão. Sabe-se que: i i i X Y 1 0 n Y Y n i i 1 e Portanto, X n X Y n i i i 1 0 1 1 0 (46) Subtraindo a expressão (46) de Yi, temos que: X X Y Y i i i 1 0 1 0 i i i x y 1 2 1 1 1 2 2 1 1 2 1 1 Re n i i i i n i i n i i i n i i x x x y x x g SQ 2 1 1 1 2 1 1 2 1 Re n i i n i i i n i i n i i x x x x g SQ (48) (47) Mas, de acordo com o demonstrado para a expressão (21), temos que: 0 1 n i ix O que reduz a expressão (48) a: 2 1 1 2 1 1 2 1 Re n i i i n i i n i i x x x g SQ Desenvolvendo o produto notável, temos: n i n i i i i n i i i n i i n i i x x x x x g SQ 1 1 2 1 2 1 2 1 2 2 1 1 2 2 1 Re (49) Assumindo que x é uma variável fixa e aplicando esperança a expressão (49), teremos: n i i i n i i n i i i n i i n i i x E x x E x x g SQ E 1 1 2 2 1 1 2 1 2 2 1 1 1 Re Como já demonstrado (veja expressão 31): n i i n i i i x x E 1 2 2 2 1 (50) Do mesmo modo que: 0 2 2 1 1 1 1 n n n i i i n i i i x E x E x E E x x E De modo que: fixa E(i) = 0, pressuposição (3) 2 1 2 12 Re n i ix g SQ E (51) A seguir será demonstrado como se obter a esperança da soma de quadrados do total. n i i n i i y Y Y SQTot 1 2 2 1 i i i x y 1 De acordo com a expressão (50): n i i x SQTot 1 2 1 1 n i i i i i x x SQTot 1 1 2 2 12 2 n i i i n i i n i i x x SQTot 1 1 1 2 1 2 12 2 Aplicando esperança a SQTot, teremos: (52) n i i i i n i i n i i x x E E x SQTot E 1 1 1 2 1 2 12 2 Mas, pode-se demonstrar que: 0 1 1 1 n i i n i i i n i i i i x x E x x E Pois, de acordo com as expressões (21) e (51), temos: 0 1 n i ix e 0 1 n i ix i E (53) mas, 1 2 2 2 2 1 1 2 1 2 n n n E E n i i n i i n i i Assim, a expressão (53) pode ser reescrita como: n i i n i i E x SQTot E 1 2 1 2 12 Assim, temos que: 2 1 2 12 1 n x SQTot E n i i n E E n E n i i n i i n i i n i i 2 1 1 2 2 1 1 2 Para compreender melhor a dedução anterior, é interessante notar que: 2 2 2 1 2 1 2 1 2 n n i i n i i E E E E E De acordo com a pressuposição (4), 2 2 i E Então, 2 2 2 2 1 2 n E n i i Por outro lado, 2 2 1 2 1 2 1 1 1 n n i i n i i n E n E n E 1 1 1 2 1 2 2 2 2 1 2 2 1 2 2 2 2 1 1 n n n n n n n E n E 2 2 i E Mas, de acordo com as pressuposições (4) e (5), (4) e 0 i j E 2 2 2 1 1 1 n n E n n i i (5) SQRes = SQTot - SQReg Falta obter a esperança da soma de quadrados de resíduos. E[SQRes] = E[SQTot] – E[SQReg] 2 1 2 2 1 2 1 2 12 1 Re n i i n i i x n x s SQ E 2 2 Re s n E SQ Por definição, os quadrados médios são obtidos dividindo-se as somas de quadrados pelos respectivos graus de liberdade, isto é: 2 Re Re n s SQ s QM g SQ g QM Re Re e e 2 2 2 2 1 2 Re Re n n n s E SQ s QM E 2 1 2 12 Re Re n i ix g E SQ g E QM FV GL SQ QM Fcalc Regressão 1 SQReg (V1) V1/V2 Resíduo n - 2 SQRes /(n -2) (V2) Total n - 1 SPDXY 1ˆ XY n i i SPD y 1 1 ˆ n i iy 1 ANOVA do modelo de Regressão Linear Simples 2 1 Re Re . V V s QM g QM Fcalc H0 : β1 = 0 Ha : β1 0 Ftab ; 1,GLRes. Continuando o exercício ... n i n i i i n Y Y SQTot 1 2 1 2 ,0 53211 12 ,3 0133 2888 ,1 2 SQTot SQDX g SQ 1ˆ2 Re SPDXY g SQ 1ˆ Re ,0 52402 ,0 0000336 Re 2 465522717,4 g SQ ou ,0 52402 ,0 0000336 Re 1086418133,1 g SQ ANÁLISE DE VARIÂNCIA DA REGRESSÃO (ANOVA) FV GL SQ QM Fcalc Regressão 1 0,52402 0,52402 647,94** Resíduo 10 0,00809 0,00081 Total 11 0,53211 10,04 1 %; 1 1 0 gl e Ftab SQRes = SQTot - SQReg SQRes = 0,53211 – 0,52402 = 0,00809 - O teste F: Interpretação: De acordo com este teste, rejeita- se H0, isto é, as variações ocorridas no volume podem ser explicadas pela variável combinada D2H, em nível de 1% de probabilidade. - Coeficiente de Determinação (r2): 98,48 0,53211100 0,52402 2 % r Interpretação: A equação ajustada explica 98,48% das variações ocorridas no volume. Interpretação: o erro médio associado ao uso da equação ajustada é de 11,33%. - Erro padrão das estimativa (Sy.x): Interpretação: o erro médio associado ao uso da equação ajustada é de 0,02844 m3. m3 0,00081 0,02844 QMResíduo SY.X - Erro padrão relativo(Sy.x(%)): 11,33 ,0 25111100 ,0 02844 % . SY X O cálculo das variâncias e covariâncias ... 2 1 2 1 2 0ˆ ˆ s x n X V n i i n i i n i ix s V 1 2 2 1ˆ ˆ 4, 465522717 1 2 n i ix 1, 1086418133 1 2 n i i X ,0 00081 Re 2 s QM s X 7193,14 ,0 0001575288 ,0 00081 *465522717 4, 12 10864181331, ˆ ˆ 0 V ,0 0000000000017 4, 465522717 ,0 00081 ˆ ˆ 1 V 𝑐𝑜𝑣 𝛽 0, 𝛽 1 = − 7193,14 ∗ 0,00081 465522717,4 = − 0,0000000125 𝑣𝑎𝑟 − 𝑐𝑜𝑣 = 0,0001575288 − 0,0000000125 − 0,0000000125 0,0000000000017