·
Engenharia Eletrônica ·
Sinais e Sistemas
Envie sua pergunta para a IA e receba a resposta na hora
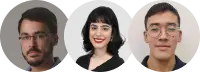
Prefere sua atividade resolvida por um tutor especialista?
- Receba resolvida até o seu prazo
- Converse com o tutor pelo chat
- Garantia de 7 dias contra erros
Recomendado para você
592
Sinais e Sistemas - 2ª Edição
Sinais e Sistemas
CEFET/RJ
30
Princípios de Telecomunicações: Aula 5 - Escala Logarítmica e Decibéis
Sinais e Sistemas
CEFET/RJ
1
Cálculo da Energia dos Sinais
Sinais e Sistemas
CEFET/RJ
1
Referências Bibliográficas
Sinais e Sistemas
CEFET/RJ
55
Princípios de Telecomunicações - Aulas 2 e 3
Sinais e Sistemas
CEFET/RJ
526
Schaum's Outline of Signals and Systems
Sinais e Sistemas
CEFET/RJ
794
Signals and Systems Analysis Using Transform Methods and MATLAB
Sinais e Sistemas
CEFET/RJ
1
Referências de Exercícios e Questões
Sinais e Sistemas
CEFET/RJ
31
Princípios de Telecomunicações - Aula 7: Transformada de Fourier
Sinais e Sistemas
CEFET/RJ
853
Sinais e Sistemas Lineares - B.P. Lathi
Sinais e Sistemas
CEFET/RJ
Texto de pré-visualização
THIRD EDITION LINEAR SYSTEMS AND SIGNALS BP Lathi ROGER GREEN OXFORD UNIVERSITY PRESS 00LathiPrelims 2017928 943 page i 1 LINEAR SYSTEMS AND SIGNALS 00LathiPrelims 2017928 943 page ii 2 T H E O X F O R D S E R I E S I N E L E C T R I C A L AND COMPUTER ENGINEERING Adel S Sedra Series Editor Allen and Holberg CMOS Analog Circuit Design 3rd edition Boncelet Probability Statistics and Random Signals Bobrow Elementary Linear Circuit Analysis 2nd edition Bobrow Fundamentals of Electrical Engineering 2nd edition Campbell Fabrication Engineering at the Micro and Nanoscale 4th edition Chen Digital Signal Processing Chen Linear System Theory and Design 4th edition Chen Signals and Systems 3rd edition Comer Digital Logic and State Machine Design 3rd edition Comer MicroprocessorBased System Design Cooper and McGillem Probabilistic Methods of Signal and System Analysis 3rd edition Dimitrijev Principles of Semiconductor Device 2nd edition Dimitrijev Understanding Semiconductor Devices Fortney Principles of Electronics Analog Digital Franco Electric Circuits Fundamentals Ghausi Electronic Devices and Circuits Discrete and Integrated Guru and Hiziroglu Electric Machinery and Transformers 3rd edition Houts Signal Analysis in Linear Systems Jones Introduction to Optical Fiber Communication Systems Krein Elements of Power Electronics 2nd Edition Kuo Digital Control Systems 3rd edition Lathi and Green Linear Systems and Signals 3rd edition Lathi and Ding Modern Digital and Analog Communication Systems 5th edition Lathi Signal Processing and Linear Systems Martin Digital Integrated Circuit Design Miner Lines and Electromagnetic Fields for Engineers Mitra Signals and Systems Parhami Computer Architecture Parhami Computer Arithmetic 2nd edition Roberts and Sedra SPICE 2nd edition Roberts Taenzler and Burns An Introduction to MixedSignal IC Test and Measurement 2nd edition Roulston An Introduction to the Physics of Semiconductor Devices Sadiku Elements of Electromagnetics 7th edition Santina Stubberud and Hostetter Digital Control System Design 2nd edition Sarma Introduction to Electrical Engineering Schaumann Xiao and Van Valkenburg Design of Analog Filters 3rd edition Schwarz and Oldham Electrical Engineering An Introduction 2nd edition Sedra and Smith Microelectronic Circuits 7th edition Stefani Shahian Savant and Hostetter Design of Feedback Control Systems 4th edition Tsividis Operation and Modeling of the MOS Transistor 3rd edition Van Valkenburg Analog Filter Design Warner and Grung Semiconductor Device Electronics Wolovich Automatic Control Systems Yariv and Yeh Photonics Optical Electronics in Modern Communications 6th edition Zak Systems and Control 00LathiPrelims 2017928 943 page iii 3 LINEAR SYSTEMS AND SIGNALS THIRD EDITION B P Lathi and R A Green New York Oxford OXFORD UNIVERSITY PRESS 2018 00LathiPrelims 2017928 943 page iv 4 Oxford University Press is a department of the University of Oxford It furthers the Universitys objective of excellence in research scholarship and education by publishing worldwide Oxford New York Auckland Cape Town Dar es Salaam Hong Kong Karachi Kuala Lumpur Madrid Melbourne Mexico City Nairobi New Delhi Shanghai Taipei Toronto With offices in Argentina Austria Brazil Chile Czech Republic France Greece Guatemala Hungary Italy Japan Poland Portugal Singapore South Korea Switzerland Thailand Turkey Ukraine Vietnam Copyright c 2018 by Oxford University Press For titles covered by Section 112 of the US Higher Education Opportunity Act please visit wwwoupcomushe for the latest information about pricing and alternate formats Published by Oxford University Press 198 Madison Avenue New York NY 10016 httpwwwoupcom Oxford is a registered trademark of Oxford University Press All rights reserved No part of this publication may be reproduced stored in a retrieval system or transmitted in any form or by any means electronic mechanical photocopying recording or otherwise without the prior permission of Oxford University Press Library of Congress CataloginginPublication Data Names Lathi B P Bhagwandas Pannalal author Green R A Roger A author Title Linear systems and signals BP Lathi and RA Green Description Third Edition New York Oxford University Press 2018 Series The Oxford Series in Electrical and Computer Engineering Identifiers LCCN 2017034962 ISBN 9780190200176 hardcover acidfree paper Subjects LCSH Signal processingMathematics System analysis Linear time invariant systems Digital filters Mathematics Classification LCC TK51025 L298 2017 DDC 6213822dc23 LC record available at httpslccnlocgov2017034962 ISBN 9780190200176 Printing number 9 8 7 6 5 4 3 2 1 Printed by RR Donnelly in the United States of America 00LathiPrelims 2017928 943 page v 5 CONTENTS PREFACE xv B BACKGROUND B1 Complex Numbers 1 B11 A Historical Note 1 B12 Algebra of Complex Numbers 5 B2 Sinusoids 16 B21 Addition of Sinusoids 18 B22 Sinusoids in Terms of Exponentials 20 B3 Sketching Signals 20 B31 Monotonic Exponentials 20 B32 The Exponentially Varying Sinusoid 22 B4 Cramers Rule 23 B5 Partial Fraction Expansion 25 B51 Method of Clearing Fractions 26 B52 The Heaviside CoverUp Method 27 B53 Repeated Factors of Qx 31 B54 A Combination of Heaviside CoverUp and Clearing Fractions 32 B55 Improper Fx with m n 34 B56 Modified Partial Fractions 35 B6 Vectors and Matrices 36 B61 Some Definitions and Properties 37 B62 Matrix Algebra 38 B7 MATLAB Elementary Operations 42 B71 MATLAB Overview 42 B72 Calculator Operations 43 B73 Vector Operations 45 B74 Simple Plotting 46 B75 ElementbyElement Operations 48 B76 Matrix Operations 49 B77 Partial Fraction Expansions 53 B8 Appendix Useful Mathematical Formulas 54 B81 Some Useful Constants 54 v 00LathiPrelims 2017928 943 page vi 6 vi Contents B82 Complex Numbers 54 B83 Sums 54 B84 Taylor and Maclaurin Series 55 B85 Power Series 55 B86 Trigonometric Identities 55 B87 Common Derivative Formulas 56 B88 Indefinite Integrals 57 B89 LHôpitals Rule 58 B810 Solution of Quadratic and Cubic Equations 58 References 58 Problems 59 1 SIGNALS AND SYSTEMS 11 Size of a Signal 64 111 Signal Energy 65 112 Signal Power 65 12 Some Useful Signal Operations 71 121 Time Shifting 71 122 Time Scaling 73 123 Time Reversal 76 124 Combined Operations 77 13 Classification of Signals 78 131 ContinuousTime and DiscreteTime Signals 78 132 Analog and Digital Signals 78 133 Periodic and Aperiodic Signals 79 134 Energy and Power Signals 82 135 Deterministic and Random Signals 82 14 Some Useful Signal Models 82 141 The Unit Step Function ut 83 142 The Unit Impulse Function δt 86 143 The Exponential Function est 89 15 Even and Odd Functions 92 151 Some Properties of Even and Odd Functions 92 152 Even and Odd Components of a Signal 93 16 Systems 95 17 Classification of Systems 97 171 Linear and Nonlinear Systems 97 172 TimeInvariant and TimeVarying Systems 102 173 Instantaneous and Dynamic Systems 103 174 Causal and Noncausal Systems 104 175 ContinuousTime and DiscreteTime Systems 107 176 Analog and Digital Systems 109 177 Invertible and Noninvertible Systems 109 178 Stable and Unstable Systems 110 00LathiPrelims 2017928 943 page vii 7 Contents vii 18 System Model InputOutput Description 111 181 Electrical Systems 111 182 Mechanical Systems 114 183 Electromechanical Systems 118 19 Internal and External Descriptions of a System 119 110 Internal Description The StateSpace Description 121 111 MATLAB Working with Functions 126 1111 Anonymous Functions 126 1112 Relational Operators and the Unit Step Function 128 1113 Visualizing Operations on the Independent Variable 130 1114 Numerical Integration and Estimating Signal Energy 131 112 Summary 133 References 135 Problems 136 2 TIMEDOMAIN ANALYSIS OF CONTINUOUSTIME SYSTEMS 21 Introduction 150 22 System Response to Internal Conditions The ZeroInput Response 151 221 Some Insights into the ZeroInput Behavior of a System 161 23 The Unit Impulse Response ht 163 24 System Response to External Input The ZeroState Response 168 241 The Convolution Integral 170 242 Graphical Understanding of Convolution Operation 178 243 Interconnected Systems 190 244 A Very Special Function for LTIC Systems The Everlasting Exponential est 193 245 Total Response 195 25 System Stability 196 251 External BIBO Stability 196 252 Internal Asymptotic Stability 198 253 Relationship Between BIBO and Asymptotic Stability 199 26 Intuitive Insights into System Behavior 203 261 Dependence of System Behavior on Characteristic Modes 203 262 Response Time of a System The System Time Constant 205 263 Time Constant and Rise Time of a System 206 264 Time Constant and Filtering 207 265 Time Constant and Pulse Dispersion Spreading 209 266 Time Constant and Rate of Information Transmission 209 267 The Resonance Phenomenon 210 27 MATLAB MFiles 212 271 Script MFiles 213 272 Function MFiles 214 3 TIMEDOMAIN ANALYSIS OF DISCRETETIME SYSTEMS 237 31 Introduction 237 311 Size of a DiscreteTime Signal 238 32 Useful Signal Operations 240 33 Some Useful DiscreteTime Signal Models 245 331 DiscreteTime Impulse Function δn 245 332 DiscreteTime Unit Step Function un 246 333 DiscreteTime Exponential γn 247 334 DiscreteTime Sinusoid cosΩn θ 251 335 DiscreteTime Complex Exponential eγn 252 34 Examples of DiscreteTime Systems 253 341 Classification of DiscreteTime Systems 262 35 DiscreteTime System Equations 265 351 Recursive Iterative Solution of Difference Equation 266 36 System Response to Internal Conditions The ZeroInput Response 270 37 The Unit Impulse Response hn 277 371 The ClosedForm Solution of hn 278 38 System Response to External Input The ZeroState Response 280 381 Graphical Procedure for the Convolution Sum 288 382 Interconnected Systems 294 383 Total Response 297 39 System Stability 298 391 External BIBO Stability 298 392 Internal Asymptotic Stability 299 393 Relationship Between BIBO and Asymptotic Stability 301 310 Intuitive Insights into System Behavior 305 311 MATLAB DiscreteTime Signals and Systems 306 3111 DiscreteTime Functions and Stem Plots 306 3112 System Responses Through Filtering 308 3113 A Custom Filter Function 310 3114 DiscreteTime Convolution 311 312 Appendix Impulse Response for a Special Case 313 313 Summary 313 Problems 314 00LathiPrelims 2017928 943 page ix 9 Contents ix 4 CONTINUOUSTIME SYSTEM ANALYSIS USING THE LAPLACE TRANSFORM 41 The Laplace Transform 330 411 Finding the Inverse Transform 338 42 Some Properties of the Laplace Transform 349 421 Time Shifting 349 422 Frequency Shifting 353 423 The TimeDifferentiation Property 354 424 The TimeIntegration Property 356 425 The Scaling Property 357 426 Time Convolution and Frequency Convolution 357 43 Solution of Differential and IntegroDifferential Equations 360 431 Comments on Initial Conditions at 0 and at 0 363 432 ZeroState Response 366 433 Stability 371 434 Inverse Systems 373 44 Analysis of Electrical Networks The Transformed Network 373 441 Analysis of Active Circuits 382 45 Block Diagrams 386 46 System Realization 388 461 Direct Form I Realization 389 462 Direct Form II Realization 390 463 Cascade and Parallel Realizations 393 464 Transposed Realization 396 465 Using Operational Amplifiers for System Realization 399 47 Application to Feedback and Controls 404 471 Analysis of a Simple Control System 406 48 Frequency Response of an LTIC System 412 481 SteadyState Response to Causal Sinusoidal Inputs 418 49 Bode Plots 419 491 Constant Ka1a2b1b3 422 492 Pole or Zero at the Origin 422 493 FirstOrder Pole or Zero 424 494 SecondOrder Pole or Zero 426 495 The Transfer Function from the Frequency Response 435 410 Filter Design by Placement of Poles and Zeros of Hs 436 4101 Dependence of Frequency Response on Poles and Zeros of Hs 436 4102 Lowpass Filters 439 4103 Bandpass Filters 441 4104 Notch Bandstop Filters 441 4105 Practical Filters and Their Specifications 444 411 The Bilateral Laplace Transform 445 00LathiPrelims 2017928 943 page x 10 x Contents 4111 Properties of the Bilateral Laplace Transform 451 4112 Using the Bilateral Transform for Linear System Analysis 452 412 MATLAB ContinuousTime Filters 455 4121 Frequency Response and Polynomial Evaluation 456 4122 Butterworth Filters and the Find Command 459 4123 Using Cascaded SecondOrder Sections for Butterworth Filter Realization 461 4124 Chebyshev Filters 463 413 Summary 466 References 468 Problems 468 5 DISCRETETIME SYSTEM ANALYSIS USING THE zTRANSFORM 51 The zTransform 488 511 Inverse Transform by Partial Fraction Expansion and Tables 495 512 Inverse zTransform by Power Series Expansion 499 52 Some Properties of the zTransform 501 521 TimeShifting Properties 501 522 zDomain Scaling Property Multiplication by γ n 505 523 zDomain Differentiation Property Multiplication by n 506 524 TimeReversal Property 506 525 Convolution Property 507 53 zTransform Solution of Linear Difference Equations 510 531 ZeroState Response of LTID Systems The Transfer Function 514 532 Stability 518 533 Inverse Systems 519 54 System Realization 519 55 Frequency Response of DiscreteTime Systems 526 551 The Periodic Nature of Frequency Response 532 552 Aliasing and Sampling Rate 536 56 Frequency Response from PoleZero Locations 538 57 Digital Processing of Analog Signals 547 58 The Bilateral zTransform 554 581 Properties of the Bilateral zTransform 559 582 Using the Bilateral zTransform for Analysis of LTID Systems 560 59 Connecting the Laplace and zTransforms 563 510 MATLAB DiscreteTime IIR Filters 565 5101 Frequency Response and PoleZero Plots 566 5102 Transformation Basics 567 5103 Transformation by FirstOrder Backward Difference 568 5104 Bilinear Transformation 569 5105 Bilinear Transformation with Prewarping 570 5106 Example Butterworth Filter Transformation 571 00LathiPrelims 2017928 943 page xi 11 Contents xi 5107 Problems Finding Polynomial Roots 572 5108 Using Cascaded SecondOrder Sections to Improve Design 572 511 Summary 574 References 575 Problems 575 6 CONTINUOUSTIME SIGNAL ANALYSIS THE FOURIER SERIES 61 Periodic Signal Representation by Trigonometric Fourier Series 593 611 The Fourier Spectrum 598 612 The Effect of Symmetry 607 613 Determining the Fundamental Frequency and Period 609 62 Existence and Convergence of the Fourier Series 612 621 Convergence of a Series 613 622 The Role of Amplitude and Phase Spectra in Waveshaping 615 63 Exponential Fourier Series 621 631 Exponential Fourier Spectra 624 632 Parsevals Theorem 632 633 Properties of the Fourier Series 635 64 LTIC System Response to Periodic Inputs 637 65 Generalized Fourier Series Signals as Vectors 641 651 Component of a Vector 642 652 Signal Comparison and Component of a Signal 643 653 Extension to Complex Signals 645 654 Signal Representation by an Orthogonal Signal Set 647 66 Numerical Computation of Dn 659 67 MATLAB Fourier Series Applications 661 671 Periodic Functions and the Gibbs Phenomenon 661 672 Optimization and Phase Spectra 664 68 Summary 667 References 668 Problems 669 7 CONTINUOUSTIME SIGNAL ANALYSIS THE FOURIER TRANSFORM 71 Aperiodic Signal Representation by the Fourier Integral 680 711 Physical Appreciation of the Fourier Transform 687 72 Transforms of Some Useful Functions 689 721 Connection Between the Fourier and Laplace Transforms 700 73 Some Properties of the Fourier Transform 701 74 Signal Transmission Through LTIC Systems 721 741 Signal Distortion During Transmission 723 742 Bandpass Systems and Group Delay 726 00LathiPrelims 2017928 943 page xii 12 xii Contents 75 Ideal and Practical Filters 730 76 Signal Energy 733 77 Application to Communications Amplitude Modulation 736 771 DoubleSideband SuppressedCarrier DSBSC Modulation 737 772 Amplitude Modulation AM 742 773 SingleSideband Modulation SSB 746 774 FrequencyDivision Multiplexing 749 78 Data Truncation Window Functions 749 781 Using Windows in Filter Design 755 79 MATLAB Fourier Transform Topics 755 791 The Sinc Function and the Scaling Property 757 792 Parsevals Theorem and Essential Bandwidth 758 793 Spectral Sampling 759 794 Kaiser Window Functions 760 710 Summary 762 References 763 Problems 764 8 SAMPLING THE BRIDGE FROM CONTINUOUS TO DISCRETE 81 The Sampling Theorem 776 811 Practical Sampling 781 82 Signal Reconstruction 785 821 Practical Difficulties in Signal Reconstruction 788 822 Some Applications of the Sampling Theorem 796 83 AnalogtoDigital AD Conversion 799 84 Dual of Time Sampling Spectral Sampling 802 85 Numerical Computation of the Fourier Transform The Discrete Fourier Transform 805 851 Some Properties of the DFT 818 852 Some Applications of the DFT 820 86 The Fast Fourier Transform FFT 824 87 MATLAB The Discrete Fourier Transform 827 871 Computing the Discrete Fourier Transform 827 872 Improving the Picture with Zero Padding 829 873 Quantization 831 88 Summary 834 References 835 Problems 835 00LathiPrelims 2017928 943 page xiii 13 Contents xiii 9 FOURIER ANALYSIS OF DISCRETETIME SIGNALS 91 DiscreteTime Fourier Series DTFS 845 911 Periodic Signal Representation by DiscreteTime Fourier Series 846 912 Fourier Spectra of a Periodic Signal xn 848 92 Aperiodic Signal Representation by Fourier Integral 855 921 Nature of Fourier Spectra 858 922 Connection Between the DTFT and the zTransform 866 93 Properties of the DTFT 867 94 LTI DiscreteTime System Analysis by DTFT 878 941 Distortionless Transmission 880 942 Ideal and Practical Filters 882 95 DTFT Connection with the CTFT 883 951 Use of DFT and FFT for Numerical Computation of the DTFT 885 96 Generalization of the DTFT to the zTransform 886 97 MATLAB Working with the DTFS and the DTFT 889 971 Computing the DiscreteTime Fourier Series 889 972 Measuring Code Performance 891 973 FIR Filter Design by Frequency Sampling 892 98 Summary 898 Reference 898 Problems 899 10 STATESPACE ANALYSIS 101 Mathematical Preliminaries 909 1011 Derivatives and Integrals of a Matrix 909 1012 The Characteristic Equation of a Matrix The CayleyHamilton Theorem 910 1013 Computation of an Exponential and a Power of a Matrix 912 102 Introduction to State Space 913 103 A Systematic Procedure to Determine State Equations 916 1031 Electrical Circuits 916 1032 State Equations from a Transfer Function 919 104 Solution of State Equations 926 1041 Laplace Transform Solution of State Equations 927 1042 TimeDomain Solution of State Equations 933 105 Linear Transformation of a State Vector 939 1051 Diagonalization of Matrix A 943 106 Controllability and Observability 947 1061 Inadequacy of the Transfer Function Description of a System 953 00LathiPrelims 2017928 943 page xiv 14 xiv Contents 107 StateSpace Analysis of DiscreteTime Systems 953 1071 Solution in State Space 955 1072 The zTransform Solution 959 108 MATLAB Toolboxes and StateSpace Analysis 961 1081 zTransform Solutions to DiscreteTime StateSpace Systems 961 1082 Transfer Functions from StateSpace Representations 964 1083 Controllability and Observability of DiscreteTime Systems 965 1084 Matrix Exponentiation and the Matrix Exponential 968 109 Summary 969 References 970 Problems 970 INDEX 975 00LathiPrelims 2017928 943 page xv 15 PREFACE This book Linear Systems and Signals presents a comprehensive treatment of signals and linear systems at an introductory level Following our preferred style it emphasizes a physical appreciation of concepts through heuristic reasoning and the use of metaphors analogies and creative explanations Such an approach is much different from a purely deductive technique that uses mere mathematical manipulation of symbols There is a temptation to treat engineering subjects as a branch of applied mathematics Such an approach is a perfect match to the public image of engineering as a dry and dull discipline It ignores the physical meaning behind various derivations and deprives students of intuitive grasp and the enjoyable experience of logical uncovering of the subject matter In this book we use mathematics not so much to prove axiomatic theory as to support and enhance physical and intuitive understanding Wherever possible theoretical results are interpreted heuristically and are enhanced by carefully chosen examples and analogies This third edition which closely follows the organization of the second edition has been refined in many ways Discussions are streamlined adding or trimming material as needed Equation example and section labeling is simplified and improved Computer examples are fully updated to reflect the most current version of MATLAB Hundreds of added problems provide new opportunities to learn and understand topics We have taken special care to improve the text without the topic creep and bloat that commonly occurs with each new edition of a text NOTABLE FEATURES The notable features of this book include the following 1 Intuitive and heuristic understanding of the concepts and physical meaning of mathematical results are emphasized throughout Such an approach not only leads to deeper appreciation and easier comprehension of the concepts but also makes learning enjoyable for students 2 Often students lack an adequate background in basic material such as complex numbers sinusoids handsketching of functions Cramers rule partial fraction expansion and matrix algebra We include a background chapter that addresses these basic and pervasive topics in electrical engineering Response by students has been unanimously enthusiastic 3 There are hundreds of worked examples in addition to drills usually with answers for students to test their understanding Additionally there are over 900 endofchapter problems of varying difficulty 4 Modern electrical engineering practice requires the use of computer calculation and simulation most often using the software package MATLAB Thus we integrate xv 00LathiPrelims 2017928 943 page xvi 16 xvi Preface MATLAB into many of the worked examples throughout the book Additionally each chapter concludes with a section devoted to learning and using MATLAB in the context and support of book topics Problem sets also contain numerous computer problems 5 The discretetime and continuoustime systems may be treated in sequence or they may be integrated by using a parallel approach 6 The summary at the end of each chapter proves helpful to students in summing up essential developments in the chapter 7 There are several historical notes to enhance students interest in the subject This information introduces students to the historical background that influenced the development of electrical engineering ORGANIZATION The book may be conceived as divided into five parts 1 Introduction Chs B and 1 2 Timedomain analysis of linear timeinvariant LTI systems Chs 2 and 3 3 Frequencydomain transform analysis of LTI systems Chs 4 and 5 4 Signal analysis Chs 6 7 8 and 9 5 Statespace analysis of LTI systems Ch 10 The organization of the book permits much flexibility in teaching the continuoustime and discretetime concepts The natural sequence of chapters is meant to integrate continuoustime and discretetime analysis It is also possible to use a sequential approach in which all the continuoustime analysis is covered first Chs 1 2 4 6 7 and 8 followed by discretetime analysis Chs 3 5 and 9 SUGGESTIONS FOR USING THIS BOOK The book can be readily tailored for a variety of courses spanning 30 to 45 lecture hours Most of the material in the first eight chapters can be covered at a brisk pace in about 45 hours The book can also be used for a 30lecturehour course by covering only analog material Chs 1 2 4 6 7 and possibly selected topics in Ch 8 Alternately one can also select Chs 1 to 5 for courses purely devoted to systems analysis or transform techniques To treat continuous and discretetime systems by using an integrated or parallel approach the appropriate sequence of chapters is 1 2 3 4 5 6 7 and 8 For a sequential approach where the continuoustime analysis is followed by discretetime analysis the proper chapter sequence is 1 2 4 6 7 8 3 5 and possibly 9 depending on the time available MATLAB MATLAB is a sophisticated language that serves as a powerful tool to better understand engineering topics including control theory filter design and of course linear systems and signals MATLABs flexible programming structure promotes rapid development and analysis Outstanding visualization capabilities provide unique insight into system behavior and signal character 00LathiPrelims 2017928 943 page xvii 17 Preface xvii As with any language learning MATLAB is incremental and requires practice This book provides two levels of exposure to MATLAB First MATLAB is integrated into many examples throughout the text to reinforce concepts and perform various computations These examples utilize standard MATLAB functions as well as functions from the control system signalprocessing and symbolic math toolboxes MATLAB has many more toolboxes available but these three are commonly available in most engineering departments A second and deeper level of exposure to MATLAB is achieved by concluding each chapter with a separate MATLAB section Taken together these eleven sections provide a selfcontained introduction to the MATLAB environment that allows even novice users to quickly gain MATLAB proficiency and competence These sessions provide detailed instruction on how to use MATLAB to solve problems in linear systems and signals Except for the very last chapter special care has been taken to avoid the use of toolbox functions in the MATLAB sessions Rather readers are shown the process of developing their own code In this way those readers without toolbox access are not at a disadvantage All of this books MATLAB code is available for download at the OUP companion website wwwoupcomuslathi CREDITS AND ACKNOWLEDGMENTS The portraits of Gauss Laplace Heaviside Fourier and Michelson have been reprinted courtesy of the Smithsonian Institution Libraries The likenesses of Cardano and Gibbs have been reprinted courtesy of the Library of Congress The engraving of Napoleon has been reprinted courtesy of BettmannCorbis The many fine cartoons throughout the text are the work of Joseph Coniglio a former student of Dr Lathi Many individuals have helped us in the preparation of this book as well as its earlier editions We are grateful to each and every one for helpful suggestions and comments Book writing is an obsessively timeconsuming activity which causes much hardship for an authors family We both are grateful to our families for their enormous but invisible sacrifices B P Lathi R A Green 00LathiPrelims 2017928 943 page xviii 18 LathiBackground 2017925 1553 page 1 1 C H A P T E R BACKGROUND B The topics discussed in this chapter are not entirely new to students taking this course You have already studied many of these topics in earlier courses or are expected to know them from your previous training Even so this background material deserves a review because it is so pervasive in the area of signals and systems Investing a little time in such a review will pay big dividends later Furthermore this material is useful not only for this course but also for several courses that follow It will also be helpful later as reference material in your professional career B1 COMPLEX NUMBERS Complex numbers are an extension of ordinary numbers and are an integral part of the modern number system Complex numbers particularly imaginary numbers sometimes seem mysterious and unreal This feeling of unreality derives from their unfamiliarity and novelty rather than their supposed nonexistence Mathematicians blundered in calling these numbers imaginary for the term immediately prejudices perception Had these numbers been called by some other name they would have become demystified long ago just as irrational numbers or negative numbers were Many futile attempts have been made to ascribe some physical meaning to imaginary numbers However this effort is needless In mathematics we assign symbols and operations any meaning we wish as long as internal consistency is maintained The history of mathematics is full of entities that were unfamiliar and held in abhorrence until familiarity made them acceptable This fact will become clear from the following historical note B11 A Historical Note Among early people the number system consisted only of natural numbers positive integers needed to express the number of children cattle and quivers of arrows These people had no need for fractions Whoever heard of two and onehalf children or three and onefourth cows However with the advent of agriculture people needed to measure continuously varying quantities such as the length of a field and the weight of a quantity of butter The number system therefore was extended to include fractions The ancient Egyptians and Babylonians knew how 1 LathiBackground 2017925 1553 page 2 2 2 CHAPTER B BACKGROUND to handle fractions but Pythagoras discovered that some numbers like the diagonal of a unit square could not be expressed as a whole number or a fraction Pythagoras a number mystic who regarded numbers as the essence and principle of all things in the universe was so appalled at his discovery that he swore his followers to secrecy and imposed a death penalty for divulging this secret 1 These numbers however were included in the number system by the time of Descartes and they are now known as irrational numbers Until recently negative numbers were not a part of the number system The concept of negative numbers must have appeared absurd to early man However the medieval Hindus had a clear understanding of the significance of positive and negative numbers 2 3 They were also the first to recognize the existence of absolute negative quantities 4 The works of Bhaskar 11141185 on arithmetic Lilavati and algebra Bijaganit not only use the decimal system but also give rules for dealing with negative quantities Bhaskar recognized that positive numbers have two square roots 5 Much later in Europe the men who developed the banking system that arose in Florence and Venice during the late Renaissance fifteenth century are credited with introducing a crude form of negative numbers The seemingly absurd subtraction of 7 from 5 seemed reasonable when bankers began to allow their clients to draw seven gold ducats while their deposit stood at five All that was necessary for this purpose was to write the difference 2 on the debit side of a ledger 6 Thus the number system was once again broadened generalized to include negative numbers The acceptance of negative numbers made it possible to solve equations such as x5 0 which had no solution before Yet for equations such as x2 1 0 leading to x2 1 the solution could not be found in the real number system It was therefore necessary to define a completely new kind of number with its square equal to 1 During the time of Descartes and Newton imaginary or complex numbers came to be accepted as part of the number system but they were still regarded as algebraic fiction The Swiss mathematician Leonhard Euler introduced the notation i for imaginary around 1777 to represent 1 Electrical engineers use the notation j instead of i to avoid confusion with the notation i often used for electrical current Thus j2 1 and 1 j This notation allows us to determine the square root of any negative number For example 4 4 1 2j When imaginary numbers are included in the number system the resulting numbers are called complex numbers ORIGINS OF COMPLEX NUMBERS Ironically and contrary to popular belief it was not the solution of a quadratic equation such as x2 1 0 but a cubic equation with real roots that made imaginary numbers plausible and acceptable to early mathematicians They could dismiss 1 as pure nonsense when it appeared as a solution to x2 1 0 because this equation has no real solution But in 1545 Gerolamo Cardano of Milan published Ars Magna The Great Art the most important algebraic work of the Renaissance In this book he gave a method of solving a general cubic equation in which a root of a negative number appeared in an intermediate step According to his method the solution to a thirdorder equation x³ ax b 0 is given by x b2 b²4 a³2713 b2 b²4 a³2713 For example to find a solution of x³ 6x 20 0 we substitute a 6 b 20 in the foregoing equation to obtain x 10 108 10 108 20392 0392 2 2 We can readily verify that 2 is indeed a solution of x³ 6x 20 0 But when Cardano tried to solve the equation x³ 15x 4 0 by this formula his solution was x 2 121 2 121 Therefore Cardanos formula gives x 2 j 2 j 4 We can readily verify that x 4 is indeed a solution of x³ 15x 4 0 Cardano tried to explain halfheartedly the presence of 121 but ultimately dismissed the whole enterprise as being as subtle as it is useless A generation later however Raphael Bombelli 15251573 after examining Cardanos results proposed acceptance of imaginary numbers as a necessary vehicle that would transport the mathematician from the real cubic equation to its real solution In other words although we begin and end with real numbers we seem compelled to move into an unfamiliar world of imaginaries to complete our journey To mathematicians of the day this proposal seemed incredibly strange 7 Yet they could not dismiss the idea of imaginary numbers so easily because this concept yielded the real solution of an equation It took two more centuries for the full importance of complex numbers to become evident in the works of Euler Gauss and Cauchy Still Bombelli deserves credit for recognizing that such numbers have a role to play in algebra 7 LathiBackground 2017925 1553 page 4 4 4 CHAPTER B BACKGROUND In 1799 the German mathematician Karl Friedrich Gauss at the ripe age of 22 proved the fundamental theorem of algebra namely that every algebraic equation in one unknown has a root in the form of a complex number He showed that every equation of the nth order has exactly n solutions roots no more and no less Gauss was also one of the first to give a coherent account of complex numbers and to interpret them as points in a complex plane It is he who introduced the term complex numbers and paved the way for their general and systematic use The number system was once again broadened or generalized to include imaginary numbers Ordinary or real numbers became a special case of generalized or complex numbers The utility of complex numbers can be understood readily by an analogy with two neighboring countries X and Y as illustrated in Fig B1 If we want to travel from City a to City b both in Gerolamo Cardano Karl Friedrich Gauss Country X Country Y a b A l t e r n a t e r o u t e Direct route Figure B1 Use of complex numbers can reduce the work B12 Algebra of Complex Numbers A complex number a b or a jb can be represented graphically by a point whose Cartesian coordinates are a b in a complex plane Fig B2 Let us denote this complex number by z so that z a jb B1 This representation is the Cartesian or rectangular form of complex number z The numbers a and b the abscissa and the ordinate of z are the real part and the imaginary part respectively of z They are also expressed as Re z a and Im z b Note that in this plane all real numbers lie on the horizontal axis and all imaginary numbers lie on the vertical axis Complex numbers may also be expressed in terms of polar coordinates If r θ are the polar coordinates of a point z a jb see Fig 2 then a r cos θ and b r sin θ Consequently z a jb r cos θ j r sin θ rcos θ jsin θ B2 Eulers formula states that eiθ cos θ jsin θ B3 To prove Eulers formula we use a Maclaurin series to expand eiθ cos θ and sin θ eiθ 1 jθ jθ² 2 jθ³ 3 jθ⁴ 4 jθ⁵ 5 jθ⁶ 6 1 jθ θ² 2 θ⁴ 4 θ⁶ 6 cos θ 1 θ² 2 θ⁴ 4 θ⁶ 6 sin θ θ θ³ 3 θ⁵ 5 Clearly it follows that eiθ cos θ jsin θ Using Eq B3 in Eq B2 yields z reiθ B4 This representation is the polar form of complex number z Summarizing a complex number can be expressed in rectangular form a jb or polar form reiθ with a rcos θ and b rsin θ θ tan1ba B5 Observe that r is the distance of the point z from the origin For this reason r is also called the magnitude or absolute value of z and is denoted by z Similarly θ is called the angle of z and is denoted by Lz Therefore we can also write polar form of Eq B4 as z zeiLz where z r and Lz θ Using polar form we see that the reciprocal of a complex number is given by 1 z 1 reiθ 1 r eiθ 1 z eiLz CONJUGATE OF A COMPLEX NUMBER We define z a jb reiθ zeiLz B6 The graphical representations of z a jb and its conjugate z are depicted in Fig B2 Observe that z is a mirror image of z about the horizontal axis To find the conjugate of any number we need only replace j with j in that number which is the same as changing the sign of its angle The sum of a complex number and its conjugate is a real number equal to twice the real part of the number z z a jb a jb 2a 2Re z Thus we see that the real part of complex number z can be computed as Re z z z 2 B7 Similarly the imaginary part of complex number z can be computed as Imz z z 2j The number 1 on the other hand is also at a unit distance from the origin but has an angle 0 more generally 0 plus any integer multiple of 2π For this reason it is advisable to draw the point in the complex plane and determine the quadrant in which the point lies This issue will be clarified by the following examples LathiBackground 2017925 1553 page 10 10 10 CHAPTER B BACKGROUND We can easily verify these results using the MATLAB abs and angle commands To obtain units of degrees we must multiply the radian result of the angle command by 180 π Furthermore the angle command correctly computes angles for all four quadrants of the complex plane To provide an example let us use MATLAB to verify that 2 j1 5ej1534 22361ej1534 abs21j ans 22361 angle21j180pi ans 1534349 One can also use the cart2pol command to convert Cartesian to polar coordinates Readers particularly those who are unfamiliar with MATLAB will benefit by reading the overview in Sec B7 EXAMPLE B2 Polar to Cartesian Form Represent the following numbers in the complex plane and express them in Cartesian form a 2ejπ3 b 4ej3π4 c 2ejπ2 d 3ej3π e 2ej4π and f 2ej4π a 2ejπ3 2cos π3 jsin π3 1 j 3 see Fig B5a b 4ej3π4 4cos 3π4 jsin 3π4 2 2 j2 2 see Fig B5b c 2ejπ2 2cos π2 jsin π2 20 j1 j2 see Fig B5c d 3ej3π 3cos 3π jsin 3π 31 j0 3 see Fig B5d e 2ej4π 2cos 4π jsin 4π 21 j0 2 see Fig B5e f 2ej4π 2cos 4π jsin 4π 21 j0 2 see Fig B5f We can readily verify these results using MATLAB First we use the exp function to represent a number in polar form Next we use the real and imag commands to determine the real and imaginary components of that number To provide an example let us use MATLAB to verify the result of part a 2ejπ3 1 j 3 1 j17321 real2exp1jpi3 ans 10000 imag2exp1jpi3 ans 17321 Since MATLAB defaults to Cartesian form we could have verified the entire result in one step 2exp1jpi3 ans 10000 17321i One can also use the pol2cart command to convert polar to Cartesian coordinates B1 Complex Numbers ARITHMETICAL OPERATIONS POWERS AND ROOTS OF COMPLEX NUMBERS To conveniently perform addition and subtraction complex numbers should be expressed in Cartesian form Thus if z₁ 3 j4 5e531 and z₂ 2 j3 13e563 then z₁ z₂ 3 j4 2 j3 5 j7 Division Cartesian Form z₁ 3 j4 z₂ 2 j3 To eliminate the complex number in the denominator we multiply both the numerator and the denominator of the righthand side by 2 j3 the denominators conjugate This yields z₁z₂ 3 j42 j32 j32 j3 18 j122 32 18 j113 1813 j113 Therefore 2z1 z2 22 j2 4 j3 22 4 j22 43 117 j41 b Xω 2 jω 3 j4ω 4 ω² etan¹ω2 9 16ω² etan¹ω3 4 ω² 9 16ω² etan¹ω2 tan¹ω3 LathiBackground 2017925 1553 page 16 16 16 CHAPTER B BACKGROUND B2 SINUSOIDS Consider the sinusoid xt Ccos2πf0t θ B13 We know that cos ϕ cosϕ 2nπ n 0123 Therefore cos ϕ repeats itself for every change of 2π in the angle ϕ For the sinusoid in Eq B13 the angle 2πf0tθ changes by 2π when t changes by 1f0 Clearly this sinusoid repeats every 1f0 seconds As a result there are f0 repetitions per second This is the frequency of the sinusoid and the repetition interval T0 given by T0 1 f0 B14 is the period For the sinusoid in Eq B13 C is the amplitude f0 is the frequency in hertz and θ is the phase Let us consider two special cases of this sinusoid when θ 0 and θ π2 as follows xt Ccos 2πf0t θ 0 and xt Ccos2πf0t π2 Csin 2πf0t θ π2 The angle or phase can be expressed in units of degrees or radians Although the radian is the proper unit in this book we shall often use the degree unit because students generally have a better feel for the relative magnitudes of angles expressed in degrees rather than in radians For example we relate better to the angle 24 than to 0419 radian Remember however when in doubt use the radian unit and above all be consistent In other words in a given problem or an expression do not mix the two units It is convenient to use the variable ω0 radian frequency to express 2πf0 ω0 2πf0 B15 With this notation the sinusoid in Eq B13 can be expressed as xt Ccosω0t θ in which the period T0 and frequency ω0 are given by see Eqs B14 and B15 T0 1 ω02π 2π ω0 and ω0 2π T0 Although we shall often refer to ω0 as the frequency of the signal cosω0tθ it should be clearly understood that ω0 is the radian frequency the hertzian frequency of this sinusoid is f0 ω02π The signals Ccos ω0t and Csin ω0t are illustrated in Figs B6a and B6b respectively A general sinusoid Ccosω0tθ can be readily sketched by shifting the signal Ccos ω0t in Fig B6a by the appropriate amount Consider for example xt Ccosω0t 60 This signal can be obtained by shifting delaying the signal C cosω0t Fig B6a to the right by a phase angle of 60 We know that a sinusoid undergoes a 360 change of phase or angle in one cycle A quartercycle segment corresponds to a 90 change of angle Alternatively if we advance C sinω0t by a quartercycle we obtain C cosω0t Therefore C sinω0t π2 C cosω0t These observations mean that sinω0t lags cosω0t by 90π2 radians and that cosω0t leads sinω0t by 90 a In this case a 1 and b 3 Using Eq B17 yields C 12 32 2 and θ tan131 60 Therefore xt 2cosω0t 60 We can verify this result by drawing phasors corresponding to the two sinusoids The sinusoid cosω0t is represented by a phasor of unit length at a zero angle with the horizontal The phasor sinω0t is represented by a unit phasor at an angle of 90 with the horizontal Therefore 3sinω0t is represented by a phasor of length 3 at 90 with the horizontal as depicted in Fig B8a Observe that tan134 tan134 531 Therefore xt 5cosω0t 1269 This result is readily verified in the phasor diagram in Fig B8b Alternately a jb 3 j4 5ej1269 a fact readily confirmed using MATLAB C abs34j C 5 theta angle34j180pi theta 1268699 Hence C 5 and θ 1268699 eat ut 1e approx 037 1e2 approx 0135 LathiBackground 2017925 1553 page 22 22 22 CHAPTER B BACKGROUND manner we see that xt 1e3 at t 15 and so on A knowledge of the values of xt at t 0 05 1 and 15 allows us to sketch the desired signal as shown in Fig B10b For a monotonically growing exponential eat the waveform increases by a factor e over each interval of 1a seconds B32 The Exponentially Varying Sinusoid We now discuss sketching an exponentially varying sinusoid xt Aeat cosω0t θ Let us consider a specific example xt 4e2t cos6t 60 We shall sketch 4e2t and cos6t 60 separately and then multiply them a Sketching 4e2t This monotonically decaying exponential has a time constant of 05 second and an initial value of 4 at t 0 Therefore its values at t 05 1 15 and 2 are 4e 4e2 4e3 and 4e4 or about 147 054 02 and 007 respectively Using these values as a guide we sketch 4e2t as illustrated in Fig B11a b Sketching cos6t 60 The procedure for sketching cos6t 60 is discussed in Sec B2 Fig B6c Here the period of the sinusoid is T0 2π6 1 and there is a phase delay of 60 or twothirds of a quartercycle which is equivalent to a delay of about 603601 16 seconds see Fig B11b c Sketching 4e2t cos6t 60 We now multiply the waveforms in steps a and b This multiplication amounts to forcing the sinusoid 4 cos6t 60 to decrease exponentially with a time constant of 05 The initial amplitude at t 0 is 4 decreasing to 4e 147 at t 05 to 147e054 at t 1 and so on This is depicted in Fig B11c Note that when cos6t 60 has a value of unity peak amplitude 4e2t cos6t 60 4e2t Therefore 4e2t cos6t60 touches 4e2t at the instants at which the sinusoid cos6t 60 is at its positive peaks Clearly 4e2t is an envelope for positive amplitudes of 4e2t cos6t 60 Similar argument shows that 4e2t cos6t 60 touches 4e2t at its negative peaks Therefore 4e2t is an envelope for negative amplitudes of 4e2t cos6t 60 Thus to sketch 4e2t cos6t 60 we first draw the envelopes 4e2t and 4e2t the mirror image of 4e2t about the horizontal axis and then sketch the sinusoid cos6t 60 with these envelopes acting as constraints on the sinusoids amplitude see Fig B11c In general Keat cosω0t θ can be sketched in this manner with Keat and Keat constraining the amplitude of cosω0t θ If we wish to refine the sketch further we could consider intervals of half the time constant over which the signal decays by a factor 1e Thus at t 025 xt 1e and at t 075 xt 1ee and so on Cramers rule offers a very convenient way to solve simultaneous linear equations in n unknowns x1 x2 ldots xn A beginvmatrix 2 1 1 1 3 1 1 1 1 endvmatrix 4 x₂ frac1A beginvmatrix 2 3 1 1 7 1 1 1 1 endvmatrix frac44 1 Fx frac2x3 9x2 11x 2x2 4x 3 2x 1 fracx 1x2 4x 3 k₁ 1 k₂ 2 k₃ 2 k₄ 3 EXAMPLE B9 Heaviside CoverUp Method Expand the following rational function Fx into partial fractions Fx 2x² 9x 11 x 1x 2x 3 k1 x 1 k2 x 2 k3 x 3 To determine k1 we let x 1 in x 1Fx Note that x 1Fx is obtained from Fx by omitting the term x 1 from its denominator Therefore to compute k1 corresponding to the factor x 1 we cover up the term x 1 in the denominator of Fx and then substitute x 1 in the remaining expression Mentally conceal the term x 1 in Fx with a finger and then let x 1 in the remaining expression The steps in covering up the function Fx are as follows Step 1 Cover up conceal the factor x 1 from Fx 2x² 9x 11 x 1x 2x 3 Step 2 Substitute x 1 in the remaining expression to obtain k1 k1 21² 91 111 121 3 2 9 11 0 2 2 186 3 Similarly to compute k2 we cover up the factor x 2 in Fx and let x 2 in the remaining function as follows k2 2x² 9x 11 x 1x 2x 3 x2 8 18 11 2 122 3 15 15 1 and k3 2x² 9x 11 x 1x 2x 3 x3 18 27 11 3 13 2 20 10 2 Therefore Fx 2x² 9x 11 x 1x 2x 3 3 x 1 1 x 2 2 x 3 COMPLEX FACTORS OF Qx The procedure just given works regardless of whether the factors of Qx are real or complex Consider for example Fx 4x² 2x 18 x 1x² 4x 13 k1 x 1 k2 x² 2 j3x² 2 j3 where k1 4x² 2x 18 x 1x² 4x 13 x2j3 2 Similarly k2 4x² 2x 18 x 1x² 2 j3x² 2 j3 x2j3 1 j2 5ej3π4 k3 4x² 2x 18 x 1x² 2 j3x² 2 j3 x2j3 1 j2 5ej3π4 Therefore Fx 2x 1 5ej3π4 x² 2 j3 5ej3π4 x² 2 j3 LathiBackground 2017925 1553 page 30 30 30 CHAPTER B BACKGROUND Equating terms of similar powers yields c1 2 c2 8 and 4x2 2x 18 x 1x2 4x 13 2 x 1 2x 8 x2 4x 13 SHORTCUTS The values of c1 and c2 in Eq B26 can also be determined by using shortcuts After computing k1 2 by the Heaviside method as before we let x 0 on both sides of Eq B26 to eliminate c1 This gives us 18 13 2 c2 13 c2 8 To determine c1 we multiply both sides of Eq B26 by x and then let x Remember that when x only the terms of the highest power are significant Therefore 4 2 c1 c1 2 In the procedure discussed here we let x 0 to determine c2 and then multiply both sides by x and let x to determine c1 However nothing is sacred about these values x 0 or x We use them because they reduce the number of computations involved We could just as well use other convenient values for x such as x 1 Consider the case Fx 2x2 4x 5 xx2 2x 5 k x c1x c2 x2 2x 5 We find k 1 by the Heaviside method in the usual manner As a result 2x2 4x 5 xx2 2x 5 1 x c1x c2 x2 2x 5 B27 If we try letting x 0 to determine c1 and c2 we obtain on both sides So let us choose x 1 This yields 11 8 1 c1 c2 8 or c1 c2 3 We can now choose some other value for x such as x 2 to obtain one more relationship to use in determining c1 and c2 In this case however a simple method is to multiply both sides of Eq B27 by x and then let x This yields 2 1 c1 c1 1 Since c1 c2 3 we see that c2 2 and therefore Fx 1 x x 2 x2 2x 5 B5 Repeated Factors of Qx If a function Fx has a repeated factor in its denominator it has the form Fx Px xλrxα1xα2 xαj Its partial fraction expansion is given by Fx a0 xλ a1 xλr ar1 xλ k1 xα1 k2 xα2 kj xαj B28 The coefficients k1 k2 kj corresponding to the unrepeated factors in this equation are determined by the Heaviside method as before Eq B24 To find the coefficients a0 a1 a2 ar1 we multiply both sides of Eq B28 by xλr This gives us xλrFx a0 a1xλ a2xλ² ar1xλr1 k1xλr xα1 k2xλr xα2 kjxλr xαj B29 If we let x λ on both sides of Eq B29 we obtain λλrFλ xλ a0 Therefore a0 is obtained by concealing the factor xλr in Fx and letting xλ in the remaining expression the Heaviside coverup method If we take the derivative with respect to x of both sides of Eq B29 the righthand side is a1 terms containing a factor xλ in their numerators Letting x λ on both sides of this equation we obtain ddx xλrFx xλ a1 Thus a1 is obtained by concealing the factor xλr in Fx taking the derivative of the remaining expression and then letting x λ Continuing in this manner we find aj 1j dj dxj xλrFx xλ B30 Observe that xλrFx is obtained from Fx by omitting the factor xλr from its denominator Therefore the coefficient aj is obtained by concealing the factor xλr in Fx taking the jth derivative of the remaining expression and then letting x λ while dividing by j Expand Fx into partial fractions if Fx 3x² 9x 20 x² x 6 3x² 9x 20 x 2x 3 Here m n 2 with b₂ 3 Therefore Fx 3x² 9x 20 x 2x 3 3 k₁ x 2 k₂ x 3 4x³ 16x² 23x 13 x 1³x 2 2 x 1³ a₁ x 1² a₂ x 1 x 2 There is only one unknown a₁ which can be readily found by setting x equal to any convenient value say x 0 This yields 13 2 2 a₁ 3 1 2 a₁ 1 in which k₁ 3x² 9x 20 x 2x 3 12 18 20 2 3 10 5 2 and k₂ 3x² 9x 20 x 2x 3 27 27 20 3 2 20 5 4 Therefore Fx 3x² 9x 20 x 2x 3 3 2 x 2 4 x 3 LathiBackground 2017925 1553 page 36 36 36 CHAPTER B BACKGROUND B6 VECTORS AND MATRICES An entity specified by n numbers in a certain order ordered ntuple is an ndimensional vector Thus an ordered ntuple x1 x2 xn represents an ndimensional vector x A vector may be represented as a row row vector x x1 x2 xn or as a column column vector x x1 x2 xn Simultaneous linear equations can be viewed as the transformation of one vector into another Consider for example the m simultaneous linear equations y1 a11x1 a12x2 a1nxn y2 a21x1 a22x2 a2nxn ym am1x1 am2x2 amnxn B31 If we define two column vectors x and y as x x1 x2 xn and y y1 y2 ym then Eq B31 may be viewed as the relationship or the function that transforms vector x into vector y Such a transformation is called a linear transformation of vectors To perform a linear transformation we need to define the array of coefficients aij appearing in Eq B31 This array is called a matrix and is denoted by A for convenience A a11 a12 a1n a21 a22 a2n am1 am2 amn A matrix with m rows and n columns is called a matrix of order mn or an m n matrix For the special case of m n the matrix is called a square matrix of order n It should be stressed at this point that a matrix is not a number such as a determinant but an array of numbers arranged in a particular order It is convenient to abbreviate the representation of matrix A with the form aijmn implying a matrix of order m n with aij as its ijth element In practice when the order m n is understood or need not be specified the notation can be A square matrix whose elements are zero everywhere except on the main diagonal is a diagonal matrix An example of a diagonal matrix is I 2 0 0 0 1 0 0 0 0 5 A diagonal matrix with unity for all its diagonal elements is called an identity matrix or a unit matrix denoted by I This is a square matrix LathiBackground 2017925 1553 page 38 38 38 CHAPTER B BACKGROUND Using the abbreviated notation if A aijmn then AT ajinm Intuitively further notice that ATT A B62 Matrix Algebra We shall now define matrix operations such as addition subtraction multiplication and division of matrices The definitions should be formulated so that they are useful in the manipulation of matrices ADDITION OF MATRICES For two matrices A and B both of the same order m n A a11 a12 a1n a21 a22 a2n am1 am2 amn and B b11 b12 b1n b21 b22 b2n bm1 bm2 bmn we define the sum A B as A B a11 b11 a12 b12 a1n b1n a21 b21 a22 b22 a2n b2n am1 bm1 am2 bm2 amn bmn or A B aij bijmn Note that two matrices can be added only if they are of the same order MULTIPLICATION OF A MATRIX BY A SCALAR We multiply a matrix A by a scalar c as follows cA c a11 a12 a1n a21 a22 a2n am1 am2 amn ca11 ca12 ca1n ca21 ca22 ca2n cam1 cam2 camn Ac Thus we also observe that the scalar c and the matrix A commute cA Ac MATRIX MULTIPLICATION We define the product AB C in which cij the element of C in the ith row and jth column is found by adding the products of the elements of A in the ith row multiplied by the corresponding elements of B in the jth column cij ai1bj1 ai2bj2 cdots ainbnj sumk1n aikbkj B33 This result is expressed as follows a11 a12 cdots a1n b1j b2j cdots bmj cij LathiBackground 2017925 1553 page 40 40 40 CHAPTER B BACKGROUND In the matrix product AB matrix A is said to be postmultiplied by B or matrix B is said to be premultiplied by A We may also verify the following relationships A BC AC BC CA B CA CB We can verify that any matrix A premultiplied or postmultiplied by the identity matrix I remains unchanged AI IA A Of course we must make sure that the order of I is such that the matrices are conformable for the corresponding product We give here without proof another important property of matrices AB AB where A and B represent determinants of matrices A and B MULTIPLICATION OF A MATRIX BY A VECTOR Consider Eq B32 which represents Eq B31 The righthand side of Eq B32 is a product of the mn matrix A and a vector x If for the time being we treat the vector x as if it were an n1 matrix then the product Ax according to the matrix multiplication rule yields the righthand side of Eq B31 Thus we may multiply a matrix by a vector by treating the vector as if it were an n 1 matrix Note that the constraint of conformability still applies Thus in this case xA is not defined and is meaningless MATRIX INVERSION To define the inverse of a matrix let us consider the set of equations represented by Eq B32 when m n y1 y2 yn a11 a12 a1n a21 a22 a2n an1 an2 ann x1 x2 xn B34 We can solve this set of equations for x1 x2 xn in terms of y1 y2 yn by using Cramers rule see Eq B21 This yields x1 x2 xn D11 A D21 A Dn1 A D12 A D22 A Dn2 A D1n A D2n A Dnn A y1 y2 yn B35 in which A is the determinant of the matrix A and Dij is the cofactor of element aij in the matrix A The cofactor of element aij is given by 1ij times the determinant of the LathiBackground 2017925 1553 page 41 41 B6 Vectors and Matrices 41 n 1 n 1 matrix that is obtained when the ith row and the jth column in matrix A are deleted We can express Eq B34 in compact matrix form as y Ax B36 We now define A1 the inverse of a square matrix A with the property A1A I unit matrix Then premultiplying both sides of Eq B36 by A1 we obtain A1y A1Ax Ix x or x A1y B37 A comparison of Eq B37 with Eq B35 shows that A1 1 A D11 D21 Dn1 D12 D22 Dn2 D1n D2n Dnn One of the conditions necessary for a unique solution of Eq B34 is that the number of equations must equal the number of unknowns This implies that the matrix A must be a square matrix In addition we observe from the solution as given in Eq B35 that if the solution is to exist A 0 Therefore the inverse exists only for a square matrix and only under the condition that the determinant of the matrix be nonzero A matrix whose determinant is nonzero is a nonsingular matrix Thus an inverse exists only for a nonsingular square matrix Since A1A I AA1 we further note that the matrices A and A1 commute The operation of matrix division can be accomplished through matrix inversion EXAMPLE B12 Computing the Inverse of a Matrix Let us find A1 if A 2 1 1 1 2 3 3 2 1 These two conditions imply that the number of equations is equal to the number of unknowns and that all the equations are independent To prove AA1 I notice first that we define A1A I Thus IA AI AA1A AA1A Subtracting AA1A we see that IA AA1A 0 or I AA1A 0 This requires AA1 I LathiBackground 2017925 1553 page 42 42 42 CHAPTER B BACKGROUND Here D11 4 D12 8 D13 4 D21 1 D22 1 D23 1 D31 1 D32 5 D33 3 and A 4 Therefore A1 1 4 4 1 1 8 1 5 4 1 3 B7 MATLAB ELEMENTARY OPERATIONS B71 MATLAB Overview Although MATLABl a registered trademark of The MathWorks Inc is easy to use it can be intimidating to new users Over the years MATLAB has evolved into a sophisticated computational package with thousands of functions and thousands of pages of documentation This section provides a brief introduction to the software environment When MATLAB is first launched its command window appears When MATLAB is ready to accept an instruction or input a command prompt is displayed in the command window Nearly all MATLAB activity is initiated at the command prompt Entering instructions at the command prompt generally results in the creation of an object or objects Many classes of objects are possible including functions and strings but usually objects are just data Objects are placed in what is called the MATLAB workspace If not visible the workspace can be viewed in a separate window by typing workspace at the command prompt The workspace provides important information about each object including the objects name size and class Another way to view the workspace is the whos command When whos is typed at the command prompt a summary of the workspace is printed in the command window The who command is a short version of whos that reports only the names of workspace objects Several functions exist to remove unnecessary data and help free system resources To remove specific variables from the workspace the clear command is typed followed by the names of the variables to be removed Just typing clear removes all objects from the workspace Additionally the clc command clears the command window and the clf command clears the current figure window Often important data and objects created in one session need to be saved for future use The save command followed by the desired filename saves the entire workspace to a file which has the mat extension It is also possible to selectively save objects by typing save followed by the filename and then the names of the objects to be saved The load command followed by the filename is used to load the data and objects contained in a MATLAB data file mat file Although MATLAB does not automatically save workspace data from one session to the next lines entered at the command prompt are recorded in the command history Previous command lines can be viewed copied and executed directly from the command history window From the LathiBackground 2017925 1553 page 43 43 B7 MATLAB Elementary Operations 43 command window pressing the up or down arrow key scrolls through previous commands and redisplays them at the command prompt Typing the first few characters and then pressing the arrow keys scrolls through the previous commands that start with the same characters The arrow keys allow command sequences to be repeated without retyping Perhaps the most important and useful command for new users is help To learn more about a function simply type help followed by the function name Helpful text is then displayed in the command window The obvious shortcoming of help is that the function name must first be known This is especially limiting for MATLAB beginners Fortunately help screens often conclude by referencing related or similar functions These references are an excellent way to learn new MATLAB commands Typing help help for example displays detailed information on the help command itself and also provides reference to relevant functions such as the lookfor command The lookfor command helps locate MATLAB functions based on a keyword search Simply type lookfor followed by a single keyword and MATLAB searches for functions that contain that keyword MATLAB also has comprehensive HTMLbased help The HTML help is accessed by using MATLABs integrated help browser which also functions as a standard web browser The HTML help facility includes a function and topic index as well as full textsearching capabilities Since HTML documents can contain graphics and special characters HTML help can provide more information than the commandline help After a little practice it is easy to find information in MATLAB When MATLAB graphics are created the print command can save figures in a common file format such as postscript encapsulated postscript JPEG or TIFF The format of displayed data such as the number of digits displayed is selected by using the format command MATLAB help provides the necessary details for both these functions When a MATLAB session is complete the exit command terminates MATLAB B72 Calculator Operations MATLAB can function as a simple calculator working as easily with complex numbers as with real numbers Scalar addition subtraction multiplication division and exponentiation are accomplished using the traditional operator symbols and Since MATLAB predefines i j 1 a complex constant is readily created using Cartesian coordinates For example z 34j z 30000 40000i assigns the complex constant 3 j4 to the variable z The real and imaginary components of z are extracted by using the real and imag operators In MATLAB the input to a function is placed parenthetically following the function name zreal realz zimag imagz When a command is terminated with a semicolon the statement is evaluated but the results are not displayed to the screen This feature is useful when one is computing intermediate results and it allows multiple instructions on a single line Although not displayed the results zreal 3 and zimag 4 are calculated and available for additional operations such as computing z There are many ways to compute the modulus or magnitude of a complex quantity Trigonometry confirms that z 3 j4 which corresponds to a 345 triangle has modulus z 13 j4 32 42 5 The MATLAB sqrt command provides one way to compute the required square root zmag sqrtzreal2 zimag2 zmag 5 In MATLAB most commands including sqrt accept inputs in a variety of forms including constants variables functions expressions and combinations thereof The same result is also obtained by computing z zz In this case complex conjugation is performed by using the conj command zmag sqrtzconjz zmag 5 More simply MATLAB computes absolute values directly by using the abs command zmag absz zmag 5 In addition to magnitude polar notation requires phase information The angle command provides the angle of a complex number zrad anglez zrad 22143 MATLAB expects and returns angles in a radian measure Angles expressed in degrees require an appropriate conversion factor zdeg anglez180pi zdeg 1268699 Notice MATLAB predefines the variable pi π It is also possible to obtain the angle of z using a twoargument arctangent function atan2 zrad atan2zimagzreal zrad 22143 Unlike a singleargument arctangent function the twoargument arctangent function ensures that the angle reflects the proper quadrant MATLAB supports a full complement of trigonometric functions standard trigonometric functions cos sin tan reciprocal trigonometric functions sec csc cot inverse trigonometric functions acos asin atan asec acsc acot and hyperbolic variations cosh sinh tanh csch coth acosh asinh atanh asec acsch and acoth Of course MATLAB comfortably supports complex arguments for any trigonometric function As with the angle command MATLAB trigonometric functions utilize units of radians The results can contradict what is often taught in introductory mathematics courses For example a common claim is that cosx 1 While this is true for real x it is not necessarily true for complex x This is readily verified by example using MATLAB and the cos function cos1j ans 15431 LathiBackground 2017925 1553 page 45 45 B7 MATLAB Elementary Operations 45 Problem B119 investigates these ideas further Similarly the claim that it is impossible to take the logarithm of a negative number is false For example the principal value of ln1 is jπ a fact easily verified by means of Eulers equation In MATLAB base10 and basee logarithms are computed by using the log10 and log commands respectively log1 ans 0 31416i B73 Vector Operations The power of MATLAB becomes apparent when vector arguments replace scalar arguments Rather than computing one value at a time a single expression computes many values Typically vectors are classified as row vectors or column vectors For now we consider the creation of row vectors with evenly spaced real elements To create such a vector the notation abc is used where a is the initial value b designates the step size and c is the termination value For example 0211 creates the length6 vector of evenvalued integers ranging from 0 to 10 k 0211 k 0 2 4 6 8 10 In this case the termination value does not appear as an element of the vector Negative and noninteger step sizes are also permissible k 111030 k 110000 76667 43333 10000 If a step size is not specified a value of 1 is assumed k 011 k 0 1 2 3 4 5 6 7 8 9 10 11 Vector notation provides the basis for solving a wide variety of problems For example consider finding the three cube roots of minus one w3 1 ejπ2πk for integer k Taking the cube root of each side yields w ejπ32πk3 To find the three unique solutions use any three consecutive integer values of k and MATLABs exp function k 02 w exp1jpi3 2pik3 w 05000 08660i 10000 00000i 05000 08660i The solutions particularly w 1 are easy to verify Finding the 100 unique roots of w100 1 is just as simple k 099 w exp1jpi100 2pik100 A semicolon concludes the final instruction to suppress the inconvenient display of all 100 solutions To view a particular solution the user must use an index to specify desired elements LathiBackground 2017925 1553 page 46 46 46 CHAPTER B BACKGROUND MATLAB indices are integers that increase from a starting value of 1 For example the fifth element of w is extracted using an index of 5 w5 ans 09603 02790i Notice that this solution corresponds to k 4 The independent variable of a function in this case k rarely serves as the index Since k is also a vector it can likewise be indexed In this way we can verify that the fifth value of k is indeed 4 k5 ans 4 It is also possible to use a vector index to access multiple values For example index vector 98100 identifies the last three solutions corresponding to k 979899 w98100 ans 09877 01564i 09956 00941i 09995 00314i Vector representations provide the foundation to rapidly create and explore various signals Consider the simple 10 Hz sinusoid described by ft sin2π10t π6 Two cycles of this sinusoid are included in the interval 0 t 02 A vector t is used to uniformly represent 500 points over this interval t 0025000202500 Next the function ft is evaluated at these points f sin2pi10tpi6 The value of ft at t 0 is the first element of the vector and is thus obtained by using an index of 1 f1 ans 05000 Unfortunately MATLABs indexing syntax conflicts with standard equation notation That is the MATLAB indexing command f1 is not the same as the standard notation f1 ftt1 Care must be taken to avoid confusion remember that the index parameter rarely reflects the independent variable of a function B74 Simple Plotting MATLABs plot command provides a convenient way to visualize data such as graphing ft against the independent variable t plottf Some other programming languages such as C begin indexing at 0 Careful attention is warranted MATLAB anonymous functions considered in Sec 111 are an important and useful exception LathiBackground 2017925 1553 page 47 47 B7 MATLAB Elementary Operations 47 0 005 01 015 02 t 1 0 1 ft Figure B12 ft sin2π10t π6 Axis labels are added using the xlabel and ylabel commands where the desired string must be enclosed by single quotation marks The result is shown in Fig B12 xlabelt ylabelft The title command is used to add a title above the current axis By default MATLAB connects data points with solid lines Plotting discrete points such as the 100 unique roots of w100 1 is accommodated by supplying the plot command with an additional string argument For example the string o tells MATLAB to mark each data point with a circle rather than connecting points with lines A full description of the supported plot options is available from MATLABs help facilities plotrealwimagwo xlabelRew ylabelImw axis equal The axis equal command ensures that the scale used for the horizontal axis is equal to the scale used for the vertical axis Without axis equal the plot would appear elliptical rather than circular Figure B13 illustrates that the 100 unique roots of w100 1 lie equally spaced on the unit circle a fact not easily discerned from the raw numerical data MATLAB also includes many specialized plotting functions For example MATLAB commands semilogx semilogy and loglog operate like the plot command but use base10 logarithmic scales for the horizontal axis vertical axis and the horizontal and vertical axes 1 05 0 05 1 Rew 05 0 05 Imw Figure B13 Unique roots of w100 1 LathiBackground 2017925 1553 page 48 48 48 CHAPTER B BACKGROUND respectively Monochrome and color images can be displayed by using the image command and contour plots are easily created with the contour command Furthermore a variety of threedimensional plotting routines are available such as plot3 contour3 mesh and surf Information about these instructions including examples and related functions is available from MATLAB help B75 ElementbyElement Operations Suppose a new function ht is desired that forces an exponential envelope on the sinusoid ft ht ftgt where gt e10t First row vector gt is created g exp10t Given MATLABs vector representation of gt and ft computing ht requires some form of vector multiplication There are three standard ways to multiply vectors inner product outer product and elementbyelement product As a matrixoriented language MATLAB defines the standard multiplication operator according to the rules of matrix algebra the multiplicand must be conformable to the multiplier A 1 N row vector times an N 1 column vector results in the scalarvalued inner product An N 1 column vector times a 1 M row vector results in the outer product which is an N M matrix Matrix algebra prohibits multiplication of two row vectors or multiplication of two column vectors Thus the operator is not used to perform elementbyelement multiplication Elementbyelement operations require vectors to have the same dimensions An error occurs if elementbyelement operations are attempted between row and column vectors In such cases one vector must first be transposed to ensure both vector operands have the same dimensions In MATLAB most elementbyelement operations are preceded by a period For example elementbyelement multiplication division and exponentiation are accomplished using and respectively Vector addition and subtraction are intrinsically elementbyelement operations and require no period Intuitively we know ht should be the same size as both gt and ft Thus ht is computed using elementbyelement multiplication h fg The plot command accommodates multiple curves and also allows modification of line properties This facilitates sidebyside comparison of different functions such as ht and ft Line characteristics are specified by using options that follow each vector pair and are enclosed in single quotes plottfkthk xlabelt ylabelAmplitude legendftht Here k instructs MATLAB to plot ft using a solid black line while k instructs MATLAB to use a dotted black line to plot ht A legend and axis labels complete the plot as shown in While grossly inefficient elementbyelement multiplication can be accomplished by extracting the main diagonal from the outer product of two Nlength vectors LathiBackground 2017925 1553 page 49 49 B7 MATLAB Elementary Operations 49 0 005 01 015 02 t 1 05 0 05 1 Amplitude ft ht Figure B14 Graphical comparison of ft and ht Fig B14 It is also possible although more cumbersome to use pull down menus to modify line properties and to add labels and legends directly in the figure window B76 Matrix Operations Many applications require more than row vectors with evenly spaced elements row vectors column vectors and matrices with arbitrary elements are typically needed MATLAB provides several functions to generate common useful matrices Given integers m n and vector x the function eyem creates the mm identity matrix the function onesmn creates the m n matrix of all ones the function zerosmn creates the m n matrix of all zeros and the function diagx uses vector x to create a diagonal matrix The creation of general matrices and vectors however requires each individual element to be specified Vectors and matrices can be input spreadsheet style by using MATLABs array editor This graphical approach is rather cumbersome and is not often used A more direct method is preferable Consider a simple row vector r r 1 0 0 The MATLAB notation abc cannot create this row vector Rather square brackets are used to create r r 1 0 0 r 1 0 0 Square brackets enclose elements of the vector and spaces or commas are used to separate row elements Next consider the 3 2 matrix A A 2 3 4 5 0 6 LathiBackground 2017925 1553 page 50 50 50 CHAPTER B BACKGROUND Matrix A can be viewed as a threehigh stack of twoelement row vectors With a semicolon to separate rows square brackets are used to create the matrix A 2 34 50 6 A 2 3 4 5 0 6 Each row vector needs to have the same length to create a sensible matrix In addition to enclosing string arguments a single quote performs the complex conjugate transpose operation In this way row vectors become column vectors and vice versa For example a column vector c is easily created by transposing row vector r c r c 1 0 0 Since vector r is real the complexconjugate transpose is just the transpose Had r been complex the simple transpose could have been accomplished by either r or conjr More formally square brackets are referred to as a concatenation operator A concatenation combines or connects smaller pieces into a larger whole Concatenations can involve simple numbers such as the sixelement concatenation used to create the 32 matrix A It is also possible to concatenate larger objects such as vectors and matrices For example vector c and matrix A can be concatenated to form a 3 3 matrix B B c A B 1 2 3 0 4 5 0 0 6 Errors will occur if the component dimensions do not sensibly match a 22 matrix would not be concatenated with a 3 3 matrix for example Elements of a matrix are indexed much like vectors except two indices are typically used to specify row and column Element 1 2 of matrix B for example is 2 B12 ans 2 Indices can likewise be vectors For example vector indices allow us to extract the elements common to the first two rows and last two columns of matrix B B1223 ans 2 3 4 5 Matrix elements can also be accessed by means of a single index which enumerates along columns Formally the element from row m and column n of an M N matrix may be obtained with a single index n 1M m For example element 1 2 of matrix B is accessed by using the index 2 13 1 4 That is B4 yields 2 LathiBackground 2017925 1553 page 51 51 B7 MATLAB Elementary Operations 51 One indexing technique is particularly useful and deserves special attention A colon can be used to specify all elements along a specified dimension For example B2 selects all column elements along the second row of B B2 ans 0 4 5 Now that we understand basic vector and matrix creation we turn our attention to using these tools on real problems Consider solving a set of three linear simultaneous equations in three unknowns x1 2x2 3x3 1 3x1 x2 5x3 π 3x1 7x2 x3 e This system of equations is represented in matrix form according to Ax y where A 1 2 3 3 1 5 3 7 1 x x1 x2 x3 and y 1 π e Although Cramers rule can be used to solve Ax y it is more convenient to solve by multiplying both sides by the matrix inverse of A That is x A1Ax A1y Solving for x by hand or by calculator would be tedious at best so MATLAB is used We first create A and y A 1 2 3sqrt3 1 sqrt53 sqrt7 1 y 1piexp1 The vector solution is found by using MATLABs inv function x invAy x 19999 38998 15999 It is also possible to use MATLABs left divide operator x Ay to find the same solution The left divide is generally more computationally efficient than matrix inverses As with matrix multiplication left division requires that the two arguments be conformable Of course Cramers rule can be used to compute individual solutions such as x1 by using vector indexing concatenation and MATLABs det command to compute determinants x1 detyA23detA x1 19999 Another nice application of matrices is the simultaneous creation of a family of curves Consider hαt eαt sin2π10t π6 over 0 t 02 Figure B14 shows hαt for α 0 and α 10 Lets investigate the family of curves hαt for α 0110 An inefficient way to solve this problem is create hαt for each α of interest This requires 11 individual cases Instead a matrix approach allows all 11 curves to be computed simultaneously First a vector is created that contains the desired values of α alpha 010 By using a sampling interval of one millisecond Δt 0001 a time vector is also created t 0000102 The result is a length201 column vector By replicating the time vector for each of the 11 curves required a time matrix T is created This replication can be accomplished by using an outer product between t and a 1 x 11 vector of ones T tones111 The result is a 201 x 11 matrix that has identical columns Right multiplying T by a diagonal matrix created from α columns of T can be individually scaled and the final result is computed H expTdiagalphasin2pi10Tpi6 Here H is a 201 x 11 matrix where each column corresponds to a different value of α That is H h0h1h10 where hx are column vectors As shown in Fig B15 the 11 desired curves are simultaneously displayed by using MATLABs plot command which allows matrix arguments plottH xlabelt ylabelht This example illustrates an important technique called vectorization which increases execution efficiency for interpretive languages such as MATLAB Algorithm vectorization uses matrix and vector operations to avoid manual repetition and loop structures It takes practice and effort to become proficient at vectorization but the worthwhile result is efficient compact code B77 Partial Fraction Expansions There are a wide variety of techniques and shortcuts to compute the partial fraction expansion of rational function Fx BαAα but few are more simple than the MATLAB residue command The basic form of this command is RPK residueBA The two input vectors B and A specify the polynomial coefficients of the numerator and denominator respectively These vectors are ordered in descending powers of the independent variable Three vectors are output The vector R contains the coefficients of each partial fraction vector P contains the corresponding roots of each partial fraction For a root repeated r times the r partial fractions are ordered in ascending powers When the rational function is not proper the vector K contains the direct terms which are ordered in descending powers of the independent variable To demonstrate the direct use of the residue command consider finding the partial fraction expansion of Fx x5 πx2x23 x5 πx4 8x3 32x 4 By hand the partial fraction expansion of Fx is difficult to compute MATLAB however makes short work of the expansion RPK residue1 0 0 0 0 pi1 sqrt8 0 sqrt32 4 R P K The two outputs R and P specify the partial fraction expansion of Fx is Fx x 28284 78888x 2 59713x 22 31107x 23 01112x 2 The signalprocessing toolbox function residuez is similar to the residue command and offers more convenient expansion of certain rational functions such as those commonly encountered in the study of discretetime systems Additional information about the residue and residuez commands is available from MATLABs help facilities B8 APPENDIX USEFUL MATHEMATICAL FORMULAS We conclude this chapter with a selection of useful mathematical facts B84 Taylor and Maclaurin Series fx fa x af a x a2 f a 2 k0 x ak k fka B87 Common Derivative Formulas d dx u d du fu du dx d dx uv u dv dx v du dx udv uv vdu B89 LHôpitals Rule If lim fxgx results in the indeterministic form 00 or then lim fx gx lim fx gx LathiBackground 2017925 1553 page 59 59 Problems 59 4 Cajori Florian A History of Mathematics 4th ed Chelsea New York 1985 5 Encyclopaedia Britannica Micropaedia IV 15th ed vol 11 p 1043 Chicago 1982 6 Singh Jagjit Great Ideas of Modern Mathematics Dover New York 1959 7 Dunham William Journey Through Genius Wiley New York 1990 PROBLEMS B11 Given a complex number w x jy the com plex conjugate of w is defined in rectangular coordinates as w xjy Use this fact to derive complex conjugation in polar form B12 Express the following numbers in polar form a wa 1 j b wb 1 ej c wc 4 j3 d wd 1 j4 j3 e we ejπ4 2ejπ4 f wf 1j 2j g wg 1 j4 j3 h wh 1j sinj B13 Express the following numbers in Cartesian rectangular form a wa j ej b wb 3ejπ4 c wc 1ej d wd 1 j4 j3 e we ejπ4 2ejπ4 f wf ej 1 g wg 12j h wh jjj j raised to the j raised to the j B14 Showing all work and simplifying your answer determine the real part of the following num bers a wa 1 j j 5e23j b wb 1 jln1 j B15 Showing all work and simplifying your answer determine the imaginary part of the following numbers a wa jejπ4 b wb 1 2je24j c wc tanj B16 For complex constant w prove a Rew w w2 b Imw w w2j B17 Given w x jy determine a Reew b Imew B18 For arbitrary complex constants w1 and w2 prove or disprove the following a Rejw1 Imw1 b Imjw1 Rew1 c Rew1 Rew2 Rew1 w2 d Imw1 Imw2 Imw1 w2 e Rew1Rew2 Rew1w2 f Imw1Imw2 Imw1w2 B19 Given w1 3 j4 and w2 2ejπ4 a Express w1 in standard polar form b Express w2 in standard rectangular form c Determine w12 and w22 d Express w1 w2 in standard rectangular form e Express w1 w2 in standard polar form f Express w1w2 in standard rectangular form g Express w1w2 in standard polar form B110 Repeat Prob B19 using w1 3 j42 and w2 25jej40π B111 Repeat Prob B19 using w1 j eπ4 and w2 cosj B112 Using the complex plane a Evaluate and locate the distinct solutions to w4 1 b Evaluate and locate the distinct solutions to w 1 j25 32 21 j c Sketch the solution to w 2j 3 d Graph wt 1 tejt for 10 t 10 B113 The distinct solutions to w w1n w2 lie on a circle in the complex plane as shown in Fig PB113 One solution is located on the real axis at 3 1 2732 and one solution is located on the imaginary axis at 31 0732 Determine w1 w2 and n B114 Find the distinct solutions to each of the following Use MATLAB to graph each solution set in the complex plane a w³ 2 b w i³ 1 c w² j 0 d 16w i4 81 0 e w 2j³ 8 f j w¹² 2 j2 g w 1¹² j2 a Show that coshw coshx cosy sinhx siny b Determine a similar expression for sinhw in rectangular form that only uses functions of real arguments such as sinx cosy and so on B25 Use Eulers identity to solve or prove the following a Find real positive constants c and φ for all real t such that 25 cos3t 15 sin3t π3 cos3t φ Sketch the resulting sinusoid b Prove that cosθ φ cosθ cosφ sinθ sinφ c Given real constants a b and α complex constant w and the fact that ewx dx 1w ewx ewx evaluate the integral ex sinax dx B26 A particularly boring stretch of interstate highway has a posted speed limit of 70 mph A highway engineer wants to install rumble bars raised ridges on the side of the road so that cars traveling the speed limit will produce quartersecond bursts of 1 kHz sound every second a strategy that is particularly effective at startling sleepy drivers awake Provide design specifications for the engineer B31 By hand accurately sketch the following signals over 0 t 1 a x₁t et b x₂t sin2πt c x₃t esin2πt B32 In 1950 the human population was approximately 25 billion people Assuming a doubling time of 40 years formulate an exponential model for human population in the form pt aekt where t is measured in years Sketch pt over the interval 1950 t 2100 According to this model in what year can we expect the population to reach the estimated 15 billion carrying capacity of the earth B33 Determine an expression for an exponentially decaying sinusoid that oscillates three times per second and whose amplitude envelopes decrease by 50 every 2 seconds Use MATLAB to plot the signal over 1 t 2 B34 By hand sketch the following against independent variable t a x₃t Ree212ty b x₄t ln3 e2t c x₆t 3 1ee12t B41 Consider the following system of equations 1 2 4 x₁ x₂ 3 Expressing all answers in rational form ratio of integers use Cramers rule to determine x₁ and x₂ Perform all calculations by hand including matrix determinants B42 Consider the following system of equations 1 2 0 x₁ 0 3 4 x₂ 5 0 6 x₃ Expressing all answers in rational form ratio of integers use Cramers rule to determine x₁ x₂ and x₃ Perform all calculations by hand including matrix determinants B43 Consider the following system of equations x₁ x₂ x₃ x₄ 1 x₁ 2x₂ 3x₃ 2 x₁ x₃ 7x₄ 3 2x₂ 3x₃ 4x₄ 4 Use Cramers rule to determine x₁ x₂ and x₃ Matrix determinants can be computed by using MATLABs det command B51 Determine the constants a₀ a₁ and a₂ of the partial fraction expansion Fs ss³ 1 a₀s 1 a₁s 1² a₂s 1³ 01LathiC01 2017925 1553 page 64 1 C H A P T E R SIGNALS AND SYSTEMS 1 In this chapter we shall discuss basic aspects of signals and systems We shall also introduce fundamental concepts and qualitative explanations of the hows and whys of systems theory thus building a solid foundation for understanding the quantitative analysis in the remainder of the book For simplicity the focus of this chapter is on continuoustime signals and systems Chapter 3 presents the same ideas for discretetime signals and systems SIGNALS A signal is a set of data or information Examples include a telephone or a television signal monthly sales of a corporation or daily closing prices of a stock market eg the Dow Jones averages In all these examples the signals are functions of the independent variable time This is not always the case however When an electrical charge is distributed over a body for instance the signal is the charge density a function of space rather than time In this book we deal almost exclusively with signals that are functions of time The discussion however applies equally well to other independent variables SYSTEMS Signals may be processed further by systems which may modify them or extract additional infor mation from them For example an antiaircraft gun operator may want to know the future location of a hostile moving target that is being tracked by his radar Knowing the radar signal he knows the past location and velocity of the target By properly processing the radar signal the input he can approximately estimate the future location of the target Thus a system is an entity that processes a set of signals inputs to yield another set of signals outputs A system may be made up of physical components as in electrical mechanical or hydraulic systems hardware realization or it may be an algorithm that computes an output from an input signal software realization 11 SIZE OF A SIGNAL The size of any entity is a number that indicates the largeness or strength of that entity Generally speaking the signal amplitude varies with time How can a signal that exists over a certain time 64 B52 Compute by hand the partial fraction expansions of the following rational functions a F₁s s² 9s⁴ 4s² 3 b F₂t t 1t² 1 c F₃t t 1t² 1 d F₄s s² 2s 3s³ 2s² d e F₅t 2t² 6t 5 f F₆t 2tt² 4t 3 g F₇t 1t² 2t 2 h F₈s ss² 4s 4 i F₉s s²s³ s 1 j F₁₀s s² 2s 1 k F₁₁s 3 5ss² 1 B61 A system of equations in terms of unknowns x₁ and x₂ and arbitrary constants a b c d e and f is given by ax₁ bx₂ c dx₁ ex₂ f a Represent this system of equations in matrix form b Identify specific constants a b c d e and f such that x₁ 3 and x₂ 2 Are the constants you selected unique c Identify nonzero constants a b c d e and f such that no solutions x₁ and x₂ exist d Identify nonzero constants a b c d e and f such that an infinite number of solutions x₁ and x₂ exist interval with varying amplitude be measured by one number that will indicate the signal size or signal strength Such a measure must consider not only the signal amplitude but also its duration For instance if we are to devise a single number V as a measure of the size of a human being we must consider not only his or her width girth but also the height If we make a simplifying assumption that the shape of a person is a cylinder of variable radius r which varies with the height h then one possible measure of the size of a person of height H is the persons volume V given by V π 0 to H r²h dh 111 Signal Energy 01LathiC01 2017925 1553 page 66 3 66 CHAPTER 1 SIGNALS AND SYSTEMS b xt t xt a t Figure 11 Examples of signals a a signal with finite energy and b a signal with finite power When xt is periodic xt2 is also periodic Hence the power of xt can be computed from Eq 12 by averaging xt2 over one period Comments The signal energy as defined in Eq 11 does not indicate the actual energy in the conventional sense of the signal because the signal energy depends not only on the signal but also on the load It can however be interpreted as the energy dissipated in a normalized load of a 1 ohm resistor if a voltage xt were to be applied across the 1 ohm resistor or if a current xt were to be passed through the 1 ohm resistor The measure of energy is therefore indicative of the energy capability of the signal not the actual energy For this reason the concepts of conservation of energy should not be applied to this signal energy Parallel observation applies to signal power defined in Eq 12 These measures are but convenient indicators of the signal size which prove useful in many applications For instance if we approximate a signal xt by another signal gt the error in the approximation is et xt gt The energy or power of et is a convenient indicator of the goodness of the approximation It provides us with a quantitative measure of determining the closeness of the approximation In communication systems during transmission over a channel message signals are corrupted by unwanted signals noise The quality of the received signal is judged by the relative sizes of the desired signal and the unwanted signal noise In this case the ratio of the message signal and noise signal powers signaltonoise power ratio is a good indication of the received signal quality Units of Energy and Power Equation 11 is not correct dimensionally This is because here we are using the term energy not in its conventional sense but to indicate the signal size The same observation applies to Eq 12 for power The units of energy and power as defined here depend on the nature of the signal xt If xt is a voltage signal its energy Ex has units of volts squaredseconds V2 s and its power Px has units of volts squared If xt is a current signal these units will be amperes squaredseconds A2 s and amperes squared respectively In this manner we may consider the area under a signal xt as a possible measure of its size because it takes account not only of the amplitude but also of the duration However this will be a defective measure because even for a large signal xt its positive and negative areas could cancel each other indicating a signal of small size This difficulty can be corrected by defining the signal size as the area under xt² which is always positive We call this measure the signal energy Eₓ defined as Eₓ to xt² dt 11 This definition simplifies for a realvalued signal xt to Eₓ to x²t dt There are also other possible measures of signal size such as the area under xt The energy measure however is not only more tractable mathematically but is also more meaningful as shown later in the sense that it is indicative of the energy that can be extracted from the signal 112 Signal Power Signal energy must be finite for it to be a meaningful measure of signal size A necessary condition for the energy to be finite is that the signal amplitude 0 as t Fig 11a Otherwise the integral in Eq 11 will not converge When the amplitude of xt does not 0 as t Fig 11b the signal energy is infinite A more meaningful measure of the signal size in such a case would be the time average of the energy if it exists This measure is called the power of the signal For a signal xt we define its power Pₓ as Pₓ lim T 1T T2 to T2 xt² dt 12 The first and second integrals on the righthand side are the powers of two sinusoids which are C1²2 and C2²2 as found in part a The third term the product of two sinusoids can be expressed as a sum of two sinusoids cosω1ω2tθ1θ2 and cosω1ω2tθ1θ2 respectively Now arguing as in part a we see that the third term is zero Hence we have Px C1²2 C2²2 and the rms value is C1²C2²2 We can readily extend this result to a sum of any number of sinusoids with distinct frequencies Thus if xt n1 to Cn cosωntθn assuming that none of the two sinusoids have identical frequencies and ωn ωm then Px 12 n1 to Cn² If xt also has a dc term as xt C0 n1 to Cn cosωntθn then Px C0² 12 n1 to Cn² c In this case the signal is complex and we use Eq 12 to compute the power Px lim T 1T T2 to T2 Dejω0t² dt Recall that ejω0t 1 so that Dejω0t² D² and Px D² 14 The rms value is D Comment In part b of Ex 12 we have shown that the power of the sum of two sinusoids is equal to the sum of the powers of the sinusoids It may appear that the power of x1t x2t is Px1 Px2 Unfortunately this conclusion is not true in general It is true only under a certain condition orthogonality discussed later Sec 653 12 SOME USEFUL SIGNAL OPERATIONS We discuss here three useful signal operations shifting scaling and inversion Since the independent variable in our signal description is time these operations are discussed as time shifting time scaling and time reversal inversion However this discussion is valid for functions having independent variables other than time eg frequency or distance 121 Time Shifting Consider a signal xt Fig 14a and the same signal delayed by T seconds Fig 14b which we shall denote by φt Whatever happens in xt Fig 14a at some instant t also happens in φt Fig 14b T seconds later at the instant t T Therefore φtT xt and φt xt T Therefore to timeshift a signal by T we replace t with t T Thus xt represents xt timeshifted by T seconds If T is positive the shift is to the right delay as in Fig 14b If T is negative the shift is to the left advance as in Fig 14c Clearly xt 2 is xt delayed rightshifted by 2 seconds and xt 2 is xt advanced leftshifted by 2 seconds The function xt can be described mathematically as xt e2t t 0 0 t 0 15 by replacing t with t 1 in Eq 15 Thus Write a mathematical description of the signal x3t in Fig 13c Next delay this signal by 2 seconds Sketch the delayed signal Show that this delayed signal x4t can be described mathematically as x4t 2t 2 for 2 t 3 and equal to 0 otherwise Now repeat the procedure with the signal advanced leftshifted by 1 second Show that this advanced signal x5t can be described as x5t 2t 1 for 1 t 0 and 0 otherwise Figure 17 a Signal xt b signal x3t and c signal xt2 DRILL 15 Compression and Expansion of Sinusoids EXAMPLE 15 Time Reversal of a Signal 01LathiC01 2017925 1553 page 78 15 78 CHAPTER 1 SIGNALS AND SYSTEMS Alternately we can first timecompress xt by factor 2 to obtain x2t then delay this signal by 3 replace t with t 3 to obtain x2t 6 13 CLASSIFICATION OF SIGNALS Classification helps us better understand and utilize the items around us Cars for example are classified as sports offroad family and so forth Knowing you have a sports car is useful in deciding whether to drive on a highway or on a dirt road Knowing you want to drive up a mountain you would probably choose an offroad vehicle over a family sedan Similarly there are several classes of signals Some signal classes are more suitable for certain applications than others Further different signal classes often require different mathematical tools Here we shall consider only the following classes of signals which are suitable for the scope of this book 1 Continuoustime and discretetime signals 2 Analog and digital signals 3 Periodic and aperiodic signals 4 Energy and power signals 5 Deterministic and probabilistic signals 131 ContinuousTime and DiscreteTime Signals A signal that is specified for a continuum of values of time t Fig 110a is a continuoustime signal and a signal that is specified only at discrete values of t Fig 110b is a discretetime signal Telephone and video camera outputs are continuoustime signals whereas the quarterly gross national product GNP monthly sales of a corporation and stock market daily averages are discretetime signals 132 Analog and Digital Signals The concept of continuous time is often confused with that of analog The two are not the same The same is true of the concepts of discrete time and digital A signal whose amplitude can take on any value in a continuous range is an analog signal This means that an analog signal amplitude can take on an infinite number of values A digital signal on the other hand is one whose amplitude can take on only a finite number of values Signals associated with a digital computer are digital because they take on only two values binary signals A digital signal whose amplitudes can take on M values is an Mary signal of which binary M 2 is a special case The terms continuous time and discrete time qualify the nature of a signal along the time horizontal axis The terms analog and digital on the other hand qualify the nature of the signal amplitude vertical axis Figure 111 shows examples of signals of various types It is clear that analog is not necessarily continuoustime and digital need not be discretetime Figure 111c shows an example of an analog discretetime signal An analog signal can be converted into a digital signal analogtodigital AD conversion through quantization rounding off as explained in Sec 83 xt 01LathiC01 2017925 1553 page 80 17 80 CHAPTER 1 SIGNALS AND SYSTEMS a c b t t t t d xt xt xt xt Figure 111 Examples of signals a analog continuous time b digital continuous time c analog discrete time and d digital discrete time t T0 xt Figure 112 A periodic signal of period T0 xt Therefore a periodic signal by definition must start at t and continue forever as illustrated in Fig 112 Another important property of a periodic signal xt is that xt can be generated by periodic extension of any segment of xt of duration T0 the period As a result we can generate xt from any segment of xt having a duration of one period by placing this segment and the reproduction thereof end to end ad infinitum on either side Figure 113 shows a periodic signal xt of period T0 6 The shaded portion of Fig 113a shows a segment of xt starting at t 1 and having a duration of one period 6 seconds This segment when repeated forever in either direction results in the periodic signal xt Figure 113b shows another shaded segment of xt of duration T0 starting at t 0 Again we see that this segment when repeated forever on either side results in xt The reader can verify that this construction is possible with any segment of xt starting at any instant as long as the segment duration is one period Quarterly GNP The return of recession In percent change seasonally adjusted annual rates Source Commerce Department news reports 01LathiC01 2017925 1553 page 82 19 82 CHAPTER 1 SIGNALS AND SYSTEMS eg an impulse and an everlasting sinusoid that cannot be generated in practice do serve a very useful purpose in the study of signals and systems 134 Energy and Power Signals A signal with finite energy is an energy signal and a signal with finite and nonzero power is a power signal The signals in Figs 12a and 12b are examples of energy and power signals respectively Observe that power is the time average of energy Since the averaging is over an infinitely large interval a signal with finite energy has zero power and a signal with finite power has infinite energy Therefore a signal cannot be both an energy signal and a power signal If it is one it cannot be the other On the other hand there are signals that are neither energy nor power signals The ramp signal is one such case Comments All practical signals have finite energies and are therefore energy signals A power signal must necessarily have infinite duration otherwise its power which is its energy averaged over an infinitely large interval will not approach a nonzero limit Clearly it is impossible to generate a true power signal in practice because such a signal has infinite duration and infinite energy Also because of periodic repetition periodic signals for which the area under xt2 over one period is finite are power signals however not all power signals are periodic DRILL 16 Neither Energy nor Power Show that an everlasting exponential eat is neither an energy nor a power signal for any real value of a However if a is imaginary it is a power signal with power Px 1 regardless of the value of a 135 Deterministic and Random Signals A signal whose physical description is known completely in either a mathematical form or a graphical form is a deterministic signal A signal whose values cannot be predicted precisely but are known only in terms of probabilistic description such as mean value or meansquared value is a random signal In this book we shall exclusively deal with deterministic signals Random signals are beyond the scope of this study 14 SOME USEFUL SIGNAL MODELS In the area of signals and systems the step the impulse and the exponential functions play very important roles Not only do they serve as a basis for representing other signals but their use can simplify many aspects of the signals and systems ut 1 t 0 0 t 0 Use the unit step function to describe the signal in Fig 116a Over the interval from 15 to 0 the signal can be described by a constant 2 and over the interval from 0 to 3 it can be described by 2et2 Therefore Show that the signal shown in Fig 118 can be described as xt t 1ut 1 t 2ut 2 ut 4 In the limit as α the pulse height and its width or duration 0 Yet the area under the pulse is unity regardless of the value of α because 0 aeαt dt 1 The definition of the unit impulse function given in Eq 19 is not mathematically rigorous which leads to serious difficulties First the impulse function does not define a unique function for example it can be shown that δt δt also satisfies Eq 19 Moreover δt is not even a true function in the ordinary sense An ordinary function is specified by its values for all time t The impulse function is zero everywhere except at t 0 and at this the only interesting part of its range it is undefined These difficulties are resolved by defining the impulse as a generalized function rather than an ordinary function A generalized function is defined by its effect on other functions instead of by its value at every instant of time This result shows that the unit step function can be obtained by integrating the unit impulse function Similarly the unit ramp function xt tut can be obtained by integrating the unit step function We may continue with unit parabolic function t²2 obtained by integrating the unit ramp and so on On the other side we have derivatives of impulse function which can be defined as generalized functions see Prob 1412 All these functions derived from the unit impulse function successive derivatives and integrals are called singularity functions Therefore est e sigma j omega t esigma t ej omega t esigma t cos omega t j sin omega t 113 Since s sigma j omega the conjugate of s then est e sigma j omega t esigma t ej omega t esigma t cos omega t j sin omega t and esigma t cos omega t frac12 est est 114 A comparison of Eq 113 with Eulers formula shows that est is a generalization of the function ej omega t where the frequency variable j omega is generalized to a complex variable s sigma j omega For this reason we designate the variable s as the complex frequency In fact function est encompasses a large class of functions The following functions are either special cases of or can be expressed in terms of est 1 A constant k ke0t s 0 2 A monotonic exponential est omega 0 s sigma 3 A sinusoid cos omega t sigma 0 s j omega 4 An exponentially varying sinusoid est cos s s sigma pm j omega These functions are illustrated in Fig 121 The complex frequency s can be conveniently represented on a complex frequency plane s plane as depicted in Fig 122 The horizontal axis is the real axis sigma axis and the vertical axis is the imaginary axis omega axis The absolute value of the imaginary part of s is omega the 01LathiC01 2017925 1553 page 91 28 14 Some Useful Signal Models 91 Exponentially increasing signals Left halfplane Right halfplane Real axis Imaginary axis jv s Exponentially decreasing signals Figure 122 Complex frequency plane radian frequency which indicates the frequency of oscillation of est the real part σ the neper frequency gives information about the rate of increase or decrease of the amplitude of est For signals whose complex frequencies lie on the real axis σ axis where ω 0 the frequency of oscillation is zero Consequently these signals are monotonically increasing or decreasing exponentials Fig 121a For signals whose frequencies lie on the imaginary axis ω axis where σ 0 eσt 1 Therefore these signals are conventional sinusoids with constant amplitude Fig 121b The case s 0 σ ω 0 corresponds to a constant dc signal because e0t 1 For the signals illustrated in Figs 121c and 121d both σ and ω are nonzero the frequency s is complex and does not lie on either axis The signal in Fig 121c decays exponentially Therefore σ is negative and s lies to the left of the imaginary axis In contrast the signal in Fig 121d grows exponentially Therefore σ is positive and s lies to the right of the imaginary axis Thus the s plane Fig 121 can be separated into two parts the left halfplane LHP corresponding to exponentially decaying signals and the right halfplane RHP corresponding to exponentially growing signals The imaginary axis separates the two regions and corresponds to signals of constant amplitude An exponentially growing sinusoid e2t cos 5t for example can be expressed as a linear combination of exponentials e2j5t and e2j5t with complex frequencies 2 j5 and 2 j5 respectively which lie in the RHP An exponentially decaying sinusoid e2t cos 5t can be expressed as a linear combination of exponentials e2j5t and e2j5t with complex frequencies 2 j5 and 2 j5 respectively which lie in the LHP A constantamplitude sinusoid cos 5t can be expressed as a linear combination of exponentials ej5t and ej5t with complex frequencies j5 which lie on the imaginary axis Observe that the monotonic exponentials e2t are also generalized sinusoids with complex frequencies 2 15 EVEN AND ODD FUNCTIONS A function xet is said to be an even function of t if it is symmetrical about the vertical axis A function xot is said to be an odd function of t if it is antisymmetrical about the vertical axis Mathematically expressed these symmetry conditions require xet xet and xot xot 115 An even function has the same value at the instants t and t for all values of t On the other hand the value of an odd function at the instant t is the negative of its value at the instant t An example even signal and an example odd signal are shown in Figs 123a and 123b respectively 151 Some Properties of Even and Odd Functions Even and odd functions have the following properties even function odd function odd function odd function odd function even function even function even function even function The proofs are trivial and follow directly from the definition of odd and even functions Eq 115 AREA Because of the symmetries of even and odd functions about the vertical axis it follows from Eq 115 or Fig 123 that intaa xet dt 2 int0a xet dt and intaa xot dt 0 116 These results are valid under the assumption that there is no impulse or its derivatives at the origin The proof of these statements is obvious from the plots of even and odd functions Formal proofs left as an exercise for the reader can be accomplished by using the definitions in Eq 115 Because of their properties study of odd and even functions proves useful in many applications as will become evident in later chapters 152 Even and Odd Components of a Signal Every signal xt can be expressed as a sum of even and odd components because xt frac12 xt xt frac12 xt xt 117 From the definitions in Eq 115 we can clearly see that the first component on the righthand side is an even function while the second component is odd This is apparent from the fact that replacing t by t in the first component yields the same function The same maneuver in the second component yields the negative of that component Find the even and odd components of eit From Eq 117 eit xet xot where xet frac12eit eit cos t and xot frac12eit eit jsin t 01LathiC01 2017925 1553 page 95 32 16 Systems 95 A MODIFICATION FOR COMPLEX SIGNALS While a complex signal can be decomposed into even and odd components it is more common to decompose complex signals using conjugate symmetries A complex signal xt is said to be conjugatesymmetric if xt xt A conjugatesymmetric signal is even in the real part and odd in the imaginary part Thus a real conjugatesymmetric signal is an even signal A signal is conjugateantisymmetric if xt xt A conjugateantisymmetric signal is odd in the real part and even in the imaginary part A real conjugateantisymmetric signal is an odd signal Any signal xt can be decomposed into a conjugatesymmetric portion xcst plus a conjugateantisymmetric portion xcat That is xt xcst xcat where xcst xt xt 2 and xcat xt xt 2 The proof is similar to the one for decomposing a signal into even and odd components As we shall see in later chapters conjugate symmetries commonly occur in realworld signals and their transforms 16 SYSTEMS As mentioned in Sec 11 systems are used to process signals to allow modification or extraction of additional information from the signals A system may consist of physical components hardware realization or of an algorithm that computes the output signal from the input signal software realization Roughly speaking a physical system consists of interconnected components which are characterized by their terminal inputoutput relationships In addition a system is governed by laws of interconnection For example in electrical systems the terminal relationships are the familiar voltagecurrent relationships for the resistors capacitors inductors transformers transistors and so on as well as the laws of interconnection ie Kirchhoffs laws We use these laws to derive mathematical equations relating the outputs to the inputs These equations then represent a mathematical model of the system A system can be conveniently illustrated by a black box with one set of accessible terminals where the input variables x1t x2t xjt are applied and another set of accessible terminals where the output variables y1t y2t ykt are observed Fig 125 The study of systems consists of three major areas mathematical modeling analysis and design Although we shall be dealing with mathematical modeling our main concern is with y1t y2t ykt x1t x2t xjt Figure 125 Representation of a system 01LathiC01 2017925 1553 page 97 34 17 Classification of Systems 97 past to t0 that we need to compute yt for t t0 Therefore the response of a system at t t0 can be determined from its inputs during the interval t0 to t and from certain initial conditions at t t0 In the preceding example we needed only one initial condition However in more complex systems several initial conditions may be necessary We know for example that in passive RLC networks the initial values of all inductor currents and all capacitor voltages are needed to determine the outputs at any instant t 0 if the inputs are given over the interval 0t 17 CLASSIFICATION OF SYSTEMS Systems may be classified broadly in the following categories 1 Linear and nonlinear systems 2 Constantparameter and timevaryingparameter systems 3 Instantaneous memoryless and dynamic with memory systems 4 Causal and noncausal systems 5 Continuoustime and discretetime systems 6 Analog and digital systems 7 Invertible and noninvertible systems 8 Stable and unstable systems Other classifications such as deterministic and probabilistic systems are beyond the scope of this text and are not considered 171 Linear and Nonlinear Systems THE CONCEPT OF LINEARITY A system whose output is proportional to its input is an example of a linear system But linearity implies more than this it also implies the additivity property that is if several inputs are acting on a system then the total effect on the system due to all these inputs can be determined by considering one input at a time while assuming all the other inputs to be zero The total effect is then the sum of all the component effects This property may be expressed as follows for a linear system if an input x1 acting alone has an effect y1 and if another input x2 also acting alone has an effect y2 then with both inputs acting on the system the total effect will be y1 y2 Thus if x1 y1 and x2 y2 then for all x1 and x2 x1 x2 y1 y2 120 In addition a linear system must satisfy the homogeneity or scaling property which states that for arbitrary real or imaginary number k if an input is increased kfold the effect also increases kfold Thus if x y Strictly speaking this means independent inductor currents and capacitor voltages 01LathiC01 2017925 1553 page 98 35 98 CHAPTER 1 SIGNALS AND SYSTEMS then for all real or imaginary k kx ky 121 Thus linearity implies two properties homogeneity scaling and additivity Both these properties can be combined into one property superposition which is expressed as follows If x1 y1 and x2 y2 then for all inputs x1 and x2 and all constants k1 and k2 k1x1 k2x2 k1y1 k2y2 122 There is another useful way to view the linearity condition described in Eq 122 the response of a linear system is unchanged whether the operations of summing and scaling precede the system sum and scale act on inputs or follow the system sum and scale act on outputs Thus linearity implies commutability between a system and the operations of summing and scaling It may appear that additivity implies homogeneity Unfortunately homogeneity does not always follow from additivity Drill 111 demonstrates such a case DRILL 111 Additivity but Not Homogeneity Show that a system with the input xt and the output yt related by yt Rext satisfies the additivity property but violates the homogeneity property Hence such a system is not linear Hint Show that Eq 121 is not satisfied when k is complex RESPONSE OF A LINEAR SYSTEM For the sake of simplicity we discuss only singleinput singleoutput SISO systems But the discussion can be readily extended to multipleinput multipleoutput MIMO systems A systems output for t 0 is the result of two independent causes the initial conditions of the system or the system state at t 0 and the input xt for t 0 If a system is to be linear the output must be the sum of the two components resulting from these two causes first the zeroinput response ZIR that results only from the initial conditions at t 0 with the input xt 0 for t 0 and then the zerostate response ZSR that results only from the input xt for t 0 when the initial conditions at t 0 are assumed to be zero When all the appropriate initial conditions are zero the system is said to be in zero state The system output is zero when the input is zero only if the system is in zero state In summary a linear system response can be expressed as the sum of the zeroinput and zerostate responses total response zeroinput response zerostate response A linear system must also satisfy the additional condition of smoothness where small changes in the systems inputs must result in small changes in its outputs 3 01LathiC01 2017925 1553 page 100 37 100 CHAPTER 1 SIGNALS AND SYSTEMS show that a system described by a differential equation of the form a0 dNyt dtN a1 dN1yt dtN1 aNyt bNM dMxt dtM bN1 dxt dt bNxt 125 is a linear system The coefficients ai and bi in this equation can be constants or functions of time Although here we proved only zerostate linearity it can be shown that such systems are also zeroinput linear and have the decomposition property DRILL 112 Linearity of a Differential Equation with TimeVarying Parameters Show that the system described by the following equation is linear dyt dt t2yt 2t 3xt DRILL 113 A Nonlinear Differential Equation Show that the system described by the following equation is nonlinear ytdyt dt 3yt xt MORE COMMENTS ON LINEAR SYSTEMS Almost all systems observed in practice become nonlinear when large enough signals are applied to them However it is possible to approximate most of the nonlinear systems by linear systems for smallsignal analysis The analysis of nonlinear systems is generally difficult Nonlinearities can arise in so many ways that describing them with a common mathematical form is impossible Not only is each system a category in itself but even for a given system changes in initial conditions or input amplitudes may change the nature of the problem On the other hand the superposition property of linear systems is a powerful unifying principle that allows for a general solution The superposition property linearity greatly simplifies the analysis of linear systems Because of the decomposition property we can evaluate separately the two components of the output The zeroinput response can be computed by assuming the input to be zero and the zerostate response can be computed by assuming zero initial conditions Moreover if we express an 01LathiC01 2017925 1553 page 103 40 17 Classification of Systems 103 It is possible to verify that the system in Fig 126 is a timeinvariant system Networks composed of RLC elements and other commonly used active elements such as transistors are timeinvariant systems A system with an inputoutput relationship described by a linear differential equation of the form given in Ex 110 Eq 125 is a linear timeinvariant LTI system when the coefficients ai and bi of such equation are constants If these coefficients are functions of time then the system is a linear timevarying system The system described in Drill 112 is linear time varying Another familiar example of a timevarying system is the carbon microphone in which the resistance R is a function of the mechanical pressure generated by sound waves on the carbon granules of the microphone The output current from the microphone is thus modulated by the sound waves as desired EXAMPLE 111 Assessing System Time Invariance Determine the time invariance of the following systems a yt xtut and b yt d dtxt a In this case the output equals the input for t 0 and is otherwise zero Clearly the input is being modified by a timedependent function so the system is likely time variant We can prove that the system is not time invariant through a counterexample Letting x1t δt1 we see that y1t 0 However x2t x1t2 δt1 produces an output of y2t δt 1 which does equal y1t 2 0 as timeinvariance would require Thus yt xtut is a time variant system b Although it appears that xt is being modified by a timedependent function this is not the case The output of this system is simply the slope of the input If the input is delayed so too is the output Applying input xt to the system produces output yt d dtxt delaying this output by T produces yt T d dtTxt T d dtxt T This is just the output of the system to a delayed input xt T Since the Tdelayed output of the system to input xt equals the output of the system to the Tdelayed input xt T the system is time invariant DRILL 114 A TimeVariant System Show that a system described by the following equation is a timevaryingparameter system yt sin txt 2 Hint Show that the system fails to satisfy the timeinvariance property 173 Instantaneous and Dynamic Systems As observed earlier a systems output at any instant t generally depends on the entire past input However in a special class of systems the output at any instant t depends only on its input at that 01LathiC01 2017925 1553 page 104 41 104 CHAPTER 1 SIGNALS AND SYSTEMS instant In resistive networks for example any output of the network at some instant t depends only on the input at the instant t In these systems past history is irrelevant in determining the response Such systems are said to be instantaneous or memoryless systems More precisely a system is said to be instantaneous or memoryless if its output at any instant t depends at most on the strength of its inputs at the same instant t and not on any past or future values of the inputs Otherwise the system is said to be dynamic or a system with memory A system whose response at t is completely determined by the input signals over the past T seconds interval from tT to t is a finitememory system with a memory of T seconds Networks containing inductive and capacitive elements generally have infinite memory because the response of such networks at any instant t is determined by their inputs over the entire past t This is true for the RC circuit of Fig 126 EXAMPLE 112 Assessing System Memory Determine whether the following systems are memoryless a yt 1 2xt 1 b yt d dtxt and c yt t 1xt a In this case the output at time t 1 is just twice the input at the same time t 1 Since the output at a particular time depends only on the strength of the input at the same time the system is memoryless b Although it appears that the output yt at time t depends on the input xt at the same time t we know that the slope derivative of xt cannot be determined solely from a single point There must be some memory even if infinitesimally small involved This is confirmed by using the fundamental theorem of calculus to express the system as yt lim T0 xt xt T T Since the output at a particular time depends on more than just the input at the same time the system is not memoryless c The output yt at time t is just the input xt at the same time t multiplied by the timedependent coefficient t 1 Since the output at a particular time depends only on the strength of the input at the same time the system is memoryless 174 Causal and Noncausal Systems A causal also known as a physical or nonanticipative system is one for which the output at any instant t0 depends only on the value of the input xt for t t0 In other words the value of the output at the present instant depends only on the past and present values of the input xt not on its future values To put it simply in a causal system the output cannot start before the input is applied If the response starts before the input it means that the system knows the input in the 01LathiC01 2017925 1553 page 106 43 106 CHAPTER 1 SIGNALS AND SYSTEMS a Here the output is a reflection of the input We can easily use a counterexample to disprove the causality of this system The input xt δt 1 which is nonzero at t 1 produces an output yt δt 1 which is nonzero at t 1 a time 2 seconds earlier than the input Clearly the system is not causal b In this case the output at time t depends on the input at future time of t 1 Clearly the system is not causal c In this case the output at time t 1 depends on the input one second in the past at time t Since the output does not depend on future values of the input the system is causal WHY STUDY NONCAUSAL SYSTEMS The foregoing discussion may suggest that noncausal systems have no practical purpose This is not the case they are valuable in the study of systems for several reasons First noncausal systems are realizable when the independent variable is other than time eg space Consider for example an electric charge of density qx placed along the x axis for x 0 This charge density produces an electric field Ex that is present at every point on the x axis from x to In this case the input ie the charge density qx starts at x 0 but its output the electric field Ex begins before x 0 Clearly this spacecharge system is noncausal This discussion shows that only temporal systems systems with time as independent variable must be causal to be realizable The terms before and after have a special connection to causality only when the independent variable is time This connection is lost for variables other than time Nontemporal systems such as those occurring in optics can be noncausal and still realizable Moreover even for temporal systems such as those used for signal processing the study of noncausal systems is important In such systems we may have all input data prerecorded This often happens with speech geophysical and meteorological signals and with space probes In such cases the inputs future values are available to us For example suppose we had a set of input signal records available for the system described by Eq 126 We can then compute yt since for any t we need only refer to the records to find the inputs value 2 seconds before and 2 seconds after t Thus noncausal systems can be realized although not in real time We may therefore be able to realize a noncausal system provided we are willing to accept a time delay in the output Consider a system whose output ˆyt is the same as yt in Eq 126 delayed by 2 seconds Fig 130c so that ˆyt yt 2 xt 4 xt Here the value of the output ˆy at any instant t is the sum of the values of the input x at t and at the instant 4 seconds earlier at t 4 In this case the output at any instant t does not depend on future values of the input and the system is causal The output of this system which is ˆyt is identical to that in Eq 126 or Fig 130b except for a delay of 2 seconds Thus a noncausal system may be realized or satisfactorily approximated in real time by using a causal system with a delay A third reason for studying noncausal systems is that they provide an upper bound on the performance of causal systems For example if we wish to design a filter for separating a signal from noise then the optimum filter is invariably a noncausal system Although unrealizable this 01LathiC01 2017925 1553 page 109 46 17 Classification of Systems 109 176 Analog and Digital Systems Analog and digital signals are discussed in Sec 132 A system whose input and output signals are analog is an analog system a system whose input and output signals are digital is a digital system A digital computer is an example of a digital binary system Observe that a digital computer is a digital as well as a discretetime system 177 Invertible and Noninvertible Systems A system S performs certain operations on input signals If we can obtain the input xt back from the corresponding output yt by some operation the system S is said to be invertible When several different inputs result in the same output as in a rectifier it is impossible to obtain the input from the output and the system is noninvertible Therefore for an invertible system it is essential that every input have a unique output so that there is a onetoone mapping between an input and the corresponding output The system that achieves the inverse operation of obtaining xt from yt is the inverse system for S For instance if S is an ideal integrator then its inverse system is an ideal differentiator Consider a system S connected in tandem with its inverse Si as shown in Fig 133 The input xt to this tandem system results in signal yt at the output of S and the signal yt which now acts as an input to Si yields back the signal xt at the output of Si Thus Si undoes the operation of S on xt yielding back xt A system whose output is equal to the input for all possible inputs is an identity system Cascading a system with its inverse system as shown in Fig 133 results in an identity system In contrast a rectifier specified by an equation yt xt is noninvertible because the rectification operation cannot be undone Inverse systems are very important in signal processing In many applications the signals are distorted during the processing and it is necessary to undo the distortion For instance in transmis sion of data over a communication channel the signals are distorted owing to nonideal frequency response and finite bandwidth of a channel It is necessary to restore the signal as closely as possi ble to its original shape Such equalization is also used in audio systems and photographic systems Si S xt yt xt Figure 133 A cascade of a system with its inverse results in an identity system EXAMPLE 114 Assessing System Invertibility Determine whether the following systems are invertible a yt xt b yt txt and c yt d dtxt a Here the output is a reflection of the input which does not cause any loss to the input The input can in fact be exactly recovered by simply reflecting the output xt yt which is to say that a reflecting system is its own inverse Thus yt xt is an invertible system 01LathiC01 2017925 1553 page 110 47 110 CHAPTER 1 SIGNALS AND SYSTEMS b In this case one might be tempted to recover the input from the output as xt 1 t yt This approach works almost everywhere except at t 0 where the input value x0 cannot be recovered Due to this single lost point the system yt txt is not invertible c Differentiation eliminates any dc component For example the inputs x1t 1 and x2t 2 both produce the same output yt 0 Given only yt 0 it is impossible to know if the original input was x1t 1 x2t 2 or something else entirely Since unique inputs do produce unique outputs we know that yt d dtxt is not an invertible system 178 Stable and Unstable Systems Systems can also be classified as stable or unstable systems Stability can be internal or external If every bounded input applied at the input terminal results in a bounded output the system is said to be stable externally External stability can be ascertained by measurements at the external terminals input and output of the system This type of stability is also known as the stability in the BIBO boundedinputboundedoutput sense The concept of internal stability is postponed to Ch 2 because it requires some understanding of internal system behavior introduced in that chapter EXAMPLE 115 Assessing System BIBO Stability Determine whether the following systems are BIBOstable a yt x2t b yt txt and c yt d dtxt a This system squares an input to produce the output If the input is bounded which is to say that xt Mx for all t then we see that yt x2t xt2 M2 x Since the output amplitude is guaranteed to be bounded for any boundedamplitude input the system yt x2t is BIBOstable b We can prove that yt txt is not BIBOstable with a simple example The boundedamplitude input xt ut produces the output yt tut whose amplitude grows to infinity as t Thus yt txt is a BIBOunstable system c We can prove that yt d dtxt is not BIBOstable with an example The boundedamplitude input xt ut produces the output yt δt whose amplitude is infinite at t 0 Thus yt d dtxt is a BIBOunstable system DRILL 116 A Noninvertible BIBOStable System Show that a system described by the equation yt x2t is noninvertible but BIBOstable 01LathiC01 2017925 1553 page 114 51 114 CHAPTER 1 SIGNALS AND SYSTEMS b Multiplying both sides of Eq 130 by D ie differentiating the equation we obtain 15D 5it Dxt Using the fact that it C dyt dt 1 5Dyt simple substitution yields 3D 1yt xt 131 DRILL 117 InputOutput Equation of a Series RLC Circuit with Inductor Voltage as Output If the inductor voltage vLt is taken as the output show that the RLC circuit in Fig 134 has an inputoutput equation of D2 3D 2vLt D2xt DRILL 118 InputOutput Equation of a Series RC Circuit with Capacitor Voltage as Output If the capacitor voltage vCt is taken as the output show that the RLC circuit in Fig 134 has an inputoutput equation of D2 3D 2vCt 2xt 182 Mechanical Systems Planar motion can be resolved into translational rectilinear motion and rotational torsional motion Translational motion will be considered first We shall restrict ourselves to motions in one dimension TRANSLATIONAL SYSTEMS The basic elements used in modeling translational systems are ideal masses linear springs and dashpots providing viscous damping The laws of various mechanical elements are now discussed For a mass M Fig 136a a force xt causes a motion yt and acceleration yt From Newtons law of motion xt Myt M d2yt dt2 MD2yt The force xt required to stretch or compress a linear spring Fig 136b by an amount yt is given by xt Kyt where K is the stiffness of the spring 01LathiC01 2017925 1553 page 115 52 18 System Model InputOutput Description 115 M a b c K B xt xt xt yt yt yt Figure 136 Some elements in translational mechanical systems For a linear dashpot Fig 136c which operates by virtue of viscous friction the force moving the dashpot is proportional to the relative velocity yt of one surface with respect to the other Thus xt Byt Bdyt dt BDyt where B is the damping coefficient of the dashpot or the viscous friction EXAMPLE 118 InputOutput Equation for a Translational Mechanical System Find the inputoutput relationship for the translational mechanical system shown in Fig 137a or its equivalent in Fig 137b The input is the force xt and the output is the mass position yt M K B Frictionless K B b a M M Byt Kyt xt yt xt yt xt yt c Figure 137 Mechanical system for Ex 118 01LathiC01 2017925 1553 page 116 53 116 CHAPTER 1 SIGNALS AND SYSTEMS In mechanical systems it is helpful to draw a freebody diagram of each junction which is a point at which two or more elements are connected In Fig 137 the point representing the mass is a junction The displacement of the mass is denoted by yt The spring is also stretched by the amount yt and therefore it exerts a force Kyt on the mass The dashpot exerts a force Byt on the mass as shown in the freebody diagram Fig 137c By Newtons second law the net force must be Myt Therefore Myt Byt Kyt xt or MD2 BD Kyt xt ROTATIONAL SYSTEMS In rotational systems the motion of a body may be defined as its motion about a certain axis The variables used to describe rotational motion are torque in place of force angular position in place of linear position angular velocity in place of linear velocity and angular acceleration in place of linear acceleration The system elements are rotational mass or moment of inertia in place of mass and torsional springs and torsional dashpots in place of linear springs and dashpots The terminal equations for these elements are analogous to the corresponding equations for translational elements If J is the moment of inertia or rotational mass of a rotating body about a certain axis then the external torque required for this motion is equal to J rotational mass times the angular acceleration If θt is the angular position of the body θt is its angular acceleration and torque J θt J d2θt dt2 JD2θt Similarly if K is the stiffness of a torsional spring per unit angular twist and θ is the angular displacement of one terminal of the spring with respect to the other then torque Kθt Finally the torque due to viscous damping of a torsional dashpot with damping coefficient B is torque B θt BDθt EXAMPLE 119 InputOutput Equation for Aircraft Roll Angle The attitude of an aircraft can be controlled by three sets of surfaces shown shaded in Fig 138 elevators rudder and ailerons By manipulating these surfaces one can set the aircraft on a desired flight path The roll angle ϕt can be controlled by deflecting in the opposite direction the two aileron surfaces as shown in Fig 138 Assuming only rolling motion find the equation relating the roll angle ϕt to the input deflection θt 01LathiC01 2017925 1553 page 117 54 18 System Model InputOutput Description 117 u u Elevator Elevator Rudder Aileron Aileron x w Figure 138 Attitude control of an airplane The aileron surfaces generate a torque about the roll axis proportional to the aileron deflection angle θt Let this torque be cθt where c is the constant of proportionality Air friction dissipates the torque B ϕt The torque available for rolling motion is then cθt B ϕt If J is the moment of inertia of the plane about the x axis roll axis then net torque J ϕt cθt B ϕt and J d2ϕt dt2 Bdϕt dt cθt or JD2 BDϕt cθt This is the desired equation relating the output roll angle ϕt to the input aileron angle θt The roll velocity ωt is ϕt If the desired output is the roll velocity ωt rather than the roll angle ϕt then the inputoutput equation would be J dωt dt Bωt cθt or JD Bωt cθt DRILL 119 InputOutput Equation of a Rotational Mechanical System Torque T t is applied to the rotational mechanical system shown in Fig 139a The torsional spring stiffness is K the rotational mass the cylinders moment of inertia about the shaft is J the viscous damping coefficient between the cylinder and the ground is B Find the equation 01LathiC01 2017925 1553 page 118 55 118 CHAPTER 1 SIGNALS AND SYSTEMS relating the output angle θt to the input torque T t Hint A freebody diagram is shown in Fig 139b ANSWER J d2θt dt2 Bdθt dt Kθt T t or JD2 BD Kθt T t J K B b a Bu t ut Kut J J Figure 139 Rotational system for Drill 119 183 Electromechanical Systems A wide variety of electromechanical systems is used to convert electrical signals into mechanical motion mechanical energy and vice versa Here we consider a rather simple example of an armaturecontrolled dc motor driven by a current source xt as shown in Fig 140a The torque T t generated in the motor is proportional to the armature current xt Therefore T t KTxt where KT is a constant of the motor This torque drives a mechanical load whose freebody diagram is shown in Fig 140b The viscous damping with coefficient B dissipates a torque B θt If J is the moment of inertia of the load including the rotor of the motor then the net torque T tB θt must be equal to J θt J θt T t B θt Thus JD2 BDθt T t KTxt which in conventional form can be expressed as J d2θt dt2 Bdθt dt KT xt 132 01LathiC01 2017925 1553 page 126 63 126 CHAPTER 1 SIGNALS AND SYSTEMS 111 MATLAB WORKING WITH FUNCTIONS Working with functions is fundamental to signals and systems applications MATLAB provides several methods of defining and evaluating functions An understanding and proficient use of these methods are therefore necessary and beneficial 1111 Anonymous Functions Many simple functions are most conveniently represented by using MATLAB anonymous functions An anonymous function provides a symbolic representation of a function defined in terms of MATLAB operators functions or other anonymous functions For example consider defining the exponentially damped sinusoid ft et cos2πt f t exptcos2pit In this context the symbol identifies the expression as an anonymous function which is assigned a name of f Parentheses following the symbol are used to identify the functions independent variables input arguments which in this case is the single time variable t Input arguments such as t are local to the anonymous function and are not related to any workspace variables with the same names Once defined ft can be evaluated simply by passing the input values of interest For example t 0 ft ans 1 evaluates ft at t 0 confirming the expected result of unity The same result is obtained by passing t 0 directly f0 ans 1 Vector inputs allow the evaluation of multiple values simultaneously Consider the task of plotting ft over the interval 2 t 2 Gross function behavior is clear ft should oscillate four times with a decaying envelope Since accurate hand sketches are cumbersome MATLABgenerated plots are an attractive alternative As the following example illustrates care must be taken to ensure reliable results Suppose vector t is chosen to include only the integers contained in 2 t 2 namely 21012 t 22 This vector input is evaluated to form a vector output ft ans 73891 27183 10000 03679 01353 01LathiC01 2017925 1553 page 127 64 111 MATLAB Working with Functions 127 The plot command graphs the result which is shown in Fig 146 plottft xlabelt ylabelft grid Grid lines added by using the grid command aid feature identification Unfortunately the plot does not illustrate the expected oscillatory behavior More points are required to adequately represent ft The question then is how many points is enough If too few points are chosen information is lost If too many points are chosen memory and time are wasted A balance is needed For oscillatory functions plotting 20 to 200 points per oscillation is normally adequate For the present case t is chosen to give 100 points per oscillation t 20012 Again the function is evaluated and plotted 2 15 1 05 0 05 1 15 2 t 0 2 4 6 8 ft Figure 146 ft et cos2πt for t 22 2 15 1 05 0 05 1 15 2 t 5 0 5 10 ft Figure 147 ft et cos2πt for t 20012 Sampling theory presented later formally addresses important aspects of this question 01LathiC01 2017925 1553 page 128 65 128 CHAPTER 1 SIGNALS AND SYSTEMS plottft xlabelt ylabelft grid The result shown in Fig 147 is an accurate depiction of ft 1112 Relational Operators and the Unit Step Function The unit step function ut arises naturally in many practical situations For example a unit step can model the act of turning on a system With the help of relational operators anonymous functions can represent the unit step function In MATLAB a relational operator compares two items If the comparison is true a logical true 1 is returned If the comparison is false a logical false 0 is returned Sometimes called indicator functions relational operators indicates whether a condition is true Six relational operators are available and The unit step function is readily defined using the relational operator u t 10t0 Any function with a jump discontinuity such as the unit step is difficult to plot Consider plotting ut by using t 22 t 22 plottut xlabelt ylabelut Two significant problems are apparent in the resulting plot shown in Fig 148 First MATLAB automatically scales plot axes to tightly bound the data In this case this normally desirable feature obscures most of the plot Second MATLAB connects plot data with lines making a true jump discontinuity difficult to achieve The coarse resolution of vector t emphasizes the effect by showing an erroneous sloping line between t 1 and t 0 The first problem is corrected by vertically enlarging the bounding box with the axis command The second problem is reduced but not eliminated by adding points to vector t 2 15 1 05 0 05 1 15 2 t 0 05 1 ut Figure 148 ut for t 22 01LathiC01 2017925 1553 page 129 66 111 MATLAB Working with Functions 129 2 15 1 05 0 05 1 15 2 t 0 05 1 ut Figure 149 ut for t 20012 with axis modification t 20012 plottut xlabelt ylabelut axis2 2 01 11 The fourelement vector argument of axis specifies x axis minimum x axis maximum y axis minimum and y axis maximum respectively The improved results are shown in Fig 149 Relational operators can be combined using logical AND logical OR and logical negation and respectively For example t0t1 and t0t1 both test if 0 t 1 To demonstrate consider defining and plotting the unit pulse pt ut ut 1 as shown in Fig 150 p t 10t0t1 t 10012 plottpt xlabelt ylabelpt utut1 axis1 2 1 11 Since anonymous functions can be constructed using other anonymous functions we could have used our previously defined unit step anonymous function to define pt as p t utut1 1 05 0 05 1 15 2 t 0 05 1 pt utut1 Figure 150 pt ut ut 1 over 1 t 2 01LathiC01 2017925 1553 page 130 67 130 CHAPTER 1 SIGNALS AND SYSTEMS For scalar operands MATLAB also supports two shortcircuit logical constructs A shortcircuit logical AND is performed by using and a shortcircuit logical OR is performed by using Shortcircuit logical operators are often more efficient than traditional logical operators because they test the second portion of the expression only when necessary That is when scalar expression A is found false in AB scalar expression B is not evaluated since a false result is already guaranteed Similarly scalar expression B is not evaluated when scalar expression A is found true in AB since a true result is already guaranteed 1113 Visualizing Operations on the Independent Variable Two operations on a functions independent variable are commonly encountered shifting and scaling Anonymous functions are well suited to investigate both operations Consider gt ftut et cos2πtut a causal version of ft MATLAB easily multiplies anonymous functions Thus we create gt by multiplying our anonymous functions for ft and ut g t ftut A combined shifting and scaling operation is represented by gat b where a and b are arbitrary real constants As an example consider plotting g2t 1 over 2 t 2 With a 2 the function is compressed by a factor of 2 resulting in twice the oscillations per unit t Adding the condition b 0 shifts the waveform to the left Given anonymous function g an accurate plot is nearly trivial to obtain t 20012 plottg2t1 xlabelt ylabelg2t1 grid Figure 151 confirms the expected waveform compression and left shift As a final check realize that function g turns on when the input argument is zero Therefore g2t 1 should turn on when 2t 1 0 or at t 05 a fact again confirmed by Fig 151 2 15 1 05 0 05 1 15 2 t 1 05 0 05 1 g2t1 Figure 151 g2t 1 over 2 t 2 Although we define g in terms of f and u the function g will not change if we later change either f or u unless we subsequently redefine g as well 01LathiC01 2017925 1553 page 131 68 111 MATLAB Working with Functions 131 2 15 1 05 0 05 1 15 2 t 1 05 0 05 1 gt1 Figure 152 gt 1 over 2 t 2 2 15 1 05 0 05 1 15 2 t 1 05 0 05 1 15 ht Figure 153 ht g2t 1 gt 1 over 2 t 2 Next consider plotting gt 1 over 2 t 2 Since a 0 the waveform will be reflected Adding the condition b 0 shifts the final waveform to the right plottgt1 xlabelt ylabelgt1 grid Figure 152 confirms both the reflection and the right shift Up to this point Figs 151 and 152 could be reasonably sketched by hand Consider plotting the more complicated function ht g2t 1 gt 1 over 2 t 2 Fig 153 an accurate hand sketch would be quite difficult With MATLAB the work is much less burdensome plottg2t1gt1 xlabelt ylabelht grid 1114 Numerical Integration and Estimating Signal Energy Interesting signals often have nontrivial mathematical representations Computing signal energy which involves integrating the square of these expressions can be a daunting task Fortunately many difficult integrals can be accurately estimated by means of numerical integration techniques 01LathiC01 2017925 1553 page 134 71 134 CHAPTER 1 SIGNALS AND SYSTEMS 4 An everlasting signal starts at t and continues forever to t Hence periodic signals are everlasting signals A causal signal is a signal that is zero for t 0 5 A signal with finite energy is an energy signal Similarly a signal with a finite and nonzero power meansquare value is a power signal A signal can be either an energy signal or a power signal but not both However there are signals that are neither energy nor power signals 6 A signal whose physical description is known completely in a mathematical or graphical form is a deterministic signal A random signal is known only in terms of its probabilistic description such as mean value or meansquare value rather than by its mathematical or graphical form A signal xt delayed by T seconds rightshifted can be expressed as xt T on the other hand xt advanced by T leftshifted is xt T A signal xt timecompressed by a factor aa 1 is expressed as xat on the other hand the same signal timeexpanded by factor aa 1 is xta The signal xt when timereversed can be expressed as xt The unit step function ut is very useful in representing causal signals and signals with different mathematical descriptions over different intervals In the classical Dirac definition the unit impulse function δt is characterized by unit area and is concentrated at a single instant t 0 The impulse function has a sampling or sifting property which states that the area under the product of a function with a unit impulse is equal to the value of that function at the instant at which the impulse is located assuming the function to be continuous at the impulse location In the modern approach the impulse function is viewed as a generalized function and is defined by the sampling property The exponential function est where s is complex encompasses a large class of signals that includes a constant a monotonic exponential a sinusoid and an exponentially varying sinusoid A real signal that is symmetrical about the vertical axis t 0 is an even function of time and a real signal that is antisymmetrical about the vertical axis is an odd function of time The product of an even function and an odd function is an odd function However the product of an even function and an even function or an odd function and an odd function is an even function The area under an odd function from t a to a is always zero regardless of the value of a On the other hand the area under an even function from t a to a is two times the area under the same function from t 0 to a or from t a to 0 Every signal can be expressed as a sum of odd and even functions of time A system processes input signals to produce output signals response The input is the cause and the output is its effect In general the output is affected by two causes the internal conditions of the system such as the initial conditions and the external input Systems can be classified in several ways 1 Linear systems are characterized by the linearity property which implies superposition if several causes such as various inputs and initial conditions are acting on a linear system the total output response is the sum of the responses from each cause assuming that all the remaining causes are absent A system is nonlinear if superposition does not hold 2 In timeinvariant systems system parameters do not change with time The parameters of timevaryingparameter systems change with time 3 For memoryless or instantaneous systems the system response at any instant t depends only on the value of the input at t For systems with memory also known as dynamic 01LathiC01 2017925 1553 page 135 72 112 Summary 135 systems the system response at any instant t depends not only on the present value of the input but also on the past values of the input values before t 4 In contrast if a system response at t also depends on the future values of the input values of input beyond t the system is noncausal In causal systems the response does not depend on the future values of the input Because of the dependence of the response on the future values of input the effect response of noncausal systems occurs before the cause When the independent variable is time temporal systems the noncausal systems are prophetic systems and therefore unrealizable although close approximation is possible with some time delay in the response Noncausal systems with independent variables other than time eg space are realizable 5 Systems whose inputs and outputs are continuoustime signals are continuoustime systems systems whose inputs and outputs are discretetime signals are discretetime systems If a continuoustime signal is sampled the resulting signal is a discretetime signal We can process a continuoustime signal by processing the samples of the signal with a discretetime system 6 Systems whose inputs and outputs are analog signals are analog systems those whose inputs and outputs are digital signals are digital systems 7 If we can obtain the input xt back from the output yt of a system S by some operation the system S is said to be invertible Otherwise the system is noninvertible 8 A system is stable if bounded input produces bounded output This defines external stability because it can be ascertained from measurements at the external terminals of the system External stability is also known as the stability in the BIBO boundedinputboundedoutput sense Internal stability discussed later in Ch 2 is measured in terms of the internal behavior of the system The system model derived from a knowledge of the internal structure of the system is its internal description In contrast an external description is a representation of a system as seen from its input and output terminals it can be obtained by applying a known input and measuring the resulting output In the majority of practical systems an external description of a system so obtained is equivalent to its internal description At times however the external description fails to describe the system adequately Such is the case with the socalled uncontrollable or unobservable systems A system may also be described in terms of certain set of key variables called state variables In this description an Nthorder system can be characterized by a set of N simultaneous firstorder differential equations in N state variables State equations of a system represent an internal description of that system REFERENCES 1 Papoulis A The Fourier Integral and Its Applications McGrawHill New York 1962 2 Mason S J Electronic Circuits Signals and Systems Wiley New York 1960 3 Kailath T Linear Systems PrenticeHall Englewood Cliffs NJ 1980 4 Lathi B P Signals and Systems BerkeleyCambridge Press Carmichael CA 1987 02LathiC02 2017925 1554 page 150 1 C H A P T E R TIMEDOMAIN ANALYSIS OF CONTINUOUSTIME SYSTEMS 2 In this book we consider two methods of analysis of linear timeinvariant LTI systems the timedomain method and the frequencydomain method In this chapter we discuss the timedomain analysis of linear timeinvariant continuoustime LTIC systems 21 INTRODUCTION For the purpose of analysis we shall consider linear differential systems This is the class of LTIC systems introduced in Ch 1 for which the input xt and the output yt are related by linear differential equations of the form dNyt dtN a1 dN1yt dtN1 aN1 dyt dt aNyt bNM dMxt dtM bNM1 dM1xt dtM1 bN1 dxt dt bNxt 21 where all the coefficients ai and bi are constants Using operator notation D to represent ddt we can express this equation as DN a1DN1 aN1D aNyt bNMDM bNM1DM1 bN1D bNxt or QDyt PDxt 22 where the polynomials QD and PD are QD DN a1DN1 aN1D aN PD bNMDM bNM1DM1 bN1D bN Theoretically the powers M and N in the foregoing equations can take on any value However practical considerations make M N undesirable for two reasons In Sec 433 we shall show that 150 02LathiC02 2017925 1554 page 151 2 22 System Response to Internal Conditions The ZeroInput Response 151 an LTIC system specified by Eq 21 acts as an M Nthorder differentiator A differentiator represents an unstable system because a bounded input like the step input results in an unbounded output δt Second noise is enhanced by a differentiator Noise is a wideband signal containing components of all frequencies from 0 to a very high frequency approaching Hence noise contains a significant amount of rapidly varying components We know that the derivative of any rapidly varying signal is high Therefore any system specified by Eq 21 in which M N will magnify the highfrequency components of noise through differentiation It is entirely possible for noise to be magnified so much that it swamps the desired system output even if the noise signal at the systems input is tolerably small Hence practical systems generally use M N For the rest of this text we assume implicitly that M N For the sake of generality we shall assume M N in Eq 21 In Ch 1 we demonstrated that a system described by Eq 22 is linear Therefore its response can be expressed as the sum of two components the zeroinput response and the zerostate response decomposition property Therefore total response zeroinput response zerostate response The zeroinput response is the system output when the input xt 0 and thus it is the result of internal system conditions such as energy storages initial conditions alone It is independent of the external input xt In contrast the zerostate response is the system output to the external input xt when the system is in zero state meaning the absence of all internal energy storages that is all initial conditions are zero 22 SYSTEM RESPONSE TO INTERNAL CONDITIONS THE ZEROINPUT RESPONSE The zeroinput response y0t is the solution of Eq 22 when the input xt 0 so that QDy0t 0 Noise is any undesirable signal natural or manufactured that interferes with the desired signals in the system Some of the sources of noise are the electromagnetic radiation from stars the random motion of electrons in system components interference from nearby radio and television stations transients produced by automobile ignition systems and fluorescent lighting We can verify readily that the system described by Eq 22 has the decomposition property If y0t is the zeroinput response then by definition QDy0t 0 If yt is the zerostate response then yt is the solution of QDyt PDxt subject to zero initial conditions zerostate Adding these two equations we have QDy0t yt PDxt Clearly y0t yt is the general solution of Eq 22 02LathiC02 2017925 1554 page 152 3 152 CHAPTER 2 TIMEDOMAIN ANALYSIS OF CONTINUOUSTIME SYSTEMS or DN a1DN1 aN1D aNy0t 0 23 A solution to this equation can be obtained systematically 1 However we will take a shortcut by using heuristic reasoning Equation 23 shows that a linear combination of y0t and its N successive derivatives is zero not at some values of t but for all t Such a result is possible if and only if y0t and all its N successive derivatives are of the same form Otherwise their sum can never add to zero for all values of t We know that only an exponential function eλt has this property So let us assume that y0t ceλt is a solution to Eq 23 Then Dy0t dy0t dt cλeλt D2y0t d2y0t dt2 cλ2eλt DNy0t dNy0t dtN cλNeλt Substituting these results in Eq 23 we obtain cλN a1λN1 aN1λ aNeλt 0 For a nontrivial solution of this equation λN a1λN1 aN1λ aN 0 24 This result means that ceλt is indeed a solution of Eq 23 provided λ satisfies Eq 24 Note that the polynomial in Eq 24 is identical to the polynomial QD in Eq 23 with λ replacing D Therefore Eq 24 can be expressed as Qλ 0 Expressing Qλ in factorized form we obtain Qλ λ λ1λ λ2 λ λN 0 25 Clearly λ has N solutions λ1 λ2 λN assuming that all λi are distinct Consequently Eq 23 has N possible solutions c1eλ1t c2eλ2t cNeλNt with c1 c2 cN as arbitrary constants We 02LathiC02 2017925 1554 page 153 4 22 System Response to Internal Conditions The ZeroInput Response 153 can readily show that a general solution is given by the sum of these N solutions so that y0t c1eλ1t c2eλ2t cNeλNt 26 where c1 c2 cN are arbitrary constants determined by N constraints the auxiliary conditions on the solution Observe that the polynomial Qλ which is characteristic of the system has nothing to do with the input For this reason the polynomial Qλ is called the characteristic polynomial of the system The equation Qλ 0 is called the characteristic equation of the system Equation 25 clearly indicates that λ1 λ2 λN are the roots of the characteristic equation consequently they are called the characteristic roots of the system The terms characteristic values eigenvalues and natural frequencies are also used for characteristic roots The exponentials eλit i 12 n in the zeroinput response are the characteristic modes also known as natural modes or simply as modes of the system There is a characteristic mode for each characteristic root of the system and the zeroinput response is a linear combination of the characteristic modes of the system An LTIC systems characteristic modes comprise its single most important attribute Characteristic modes not only determine the zeroinput response but also play an important role in determining the zerostate response In other words the entire behavior of a system is dictated primarily by its characteristic modes In the rest of this chapter we shall see the pervasive presence of characteristic modes in every aspect of system behavior REPEATED ROOTS The solution of Eq 23 as given in Eq 26 assumes that the N characteristic roots λ1 λ2 λN are distinct If there are repeated roots same root occurring more than once the form of the solution is modified slightly By direct substitution we can show that the solution of the equation D λ2y0t 0 is given by y0t c1 c2teλt To prove this assertion assume that y1t y2t yNt are all solutions of Eq 23 Then QDy1t 0 QDy2t 0 QDyNt 0 Multiplying these equations by c1 c2 cN respectively and adding them together yield QDc1y1t c2y2t cNynt 0 This result shows that c1y1t c2y2t cNynt is also a solution of the homogeneous equation Eq 23 Eigenvalue is German for characteristic value 02LathiC02 2017925 1554 page 155 6 22 System Response to Internal Conditions The ZeroInput Response 155 EXAMPLE 21 Finding the ZeroInput Response Find y0t the zeroinput response of the response for an LTIC system described by a the simpleroot system D2 3D 2yt Dxt with initial conditions y00 0 and y00 5 b the repeatedroot system D2 6D 9yt 3D 5xt with initial conditions y00 3 and y00 7 c the complexroot system D2 4D 40yt D 2xt with initial conditions y00 2 and y00 1678 a Note that y0t being the zeroinput response xt 0 is the solution of D2 3D 2y0t 0 The characteristic polynomial of the system is λ2 3λ 2 The characteristic equation of the system is therefore λ2 3λ 2 λ 1λ 2 0 The characteristic roots of the system are λ1 1 and λ2 2 and the characteristic modes of the system are et and e2t Consequently the zeroinput response is y0t c1et c2e2t Differentiating this expression we obtain y0t c1et 2c2e2t To determine the constants c1 and c2 we set t 0 in the equations for y0t and y0t and substitute the initial conditions y00 0 and y00 5 yielding 0 c1 c2 5 c1 2c2 Solving these two simultaneous equations in two unknowns for c1 and c2 yields c1 5 and c2 5 Therefore y0t 5et 5e2t 29 This is the zeroinput response of yt Because y0t is present at t 0 we are justified in assuming that it exists for t 0 b The characteristic polynomial is λ2 6λ 9 λ 32 and its characteristic roots are λ1 3 λ2 3 repeated roots Consequently the characteristic modes of the system are e3t and te3t The zeroinput response being a linear combination of the characteristic modes is given by y0t c1 c2te3t y0t may be present even before t 0 However we can be sure of its presence only from t 0 onward 02LathiC02 2017925 1554 page 157 8 22 System Response to Internal Conditions The ZeroInput Response 157 EXAMPLE 22 Using MATLAB to Find Polynomial Roots Find the roots λ1 and λ2 of the polynomial λ2 4λ k for three values of k a k 3 b k 4 and c k 40 a r roots1 4 3 r 3 1 For k 3 the polynomial roots are therefore λ1 3 and λ2 1 b r roots1 4 4 r 2 2 For k 4 the polynomial roots are therefore λ1 λ2 2 c r roots1 4 40 r 200600i 200600i For k 40 the polynomial roots are therefore λ1 2 j6 and λ2 2 j6 EXAMPLE 23 Using MATLAB to Find the ZeroInput Response Consider an LTIC system specified by the differential equation D2 4D kyt 3D 5xt Using initial conditions y00 3 and y00 7 apply MATLABs dsolve command to determine the zeroinput response when a k 3 b k 4 and c k 40 a y0 dsolveD2y4Dy3y0y03Dy07t y0 1expt 2exp3t For k 3 the zeroinput response is therefore y0t et 2e3t b y0 dsolveD2y4Dy4y0y03Dy07t y0 3exp2t texp2t 02LathiC02 2017925 1554 page 158 9 158 CHAPTER 2 TIMEDOMAIN ANALYSIS OF CONTINUOUSTIME SYSTEMS For k 4 the zeroinput response is therefore y0t 3e2t te2t c y0 dsolveD2y4Dy40y0y03Dy07t y0 3cos6texp2t sin6t6exp2t For k 40 the zeroinput response is therefore y0t 3e2t cos6t 1 6e2t sin6t DRILL 21 Finding the ZeroInput Response of a FirstOrder System Find the zeroinput response of an LTIC system described by D 5yt xt if the initial condition is y0 5 ANSWER y0t 5e5t t 0 DRILL 22 Finding the ZeroInput Response of a SecondOrder System Letting y00 1 and y00 4 solve D2 2Dy0t 0 ANSWER y0t 3 2e2t t 0 PRACTICAL INITIAL CONDITIONS AND THE MEANING OF 0 AND 0 In Ex 21 the initial conditions y00 and y00 were supplied In practical problems we must derive such conditions from the physical situation For instance in an RLC circuit we may be given the conditions initial capacitor voltages initial inductor currents etc From this information we need to derive y00 y00 for the desired variable as demonstrated in the next example In much of our discussion the input is assumed to start at t 0 unless otherwise mentioned Hence t 0 is the reference point The conditions immediately before t 0 just before the input is applied are the conditions at t 0 and those immediately after t 0 just after the input is applied are the conditions at t 0 compare this with the historical time frames BCE and CE In 02LathiC02 2017925 1554 page 159 10 22 System Response to Internal Conditions The ZeroInput Response 159 practice we are likely to know the initial conditions at t 0 rather than at t 0 The two sets of conditions are generally different although in some cases they may be identical The total response yt consists of two components the zeroinput response y0t response due to the initial conditions alone with xt 0 and the zerostate response resulting from the input alone with all initial conditions zero At t 0 the total response yt consists solely of the zeroinput response y0t because the input has not started yet Hence the initial conditions on yt are identical to those of y0t Thus y0 y00 y0 y00 and so on Moreover y0t is the response due to initial conditions alone and does not depend on the input xt Hence application of the input at t 0 does not affect y0t This means the initial conditions on y0t at t 0 and 0 are identical that is y00 y00 are identical to y00 y00 respectively It is clear that for y0t there is no distinction between the initial conditions at t 0 0 and 0 They are all the same But this is not the case with the total response yt which consists of both the zeroinput and zerostate responses Thus in general y0 y0 y0 y0 and so on EXAMPLE 24 Consideration of Initial Conditions A voltage xt 10e3tut is applied at the input of the RLC circuit illustrated in Fig 22a Find the loop current yt for t 0 if the initial inductor current is zero y0 0 and the initial capacitor voltage is 5 volts vC0 5 The differential loop equation relating yt to xt was derived in Eq 129 as D2 3D 2yt Dxt The zerostate component of yt resulting from the input xt assuming that all initial conditions are zero that is y0 vC0 0 will be obtained later in Ex 29 In this example we shall find the zeroinput reponse y0t For this purpose we need two initial conditions y00 and y00 These conditions can be derived from the given initial conditions y0 0 and vC0 5 as follows Recall that y0t is the loop current when the input terminals are shorted so that the input xt 0 zeroinput as depicted in Fig 22b We now compute y00 and y00 the values of the loop current and its derivative at t 0 from the initial values of the inductor current and the capacitor voltage Remember that the inductor current cannot change instantaneously in the absence of an impulsive voltage Similarly the capacitor voltage cannot change instantaneously in the absence of an impulsive current Therefore when the input terminals are shorted at t 0 the inductor current is still zero and the capacitor voltage is still 5 volts Thus y00 0 02LathiC02 2017925 1554 page 161 12 22 System Response to Internal Conditions The ZeroInput Response 161 The loop current y0 y0 0 because it cannot change instantaneously in the absence of impulsive voltage The same is true of the capacitor voltage Hence vC0 vC0 5 Substituting these values in the foregoing equations we obtain y0 5 and y0 5 Thus y0 0 y0 5 and y0 0 y0 5 210 DRILL 23 ZeroInput Response of an RC Circuit In the circuit in Fig 22a the inductance L 0 and the initial capacitor voltage vC0 30 volts Show that the zeroinput component of the loop current is given by y0t 10e2t3 for t 0 INDEPENDENCE OF THE ZEROINPUT AND ZEROSTATE RESPONSES In Ex 24 we computed the zeroinput component without using the input xt The zerostate response can be computed from the knowledge of the input xt alone the initial conditions are assumed to be zero system in zero state The two components of the system response the zeroinput and zerostate responses are independent of each other The two worlds of zeroinput response and zerostate response coexist side by side neither one knowing or caring what the other is doing For each component the other is totally irrelevant ROLE OF AUXILIARY CONDITIONS IN SOLUTION OF DIFFERENTIAL EQUATIONS The solution of a differential equation requires additional pieces of information the auxiliary conditions Why We now show heuristically why a differential equation does not in general have a unique solution unless some additional constraints or conditions on the solution are known Differentiation operation is not invertible unless one piece of information about yt is given To get back yt from dydt we must know one piece of information such as y0 Thus differentiation is an irreversible noninvertible operation during which certain information is lost To invert this operation one piece of information about yt must be provided to restore the original yt Using a similar argument we can show that given d2ydt2 we can determine yt uniquely only if two additional pieces of information constraints about yt are given In general to determine yt uniquely from its Nth derivative we need N additional pieces of information constraints about yt These constraints are also called auxiliary conditions When these conditions are given at t 0 they are called initial conditions 221 Some Insights into the ZeroInput Behavior of a System By definition the zeroinput response is the system response to its internal conditions assuming that its input is zero Understanding this phenomenon provides interesting insight into system behavior If a system is disturbed momentarily from its rest position and if the disturbance is then 02LathiC02 2017925 1554 page 163 14 23 The Unit Impulse Response ht 163 Clearly the loop current yt ce2t is sustained by the RL circuit on its own without the necessity of an external input THE RESONANCE PHENOMENON We have seen that any signal consisting of a systems characteristic mode is sustained by the system on its own the system offers no obstacle to such signals Imagine what would happen if we were to drive the system with an external input that is one of its characteristic modes This would be like pouring gasoline on a fire in a dry forest or hiring a child to eat ice cream A child would gladly do the job without pay Think what would happen if he were paid by the amount of ice cream he ate He would work overtime He would work day and night until he became sick The same thing happens with a system driven by an input of the form of characteristic mode The system response grows without limit until it burns out We call this behavior the resonance phenomenon An intelligent discussion of this important phenomenon requires an understanding of the zerostate response for this reason we postpone this topic until Sec 267 23 THE UNIT IMPULSE RESPONSE ht In Ch 1 we explained how a system response to an input xt may be found by breaking this input into narrow rectangular pulses as illustrated earlier in Fig 127a and then summing the system response to all the components The rectangular pulses become impulses in the limit as their widths approach zero Therefore the system response is the sum of its responses to various impulse components This discussion shows that if we know the system response to an impulse input we can determine the system response to an arbitrary input xt We now discuss a method of determining ht the unit impulse response of an LTIC system described by the Nthorder differential equation Eq 21 dNyt dtN a1 dN1yt dtN1 aN1 dyt dt aNyt bNM dMxt dtM bNM1 dM1xt dtM1 bN1 dxt dt bNxt Recall that noise considerations restrict practical systems to M N Under this constraint the most general case is M N Therefore Eq 21 can be expressed as DN a1DN1 aN1D aNyt b0DN b1DN1 bN1D bNxt 211 Before deriving the general expression for the unit impulse response ht it is illuminating to understand qualitatively the nature of ht The impulse response ht is the system response to an impulse input δt applied at t 0 with all the initial conditions zero at t 0 An impulse input δt is like lightning which strikes instantaneously and then vanishes But in its wake in that single moment objects that have been struck are rearranged Similarly an impulse input δt appears momentarily at t 0 and then it is gone forever But in that moment it generates energy storages that is it creates nonzero initial conditions instantaneously within the system at In practice the system in resonance is more likely to go in saturation because of high amplitude levels 02LathiC02 2017925 1554 page 164 15 164 CHAPTER 2 TIMEDOMAIN ANALYSIS OF CONTINUOUSTIME SYSTEMS t 0 Although the impulse input δt vanishes for t 0 so that the system has no input after the impulse has been applied the system will still have a response generated by these newly created initial conditions The impulse response ht therefore must consist of the systems characteristic modes for t 0 As a result ht characteristic mode terms t 0 This response is valid for t 0 But what happens at t 0 At a single moment t 0 there can at most be an impulse so the form of the complete response ht is ht A0δt characteristic mode terms t 0 212 because ht is the unit impulse response Setting xt δt and yt ht in Eq 211 yields DN a1DN1 aN1D aNht b0DN b1DN1 bN1D bNδt In this equation we substitute ht from Eq 212 and compare the coefficients of similar impulsive terms on both sides The highest order of the derivative of impulse on both sides is N with its coefficient value as A0 on the lefthand side and b0 on the righthand side The two values must be matched Therefore A0 b0 and ht b0δt characteristic modes 213 In Eq 211 if M N b0 0 Hence the impulse term b0δt exists only if M N The unknown coefficients of the N characteristic modes in ht in Eq 213 can be determined by using the technique of impulse matching as explained in the following example EXAMPLE 25 Impulse Response via Impulse Matching Find the impulse response ht for a system specified by D2 5D 6yt D 1xt 214 In this case b0 0 Hence ht consists of only the characteristic modes The characteristic polynomial is λ2 5λ 6 λ 2λ 3 The roots are 2 and 3 Hence the impulse It might be possible for the derivatives of δt to appear at the origin However if M N it is impossible for ht to have any derivatives of δt This conclusion follows from Eq 211 with xt δt and yt ht The coefficients of the impulse and all its derivatives must be matched on both sides of this equation If ht contains δ1t the first derivative of δt the lefthand side of Eq 211 will contain a term δN1t But the highestorder derivative term on the righthand side is δNt Therefore the two sides cannot match Similar arguments can be made against the presence of the impulses higherorder derivatives in ht 02LathiC02 2017925 1554 page 167 18 23 The Unit Impulse Response ht 167 Comment In the above discussion we have assumed M N as specified by Eq 211 Section 28 shows that the expression for ht applicable to all possible values of M and N is given by ht PDyntut where ynt is a linear combination of the characteristic modes of the system subject to initial conditions Eq 218 This expression reduces to Eq 217 when M N Determination of the impulse response ht using the procedures in this section is relatively simple However in Ch 4 we shall discuss another even simpler method using the Laplace transform As the next example demonstrates it is also possible to find ht using functions from MATLABs symbolic math toolbox EXAMPLE 27 Using MATLAB to Find the Impulse Response Determine the impulse response ht for an LTIC system specified by the differential equation D2 3D 2yt Dxt This is a secondorder system with b0 0 First we find the zeroinput component for initial conditions y0 0 and y0 1 Since PD D the zeroinput response is differentiated and the impulse response immediately follows as ht 0δt Dyntut yn dsolveD2y3Dy2y0y00Dy01t h diffyn h 2exp2t 1expt Therefore ht 2e2t etut DRILL 24 Finding the Impulse Response Determine the unit impulse response of LTIC systems described by the following equations a D 2yt 3D 5xt b DD 2yt D 4xt c D2 2D 1yt Dxt ANSWERS a 3δt e2tut b 2 e2tut c 1 tetut 02LathiC02 2017925 1554 page 175 26 24 System Response to External Input The ZeroState Response 175 DRILL 26 ZeroState Response with Resonance Repeat Drill 25 for the input xt etut ANSWER 6tetut THE CONVOLUTION TABLE The task of convolution is considerably simplified by a readymade convolution table Table 21 This table which lists several pairs of signals and their convolution can conveniently determine yt a system response to an input xt without performing the tedious job of integration For instance we could have readily found the convolution in Ex 28 by using pair 4 with λ1 1 and λ2 2 to be et e2tut The following example demonstrates the utility of this table EXAMPLE 29 Convolution by Tables Use Table 21 to compute the loop current yt of the RLC circuit in Ex 24 for the input xt 10e3tut when all the initial conditions are zero The loop equation for this circuit see Ex 116 or Eq 129 is D2 3D 2yt Dxt The impulse response ht for this system as obtained in Ex 26 is ht 2e2t etut The input is xt 10e3tut and the response yt is yt xt ht 10e3tut 2e2t etut Using the distributive property of the convolution Eq 226 we obtain yt 10e3tut 2e2tut 10e3tut etut 20e3tut e2tut 10e3tut etut Now the use of pair 4 in Table 21 yields yt 20 3 2e3t e2tut 10 3 1e3t etut 20e3t e2tut 5e3t etut 5et 20e2t 15e3tut 02LathiC02 2017925 1554 page 180 31 180 CHAPTER 2 TIMEDOMAIN ANALYSIS OF CONTINUOUSTIME SYSTEMS A similar procedure is followed in computing the value of ct at t t2 where t2 is negative Fig 27g In this case the function gτ is shifted by a negative amount that is leftshifted to obtain gt2τ Multiplication of this function with xτ yields the product xτgt2τ The area under this product is ct2 A2 giving us another point on the curve ct at t t2 Fig 27i This procedure can be repeated for all values of t from to The result will be a curve describing ct for all time t Note that when t 3xτ and gtτ do not overlap see Fig 27h therefore ct 0 for t 3 SUMMARY OF THE GRAPHICAL PROCEDURE The procedure for graphical convolution can be summarized as follows 1 Keep the function xτ fixed 2 Visualize the function gτ as a rigid wire frame and rotate or invert this frame about the vertical axis τ 0 to obtain gτ 3 Shift the inverted frame along the τ axis by t0 seconds The shifted frame now represents gt0 τ 4 The area under the product of xτ and gt0 τ the shifted frame is ct0 the value of the convolution at t t0 5 Repeat this procedure shifting the frame by different values positive and negative to obtain ct for all values of t The graphical procedure discussed here appears very complicated and discouraging at first reading Indeed some people claim that convolution has driven many electrical engineering undergraduates to contemplate theology either for salvation or as an alternative career IEEE Spectrum March 1991 p 60 Actually the bark of convolution is worse than its bite In graphical convolution we need to determine the area under the product xτgt τ for all values of t from to However a mathematical description of xτgt τ is generally valid over a range Convolution Its bark is worse than its bite 02LathiC02 2017925 1554 page 187 38 24 System Response to External Input The ZeroState Response 187 Both Eqs 233 and 234 apply at the transition point t 2 We can readily verify that c2 43 when either of these expressions is used For t 4 xt τ has been shifted so far to the right that it no longer overlaps with gτ as depicted in Fig 210g Consequently ct 0 t 4 We now turn our attention to negative values of t We have already determined ct up to t 1 For t 1 there is no overlap between the two functions as illustrated in Fig 210h so that ct 0 t 1 Combining our results we see that ct 1 6t 12 1 t 1 2 3t 1 t 2 1 6t2 2t 8 2 t 4 0 otherwise Figure 210i plots ct according to this expression THE WIDTH OF CONVOLVED FUNCTIONS The widths durations of xt gt and ct in Ex 212 Fig 210 are 2 3 and 5 respectively Note that the width of ct in this case is the sum of the widths of xt and gt This observation is not a coincidence Using the concept of graphical convolution we can readily see that if xt and gt have the finite widths of T1 and T2 respectively then the width of ct is equal to T1 T2 The reason is that the time it takes for a signal of width duration T1 to completely pass another signal of width duration T2 so that they become nonoverlapping is T1T2 When the two signals become nonoverlapping the convolution goes to zero DRILL 210 Interchanging Convolution Order Rework Ex 211 by evaluating gt xt DRILL 211 Showing Commutability Using Two Causal Signals Use graphical convolution to show that xt gt gt xt ct in Fig 211 02LathiC02 2017925 1554 page 189 40 24 System Response to External Input The ZeroState Response 189 THE PHANTOM OF THE SIGNALS AND SYSTEMS OPERA In the study of signals and systems we often come across some signals such as an impulse which cannot be generated in practice and have never been sighted by anyone One wonders why we even consider such idealized signals The answer should be clear from our discussion so far in this chapter Even if the impulse function has no physical existence we can compute the system response ht to this phantom input according to the procedure in Sec 23 and knowing ht we can compute the system response to any arbitrary input The concept of impulse response therefore provides an effective intermediary for computing system response to an arbitrary input In addition the impulse response ht itself provides a great deal of information and insight about the system behavior In Sec 26 we show that the knowledge of impulse response provides much valuable information such as the response time pulse dispersion and filtering properties of the system Many other useful insights about the system behavior can be obtained by inspection of ht Similarly in frequencydomain analysis discussed in later chapters we use an everlasting exponential or sinusoid to determine system response An everlasting exponential or sinusoid too is a phantom which nobody has ever seen and which has no physical existence But it provides another effective intermediary for computing the system response to an arbitrary input Moreover the system response to everlasting exponential or sinusoid provides valuable information and insight regarding the systems behavior Clearly idealized impulses and everlasting sinusoids are friendly and helpful spirits Interestingly the unit impulse and the everlasting exponential or sinusoid are the dual of each other in the timefrequency duality to be studied in Ch 7 Actually the timedomain and the frequencydomain methods of analysis are the dual of each other WHY CONVOLUTION AN INTUITIVE EXPLANATION OF SYSTEM RESPONSE On the surface it appears rather strange that the response of linear systems those gentlest of the gentle systems should be given by such a tortuous operation of convolution where one signal is fixed and the other is inverted and shifted To understand this odd behavior consider a hypothetical impulse response ht that decays linearly with time Fig 214a This response is strongest at t 0 the moment the impulse is applied and it decays linearly at future instants so that one second later at t 1 and beyond it ceases to exist This means that the closer the impulse input is to an instant t the stronger is its response at t Now consider the input xt shown in Fig 214b To compute the system response we break the input into rectangular pulses and approximate these pulses with impulses Generally the response of a causal system at some instant t will be determined by all the impulse components of the input before t Each of these impulse components will have different weight in determining the response at the instant t depending on its proximity to t As seen earlier the closer the impulse is to t the stronger is its influence at t The impulse at t has the greatest weight unity in determining The late Prof S J Mason the inventor of signal flow graph techniques used to tell a story of a student frustrated with the impulse function The student said The unit impulse is a thing that is so small you cant see it except at one place the origin where it is so big you cant see it In other words you cant see it at all at least I cant 2 02LathiC02 2017925 1554 page 194 45 194 CHAPTER 2 TIMEDOMAIN ANALYSIS OF CONTINUOUSTIME SYSTEMS For a system specified by Eq 22 the transfer function is given by Hs Ps Qs 241 This follows readily by considering an everlasting input xt est According to Eq 238 the output is yt Hsest Substitution of this xt and yt in Eq 22 yields HsQDest PDest Moreover Drest drest dtr srest Hence PDest Psest and QDest Qsest Consequently Hs Ps Qs DRILL 214 Ideal Integrator and Differentiator Transfer Functions Show that the transfer function of an ideal integrator is Hs 1s and that of an ideal differentiator is Hs s Find the answer in two ways using Eq 239 and using Eq 241 Hint Find ht for the ideal integrator and differentiator You also may need to use the result in Prob 1412 A FUNDAMENTAL PROPERTY OF LTI SYSTEMS We can show that Eq 238 is a fundamental property of LTI systems and it follows directly as a consequence of linearity and time invariance To show this let us assume that the response of an LTI system to an everlasting exponential est is yst If we define Hst yst est then yst Hstest Because of the timeinvariance property the system response to input estT is Hst TestT that is yst T Hst TestT 242 The delayed input estT represents the input est multiplied by a constant esT Hence according to the linearity property the system response to estT must be ystesT Hence yst T ystesT HstestT 02LathiC02 2017925 1554 page 199 50 25 System Stability 199 a b c d e f g h Characteristic root location Characteristic root location Zeroinput response Zeroinput response t t t t t t t t 0 0 0 0 0 0 0 0 0 0 0 0 0 0 0 0 Figure 217 Location of characteristic roots and the corresponding characteristic modes 253 Relationship Between BIBO and Asymptotic Stability External stability is determined by applying an external input with zero initial conditions while internal stability is determined by applying the nonzero initial conditions and no external input This is why these stabilities are also called the zerostate stability and the zeroinput stability respectively Recall that ht the impulse response of an LTIC system is a linear combination of the system characteristic modes For an LTIC system specified by Eq 21 we can readily show that when a characteristic root λk is in the LHP the corresponding mode eλkt is absolutely integrable In 02LathiC02 2017925 1554 page 202 53 202 CHAPTER 2 TIMEDOMAIN ANALYSIS OF CONTINUOUSTIME SYSTEMS The characteristic polynomials of these systems are a λ 1λ2 4λ 8 λ 1λ 2 j2λ 2 j2 b λ 1λ2 4λ 8 λ 1λ 2 j2λ 2 j2 c λ 2λ2 4 λ 2λ j2λ j2 d λ 1λ2 42 λ 2λ j22λ j22 Consequently the characteristic roots of the systems are see Fig 220 a 1 2 j2 b 1 2 j2 c 2 j2 d 1 j2 j2 System a is asymptotically stable all roots in LHP system b is unstable one root in RHP system c is marginally stable unrepeated roots on imaginary axis and no roots in RHP and system d is unstable repeated roots on the imaginary axis BIBO stability is readily determined from the asymptotic stability System a is BIBOstable system b is BIBOunstable system c is BIBOunstable and system d is BIBOunstable We have assumed that these systems are controllable and observable d a 0 c 0 0 b 0 Figure 220 Characteristic root locations for the systems of Ex 214 DRILL 215 Assessing Stability by Characteristic Roots For each case plot the characteristic roots and determine asymptotic and BIBO stabilities Assume the equations reflect internal descriptions a DD 2yt 3xt b D2D 3yt D 5xt c D 1D 2yt 2D 3xt d D2 1D2 9yt D2 2D 4xt e D 1D2 4D 9yt D 7xt 02LathiC02 2017925 1554 page 204 55 204 CHAPTER 2 TIMEDOMAIN ANALYSIS OF CONTINUOUSTIME SYSTEMS ground however the plant that springs from it is totally determined by the seed The imprint of the seed exists on every cell of the plant To understand this interesting phenomenon recall that the characteristic modes of a system are very special to that system because it can sustain these signals without the application of an external input In other words the system offers a free ride and ready access to these signals Now imagine what would happen if we actually drove the system with an input having the form of a characteristic mode We would expect the system to respond strongly this is in fact the resonance phenomenon discussed later in this section If the input is not exactly a characteristic mode but is close to such a mode we would still expect the system response to be strong However if the input is very different from any of the characteristic modes we would expect the system to respond poorly We shall now show that these intuitive deductions are indeed true Intuition can cut the math jungle instantly We have devised a measure of similarity of signals later see in Ch 6 Here we shall take a simpler approach Let us restrict the systems inputs to exponentials of the form eζt where ζ is generally a complex number The similarity of two exponential signals eζt and eλt will then be measured by the closeness of ζ and λ If the difference ζ λ is small the signals are similar if ζ λ is large the signals are dissimilar Now consider a firstorder system with a single characteristic mode eλt and the input eζt The impulse response of this system is then given by Aeλt where the exact value of A is not important for this qualitative discussion The system response yt is given by yt ht xt Aeλtut eζtut From the convolution table Table 21 we obtain yt A ζ λeζt eλtut 246 02LathiC02 2017925 1554 page 208 59 208 CHAPTER 2 TIMEDOMAIN ANALYSIS OF CONTINUOUSTIME SYSTEMS with a time constant Th acts as a lowpass filter having a cutoff frequency of fc 1Th hertz so that sinusoids with frequencies below fc Hz are transmitted reasonably well while those with frequencies above fc Hz are suppressed To demonstrate this fact let us determine the system response to a sinusoidal input xt by convolving this input with the effective impulse response ht in Fig 223a From Figs 223b and 223c we see the process of convolution of ht with the sinusoidal inputs of two different frequencies The sinusoid in Fig 223b has a relatively high frequency while the frequency of the sinusoid in Fig 223c is low Recall that the convolution of xt and ht is equal to the area under the product xτht τ This area is shown shaded in Figs 223b and 223c for the two cases For the highfrequency sinusoid it is clear from Fig 223b that the area under xτht τ is very small because its positive and negative areas nearly cancel each other out In this case the output yt remains periodic but has a rather small amplitude This happens when the period of the sinusoid is much smaller than the system time constant Th In contrast for the lowfrequency sinusoid the period of the sinusoid is larger than Th rendering the partial cancellation of area under xτht τ less effective Consequently the output yt is much larger as depicted in Fig 223c Between these two possible extremes in system behavior a transition point occurs when the period of the sinusoid is equal to the system time constant Th The frequency at which this transition occurs is known as the cutoff frequency fc of the system Because Th is the period of cutoff frequency fc fc 1 Th The frequency fc is also known as the bandwidth of the system because the system transmits or passes sinusoidal components with frequencies below fc while attenuating components with frequencies above fc Of course the transition in system behavior is gradual There is no dramatic change in system behavior at fc 1Th Moreover these results are based on an idealized rectangular pulse impulse response in practice these results will vary somewhat depending on the exact shape of ht Remember that the feel of general system behavior is more important than exact system response for this qualitative discussion Since the system time constant is equal to its rise time we have Tr 1 fc or fc 1 Tr 248 Thus a systems bandwidth is inversely proportional to its rise time Although Eq 248 was derived for an idealized rectangular impulse response its implications are valid for lowpass LTIC systems in general For a general case we can show that 1 fc k Tr where the exact value of k depends on the nature of ht An experienced engineer often can estimate quickly the bandwidth of an unknown system by simply observing the system response to a step input on an oscilloscope 02LathiC02 2017925 1554 page 209 60 26 Intuitive Insights into System Behavior 209 265 Time Constant and Pulse Dispersion Spreading In general the transmission of a pulse through a system causes pulse dispersion or spreading Therefore the output pulse is generally wider than the input pulse This system behavior can have serious consequences in communication systems in which information is transmitted by pulse amplitudes Dispersion or spreading causes interference or overlap with neighboring pulses thereby distorting pulse amplitudes and introducing errors in the received information Earlier we saw that if an input xt is a pulse of width Tx then Ty the width of the output yt is Ty Tx Th This result shows that an input pulse spreads out disperses as it passes through a system Since Th is also the systems time constant or rise time the amount of spread in the pulse is equal to the time constant or rise time of the system 266 Time Constant and Rate of Information Transmission In pulse communications systems which convey information through pulse amplitudes the rate of information transmission is proportional to the rate of pulse transmission We shall demonstrate that to avoid the destruction of information caused by dispersion of pulses during their transmission through the channel transmission medium the rate of information transmission should not exceed the bandwidth of the communications channel Since an input pulse spreads out by Th seconds the consecutive pulses should be spaced Th seconds apart to avoid interference between pulses Thus the rate of pulse transmission should not exceed 1Th pulsessecond But 1Th fc the channels bandwidth so that we can transmit pulses through a communications channel at a rate of fc pulses per second and still avoid significant interference between the pulses The rate of information transmission is therefore proportional to the channels bandwidth or to the reciprocal of its time constant The discussion of Secs 262 263 264 265 and 266 shows that the system time constant determines much of a systems behaviorits filtering characteristics rise time pulse dispersion and so on In turn the time constant is determined by the systems characteristic roots Clearly the characteristic roots and their relative amounts in the impulse response ht determine the behavior of a system EXAMPLE 215 Intuitive Insights into Lowpass System Behavior Find the time constant Th rise time Tr and cutoff frequency fc for a lowpass system that has impulse response ht tetut Determine the maximum rate that pulses of 1 second Theoretically a channel of bandwidth fc can transmit correctly up to 2fc pulse amplitudes per second 4 Our derivation here being very simple and qualitative yields only half the theoretical limit In practice it is not easy to attain the upper theoretical limit 02LathiC02 2017925 1554 page 212 63 212 CHAPTER 2 TIMEDOMAIN ANALYSIS OF CONTINUOUSTIME SYSTEMS is a characteristic mode But even in an asymptotically stable system we see a manifestation of resonance if its characteristic roots are close to the imaginary axis so that Re λ is a small negative value We can show that when the characteristic roots of a system are σ jω0 then the system response to the input ejω0t or the sinusoid cosω0t is very large for small σ The system response drops off rapidly as the input signal frequency moves away from ω0 This frequencyselective behavior can be studied more profitably after an understanding of frequencydomain analysis has been acquired For this reason we postpone full discussion of this subject until Ch 4 IMPORTANCE OF THE RESONANCE PHENOMENON The resonance phenomenon is very important because it allows us to design frequencyselective systems by choosing their characteristic roots properly Lowpass bandpass highpass and bandstop filters are all examples of frequencyselective networks In mechanical systems the inadvertent presence of resonance can cause signals of such tremendous magnitude that the system may fall apart A musical note periodic vibrations of proper frequency can shatter glass if the frequency is matched to the characteristic root of the glass which acts as a mechanical system Similarly a company of soldiers marching in step across a bridge amounts to applying a periodic force to the bridge If the frequency of this input force happens to be nearer to a characteristic root of the bridge the bridge may respond vibrate violently and collapse even though it would have been strong enough to carry many soldiers marching out of step A case in point is the Tacoma Narrows Bridge failure of 1940 This bridge was opened to traffic in July 1940 Within four months of opening on November 7 1940 it collapsed in a mild gale not because of the winds brute force but because the frequencies of windgenerated vortices which matched the natural frequencies characteristic roots of the bridge caused resonance Because of the great damage that may occur mechanical resonance is generally to be avoided especially in structures or vibrating mechanisms If an engine with periodic force such as piston motion is mounted on a platform the platform with its mass and springs should be designed so that their characteristic roots are not close to the engines frequency of vibration Proper design of this platform can not only avoid resonance but also attenuate vibrations if the system roots are placed far away from the frequency of vibration 27 MATLAB MFILES Mfiles are stored sequences of MATLAB commands and help simplify complicated tasks There are two types of Mfile script and function Both types are simple text files and require a m filename extension Although Mfiles can be created by using any text editor MATLABs builtin editor is the preferable choice because of its special features As with any program comments improve the readability of an Mfile Comments begin with the character and continue through the end of the line An Mfile is executed by simply typing the filename without the m extension To execute Mfiles need to be located in the current directory or any other directory in the MATLAB path New directories are easily added to the MATLAB path by using the addpath command This follows directly from Eq 249 with λ σ jω0 and ϵ σ 02LathiC02 2017925 1554 page 214 65 214 CHAPTER 2 TIMEDOMAIN ANALYSIS OF CONTINUOUSTIME SYSTEMS CH2MP1m Chapter 2 MATLAB Program 1 Script Mfile determines characteristic roots of opamp circuit Set component values R 1e4 1e4 1e4 C 1e6 1e6 Determine coefficients for characteristic equation A 1 1R11R21R3C2 1R1R2C1C2 Determine characteristic roots lambda rootsA A script file is created by placing these commands in a text file which in this case is named CH2MP1m While comment lines improve program clarity their removal does not affect program functionality The program is executed by typing CH2MP1 After execution all the resulting variables are available in the workspace For example to view the characteristic roots type lambda lambda 2618034 381966 Thus the characteristic modes are simple decaying exponentials e2618034t and e381966t Script files permit simple or incremental changes thereby saving significant effort Consider what happens when capacitor C1 is changed from 10 µF to 10 nF Changing CH2MP1m so that C 1e9 1e6 allows computation of the new characteristic roots CH2MP1 lambda lambda 10e003 01500 31587i 01500 31587i Perhaps surprisingly the characteristic modes are now complex exponentials capable of supporting oscillations The imaginary portion of λ dictates an oscillation rate of 31587 rads or about 503 Hz The real portion dictates the rate of decay The time expected to reduce the amplitude to 25 is approximately t ln025Reλ 001 second 272 Function MFiles It is inconvenient to modify and save a script file each time a change of parameters is desired Function Mfiles provide a sensible alternative Unlike script Mfiles function Mfiles can accept input arguments as well as return outputs Functions truly extend the MATLAB language in ways that script files cannot 02LathiC02 2017925 1554 page 217 68 27 MATLAB MFiles 217 240 220 200 180 160 140 120 100 Real 5000 0 5000 Imaginary Char Roots Min Val Roots Max Val Roots Figure 226 Effect of component values on characteristic root locations The command lambda zeros2243 preallocates a 2243 array to store the computed roots When necessary MATLAB performs dynamic memory allocation so this command is not strictly necessary However preallocation significantly improves script execution speed Notice also that it would be nearly useless to call script CH2MP1 from within the nested loop script file parameters cannot be changed during execution The plot instruction is quite long Long commands can be broken across several lines by terminating intermediate lines with three dots The three dots tell MATLAB to continue the present command to the next line Black xs locate roots of each permutation The command lambda vectorizes the 2 243 matrix lambda into a 486 1 vector This is necessary in this case to ensure that a proper legend is generated Because of loop order permutation p 1 corresponds to the case of all components at the smallest values and permutation p 243 corresponds to the case of all components at the largest values This information is used to separately highlight the minimum and maximum cases using downtriangles and uptriangles respectively In addition to terminating each for loop end is used to indicate the final index along a particular dimension which eliminates the need to remember the particular size of a variable An overloaded function such as end serves multiple uses and is typically interpreted based on context The graphical results provided by CH2MP3 are shown in Fig 226 Between extremes root oscillations vary from 365 to 745 Hz and decay times to 25 amplitude vary from 62 to 127 ms Clearly this circuits behavior is quite sensitive to ordinary component variations 274 Graphical Understanding of Convolution MATLAB graphics effectively illustrate the convolution process Consider the case of yt xt ht where xt 15sinπtut ut 1 and ht 15ut ut 15 ut 2 ut 25 Program CH2MP4 steps through the convolution over the time interval 025 t 375 02LathiC02 2017925 1554 page 218 69 218 CHAPTER 2 TIMEDOMAIN ANALYSIS OF CONTINUOUSTIME SYSTEMS CH2MP4m Chapter 2 MATLAB Program 4 Script Mfile graphically demonstrates the convolution process figure1 Create figure window and make visible on screen u t 10t0 x t 15sinpitutut1 h t 15utut15ut2ut25 dtau 0005 tau 1dtau4 ti 0 tvec 251375 y NaNzeros1lengthtvec Preallocate memory for t tvec ti ti1 Time index xh xttauhtau lxh lengthxh yti sumxhdtau Trapezoidal approximation of convolution integral subplot211plottauhtauktauxttaukt0ok axistau1 tauend 20 25 patchtau1end1tau1end1tau2endtau2end zeros1lxh1xh1end1xh2endzeros1lxh1 8 8 8edgecolornone xlabel au titleh au solid xt au dashed h auxt au gray c getgcachildren setgcachildrenc2c3c4c1 subplot212plottvecyktvectiytiok xlabelt ylabelyt int h auxt au d au axistau1 tauend 10 20 grid drawnow end At each step the program plots hτ xt τ and shades the area hτxt τ gray This gray area which reflects the integral of hτxt τ is also the desired result yt Figures 227 228 and 229 display the convolution process at times t of 075 225 and 285 seconds respectively These figures help illustrate how the regions of integration change with time Figure 227 has limits of integration from 0 to t 075 Figure 228 has two regions of integration with limits t 1 125 to 15 and 20 to t 225 The last plot Fig 229 has limits from 20 to 25 Several comments regarding CH2MP4 are in order The command figure1 opens the first figure window and more important makes sure it is visible Anonymous functions are used to represent the functions ut xt and ht NaN standing for notanumber usually results from operations such as 00 or MATLAB refuses to plot NaN values so preallocating yt with NaNs ensures that MATLAB displays only values of yt that have been computed As its name suggests length returns the length of the input vector The subplotabc command partitions the current figure window into an abyb matrix of axes and selects axes c for use Subplots facilitate graphical comparison by allowing multiple axes in a single figure window The patch command is used to create the grayshaded area for hτxt τ In CH2MP4 the get and set commands are used to reorder plot objects so that the gray area does not obscure other lines Details of the patch get and set commands as used in CH2MP4 are somewhat advanced and are not pursued here MATLAB also prints most Greek letters if the Greek name is preceded by a backslash character For example au in the xlabel command produces the symbol τ in the plots axis label Similarly an integral sign is produced by int Finally the drawnow Interested students should consult the MATLAB help facilities for further information Actually the get and set commands are extremely powerful and can help modify plots in almost any conceivable way 02LathiC02 2017925 1554 page 219 70 28 MATLAB MFiles 219 1 05 0 05 1 15 2 25 3 35 4 2 0 2 hτ solid xtτ dashed hτtτ gray 1 05 0 05 1 15 2 25 3 35 4 t τ 1 0 1 2 yt hτtτ dτ Figure 227 Graphical convolution at step t 075 second 2 0 2 1 05 0 05 1 15 2 25 3 35 4 t 1 05 0 05 1 15 2 25 3 35 4 τ 1 0 1 2 hτ solid xtτ dashed hτtτ gray yt hτtτ dτ Figure 228 Graphical convolution at step t 225 seconds command forces MATLAB to update the graphics window for each loop iteration Although slow this creates an animationlike effect Replacing drawnow with the pause command allows users to manually step through the convolution process The pause command still forces the graphics window to update but the program will not continue until a key is pressed 02LathiC02 2017925 1554 page 220 71 220 CHAPTER 2 TIMEDOMAIN ANALYSIS OF CONTINUOUSTIME SYSTEMS 1 05 0 05 1 15 2 25 3 35 4 2 0 2 1 05 0 05 1 15 2 25 3 35 4 t τ 1 0 1 2 hτ solid xtτ dashed hτtτ gray yt hτtτ dτ Figure 229 Graphical convolution at step t 285 seconds 28 APPENDIX DETERMINING THE IMPULSE RESPONSE In Eq 213 we showed that for an LTIC system S specified by Eq 211 the unit impulse response ht can be expressed as ht b0δt characteristic modes 251 To determine the characteristic mode terms in Eq 251 let us consider a system S0 whose input xt and the corresponding output wt are related by QDwt xt 252 Observe that both the systems S and S0 have the same characteristic polynomial namely Qλ and consequently the same characteristic modes Moreover S0 is the same as S with PD 1 that is b0 0 Therefore according to Eq 251 the impulse response of S0 consists of characteristic mode terms only without an impulse at t 0 Let us denote this impulse response of S0 by ynt Observe that ynt consists of characteristic modes of S and therefore may be viewed as a zeroinput response of S Now ynt is the response of S0 to input δt Therefore according to Eq 252 QDynt δt or DN a1DN1 aN1D aNynt δt or yN n t a1yN1 n t aN1y1 n t aNynt δt 02LathiC02 2017925 1554 page 221 72 29 Summary 221 where yk n t represents the kth derivative of ynt The righthand side contains a single impulse term δt This is possible only if yN1 n t has a unit jump discontinuity at t 0 so that yN n t δt Moreover the lowerorder terms cannot have any jump discontinuity because this would mean the presence of the derivatives of δt Therefore yn0 y1 n 0 yN2 n 0 0 no discontinuity at t 0 and the N initial conditions on ynt are yn0 y1 n 0 yN2 n 0 0 and yN1 n 0 1 253 This discussion means that ynt is the zeroinput response of the system S subject to initial conditions Eq 253 We now show that for the same input xt to both systems S and S0 their respective outputs yt and wt are related by yt PDwt 254 To prove this result we operate on both sides of Eq 252 by PD to obtain QDPDwt PDxt Comparison of this equation with Eq 22 leads immediately to Eq 254 Now if the input xt δt the output of S0 is ynt and the output of S according to Eq 254 is PDynt This output is ht the unit impulse response of S Note however that because it is an impulse response of a causal system S0 the function ynt is causal To incorporate this fact we must represent this function as yntut Now it follows that ht the unit impulse response of the system S is given by ht PDyntut 255 where ynt is a linear combination of the characteristic modes of the system subject to initial conditions 253 The righthand side of Eq 255 is a linear combination of the derivatives of yntut Evaluating these derivatives is clumsy and inconvenient because of the presence of ut The derivatives will generate an impulse and its derivatives at the origin Fortunately when M N Eq 211 we can avoid this difficulty by using the observation in Eq 251 which asserts that at t 0 the origin ht b0δt Therefore we need not bother to find ht at the origin This simplification means that instead of deriving PDyntut we can derive PDynt and add to it the term b0δt so that ht b0δt PDynt t 0 b0δt PDyntut This expression is valid when M N the form given in Eq 211 When M N Eq 255 should be used 29 SUMMARY This chapter discusses timedomain analysis of LTIC systems The total response of a linear system is a sum of the zeroinput response and zerostate response The zeroinput response is the system 02LathiC02 2017925 1554 page 222 73 222 CHAPTER 2 TIMEDOMAIN ANALYSIS OF CONTINUOUSTIME SYSTEMS response generated only by the internal conditions initial conditions of the system assuming that the external input is zero hence the adjective zeroinput The zerostate response is the system response generated by the external input assuming that all initial conditions are zero that is when the system is in zero state Every system can sustain certain forms of response on its own with no external input zero input These forms are intrinsic characteristics of the system that is they do not depend on any external input For this reason they are called characteristic modes of the system Needless to say the zeroinput response is made up of characteristic modes chosen in a combination required to satisfy the initial conditions of the system For an Nthorder system there are N distinct modes The unit impulse function is an idealized mathematical model of a signal that cannot be generated in practice Nevertheless introduction of such a signal as an intermediary is very helpful in analysis of signals and systems The unit impulse response of a system is a combination of the characteristic modes of the system because the impulse δt 0 for t 0 Therefore the system response for t 0 must necessarily be a zeroinput response which as seen earlier is a combination of characteristic modes The zerostate response response due to external input of a linear system can be obtained by breaking the input into simpler components and then adding the responses to all the components In this chapter we represent an arbitrary input xt as a sum of narrow rectangular pulses staircase approximation of xt In the limit as the pulse width 0 the rectangular pulse components approach impulses Knowing the impulse response of the system we can find the system response to all the impulse components and add them to yield the system response to the input xt The sum of the responses to the impulse components is in the form of an integral known as the convolution integral The system response is obtained as the convolution of the input xt with the systems impulse response ht Therefore the knowledge of the systems impulse response allows us to determine the system response to any arbitrary input LTIC systems have a very special relationship to the everlasting exponential signal est because the response of an LTIC system to such an input signal is the same signal within a multiplicative constant The response of an LTIC system to the everlasting exponential input est is Hsest where Hs is the transfer function of the system If every bounded input results in a bounded output the system is stable in the boundedinputboundedoutput BIBO sense An LTIC system is BIBOstable if and only if its impulse response is absolutely integrable Otherwise it is BIBOunstable BIBO stability is a stability seen from external terminals of the system Hence it is also called external stability or zerostate stability In contrast internal stability or the zeroinput stability examines the system stability from inside When some initial conditions are applied to a system in zero state then if the system eventually returns to zero state the system is said to be stable in the asymptotic or Lyapunov sense If the systems response increases without bound it is unstable If the system does not go to zero state and the response does not increase indefinitely the system is marginally stable The internal stability criterion in terms of the location of a systems characteristic roots can be summarized as follows However it can be closely approximated by a narrow pulse of unit area and having a width that is much smaller than the time constant of an LTIC system in which it is used There is the possibility of an impulse in addition to the characteristic modes 02LathiC02 2017925 1554 page 223 74 Problems 223 1 An LTIC system is asymptotically stable if and only if all the characteristic roots are in the LHP The roots may be repeated or unrepeated 2 An LTIC system is unstable if and only if either one or both of the following conditions exist i at least one root is in the RHP ii there are repeated roots on the imaginary axis 3 An LTIC system is marginally stable if and only if there are no roots in the RHP and there are some unrepeated roots on the imaginary axis It is possible for a system to be externally BIBO stable but internally unstable When a system is controllable and observable its external and internal descriptions are equivalent Hence external BIBO and internal asymptotic stabilities are equivalent and provide the same information Such a BIBOstable system is also asymptotically stable and vice versa Similarly a BIBOunstable system is either marginally stable or asymptotically unstable system The characteristic behavior of a system is extremely important because it determines not only the system response to internal conditions zeroinput behavior but also the system response to external inputs zerostate behavior and the system stability The system response to external inputs is determined by the impulse response which itself is made up of characteristic modes The width of the impulse response is called the time constant of the system which indicates how fast the system can respond to an input The time constant plays an important role in determining such diverse system behaviors as the response time and filtering properties of the system dispersion of pulses and the rate of pulse transmission through the system REFERENCES 1 Lathi B P Signals and Systems BerkeleyCambridge Press Carmichael CA 1987 2 Mason S J Electronic Circuits Signals and Systems Wiley New York 1960 3 Kailath T Linear System PrenticeHall Englewood Cliffs NJ 1980 4 Lathi B P Modern Digital and Analog Communication Systems 3rd ed Oxford University Press New York 1998 PROBLEMS 221 Determine the constants c1 c2 λ1 and λ2 for each of the following secondorder systems which have zeroinput responses of the form yzirt c1eλ1t c2eλ2t a yt 2yt 5yt xt 5xt with yzir0 2 and yzir0 0 b yt 2yt 5yt xt 5xt with yzir0 4 and yzir0 1 c d2 dt2 yt 2 d dtyt xt with yzir0 1 and yzir0 2 d D2 2D10yt D5 Dxt with yzir0 yzir0 1 e D2 7 2D 3 2yt D 2xt with yzir0 3 and yzir0 8 Caution The second IC is given in terms of the second derivative not the first derivative f 13yt 4 d dtyt d2 dt2 yt 2xt 4 d dtxt with yzir0 3 and yzir0 15 Caution The second IC is given in terms of the second derivative not the first derivative 222 Consider a linear timeinvariant system with input xt and output yt that is described by the differential equation D 1D2 1yt D5 1xt Furthermore assume y0 y0 y0 1 02LathiC02 2017925 1554 page 227 78 Problems 227 2410 If xt gt ct then show that xat gat 1acat This timescaling property of convolution states that if both xt and gt are timescaled by a their convolution is also timescaled by a and multiplied by 1a ht t 0 2 1 1 2 3 4 5 1 Figure P248 2411 Show that the convolution of an odd and an even function is an odd function and the convolution of two odd or two even functions is an even function Hint Use the timescaling property of convolution in Prob 2410 2412 Suppose an LTIC system has impulse response ht 1 tut ut 1 and input xt ut 1ut 1 Use the graphical convolu tion procedure to determine yzsrt xt ht Accurately sketch yzsrt When solving for yzsrt flip and shift ht explicitly show all integration steps and simplify your answer 2413 Using direct integration find eatut ebtut 2414 Using direct integration find ut ut eatut eatut and tut ut 2415 Using direct integration find sin tut ut and cos tut ut 2416 The unit impulse response of an LTIC system is ht etut Find this systems zerostate response yt if the input xt is a ut b etut c e2tut d sin3tut Use the convolution table Table 21 to find your answers 2417 Repeat Prob 2416 for ht 2e3t e2tut and if the input xt is a ut b etut c e2tut 2418 Repeat Prob 2416 for ht 1 2te2tut and input xt ut 2419 Repeat Prob 2416 for ht 4e2t cos3tut and each of the following inputs xt a ut b etut 2420 Repeat Prob 2416 for ht etut and each of the following inputs xt a e2tut b e2t3ut c e2tut 3 d The gate pulse depicted in Fig P2420and provide a sketch of yt xt 0 t 1 1 Figure P2420 2421 A firstorder allpass filter impulse response is given by ht δt 2etut a Find the zerostate response of this filter for the input etut b Sketch the input and the corresponding zerostate response 2422 Figure P2422 shows the input xt and the impulse response ht for an LTIC system Let the output be yt a By inspection of xt and ht find y1y0y1y2y3y4y5 and 02LathiC02 2017925 1554 page 230 81 230 CHAPTER 2 TIMEDOMAIN ANALYSIS OF CONTINUOUSTIME SYSTEMS ht t 1 1 1 t 1 1 1 2 xt Figure P2430 t 1 1 1 2 ht xt yt ht ht Figure P2431 xt yt C L Figure P2432 a b h1 h2 xt ypt xt h1 h2 yst Figure P2433 2433 Two LTIC systems have impulse response functions given by h1t 1tutut1 and h2t tut 2 ut 2 a Carefully sketch the functions h1t and h2t b Assume that the two systems are connected in parallel as shown in Fig P2433a Carefully plot the equivalent impulse response function hpt c Assume that the two systems are connected in cascade as shown in Fig P2433b Carefully plot the equivalent impulse response function hst 2434 Consider the circuit shown in Fig P2434 a Find the output yt given an initial capacitor voltage of y0 2 volts and an input xt ut b Given an input xt ut 1 determine the initial capacitor voltage y0 so that the output yt is 05 volt at t 2 seconds xt yt C R Figure P2434 03LathiC03 2017925 1554 page 237 1 C H A P T E R TIMEDOMAIN ANALYSIS OF DISCRETETIME SYSTEMS 3 In this chapter we introduce the basic concepts of discretetime signals and systems Furthermore we explore the timedomain analysis of linear timeinvariant discretetime LTID systems We show how to compute the zeroinput response determine the unit impulse response and use convolution to evaluate the zerostate response 31 INTRODUCTION A discretetime signal is basically a sequence of numbers Such signals arise naturally in inherently discretetime situations such as population studies amortization problems national income models and radar tracking They may also arise as a result of sampling continuoustime signals in sampled data systems and digital filtering Such signals can be denoted by xn yn and so on where the variable n takes integer values and xn denotes the nth number in the sequence labeled x In this notation the discretetime variable n is enclosed in square brackets instead of parentheses which we have reserved for enclosing continuoustime variables such as t Systems whose inputs and outputs are discretetime signals are called discretetime systems A digital computer is a familiar example of this type of system A discretetime signal is a sequence of numbers and a discretetime system processes a sequence of numbers xn to yield another sequence yn as the output A discretetime signal when obtained by uniform sampling of a continuoustime signal xt can also be expressed as xnT where T is the sampling interval and n the discrete variable taking on integer values Thus xnT denotes the value of the signal xt at t nT The signal xnT is a sequence of numbers sample values and hence by definition is a discretetime signal Such a signal can also be denoted by the customary discretetime notation xn where xn xnT A typical discretetime signal is depicted in Fig 31 which shows both forms of notation By way of an example a continuoustime exponential xt et when sampled every T 01 seconds results in a discretetime signal xnT given by xnT enT e01n There may be more than one input and more than one output 237 03LathiC03 2017925 1554 page 242 6 242 CHAPTER 3 TIMEDOMAIN ANALYSIS OF DISCRETETIME SYSTEMS DRILL 33 RightShift Operation Show that xk n can be obtained from xn by first rightshifting xn by k units and then timereversing this shifted signal TIME REVERSAL To timereverse xn in Fig 34a we rotate xn about the vertical axis to obtain the timereversed signal xrn shown in Fig 34c Using the argument employed for a similar operation in continuoustime signals Sec 12 we obtain xrn xn Therefore to timereverse a signal we replace n with n so that xn is the timereversed xn For example if xn 09n for 3 n 10 then xrn 09n for 3 n 10 that is 3 n 10 as shown in Fig 34c The origin n 0 is the anchor point which remains unchanged under timereversal operation because at n 0 xn xn x0 Note that while the reversal of xn about the vertical axis is xn the reversal of xn about the horizontal axis is xn EXAMPLE 32 Time Reversal and Shifting In the convolution operation discussed later we need to find the function xk n from xn This can be done in two steps i timereverse the signal xn to obtain xn ii now rightshift xn by k Recall that rightshifting is accomplished by replacing n with n k Hence rightshifting xn by k units is xn k xk n Figure 34d shows x5 n obtained this way We first timereverse xn to obtain xn in Fig 34c Next we shift xn by k 5 to obtain xk n x5 n as shown in Fig 34d In this particular example the order of the two operations employed is interchangeable We can first leftshift xk to obtain xn 5 Next we timereverse xn 5 to obtain xn 5 x5 n The reader is encouraged to verify that this procedure yields the same result as in Fig 34d DRILL 34 Time Reversal Sketch the signal xn e05n for 3 n 2 and zero otherwise Sketch the corresponding timereversed signal and show that it can be expressed as xrn e05n for 2 n 3 03LathiC03 2017925 1554 page 250 14 250 CHAPTER 3 TIMEDOMAIN ANALYSIS OF DISCRETETIME SYSTEMS Accurately handsketching DT signals can be tedious and difficult As the next example shows MATLAB is particularly well suited to plot DT signals including exponentials EXAMPLE 34 Plotting DT Exponentials with MATLAB Use MATLAB to plot the following discretetime signals over 0 n 8 a xan 08n b xbn 08n c xcn 05n and d xdn 11n To begin we use anonymous functions to represent each of the four signals Next we plot these functions over the desired range of n The results shown in Fig 310 match the earlier Fig 39 plots of the same signals n 08 xa n 08n xb n 08n xc n 05n xd n 11n subplot221 stemnxank ylabelxan xlabeln subplot222 stemnxbnk ylabelxbn xlabeln subplot223 stemnxcnk ylabelxcn xlabeln subplot224 stemnxdnk ylabelxdn xlabeln 0 05 1 xan 1 0 1 xbn 0 2 4 6 8 0 2 4 6 8 n 0 05 1 xcn n 0 2 4 6 8 0 2 4 6 8 n n 0 1 2 3 xdn Figure 310 DT plots for Ex 34 03LathiC03 2017925 1554 page 253 17 34 Examples of DiscreteTime Systems 253 30 20 10 0 10 20 30 n 1 0 1 xn Figure 312 Sinusoid plot for Ex 35 34 EXAMPLES OF DISCRETETIME SYSTEMS We shall give here four examples of discretetime systems In the first two examples the signals are inherently of the discretetime variety In the third and fourth examples a continuoustime signal is processed by a discretetime system as illustrated in Fig 32 by discretizing the signal through sampling EXAMPLE 36 Savings Account A person makes a deposit the input in a bank regularly at an interval of T say 1 month The bank pays a certain interest on the account balance during the period T and mails out a periodic statement of the account balance the output to the depositor Find the equation relating the output yn the balance to the input xn the deposit In this case the signals are inherently discrete time Let xn deposit made at the nth discrete instant yn account balance at the nth instant computed immediately after receipt of the nth deposit xn r interest per dollar per period T The balance yn is the sum of i the previous balance yn 1 ii the interest on yn 1 during the period T and iii the deposit xn yn yn 1 ryn 1 xn 1 ryn 1 xn or yn ayn 1 xn a 1 r 33 In this example the deposit xn is the input cause and the balance yn is the output effect 03LathiC03 2017925 1554 page 260 24 260 CHAPTER 3 TIMEDOMAIN ANALYSIS OF DISCRETETIME SYSTEMS KINSHIP OF DIFFERENCE EQUATIONS TO DIFFERENTIAL EQUATIONS We now show that a digitized version of a differential equation results in a difference equation Let us consider a simple firstorder differential equation dyt dt cyt xt 312 Consider uniform samples of xt at intervals of T seconds As usual we use the notation xn to denote xnT the nth sample of xt Similarly yn denotes ynT the nth sample of yt From the basic definition of a derivative we can express Eq 312 at t nT as lim T0 yn yn 1 T cyn xn Clearing the fractions and rearranging the terms yield assuming nonzero but very small T yn αyn 1 βxn 313 where α 1 1 cT and β T 1 cT We can also express Eq 313 in advance form as yn 1 αyn βxn 1 It is clear that a differential equation can be approximated by a difference equation of the same order In this way we can approximate an nthorder differential equation by a difference equation of nth order Indeed a digital computer solves differential equations by using an equivalent difference equation which can be solved by means of simple operations of addition multiplication and shifting Recall that a computer can perform only these simple operations It must necessarily approximate complex operation like differentiation and integration in terms of such simple operations The approximation can be made as close to the exact answer as possible by choosing sufficiently small value for T At this stage we have not developed tools required to choose a suitable value of the sampling interval T This subject is discussed in Ch 5 and also in Ch 8 In Sec 57 we shall discuss a systematic procedure impulse invariance method for finding a discretetime system with which to realize an Nthorder LTIC system ORDER OF A DIFFERENCE EQUATION Equations 33 35 39 311 and 313 are examples of difference equations The highestorder difference of the output signal or the input signal whichever is higher represents the order of the difference equation Hence Eqs 33 39 311 and 313 are firstorder difference equations whereas Eq 35 is of the second order 03LathiC03 2017925 1554 page 261 25 34 Examples of DiscreteTime Systems 261 DRILL 38 Digital Integrator Design Design a digital integrator in Ex 39 using the fact that for an integrator the output yt and the input xt are related by dytdt xt Approximation similar to that in Ex 38 of this equation at t nT yields the recursive form in Eq 311 ANALOG DIGITAL CONTINUOUSTIME AND DISCRETETIME SYSTEMS The basic difference between continuoustime systems and analog systems as also between discretetime and digital systems is fully explained in Secs 175 and 176 Historically discretetime systems have been realized with digital computers where continuoustime signals are processed through digitized samples rather than unquantized samples Therefore the terms digital filters and discretetime systems are used synonymously in the literature This distinction is irrelevant in the analysis of discretetime systems For this reason we follow this loose convention in this book where the term digital filter implies a discretetime system and analog filter means continuoustime system Moreover the terms CD continuoustodiscretetime and DC will occasionally be used interchangeably with terms AD analogtodigital and DA respectively ADVANTAGES OF DIGITAL SIGNAL PROCESSING 1 Digital systems operation can tolerate considerable variation in signal values and hence are less sensitive to changes in the component parameter values due to temperature variation aging and other factors This results in greater degree of precision and stability Since digital systems are binary circuits their accuracy can be increased by using more complex circuitry to increase word length subject to cost limitations 2 Digital systems do not require any factory adjustment and can be easily duplicated in volume without having to worry about precise component values They can be fully integrated and even highly complex systems can be placed on a single chip by using VLSI verylargescale integrated circuits 3 Digital filters are more flexible Their characteristics can be easily altered simply by changing the program Digital hardware implementation permits the use of microprocessors miniprocessors digital switching and largescale integrated circuits 4 A greater variety of filters can be realized by digital systems 5 Digital signals can be stored easily and inexpensively on various media eg magnetic optical and solid state without deterioration of signal quality It is also possible and increasingly popular to search and select information from distant electronic storehouses such as the cloud 6 Digital signals can be coded to yield extremely low error rates and high fidelity as well as privacy Also more sophisticated signalprocessing algorithms can be used to process digital signals The terms discretetime and continuoustime qualify the nature of a signal along the time axis horizontal axis The terms analog and digital in contrast qualify the nature of the signal amplitude vertical axis 03LathiC03 2017925 1554 page 262 26 262 CHAPTER 3 TIMEDOMAIN ANALYSIS OF DISCRETETIME SYSTEMS 7 Digital filters can be easily timeshared and therefore can serve a number of inputs simultaneously Moreover it is easier and more efficient to multiplex several digital signals on the same channel 8 Reproduction with digital messages is extremely reliable without deterioration Analog messages such as photocopies and films for example lose quality at each successive stage of reproduction and have to be transported physically from one distant place to another often at relatively high cost One must weigh these advantages against such disadvantages as increased system complexity due to use of AD and DA interfaces limited range of frequencies available in practice affordable rates are gigahertz or less and use of more power than is needed for the passive analog circuits Digital systems use powerconsuming active devices 341 Classification of DiscreteTime Systems Before examining the nature of discretetime system equations let us consider the concepts of linearity time invariance or shift invariance and causality which apply to discretetime systems also LINEARITY AND TIME INVARIANCE For discretetime systems the definition of linearity is identical to that for continuoustime systems as given in Eq 122 We can show that the systems in Exs 36 37 38 and 39 are all linear Time invariance or shift invariance for discretetime systems is also defined in a way similar to that for continuoustime systems Systems whose parameters do not change with time with n are timeinvariant or shiftinvariant also constantparameter systems For such a system if the input is delayed by k units or samples the output is the same as before but delayed by k samples assuming the initial conditions also are delayed by k The systems in Exs 36 37 38 and 39 are timeinvariant because the coefficients in the system equations are constants independent of n If these coefficients were functions of n time then the systems would be linear timevarying systems Consider for example a system described by yn enxn For this system let a signal x1n yield the output y1n and another input x2n yield the output y2n Then y1n enx1n and y2n enx2n If we let x2n x1n N0 then y2n enx2n enx1n N0 y1n N0 Clearly this is a timevarying parameter system 03LathiC03 2017925 1554 page 263 27 34 Examples of DiscreteTime Systems 263 CAUSAL AND NONCAUSAL SYSTEMS A causal also known as a physical or nonanticipative system is one for which the output at any instant n k depends only on the value of the input xn for n k In other words the value of the output at the present instant depends only on the past and present values of the input xn not on its future values As we shall see the systems in Exs 36 37 38 and 39 are all causal INVERTIBLE AND NONINVERTIBLE SYSTEMS A discretetime system S is invertible if an inverse system Si exists such that the cascade of S and Si results in an identity system An identity system is defined as one whose output is identical to the input In other words for an invertible system the input can be uniquely determined from the corresponding output For every input there is a unique output When a signal is processed through such a system its input can be reconstructed from the corresponding output There is no loss of information when a signal is processed through an invertible system A cascade of a unit delay with a unit advance results in an identity system because the output of such a cascaded system is identical to the input Clearly the inverse of an ideal unit delay is ideal unit advance which is a noncausal and unrealizable system In contrast a compressor yn xMn is not invertible because this operation loses all but every Mth sample of the input and generally the input cannot be reconstructed Similarly operations such as yn cosxn or yn xn are not invertible DRILL 39 Invertibility Show that a system specified by equation yn axn b is invertible but that the system yn xn2 is noninvertible STABLE AND UNSTABLE SYSTEMS The concept of stability is similar to that in continuoustime systems Stability can be internal or external If every bounded input applied at the input terminal results in a bounded output the system is said to be stable externally External stability can be ascertained by measurements at the external terminals of the system This type of stability is also known as the stability in the BIBO boundedinputboundedoutput sense Both internal and external stability are discussed in greater detail in Sec 39 MEMORYLESS SYSTEMS AND SYSTEMS WITH MEMORY The concepts of memoryless or instantaneous systems and those with memory or dynamic are identical to the corresponding concepts of the continuoustime case A system is memoryless if its response at any instant n depends at most on the input at the same instant n The output at any instant of a system with memory generally depends on the past present and future values of the input For example yn sinxn is an example of instantaneous system and ynyn1 xn is an example of a dynamic system or a system with memory 03LathiC03 2017925 1554 page 265 29 35 DiscreteTime System Equations 265 Since any bounded input is guaranteed to produce a bounded output it follows that the system is BIBOstable f To be memoryless a systems output can only depend on the strength of the current input Since the output y at time n depends on the input x not only at present time n but also on past time n 1 we see that the system is not memoryless 35 DISCRETETIME SYSTEM EQUATIONS In this section we discuss timedomain analysis of LTID linear timeinvariant discretetime systems With minor differences the procedure is parallel to that for continuoustime systems DIFFERENCE EQUATIONS Equations 33 35 38 and 313 are examples of difference equations Equations 33 38 and 313 are firstorder difference equations and Eq 35 is a secondorder difference equation All these equations are linear with constant not timevarying coefficients Before giving a general form of an Nthorder linear difference equation we recall that a difference equation can be written in two forms the first form uses delay terms such as yn 1 yn 2 xn 1 xn 2 and so on and the alternate form uses advance terms such as yn 1 yn 2 and so on Although the delay form is more natural we shall often prefer the advance form not just for the general notational convenience but also for resulting notational uniformity with the operator form for differential equations This facilitates the commonality of the solutions and concepts for continuoustime and discretetime systems We start here with a general difference equation written in advance form as yn N a1yn N 1 aN1yn 1 aNyn bNMxn M bNM1xn M 1 bN1xn 1 bNxn 314 This is a linear difference equation whose order is maxNM We have assumed the coefficient of yn N to be unity a0 1 without loss of generality If a0 1 we can divide the equation throughout by a0 to normalize the equation to have a0 1 CAUSALITY CONDITION For a causal system the output cannot depend on future input values This means that when the system equation is in the advance form of Eq 314 causality requires M N If M were to be greater than N then ynN the output at nN would depend on xnM which is the input at the later instant n M For a general causal case M N and Eq 314 can be expressed as yn N a1yn N 1 aN1yn 1 aNyn b0xn N b1 xn N 1 bN1xn 1 bNxn 315 Equations such as 33 35 38 and 313 are considered to be linear according to the classical definition of linearity Some authors label such equations as incrementally linear We prefer the classical definition It is just a matter of individual choice and makes no difference in the final results 03LathiC03 2017925 1554 page 266 30 266 CHAPTER 3 TIMEDOMAIN ANALYSIS OF DISCRETETIME SYSTEMS where some of the coefficients on either side can be zero In this Nthorder equation a0 the coefficient of ynN is normalized to unity Equation 315 is valid for all values of n Therefore it is still valid if we replace n by n N throughout the equation see Eqs 33 and 34 Such replacement yields a delayform alternative yn a1yn 1 aN1yn N 1 aNyn N b0xn b1xn 1 bN1xn N 1 bNxn N 316 351 Recursive Iterative Solution of Difference Equation Equation 316 can be expressed as yn a1yn 1 a2yn 2 aNyn N b0xn b1xn 1 bNxn N 317 In Eq 317 yn is computed from 2N 1 pieces of information the preceding N values of the output yn 1 yn 2 yn N and the preceding N values of the input xn 1 xn 2 xn N and the present value of the input xn Initially to compute y0 the N initial conditions y1 y2 yN serve as the preceding N output values Hence knowing the N initial conditions and the input we can determine recursively the entire output y0 y1 y2 y3 one value at a time For instance to find y0 we set n 0 in Eq 317 The lefthand side is y0 and the righthand side is expressed in terms of N initial conditions y1 y2 yN and the input x0 if xn is causal because of causality other input terms xn 0 Similarly knowing y0 and the input we can compute y1 by setting n 1 in Eq 317 Knowing y0 and y1 we find y2 and so on Thus we can use this recursive procedure to find the complete response y0 y1 y2 For this reason this equation is classed as a recursive form This method basically reflects the manner in which a computer would solve a recursive difference equation given the input and initial conditions Equation 317 or Eq 316 is nonrecursive if all the N 1 coefficients ai 0 i 12 N 1 In this case it can be seen that yn is computed only from the input values and without using any previous outputs Generally speaking the recursive procedure applies only to equations in the recursive form The recursive iterative procedure is demonstrated by the following examples EXAMPLE 311 Iterative Solution to a FirstOrder Difference Equation Solve iteratively yn 05yn 1 xn with initial condition y1 16 and causal input xn n2un This equation can be expressed as yn 05yn 1 xn 318 03LathiC03 2017925 1554 page 267 31 35 DiscreteTime System Equations 267 If we set n 0 in Eq 318 we obtain y0 05y1 x0 0516 0 8 Now setting n 1 in Eq 318 and using the value y0 8 computed in the first step and x1 12 1 we obtain y1 058 12 5 Next setting n 2 in Eq 318 and using the value y1 5 computed in the previous step and x2 22 we obtain y2 055 22 65 Continuing in this way iteratively we obtain y3 0565 32 1225 y4 051225 42 22125 The output yn is depicted in Fig 317 yn 8 5 65 1225 0 1 2 3 4 5 n Figure 317 Iterative solution of a difference equation We now present one more example of iterative solutionthis time for a secondorder equation The iterative method can be applied to a difference equation in delay form or advance form In Ex 311 we considered the former Let us now apply the iterative method to the advance form EXAMPLE 312 Iterative Solution to a SecondOrder Difference Equation Solve iteratively yn 2 yn 1 024yn xn 2 2xn 1 03LathiC03 2017925 1554 page 268 32 268 CHAPTER 3 TIMEDOMAIN ANALYSIS OF DISCRETETIME SYSTEMS with initial conditions y1 2 y2 1 and a causal input xn nun The system equation can be expressed as yn 2 yn 1 024yn xn 2 2xn 1 319 Setting n 2 in Eq 319 and then substituting y1 2 y2 1 x0 x1 0 we obtain y0 2 0241 0 0 176 Setting n 1 in Eq 319 and then substituting y0 176 y1 2 x1 1 x0 0 we obtain y1 176 0242 1 0 228 Setting n 0 in Eq 319 and then substituting y0 176 y1 228 x2 2 and x1 1 yield y2 228 024176 2 21 18576 and so on With MATLAB we can readily verify and extend these recursive calculations n 25 y 12zeros1lengthn2 x 00n3end for k 1lengthn2 yk2 yk1024ykxk22xk1 end ny n 2 1 0 1 2 3 4 5 y 10000 20000 17600 22800 18576 03104 21354 52099 Note carefully the recursive nature of the computations From the N initial conditions and the input we obtained y0 first Then using this value of y0 and the preceding N 1 initial conditions along with the input we find y1 Next using y0 y1 along with the past N 2 initial conditions and input we obtained y2 and so on This method is general and can be applied to a recursive difference equation of any order It is interesting that the hardware realization of Eq 318 depicted in Fig 314 with a 05 generates the solution precisely in this iterative fashion DRILL 310 Iterative Solution to a Difference Equation Using the iterative method find the first three terms of yn for yn 1 2yn xn The initial condition is y1 10 and the input xn 2 starting at n 0 ANSWER y0 20 y1 42 and y2 86 03LathiC03 2017925 1554 page 270 34 270 CHAPTER 3 TIMEDOMAIN ANALYSIS OF DISCRETETIME SYSTEMS RESPONSE OF LINEAR DISCRETETIME SYSTEMS Following the procedure used for continuoustime systems we can show that Eq 320 is a linear equation with constant coefficients A system described by such an equation is a linear timeinvariant discretetime LTID system We can verify as in the case of LTIC systems see the footnote on page 151 that the general solution of Eq 320 consists of zeroinput and zerostate components 36 SYSTEM RESPONSE TO INTERNAL CONDITIONS THE ZEROINPUT RESPONSE The zeroinput response y0n is the solution of Eq 320 with xn 0 that is QEy0n 0 or EN a1EN1 aN1E aNy0n 0 321 Although we can solve this equation systematically even a cursory examination points to the solution This equation states that a linear combination of y0n and advanced y0n is zero not for some values of n but for all n Such a situation is possible if and only if y0n and advanced y0n have the same form Only an exponential function γ n has this property as the following equation indicates Ekγ n γ nk γ kγ n This expression shows that γ n advanced by k units is a constant γ k times γ n Therefore the solution of Eq 321 must be of the form y0n cγ n 322 To determine c and γ we substitute this solution in Eq 321 Since Eky0n y0nk cγ nk this produces cγ N a1γ N1 aN1γ aNγ n 0 For a nontrivial solution of this equation γ N a1γ n1 aN1γ aN 0 323 or Qγ 0 Our solution cγ n Eq 322 is correct provided γ satisfies Eq 323 Now Qγ is an Nthorder polynomial and can be expressed in the factored form assuming all distinct roots γ γ1γ γ2 γ γN 0 Clearly γ has N solutions γ1 γ2 γN and therefore Eq 321 also has N solutions c1γ n 1 c2γ n 2 cnγ n N In such a case we have shown that the general solution is a linear combination A signal of the form nmγ n also satisfies this requirement under certain conditions repeated roots discussed later 03LathiC03 2017925 1554 page 272 36 272 CHAPTER 3 TIMEDOMAIN ANALYSIS OF DISCRETETIME SYSTEMS Therefore y0n 1 502n 4 508n n 0 The reader can verify this solution by computing the first few terms using the iterative method see Exs 311 and 312 DRILL 311 ZeroInput Response of FirstOrder Systems Find and sketch the zeroinput response for the systems described by the following equations a yn 1 08yn 3xn 1 b yn 1 08yn 3xn 1 In each case the initial condition is y1 10 Verify the solutions by computing the first three terms using the iterative method ANSWERS a 808n b 808n DRILL 312 ZeroInput Response of a SecondOrder System with Real Roots Find the zeroinput response of a system described by the equation yn 03yn 1 01yn 2 xn 2xn 1 The initial conditions are y01 1 and y02 33 Verify the solution by computing the first three terms iteratively ANSWER y0n 02n 205n Section 351 introduced the method of recursion to solve difference equations As the next example illustrates the zeroinput response can likewise be found through recursion Since it does 03LathiC03 2017925 1554 page 273 37 36 System Response to Internal Conditions The ZeroInput Response 273 not provide a closedform solution recursion is generally not the preferred method of solving difference equations EXAMPLE 314 Iterative Solution to ZeroInput Response Using the initial conditions y1 2 and y2 1 use MATLAB to iteratively compute and then plot the zeroinput response for the system described by E2 156E 081yn E 3xn n 220 y 12zeroslengthn21 for k 1lengthn2 yk2 156yk1081yk end clf stemnyk xlabeln ylabelyn axis2 20 15 25 0 5 10 15 20 n 1 0 1 2 yn Figure 318 Zeroinput response for Ex 314 REPEATED ROOTS So far we have assumed the system to have N distinct characteristic roots γ1 γ2 γN with corresponding characteristic modes γ n 1 γ n 2 γ n N If two or more roots coincide repeated roots the form of characteristic modes is modified Direct substitution shows that if a root γ repeats r times root of multiplicity r the corresponding characteristic modes for this root are γ n nγ n n2γ n nr1γ n Thus if the characteristic equation of a system is Qγ γ γ1rγ γr1γ γr2 γ γN then the zeroinput response of the system is y0n c1 c2n c3n2 crnr1γ n 1 cr1γ n r1 cr2γ n r2 cnγ n N 03LathiC03 2017925 1554 page 274 38 274 CHAPTER 3 TIMEDOMAIN ANALYSIS OF DISCRETETIME SYSTEMS EXAMPLE 315 ZeroInput Response of a SecondOrder System with Repeated Roots Consider a secondorder difference equation with repeated roots E2 6E 9yn 2E2 6Exn Determine the zeroinput response y0n if the initial conditions are y01 13 and y02 29 The characteristic polynomial is γ 2 6γ 9 γ 32 and we have a repeated characteristic root at γ 3 The characteristic modes are 3n and n3n Hence the zeroinput response is y0n c1 c2n3n Although we can determine the constants c1 and c2 from the initial conditions following a procedure similar to Ex 313 we instead use MATLAB to perform the needed calculations c inv31 13132 2321329 c 4 3 Thus the zeroinput response is y0n 4 3n3n n 0 COMPLEX ROOTS As in the case of continuoustime systems the complex roots of a discretetime system will occur in pairs of conjugates if the system equation coefficients are real Complex roots can be treated exactly as we would treat real roots However just as in the case of continuoustime systems we can also use the real form of solution as an alternative First we express the complex conjugate roots γ and γ in polar form If γ is the magnitude and β is the angle of γ then γ γ ejβ and γ γ ejβ The zeroinput response is given by y0n c1γ n c2γ n c1γ nejβn c2γ nejβn For a real system c1 and c2 must be conjugates so that y0n is a real function of n Let c1 c 2ejθ and c2 c 2ejθ 03LathiC03 2017925 1554 page 280 44 280 CHAPTER 3 TIMEDOMAIN ANALYSIS OF DISCRETETIME SYSTEMS EXAMPLE 319 Filtering Perspective of the Unit Impulse Response Use the MATLAB filter command to solve Ex 318 There are several ways to find the impulse response using MATLAB In this method we first specify the unit impulse function which will serve as our input Vectors a and b are created to specify the system The filter command is then used to determine the impulse response In fact this method can be used to determine the zerostate response for any input n 019 delta n 10n0 a 1 06 016 b 5 0 0 h filterbadeltan clf stemnhk xlabeln ylabelhn 0 5 10 15 20 n 0 2 4 6 hn Figure 319 Impulse response for Ex 319 Comment Although it is relatively simple to determine the impulse response hn by using the procedure in this section in Ch 5 we shall discuss the much simpler method of the ztransform 38 SYSTEM RESPONSE TO EXTERNAL INPUT THE ZEROSTATE RESPONSE The zerostate response yn is the system response to an input xn when the system is in the zero state In this section we shall assume that systems are in the zero state unless mentioned otherwise so that the zerostate response will be the total response of the system Here we follow the procedure parallel to that used in the continuoustime case by expressing an arbitrary input xn as a sum of impulse components A signal xn in Fig 320a can be expressed as a sum of impulse components such as those depicted in Figs 320b320f The component of xn at n m is xmδn m and xn is the sum of all these components summed from m to 03LathiC03 2017925 1554 page 291 55 38 System Response to External Input The ZeroState Response 291 EXAMPLE 324 SlidingTape Method for the Convolution Sum Use the slidingtape method to convolve the two sequences xn and gn depicted in Figs 323a and 323b respectively In this procedure we write the sequences xnandgn in the slots of two tapes x tape and g tape Fig 323c Now leave the x tape stationary to correspond to xm The gm tape is obtained by inverting the gm tape about the origin m 0 so that the slots corresponding to x0 and g0 remain aligned Fig 323d We now shift the inverted tape by n slots multiply values on two tapes in adjacent slots and add all the products to find cn Figures 323d323i show the cases for n 05 Figures 323j 323k and 323l show the cases for n 12 and 3 respectively For the case of n 0 for example Fig 323d c0 2 1 1 1 0 1 3 For n 1 Fig 323e c1 2 1 1 1 0 1 1 1 2 Similarly c2 2 1 1 1 0 1 1 1 2 1 0 c3 2 1 1 1 0 1 1 1 2 1 3 1 3 c4 2 1 1 1 0 1 1 1 2 1 3 1 4 1 7 c5 2 1 1 1 0 1 1 1 2 1 3 1 4 1 7 Figure 323i shows that cn 7 for n 4 Similarly we compute cn for negative n by sliding the tape backward one slot at a time as shown in the plots corresponding to n 1 2 and 3 respectively Figs 323j 323k and 323l c1 2 1 1 1 3 c2 2 1 2 c3 0 Figure 323l shows that cn 0 for n 3 Figure 323m shows the plot of cn 03LathiC03 2017925 1554 page 293 57 38 System Response to External Input The ZeroState Response 293 DRILL 319 SlidingTape Method for the Convolution Sum Use the graphical procedure of Ex 324 slidingtape technique to show that xngn cn in Fig 324 Verify the width property of convolution a xn 1 n 2 3 1 2 3 4 5 6 0 1 2 3 4 5 gn b 1 1 2 3 4 5 6 7 8 9 1011 cn c 1 n n 3 0 6 9 8 Figure 324 Signals for Drill 319 EXAMPLE 325 Convolution of Two FiniteDuration Signals Using MATLAB For the signals xn and gn depicted in Fig 324 use MATLAB to compute and plot cn xn gn x 0 1 2 3 2 1 g 1 1 1 1 1 1 n 01lengthxlengthg2 c convxg clf stemnck xlabeln ylabelcn axis05 105 0 10 0 2 4 6 8 10 n 0 5 10 cn Figure 325 Convolution result for Ex 325 03LathiC03 2017925 1554 page 300 64 300 CHAPTER 3 TIMEDOMAIN ANALYSIS OF DISCRETETIME SYSTEMS n n n n n n n n a b d c n n n n Complex plane Figure 327 Characteristic roots locations and the corresponding characteristic modes 03LathiC03 2017925 1554 page 305 69 310 Intuitive Insights into System Behavior 305 DRILL 321 Assessing Stability by Characteristic Roots Using the complex plane locate the characteristic roots of the following systems and use the characteristic root locations to determine external and internal stability of each system a E 1E2 6E 25yn 3Exn b E 12E 05yn E2 2E 3xn ANSWERS Both systems are BIBOand asymptotically unstable 310 INTUITIVE INSIGHTS INTO SYSTEM BEHAVIOR The intuitive insights into the behavior of continuoustime systems and their qualitative proofs discussed in Sec 26 also apply to discretetime systems For this reason we shall merely mention here without discussion some of the insights presented in Sec 26 The systems entire zeroinput and zerostate behavior is strongly influenced by the characteristic roots or modes of the system The system responds strongly to input signals similar to its characteristic modes and poorly to inputs very different from its characteristic modes In fact when the input is a characteristic mode of the system the response goes to infinity provided the mode is a nondecaying signal This is the resonance phenomenon The width of an impulse response hn indicates the response time time required to respond fully to an input of the system It is the time constant of the system Discretetime pulses are generally dispersed when passed through a discretetime system The amount of dispersion or spreading out is equal to the system time constant or width of hn The system time constant also determines the rate at which the system can transmit information A smaller time constant corresponds to a higher rate of information transmission and vice versa We keep in mind that concepts such as time constant and pulse dispersion only coarsely illustrate system behavior Let us illustrate these ideas with an example EXAMPLE 328 Intuitive Insights into Lowpass DT System Behavior Determine the time constant rise time pulse dispersion and filter characteristics of a lowpass DT system with impulse response hn 206nun This part of the discussion applies to systems with impulse response hn that is a mostly positive or mostly negative pulse 03LathiC03 2017925 1554 page 307 71 311 MATLAB DiscreteTime Signals and Systems 307 A true discretetime function is undefined or zero for noninteger n Although anonymous function f is intended as a discretetime function its present construction does not restrict n to be integer and it can therefore be misused For example MATLAB dutifully returns 08606 to f05 when a NaN notanumber or zero is more appropriate The user is responsible for appropriate function use Next consider plotting the discretetime function fn over 10 n 10 The stem command simplifies this task n 1010 stemnfnk xlabeln ylabelfn Here stem operates much like the plot command dependent variable fn is plotted against independent variable n with black lines The stem command emphasizes the discretetime nature of the data as Fig 331 illustrates For discretetime functions the operations of shifting inversion and scaling can have surprising results Compare f2n with f2n 1 Contrary to the continuous case the second is not a shifted version of the first We can use separate subplots each over 10 n 10 to help illustrate this fact Notice that unlike the plot command the stem command cannot simultaneously plot multiple functions on a single axis overlapping stem lines would make such plots difficult to read anyway subplot211 stemnf2nk ylabelf2n subplot212 stemnf2n1k ylabelf2n1 xlabeln The results are shown in Fig 332 Interestingly the original function fn can be recovered by interleaving samples of f2n and f2n 1 and then timereflecting the result Care must always be taken to ensure that MATLAB performs the desired computations Our anonymous function f is a case in point although it correctly downsamples it does not properly upsample see Prob 3112 MATLAB does what it is told but it is not always told how to do everything correctly 10 5 0 5 10 n 05 0 05 1 fn Figure 331 fn over 10 n 10 03LathiC03 2017925 1554 page 311 75 311 MATLAB DiscreteTime Signals and Systems 311 function y CH3MP1baxyi CH3MP1m Chapter 3 MATLAB Program 1 Function Mfile filters data x to create y INPUTS b vector of feedforward coefficients a vector of feedback coefficients x input data vector yi vector of initial conditions y1 y2 OUTPUTS y vector of filtered output data yi flipudyi Properly format ICs y yizeroslengthx1 Preinitialize y beginning with ICs x zeroslengthyi1x Append x with zeros to match size of y b ba1a aa1 Normalize coefficients for n lengthyi1lengthy for nb 0lengthb1 yn yn bnb1xnnb Feedforward terms end for na 1lengtha1 yn yn ana1ynna Feedback terms end end y ylengthyi1end Strip off ICs for final output Most instructions in CH3MP1 have been discussed now we turn to the flipud instruction The flip updown command flipud reverses the order of elements in a column vector Although not used here the flip leftright command fliplr reverses the order of elements in a row vector Note that typing help filename displays the first contiguous set of comment lines in an Mfile Thus it is good programming practice to document Mfiles as in CH3MP1 with an initial block of clear comment lines As an exercise the reader should verify that CH3MP1 correctly computes the impulse response hn the zerostate response yn the zeroinput response y0n and the total response yny0n 3114 DiscreteTime Convolution Convolution of two finiteduration discretetime signals is accomplished by using the conv command For example the discretetime convolution of two length4 rectangular pulses gn unun4unun4 is a length441 7 triangle Representing unun4 by the vector 1111 the convolution is computed by conv1 1 1 11 1 1 1 ans 1 2 3 4 3 2 1 Notice that un4ununun4 is also computed by conv1 1 1 11 1 1 1 and obviously yields the same result The difference between these two cases is the regions of support 0 n 6 for the first and 4 n 2 for the second Although the conv command 03LathiC03 2017925 1554 page 313 77 313 Summary 313 312 APPENDIX IMPULSE RESPONSE FOR A SPECIAL CASE When aN 0 A0 bNaN becomes indeterminate and the procedure needs to be modified slightly When aN 0 QE can be expressed as E ˆQE and Eq 326 can be expressed as E ˆQEhn PEδn PEEδn 1 EPEδn 1 Hence ˆQEhn PEδn 1 In this case the input vanishes not for n 1 but for n 2 Therefore the response consists not only of the zeroinput term and an impulse A0δn at n 0 but also of an impulse A1δn1 at n 1 Therefore hn A0δn A1δn 1 ycnun We can determine the unknowns A0 A1 and the N 1 coefficients in ycn from the N 1 number of initial values h0 h1 hN determined as usual from the iterative solution of the equation QEhn PEδn Similarly if aN aN1 0 we need to use the form hn A0δnA1δn1A2δn2ycnun The N 1 unknown constants are determined from the N 1 values h0 h1 hN determined iteratively and so on 313 SUMMARY This chapter discusses timedomain analysis of LTID linear timeinvariant discretetime systems The analysis is parallel to that of LTIC systems with some minor differences Discretetime systems are described by difference equations For an Nthorder system N auxiliary conditions must be specified for a unique solution Characteristic modes are discretetime exponentials of the form γ n corresponding to an unrepeated root γ and the modes are of the form niγ n corresponding to a repeated root γ The unit impulse function δn is a sequence of a single number of unit value at n 0 The unit impulse response hn of a discretetime system is a linear combination of its characteristic modes The zerostate response response due to external input of a linear system is obtained by breaking the input into impulse components and then adding the system responses to all the impulse components The sum of the system responses to the impulse components is in the form of a sum known as the convolution sum whose structure and properties are similar to the convolution integral The system response is obtained as the convolution sum of the input xn with the systems impulse response hn Therefore the knowledge of the systems impulse response allows us to determine the system response to any arbitrary input LTID systems have a very special relationship to the everlasting exponential signal zn because the response of an LTID system to such an input signal is the same signal within a multiplicative ˆQγ is now an N 1order polynomial Hence there are only N 1 unknowns in ycn There is a possibility of an impulse δn in addition to characteristic modes 03LathiC03 2017925 1554 page 320 84 320 CHAPTER 3 TIMEDOMAIN ANALYSIS OF DISCRETETIME SYSTEMS 355 Solve the following equation recursively first three terms only yn 2 3yn 1 2yn xn 2 3xn 1 3xn with xn 3nun y1 3 and y2 2 356 Repeat Prob 355 for yn 2yn 1 yn 2 2xn xn 1 withxn 3nuny1 2andy2 3 361 Given y01 3 and y02 1 determine the closedform expression of the zeroinput response y0n of an LTID system described by the equation yn 1 6yn1 1 6yn2 1 3xn 2 3xn 2 362 Solve yn 2 3yn 1 2yn 0 if y1 0 and y2 1 363 Solve yn 2 2yn 1 yn 0 if y1 1 and y2 1 364 Solve yn 2 2yn 1 2yn 0 if y1 1 and y2 0 365 For the general Nthorder difference Eq 316 letting a1 a2 aN1 0 results in a general causal Nthorder LTI nonre cursive difference equation yn b0xn b1xn 1 bNxn N Show that the characteristic roots for this system are zerohence that the zeroinput response is zero Consequently the total response consists of the zerostate component only 366 Leonardo Pisano Fibonacci a famous thirteenth century mathematician generated the sequence of integers 0112358132134 while addressing oddly enough a problem involving rabbit reproduction An element of the Fibonacci sequence is the sum of the previous two a Find the constantcoefficient difference equation whose zeroinput response fn with auxiliary conditions f1 0 and f2 1 is a Fibonacci sequence Given fn is the system output what is the system input b What are the characteristic roots of this system Is the system stable c Designating 0 and 1 as the first and second Fibonacci numbers determine the fiftieth Fibonacci number Determine the one thou sandth Fibonacci number 367 Find vn the voltage at the nth node of the resistive ladder depicted in Fig P348 if V 100 volts and a 2 Hint 1 Consider the node equation at the nth node with voltage vn Hint 2 See Prob 348 for the equation for vn The auxiliary conditions are v0 100 and vN 0 368 Consider the discretetime system yn yn 1 025yn 2 3xn 8 Find the zero input response y0n if y01 1 and y01 1 369 Provide a standardform polynomial QX such that QEyn xn corresponds to a marginally stable thirdorder LTID system and QDyt xt corresponds to a stable thirdorder LTIC system 371 Find the unit impulse response hn of systems specified by the following equations a yn 1 2yn xn b yn 2yn 1 xn 372 Determine the unit impulse response hn of the following systems In each case use recursion to verify the n 3 value of the closedform expression of hn a E2 1yn E 05xn b yn yn 1 025yn 2 xn c yn 1 6yn 1 1 6yn 2 1 3xn 2 03LathiC03 2017925 1554 page 326 90 326 CHAPTER 3 TIMEDOMAIN ANALYSIS OF DISCRETETIME SYSTEMS continues sometimes for many many cups of coffee Joe has noted that his coffee tends to taste sweeter with the number of refills Let independent variable n designate the coffee refill number In this way n 0 indicates the first cup of coffee n 1 is the first refill and so forth Let xn represent the sugar measured in teaspoons added into the system a coffee mug on refill n Let yn designate the amount of sugar again teaspoons contained in the mug on refill n a The sugar teaspoons in Joes coffee can be represented using a standard secondorder constant coefficient difference equation yn a1yn 1 a2yn 2 b0xn b1xn 1 b2xn 2 Determine the constants a1 a2 b0 b1 and b2 b Determine xn the driving function to this system c Solve the difference equation for yn This requires finding the total solution Joe always starts with a clean mug from the dishwasher so y1 the sugar content before the first cup is zero d Determine the steadystate value of yn That is what is yn as n If possible suggest a way of modifying xn so that the sugar content of Joes coffee remains a constant for all nonnegative n 3837 A system is called complex if a realvalued input can produce a complexvalued output Con sider a causal complex system described by a firstorder constant coefficient linear difference equation jE 05yn 5Exn a Determine the impulse response function hn for this system b Given input xn un 5 and initial con dition y01 j determine the systems total output yn for n 0 3838 A discretetime LTI system has impulse response function hn nun 2 un 2 a Carefully sketch the function hn over 5 n 5 b Determine the difference equation represen tation of this system using yn to designate the output and xn to designate the input 3839 Consider three discretetime signals xn yn and zn Denoting convolution as iden tify the expressions that isare equivalent to xnyn zn a xn ynzn b xnyn xnzn c xnyn zn d none of the above Justify your answer 3840 A causal system with input xn and output yn is described by yn nyn 1 xn a By recursion determine the first six nonzero values of hn the response to xn δn Do you think this system is BIBOstable Why b Compute yR4 recursively from yRn nyRn1 xn assuming all initial condi tions are zero and xn un The subscript R is only used to emphasize a recursive solution c Define yCn xnhn Using xn un and hn from part a compute yC4 The subscript C is only used to emphasize a convolution solution d In this chapter both recursion and convo lution are presented as potential methods to compute the zerostate response ZSR of a discretetime system Comparing parts b and c we see that yR4 yC4 Why are the two results not the same Which method if any yields the correct ZSR value 391 In Sec 391 we showed that for BIBO stability in an LTID system it is sufficient for its impulse response hn to satisfy Eq 343 Show that this is also a necessary condition for the system to be BIBOstable In other words show that if Eq 343 is not satisfied there exists a bounded input that produces unbounded output Hint Assume that a system exists for which hn violates Eq 343 yet its output is bounded for every bounded input Establish the contradiction in this statement by considering an input xn defined by xn1 m 1 when hm 0 and xn1m 1 when hm 0 where n1 is some fixed integer 04LathiC04 2017925 1946 page 344 15 344 CHAPTER 4 CONTINUOUSTIME SYSTEM ANALYSIS EXAMPLE 44 Inverse Laplace Transform with MATLAB Using the MATLAB residue command determine the inverse Laplace transform of each of the following functions a Xas 2s2 5 s2 3s 2 b Xbs 2s2 7s 4 s 1s 22 c Xcs 8s2 21s 19 s 2s2 s 7 In each case we use the MATLAB residue command to perform the necessary partial fraction expansions The inverse Laplace transform follows using Table 41 a num 2 0 5 den 1 3 2 r p k residuenumden r 13 7 p 2 1 k 2 Therefore Xas 13s 2 7s 1 2 and xat 13e2t 7etut 2δt b num 2 7 4 den conv1 1conv1 21 2 r p k residuenumden r 3 2 1 p 2 2 1 k Therefore Xbs 3s 2 2s 22 1s 1 and xbt 3e2t 2te2t etut c In this case a few calculations are needed beyond the results of the residue command so that pair 10b of Table 41 can be utilized num 8 21 19 den conv1 21 1 7 r p k residuenumden 04LathiC04 2017925 1946 page 345 16 41 The Laplace Transform 345 r 35000048113i 35000048113i 10000 p 0500025981i 0500025981i 20000 k ang angler mag absr ang 013661 013661 0 mag 35329 35329 10000 Thus Xcs 1 s 2 35329ej013661 s 05 j25981 35329ej013661 s 05 j25981 and xct e2t 17665e05t cos25981t 01366ut EXAMPLE 45 Symbolic Laplace and Inverse Laplace Transforms with MATLAB Using MATLABs symbolic math toolbox determine the following a the direct unilateral Laplace transform of xat sinat cosbt b the inverse unilateral Laplace transform of Xbs as2s2 b2 a Here we use the sym command to symbolically define our variables and expression for xat and then we use the laplace command to compute the unilateral Laplace transform syms a b t xa sinatcosbt Xa laplacexa Xa aa2 s2 sb2 s2 Therefore Xas a s2a2 s s2b2 It is also easy to use MATLAB to determine Xas in standard rational form Xa collectXa Xa a2s ab2 as2 s3s4 a2 b2s2 a2b2 Thus we also see that Xas s3as2a2sab2 s4a2b2s2a2b2 b A similar approach is taken for the inverse Laplace transform except that the ilaplace command is used rather than the laplace command 04LathiC04 2017925 1946 page 347 18 41 The Laplace Transform 347 PierreSimon de Laplace and Oliver Heaviside been unable to explain the irregularities of some heavenly bodies in desperation he concluded that God himself must intervene now and then to prevent such catastrophes as Jupiter eventually falling into the sun and the moon into the earth as predicted by Newtons calculations Laplace proposed to show that these irregularities would correct themselves periodically and that a little patiencein Jupiters case 929 yearswould see everything returning automatically to order thus there was no reason why the solar and the stellar systems could not continue to operate by the laws of Newton and Laplace to the end of time 4 Laplace presented a copy of Mécanique céleste to Napoleon who after reading the book took Laplace to task for not including God in his scheme You have written this huge book on the system of the world without once mentioning the author of the universe Sire Laplace retorted I had no need of that hypothesis Napoleon was not amused and when he reported this reply to another great mathematicianastronomer Louis de Lagrange the latter remarked Ah but that is a fine hypothesis It explains so many things 5 Napoleon following his policy of honoring and promoting scientists made Laplace the minister of the interior To Napoleons dismay however the new appointee attempted to bring the spirit of infinitesimals into administration and so Laplace was transferred hastily to the Senate OLIVER HEAVISIDE 18501925 Although Laplace published his transform method to solve differential equations in 1779 the method did not catch on until a century later It was rediscovered independently in a rather awkward form by an eccentric British engineer Oliver Heaviside 18501925 one of the tragic figures in the history of science and engineering Despite his prolific contributions to electrical engineering he was severely criticized during his lifetime and was neglected later to the point that 04LathiC04 2017925 1946 page 348 19 348 CHAPTER 4 CONTINUOUSTIME SYSTEM ANALYSIS hardly a textbook today mentions his name or credits him with contributions Nevertheless his studies had a major impact on many aspects of modern electrical engineering It was Heaviside who made transatlantic communication possible by inventing cable loading but few mention him as a pioneer or an innovator in telephony It was Heaviside who suggested the use of inductive cable loading but the credit is given to M Pupin who was not even responsible for building the first loading coil In addition Heaviside was 6 The first to find a solution to the distortionless transmission line The innovator of lowpass filters The first to write Maxwells equations in modern form The codiscoverer of rate energy transfer by an electromagnetic field An early champion of the nowcommon phasor analysis An important contributor to the development of vector analysis In fact he essentially created the subject independently of Gibbs 7 An originator of the use of operational mathematics used to solve linear integrodifferential equations which eventually led to rediscovery of the ignored Laplace transform The first to theorize along with Kennelly of Harvard that a conducting layer the KennellyHeaviside layer of atmosphere exists which allows radio waves to follow earths curvature instead of traveling off into space in a straight line The first to posit that an electrical charge would increase in mass as its velocity increases an anticipation of an aspect of Einsteins special theory of relativity 8 He also forecast the possibility of superconductivity Heaviside was a selfmade selfeducated man Although his formal education ended with elementary school he eventually became a pragmatically successful mathematical physicist He began his career as a telegrapher but increasing deafness forced him to retire at the age of 24 He then devoted himself to the study of electricity His creative work was disdained by many professional mathematicians because of his lack of formal education and his unorthodox methods Heaviside had the misfortune to be criticized both by mathematicians who faulted him for lack of rigor and by men of practice who faulted him for using too much mathematics and thereby confusing students Many mathematicians trying to find solutions to the distortionless transmission line failed because no rigorous tools were available at the time Heaviside succeeded because he used mathematics not with rigor but with insight and intuition Using his much maligned operational method Heaviside successfully attacked problems that the rigid mathematicians could not solve problems such as the flowofheat in a body of spatially varying conductivity Heaviside brilliantly used this method in 1895 to demonstrate a fatal flaw in Lord Kelvins determination of the geological age of the earth by secular cooling he used the same flowofheat theory as for his cable analysis Yet the mathematicians of the Royal Society remained unmoved and were not the least impressed by the fact that Heaviside had found the answer to problems no one else could solve Many mathematicians who examined his work dismissed it Heaviside developed the theory for cable loading George Campbell built the first loading coil and the telephone circuits using Campbells coils were in operation before Pupin published his paper In the legal fight over the patent however Pupin won the battle he was a shrewd selfpromoter and Campbell had poor legal support 04LathiC04 2017925 1946 page 349 20 42 Some Properties of the Laplace Transform 349 with contempt asserting that his methods were either complete nonsense or a rehash of known ideas 6 Sir William Preece the chief engineer of the British Post Office a savage critic of Heaviside ridiculed Heavisides work as too theoretical and therefore leading to faulty conclusions Heavisides work on transmission lines and loading was dismissed by the British Post Office and might have remained hidden had not Lord Kelvin himself publicly expressed admiration for it 6 Heavisides operational calculus may be formally inaccurate but in fact it anticipated the operational methods developed in more recent years 9 Although his method was not fully understood it provided correct results When Heaviside was attacked for the vague meaning of his operational calculus his pragmatic reply was Shall I refuse my dinner because I do not fully understand the process of digestion Heaviside lived as a bachelor hermit often in nearsqualid conditions and died largely unnoticed in poverty His life demonstrates the persistent arrogance and snobbishness of the intellectual establishment which does not respect creativity unless it is presented in the strict language of the establishment 42 SOME PROPERTIES OF THE LAPLACE TRANSFORM Properties of the Laplace transform are useful not only in the derivation of the Laplace transform of functions but also in the solutions of linear integrodifferential equations A glance at Eqs 42 and 41 shows that there is a certain measure of symmetry in going from xt to Xs and vice versa This symmetry or duality is also carried over to the properties of the Laplace transform This fact will be evident in the following development We are already familiar with two properties linearity Eq 43 and the uniqueness property of the Laplace transform discussed earlier 421 Time Shifting The timeshifting property states that if xt Xs then for t0 0 xt t0 Xsest0 412 Observe that xt starts at t 0 and therefore xt t0 starts at t t0 This fact is implicit but is not explicitly indicated in Eq 412 This often leads to inadvertent errors To avoid such a pitfall we should restate the property as follows If xtut Xs then xt t0ut t0 Xsest0 t0 0 04LathiC04 2017925 1946 page 364 35 364 CHAPTER 4 CONTINUOUSTIME SYSTEM ANALYSIS of linear systems because the response obtained cannot be separated into zeroinput and zerostate components As we know the zerostate component represents the system response as an explicit function of the input and without knowing this component it is not possible to assess the effect of the input on the system response in a general way The L version can separate the response in terms of the natural and the forced components which are not as interesting as the zeroinput and the zerostate components Note that we can always determine the natural and the forced components from the zeroinput and the zerostate components eg Eq 244 from Eq 243 but the converse is not true Because of these and some other problems electrical engineers wisely started discarding the L version in the early 1960s It is interesting to note the timedomain duals of these two Laplace versions The classical method is the dual of the L method and the convolution zeroinputzerostate method is the dual of the L method The first pair uses the initial conditions at 0 and the second pair uses those at t 0 The first pair the classical method and the L version is awkward in the theoretical study of linear system analysis It was no coincidence that the L version was adopted immediately after the introduction to the electrical engineering community of statespace analysis which uses zeroinputzerostate separation of the output DRILL 47 Laplace Transform to Solve a SecondOrder Linear Differential Equation Solve d2yt dt2 4dyt dt 3yt 2dxt dt xt for the input xt ut The initial conditions are y0 1 and y0 2 ANSWER yt 1 31 9et 7e3tut EXAMPLE 413 Laplace Transform to Solve an Electric Circuit In the circuit of Fig 47a the switch is in the closed position for a long time before t 0 when it is opened instantaneously Find the inductor current yt for t 0 When the switch is in the closed position for a long time the inductor current is 2 amperes and the capacitor voltage is 10 volts When the switch is opened the circuit is equivalent to that depicted in Fig 47b with the initial inductor current y0 2 and the initial capacitor voltage vC0 10 The input voltage is 10 volts starting at t 0 and therefore can be represented by 10ut 04LathiC04 2017925 1946 page 371 42 43 Solution of Differential and IntegroDifferential Equations 371 433 Stability Equation 427 shows that the denominator of Hs is Qs which is apparently identical to the characteristic polynomial Qλ defined in Ch 2 Does this mean that the denominator of Hs is the characteristic polynomial of the system This may or may not be the case since if Ps and Qs in Eq 427 have any common factors they cancel out and the effective denominator of Hs is not necessarily equal to Qs Recall also that the system transfer function Hs like ht is defined in terms of measurements at the external terminals Consequently Hs and ht are both external descriptions of the system In contrast the characteristic polynomial Qs is an internal description Clearly we can determine only external stability that is BIBO stability from Hs If all the poles of Hs are in LHP all the terms in ht are decaying exponentials and ht is absolutely integrable see Eq 245 Consequently the system is BIBOstable Otherwise the system is BIBOunstable Beware of right halfplane poles So far we have assumed that Hs is a proper function that is M N We now show that if Hs is improper that is if M N the system is BIBOunstable In such a case using long division we obtain Hs Rs Hs where Rs is an M Nthorder polynomial and Hs is a proper transfer function For example Hs s3 4s2 4s 5 s2 3s 2 s s2 2s 5 s2 3s 2 As shown in Eq 431 the term s is the transfer function of an ideal differentiator If we apply step function bounded input to this system the output will contain an impulse unbounded output Clearly the system is BIBOunstable Moreover such a system greatly amplifies noise because differentiation enhances higher frequencies which generally predominate in a noise signal These Values of s for which Hs is are the poles of Hs Thus poles of Hs are the values of s for which the denominator of Hs is zero 04LathiC04 2017925 1946 page 373 44 44 Analysis of Electrical Networks The Transformed Network 373 black box with only the input and the output terminals accessible any measurement from these external terminals would show that the transfer function of the system is 1s1 without any hint of the fact that the system is housing an unstable system Fig 49b The impulse response of the cascade system is ht etut which is absolutely integrable Consequently the system is BIBOstable To determine the asymptotic stability we note that S1 has one characteristic root at 1 and S2 also has one root at 1 Recall that the two systems are independent one does not load the other and the characteristic modes generated in each subsystem are independent of the other Clearly the mode et will not be eliminated by the presence of S2 Hence the composite system has two characteristic roots located at 1 and the system is asymptotically unstable though BIBOstable Interchanging the positions of S1 and S2 makes no difference in this conclusion This example shows that BIBO stability can be misleading If a system is asymptotically unstable it will destroy itself or more likely lead to saturation condition because of unchecked growth of the response due to intended or unintended stray initial conditions BIBO stability is not going to save the system Control systems are often compensated to realize certain desirable characteristics One should never try to stabilize an unstable system by canceling its RHP poles with RHP zeros Such a misguided attempt will fail not because of the practical impossibility of exact cancellation but for the more fundamental reason as just explained DRILL 49 BIBO and Asymptotic Stability Show that an ideal integrator is marginally stable but BIBOunstable 434 Inverse Systems If Hs is the transfer function of a system S then Si its inverse system has a transfer function His given by His 1 Hs This follows from the fact the cascade of S with its inverse system Si is an identity system with impulse response δt implying HsHis 1 For example an ideal integrator and its inverse an ideal differentiator have transfer functions 1s and s respectively leading to HsHis 1 44 ANALYSIS OF ELECTRICAL NETWORKS THE TRANSFORMED NETWORK Example 412 shows how electrical networks may be analyzed by writing the integrodifferential equations of the system and then solving these equations by the Laplace transform We now show that it is also possible to analyze electrical networks directly without having to write the 04LathiC04 2017925 1946 page 384 55 384 CHAPTER 4 CONTINUOUSTIME SYSTEM ANALYSIS Figure 417 a SallenKey circuit and b its equivalent We are required to find Hs Vos Vis assuming all initial conditions to be zero Figure 417b shows the transformed version of the circuit in Fig 417a The noninverting amplifier is replaced by its equivalent circuit All the voltages are replaced by their Laplace transforms and all the circuit elements are shown by their impedances All the initial conditions are assumed to be zero as required for determining Hs We shall use node analysis to derive the result There are two unknown node voltages Vas and Vbs requiring two node equations At node a IR1s the current in R1 leaving the node a is Vas VisR1 Similarly IR2s the current in R2 leaving the node a is Vas VbsR2 and IC1s the current in capacitor C1 leaving the node a is Vas VosC1s Vas KVbsC1s 04LathiC04 2017925 1946 page 387 58 45 Block Diagrams 387 We can extend this result to any number of transfer functions in cascade It follows from this discussion that the subsystems in cascade can be interchanged without affecting the overall transfer function This commutation property of LTI systems follows directly from the commutative and associative property of convolution We have already proved this property in Sec 243 Every possible ordering of the subsystems yields the same overall transfer function However there may be practical consequences such as sensitivity to parameter variation affecting the behavior of different ordering Similarly when two transfer functions H1s and H2s appear in parallel as illustrated in Fig 418c the overall transfer function is given by H1s H2s the sum of the two transfer functions The proof is trivial This result can be extended to any number of systems in parallel When the output is fed back to the input as shown in Fig 418d the overall transfer function YsXs can be computed as follows The inputs to the adder are Xs and HsYs Therefore Es the output of the adder is Es Xs HsYs But Ys GsEs GsXs HsYs Therefore Ys1 GsHs GsXs so that Ys Xs Gs 1 GsHs 435 Therefore the feedback loop can be replaced by a single block with the transfer function shown in Eq 435 see Fig 418d In deriving these equations we implicitly assume that when the output of one subsystem is connected to the input of another subsystem the latter does not load the former For example the transfer function H1s in Fig 418b is computed by assuming that the second subsystem H2s was not connected This is the same as assuming that H2s does not load H1s In other words the inputoutput relationship of H1s will remain unchanged regardless of whether H2s is connected Many modern circuits use op amps with high input impedances so this assumption is justified When such an assumption is not valid H1s must be computed under operating conditions ie with H2s connected EXAMPLE 421 Transfer Functions of Feedback Systems Using MATLAB Consider the feedback system of Fig 418d with Gs Kss 8 and Hs 1 Use MATLAB to determine the transfer function for each of the following cases a K 7 b K 16 and c K 80 We solve these cases using the control system toolbox function feedback 04LathiC04 2017925 1946 page 388 59 388 CHAPTER 4 CONTINUOUSTIME SYSTEM ANALYSIS a H tf11 K 7 G tf0 0 K1 8 0 TFa feedbackGH Ha 7 s2 8 s 7 Thus Has 7s2 8s 7 b H tf11 K 16 G tf0 0 K1 8 0 TFb feedbackGH Hb 16 s2 8 s 16 Thus Hbs 16s2 8s 16 c H tf11 K 80 G tf0 0 K1 8 0 TFc feedbackGH Hc 80 s2 8 s 80 Thus Hcs 80s2 8s 80 46 SYSTEM REALIZATION We now develop a systematic method for realization or implementation of an arbitrary Nthorder transfer function The most general transfer function with M N is given by Hs b0sN b1sN1 bN1s bN sN a1sN1 aN1s aN 436 Since realization is basically a synthesis problem there is no unique way of realizing a system A given transfer function can be realized in many different ways A transfer function Hs can be realized by using integrators or differentiators along with adders and multipliers We avoid use of differentiators for practical reasons discussed in Secs 21 and 433 Hence in our implementation we shall use integrators along with scalar multipliers and adders We are already familiar with representation of all these elements except the integrator The integrator can be represented by a box with integral sign timedomain representation Fig 419a or by a box with transfer function 1s frequencydomain representation Fig 419b 04LathiC04 2017925 1946 page 392 63 392 CHAPTER 4 CONTINUOUSTIME SYSTEM ANALYSIS EXAMPLE 422 Canonic Direct Form Realizations Find the canonic direct form realization of the following transfer functions a 5 s 7 b s s 7 c s 5 s 7 d 4s 28 s2 6s 5 All four of these transfer functions are special cases of Hs in Eq 436 a The transfer function 5s 7 is of the first order N 1 therefore we need only one integrator for its realization The feedback and feedforward coefficients are a1 7 and b0 0 b1 5 The realization is depicted in Fig 423a Because N 1 there is a single feedback connection from the output of the integrator to the input adder with coefficient a1 7 For N 1 generally there are N 1 2 feedforward connections However in this case b0 0 and there is only one feedforward connection with coefficient b1 5 from the output of the integrator to the output adder Because there is only one input signal to the output adder we can do away with the adder as shown in Fig 423a b Hs s s 7 In this firstorder transfer function b1 0 The realization is shown in Fig 423b Because there is only one signal to be added at the output adder we can discard the adder c Hs s 5 s 7 The realization appears in Fig 423c Here Hs is a firstorder transfer function with a1 7 and b0 1 b1 5 There is a single feedback connection with coefficient 7 from the integrator output to the input adder There are two feedforward connections Fig 423c When M N as in this case Hs can also be realized in another way by recognizing that Hs 1 2 s 7 We now realize Hs as a parallel combination of two transfer functions as indicated by this equation 04LathiC04 2017925 1946 page 406 77 406 CHAPTER 4 CONTINUOUSTIME SYSTEM ANALYSIS gain which is reduced from 10000 to 99 There is no dearth of forward gain obtained by cascading stages But low sensitivity is extremely precious in precision systems Now consider what happens when we add instead of subtract the signal fed back to the input Such addition means the sign on the feedback connection is instead of which is same as changing the sign of H in Fig 434 Consequently T G 1 GH If we let G 10000 as before and H 09 104 then T 10000 1 09104104 100000 Suppose that because of aging or replacement of some transistors the gain of the forward amplifier changes to 11000 The new gain of the feedback amplifier is T 11000 1 0911000104 1100000 Observe that in this case a mere 10 increase in the forward gain G caused 1000 increase in the gain T from 100000 to 1100000 Clearly the amplifier is very sensitive to parameter variations This behavior is exactly opposite of what was observed earlier when the signal fed back was subtracted from the input What is the difference between the two situations Crudely speaking the former case is called the negative feedback and the latter is the positive feedback The positive feedback increases system gain but tends to make the system more sensitive to parameter variations It can also lead to instability In our example if G were to be 111111 then GH 1 T and the system would become unstable because the signal fed back was exactly equal to the input signal itself since GH 1 Hence once a signal has been applied no matter how small and how short in duration it comes back to reinforce the input undiminished which further passes to the output and is fed back again and again and again In essence the signal perpetuates itself forever This perpetuation even when the input ceases to exist is precisely the symptom of instability Generally speaking a feedback system cannot be described in black and white terms such as positive or negative Usually H is a frequencydependent component more accurately represented by Hs hence it varies with frequency Consequently what was negative feedback at lower frequencies can turn into positive feedback at higher frequencies and may give rise to instability This is one of the serious aspects of feedback systems which warrants a designers careful attention 471 Analysis of a Simple Control System Figure 435a represents an automatic position control system which can be used to control the angular position of a heavy object eg a tracking antenna an antiaircraft gun mount or the position of a ship The input θi is the desired angular position of the object which can be set at any given value The actual angular position θo of the object the output is measured by a potentiometer whose wiper is mounted on the output shaft The difference between the input θi 04LathiC04 2017925 1946 page 411 82 47 Application to Feedback and Controls 411 6 4 2 0 Time seconds 05 1 Amplitude Step Response K7 K16 K80 Figure 436 Step responses for Ex 426 d The unit ramp response is equivalent to the integral of the unit step response We can obtain the ramp response by taking the step response of the system in cascade with an integrator To help highlight waveform detail we compute the ramp response over the short time interval of 0 t 15 t 000115 Hd seriesHctf11 0 stepHdkt titleUnit Ramp Response 05 0 15 1 Time seconds 05 1 15 Amplitude Unit Ramp Response Figure 437 Ramp response for Ex 426 with K 80 DESIGN SPECIFICATIONS Now the reader has some idea of the various specifications a control system might require Generally a control system is designed to meet given transient specifications steadystate error specifications and sensitivity specifications Transient specifications include overshoot rise time and settling time of the response to step input The steadystate error is the difference between 04LathiC04 2017925 1946 page 413 84 48 Frequency Response of an LTIC System 413 ROC for Hs does not include the ω axis where s jω see Eq 410 This means that Hs when s jω is meaningless for BIBOunstable systems Equation 443 shows that for a sinusoidal input of radian frequency ω the system response is also a sinusoid of the same frequency ω The amplitude of the output sinusoid is Hjω times the input amplitude and the phase of the output sinusoid is shifted by Hjω with respect to the input phase see later Fig 438 in Ex 427 For instance a certain system with Hj10 3 and Hj10 30 amplifies a sinusoid of frequency ω 10 by a factor of 3 and delays its phase by 30 The system response to an input 5cos10t 50 is 3 5cos10t 50 30 15cos10t 20 Clearly Hjω is the amplitude gain of the system and a plot of Hjω versus ω shows the amplitude gain as a function of frequency ω We shall call Hjω the amplitude response It also goes under the name magnitude response Similarly Hjω is the phase response and a plot of Hjω versus ω shows how the system modifies or changes the phase of the input sinusoid Plots of the magnitude response Hjω and phase response Hjω show at a glance how a system responds to sinusoids of various frequencies Observe that Hjω has the information of Hjω and Hjω and is therefore termed the frequency response of the system Clearly the frequency response of a system represents its filtering characteristics EXAMPLE 427 Frequency Response Find the frequency response amplitude and phase responses of a system whose transfer function is Hs s 01 s 5 Also find the system response yt if the input xt is a cos 2t b cos10t 50 In this case Hjω jω 01 jω 5 This may also be argued as follows For BIBOunstable systems the zeroinput response contains nondecaying natural mode terms of the form cosω0t or eat cosω0t a 0 Hence the response of such a system to a sinusoid cosωt will contain not just the sinusoid of frequency ω but also nondecaying natural modes rendering the concept of frequency response meaningless Strictly speaking Hω is magnitude response There is a fine distinction between amplitude and magnitude Amplitude A can be positive and negative In contrast the magnitude A is always nonnegative We refrain from relying on this useful distinction between amplitude and magnitude in the interest of avoiding proliferation of essentially similar entities This is also why we shall use the amplitude instead of magnitude spectrum for Hω 04LathiC04 2017925 1946 page 415 86 48 Frequency Response of an LTIC System 415 We also could have read these values directly from the frequency response plots in Fig 438a corresponding to ω 2 This result means that for a sinusoidal input with frequency ω 2 the amplitude gain of the system is 0372 and the phase shift is 653 In other words the output amplitude is 0372 times the input amplitude and the phase of the output is shifted with respect to that of the input by 653 Therefore the system response to the input cos 2t is yt 0372cos2t 653 The input cos 2t and the corresponding system response 0372cos2t 653 are illustrated in Fig 438b b For the input cos10t 50 instead of computing the values Hjω and Hjω as in part a we shall read them directly from the frequency response plots in Fig 438a corresponding to ω 10 These are Hj10 0894 and Hj10 26 Therefore for a sinusoidal input of frequency ω 10 the output sinusoid amplitude is 0894 times the input amplitude and the output sinusoid is shifted with respect to the input sinusoid by 26 Therefore the system response yt to an input cos10t 50 is yt 0894cos10t 50 26 0894cos10t 24 If the input were sin10t 50 the response would be 0894sin10t 50 26 0894 sin10t 24 The frequency response plots in Fig 438a show that the system has highpass filtering characteristics it responds well to sinusoids of higher frequencies ω well above 5 and suppresses sinusoids of lower frequencies ω well below 5 PLOTTING FREQUENCY RESPONSE WITH MATLAB It is simple to use MATLAB to create magnitude and phase response plots Here we consider two methods In the first method we use an anonymous function to define the transfer function Hs and then obtain the frequency response plots by substituting jω for s H s s01s5 omega 00120 subplot121 plotomegaabsH1jomegak subplot122 plotomegaangleH1jomega180pik In the second method we define vectors that contain the numerator and denominator coefficients of Hs and then use the freqs command to compute frequency response B 1 01 A 1 5 H freqsBAomega omega 00120 subplot121 plotomegaabsHk subplot122 plotomegaangleH180pik Both approaches generate plots that match Fig 438a 04LathiC04 2017925 1946 page 416 87 416 CHAPTER 4 CONTINUOUSTIME SYSTEM ANALYSIS EXAMPLE 428 Frequency Responses of Delay Differentiator and Integrator Systems Find and sketch the frequency responses magnitude and phase for a an ideal delay of T seconds b an ideal differentiator and c an ideal integrator a Ideal delay of T seconds The transfer function of an ideal delay is see Eq 430 Hs esT Therefore Hjω ejωT Consequently Hjω 1 and Hjω ωT These amplitude and phase responses are shown in Fig 439a The amplitude response is constant unity for all frequencies The phase shift increases linearly with frequency with a slope of T This result can be explained physically by recognizing that if a sinusoid cosωt is passed through an ideal delay of T seconds the output is cosωt T The output sinusoid amplitude is the same as that of the input for all values of ω Therefore the amplitude response gain is unity for all frequencies Moreover the output cosωt T cosωt ωT has a phase shift ωT with respect to the input cosωt Therefore the phase response is linearly proportional to the frequency ω with a slope T b An ideal differentiator The transfer function of an ideal differentiator is see Eq 431 Hs s Therefore Hjω jω ωejπ2 Consequently Hjω ω and Hjω π 2 These amplitude and phase responses are depicted in Fig 439b The amplitude response increases linearly with frequency and phase response is constant π2 for all frequencies This result can be explained physically by recognizing that if a sinusoid cosωt is passed through an ideal differentiator the output is ωsin ωt ωcosωt π2 Therefore the output sinusoid amplitude is ω times the input amplitude that is the amplitude response gain increases linearly with frequency ω Moreover the output sinusoid undergoes a phase shift π2 with respect to the input cosωt Therefore the phase response is constant π2 with frequency 04LathiC04 2017925 1946 page 418 89 418 CHAPTER 4 CONTINUOUSTIME SYSTEM ANALYSIS with frequency Because its gain is 1ω the ideal integrator suppresses higherfrequency components but enhances lowerfrequency components with ω 1 Consequently noise signals if they do not contain an appreciable amount of verylowfrequency components are suppressed smoothed out by an integrator DRILL 415 Sinusoidal Response of an LTIC System Find the response of an LTIC system specified by d2yt dt2 3dyt dt 2yt dxt dt 5xt if the input is a sinusoid 20sin3t 35 ANSWER 1023sin3t 6191 481 SteadyState Response to Causal Sinusoidal Inputs So far we have discussed the LTIC system response to everlasting sinusoidal inputs starting at t In practice we are more interested in causal sinusoidal inputs sinusoids starting at t 0 Consider the input ejωtut which starts at t 0 rather than at t In this case Xs 1s jω Moreover according to Eq 427 Hs PsQs where Qs is the A puzzling aspect of this result is that in deriving the transfer function of the integrator in Eq 432 we have assumed that the input starts at t 0 In contrast in deriving its frequency response we assume that the everlasting exponential input ejωt starts at t There appears to be a fundamental contradiction between the everlasting input which starts at t and the integrator which opens its gates only at t 0 Of what use is everlasting input since the integrator starts integrating at t 0 The answer is that the integrator gates are always open and integration begins whenever the input starts We restricted the input to start at t 0 in deriving Eq 432 because we were finding the transfer function using the unilateral transform where the inputs begin at t 0 So the integrator starting to integrate at t 0 is restricted because of the limitations of the unilateral transform method not because of the limitations of the integrator itself If we were to find the integrator transfer function using Eq 240 where there is no such restriction on the input we would still find the transfer function of an integrator as 1s Similarly even if we were to use the bilateral Laplace transform where t starts at we would find the transfer function of an integrator to be 1s The transfer function of a system is the property of the system and does not depend on the method used to find it 04LathiC04 2017925 1946 page 422 93 422 CHAPTER 4 CONTINUOUSTIME SYSTEM ANALYSIS We can sketch these four basic terms as functions of ω and use them to construct the logamplitude plot of any desired transfer function Let us discuss each of the terms 491 Constant Ka1a2b1b3 The log amplitude of the constant Ka1a2b1b2 term is also a constant 20logKa1a2b1b3 The phase contribution from this term is zero for positive value and π for negative value of the constant complex constants can have different phases 492 Pole or Zero at the Origin LOG MAGNITUDE A pole at the origin gives rise to the term 20logjω which can be expressed as 20logjω 20logω This function can be plotted as a function of ω However we can effect further simplification by using the logarithmic scale for the variable ω itself Let us define a new variable u such that u logω Hence 20logω 20u The logamplitude function 20u is plotted as a function of u in Fig 440a This is a straight line with a slope of 20 It crosses the u axis at u 0 The ωscale u logω also appears in Fig 440a Semilog graphs can be conveniently used for plotting and we can directly plot ω on semilog paper A ratio of 10 is a decade and a ratio of 2 is known as an octave Furthermore a decade along the ω scale is equivalent to 1 unit along the u scale We can also show that a ratio of 2 an octave along the ω scale equals to 03010 which is log10 2 along the u scale This point can be shown as follows Let ω1 and ω2 along the ω scale correspond to u1 and u2 along the u scale so that logω1 u1 and logω2 u2 Then u2 u1 log10 ω2 log10 ω1 log10ω2ω1 Thus if ω2ω1 10 which is a decade then u2 u1 log10 10 1 and if ω2ω1 2 which is an octave then u2 u1 log10 2 03010 04LathiC04 2017925 1946 page 432 103 432 CHAPTER 4 CONTINUOUSTIME SYSTEM ANALYSIS d The correction at ω 100 because of the corner frequency at ω 100 is 3 dB and the corrections because of the other corner frequencies may be ignored e In addition to the corrections at corner frequencies we may consider corrections at intermediate points for more accurate plots For instance the corrections at ω 4 because of corner frequencies at ω 2 and 10 are 1 and about 065 totaling 165 dB In the same way the corrections at ω 5 because of corner frequencies at ω 2 and 10 are 065 and 1 totaling 165 dB With these corrections the resulting amplitude plot is illustrated in Fig 445a PHASE PLOT We draw the asymptotes corresponding to each of the four factors a The zero at the origin causes a 90 phase shift b The pole at s 2 has an asymptote with a zero value for ω 02 and a slope of 45decade beginning at ω 02 and going up to ω 20 The asymptotic value for ω 20 is 90 c The pole at s 10 has an asymptote with a zero value for ω 1 and a slope of 45decade beginning at ω 1 and going up to ω 100 The asymptotic value for ω 100 is 90 d The zero at s 100 has an asymptote with a zero value for ω 10 and a slope of 45decade beginning at ω 10 and going up to ω 1000 The asymptotic value for ω 1000 is 90 All the asymptotes are added as shown in Fig 445b The appropriate corrections are applied from Fig 442b and the exact phase plot is depicted in Fig 445b EXAMPLE 430 Bode Plots for SecondOrder Transfer Function with Complex Poles Sketch the amplitude and phase response Bode plots for the transfer function Hs 10s 100 s2 2s 100 10 1 s 100 1 s 50 s2 100 MAGNITUDE PLOT Here the constant term is 10 that is 20 dB20 log10 20 To add this term we simply label the horizontal axis from which the asymptotes begin as the 20 dB line as before see Fig 446a 04LathiC04 2017925 1946 page 434 105 434 CHAPTER 4 CONTINUOUSTIME SYSTEM ANALYSIS we have ωn 10 and ζ 01 Step 1 Draw an asymptote of 40 dBdecade 12 dBoctave starting at ω 10 for the complex conjugate poles and draw another asymptote of 20 dBdecade starting at ω 100 for the real zero Step 2 Add both asymptotes Step 3 Apply the correction at ω 100 where the correction because of the corner frequency ω 100 is 3 dB The correction because of the corner frequency ω 10 as seen from Fig 444a for ζ 01 can be safely ignored Next the correction at ω 10 because of the corner frequency ω 10 is 1390 dB see Fig 444a for ζ 01 The correction because of the real zero at 100 can be safely ignored at ω 10 We may find corrections at a few more points The resulting plot is illustrated in Fig 446a PHASE PLOT The asymptote for the complex conjugate poles is a step function with a jump of 180 at ω 10 The asymptote for the zero at s 100 is zero for ω 10 and is a straight line with a slope of 45decade starting at ω 10 and going to ω 1000 For ω 1000 the asymptote is 90 The two asymptotes add to give the sawtooth shown in Fig 446b We now apply the corrections from Figs 442b and 444b to obtain the exact plot 60 40 20 0 20 40 Magnitude dB Bode Diagram 10 0 10 1 10 2 10 3 10 4 Frequency rads 180 135 90 45 0 Phase deg Figure 447 MATLABgenerated Bode plots for Ex 430 04LathiC04 2017925 1946 page 436 107 436 CHAPTER 4 CONTINUOUSTIME SYSTEM ANALYSIS system from the systems response to sinusoids This application has significant practical utility If we are given a system in a black box with only the input and output terminals available the transfer function has to be determined by experimental measurements at the input and output terminals The frequency response to sinusoidal inputs is one of the possibilities that is very attractive because the measurements involved are so simple One needs only to apply a sinusoidal signal at the input and observe the output We find the amplitude gain Hjω and the output phase shift Hjω with respect to the input sinusoid for various values of ω over the entire range from 0 to This information yields the frequency response plots Bode plots when plotted against log ω From these plots we determine the appropriate asymptotes by taking advantage of the fact that the slopes of all asymptotes must be multiples of 20 dBdecade if the transfer function is a rational function function that is a ratio of two polynomials in s From the asymptotes the corner frequencies are obtained Corner frequencies determine the poles and zeros of the transfer function Because of the ambiguity about the location of zeros since LHP and RHP zeros zeros at s a have identical magnitudes this procedure works only for minimum phase systems 410 FILTER DESIGN BY PLACEMENT OF POLES AND ZEROS OF Hs In this section we explore the strong dependence of frequency response on the location of poles and zeros of Hs This dependence points to a simple intuitive procedure to filter design 4101 Dependence of Frequency Response on Poles and Zeros of Hs Frequency response of a system is basically the information about the filtering capability of the system A system transfer function can be expressed as Hs Ps Qs b0 s z1s z2 s zN s λ1s λ2 s λN where z1 z2 zN are λ1 λ2 λN are the poles of Hs Now the value of the transfer function Hs at some frequency s p is Hssp b0 p z1p z2 p zN p λ1p λ2 p λN 453 This equation consists of factors of the form pzi and pλi The factor pzi is a complex number represented by a vector drawn from point z to the point p in the complex plane as illustrated in Fig 448a The length of this line segment is p zi the magnitude of p zi The angle of this directed line segment with the horizontal axis is p zi To compute Hs at s p we draw line segments from all poles and zeros of Hs to the point p as shown in Fig 448b The vector connecting a zero zi to the point p is p zi Let the length of this vector be ri and let its angle with the horizontal axis be φi Then pzi riejφi Similarly the vector connecting a pole λi to the point p is p λi diejθi where di and θi are the length and the angle with the horizontal axis 04LathiC04 2017925 1946 page 439 110 410 Filter Design by Placement of Poles and Zeros of Hs 439 behavior in the vicinity of ω0 This is because the gain in this case is Kdd where d is the distance of a point jω from the conjugate pole α jω0 Because the conjugate pole is far from jω0 there is no dramatic change in the length d as ω varies in the vicinity of ω0 There is a gradual increase in the value of d as ω increases which leaves the frequencyselective behavior as it was originally with only minor changes GAIN SUPPRESSION BY A ZERO Using the same argument we observe that zeros at α jω0 Fig 449d will have exactly the opposite effect of suppressing the gain in the vicinity of ω0 as shown in Fig 449e A zero on the imaginary axis at jω0 will totally suppress the gain zero gain at frequency ω0 Repeated zeros will further enhance the effect Also a closely placed pair of a pole and a zero dipole tend to cancel out each others influence on the frequency response Clearly a proper placement of poles and zeros can yield a variety of frequencyselective behavior We can use these observations to design lowpass highpass bandpass and bandstop or notch filters Phase response can also be computed graphically In Fig 449a angles formed by the complex conjugate poles αjω0 at ω 0 the origin are equal and opposite As ω increases from 0 up the angle θ1 due to the pole α jω0 which has a negative value at ω 0 is reduced in magnitude the angle θ2 because of the pole α jω0 which has a positive value at ω 0 increases in magnitude As a result θ1 θ2 the sum of the two angles increases continuously approaching a value π as ω The resulting phase response Hjω θ1 θ2 is illustrated in Fig 449c Similar arguments apply to zeros at α jω0 The resulting phase response Hjω φ1 φ2 is depicted in Fig 449f We now focus on simple filters using the intuitive insights gained in this discussion The discussion is essentially qualitative 4102 Lowpass Filters A typical lowpass filter has a maximum gain at ω 0 Because a pole enhances the gain at frequencies in its vicinity we need to place a pole or poles on the real axis opposite the origin jω 0 as shown in Fig 450a The transfer function of this system is Hs ωc s ωc We have chosen the numerator of Hs to be ωc to normalize the dc gain H0 to unity If d is the distance from the pole ωc to a point jω Fig 450a then Hjω ωc d with H0 1 As ω increases d increases and Hjω decreases monotonically with ω as illustrated in Fig 450d with label N 1 This is clearly a lowpass filter with gain enhanced in the vicinity of ω 0 WALL OF POLES An ideal lowpass filter characteristic shaded in Fig 450d has a constant gain of unity up to frequency ωc Then the gain drops suddenly to 0 for ω ωc To achieve the ideal lowpass 04LathiC04 2017925 1946 page 448 119 448 CHAPTER 4 CONTINUOUSTIME SYSTEM ANALYSIS Figure 457 Regions of convergence for causal anticausal and combined signals rightsided the poles of Xs lie to the left of the ROC and if xt is anticausal or leftsided the poles of Xs lie to the right of the ROC To prove this generalization we observe that a rightsided signal can be expressed as xt xf t where xt is a causal signal and xf t is some finiteduration signal The ROC of any finiteduration signal is the entire splane no finite poles Hence the ROC of the rightsided signal xt xf t is the region common to the ROCs of xt and xf t which is same as the ROC for xt This proves the generalization for rightsided signals We can use a similar argument to generalize the result for leftsided signals Let us find the bilateral Laplace transform of xt ebtut eatut 458 We already know the Laplace transform of the causal component eatut 1 s a Res a 459 For the anticausal component x2t ebtut we have x2t ebtut 1 s b Res b so that X2s 1 s b 1 s b Res b Therefore ebtut 1 s b Res b 460 04LathiC04 2017925 1946 page 454 125 454 CHAPTER 4 CONTINUOUSTIME SYSTEM ANALYSIS s 2 lies to the right of the ROC and thus represents an anticausal signal Hence yt 1 6etut 1 2etut 2 3e2tut Figure 460c shows yt Note that in this example if xt e4tut e2tut then the ROC of Xs is 4 Res 2 Here no region of convergence exists for XsHs Hence the response yt goes to infinity EXAMPLE 434 Response of a Noncausal System Find the response yt of a noncausal system with the transfer function Hs 1 s 1 Res 1 to the input xt e2tut We have Xs 1 s 2 Res 2 and Ys XsHs 1 s 1s 2 The ROC of XsHs is the region 2 Res 1 By partial fraction expansion Ys 13 s 1 13 s 2 2 Res 1 and yt 1 3etut e2tut Note that the pole of Hs lies in the RHP at 1 Yet the system is not unstable The poles in the RHP may indicate instability or noncausality depending on its location with respect to the region of convergence of Hs For example if Hs 1s1 with Res 1 the system is causal and unstable with ht etut In contrast if Hs 1s 1 with Res 1 the system is noncausal and stable with ht etut 04LathiC04 2017925 1946 page 458 129 458 CHAPTER 4 CONTINUOUSTIME SYSTEM ANALYSIS 0 02 04 06 08 1 12 14 16 18 2 f Hz 104 0 02 04 06 08 1 Hj2 π f Ideal Firstorder RC Figure 462 Magnitude response HRCj2πf of a firstorder RC filter xt R yt R C C Figure 463 A cascaded RC filter A CASCADED RC FILTER AND POLYNOMIAL EXPANSION A firstorder RC filter is destined for poor performance one pole is simply insufficient to obtain good results A cascade of RC circuits increases the number of poles and improves the filter response To simplify the analysis and prevent loading between stages we employ opamp followers to buffer the output of each stage as shown in Fig 463 A cascade of N stages results in an Nthorder filter with transfer function given by Hcascades HRCsN RCs 1N Upon choosing a cascade of 10 stages and C 1 nF a 3 kHz cutoff frequency is obtained by setting R 2110 1Cωc 2110 16π106 R sqrt21101Comegac R 14213e004 This cascaded filter has a 10thorder pole at λ 1RC and no finite zeros To compute the magnitude response polynomial coefficient vectors A and B are needed Setting B 1 ensures there are no finite zeros or equivalently that all zeros are at infinity The poly command which expands a vector of roots into a corresponding vector of polynomial coefficients is used to obtain A 04LathiC04 2017925 1946 page 459 130 412 MATLAB ContinuousTime Filters 459 B 1 A poly1RCones101A AAend Hmagcascade absCH4MP1BAf2pi plotfabsf2piomegackfHmagcascadek axis0 20000 005 105 xlabelf Hz ylabelHj2pi f legendIdealTenthorder RC cascadelocationbest Notice that scaling a polynomial by a constant does not change its roots Conversely the roots of a polynomial specify a polynomial within a scale factor The command A AAend properly scales the denominator polynomial to ensure unity gain at ω 0 The magnitude response plot of the tenthorder RC cascade is shown in Fig 464 Compared with the simple RC response of Fig 462 the passband remains relatively unchanged but stopband attenuation is greatly improved to over 60 dB at 20 kHz 0 02 04 06 08 1 12 14 16 18 2 f Hz 10 4 0 02 04 06 08 1 Hj2 π f Ideal Tenthorder RC cascade Figure 464 Magnitude response Hcascadej2πf of a tenthorder RC cascade 4122 Butterworth Filters and the Find Command The pole location of a firstorder lowpass filter is necessarily fixed by the cutoff frequency There is little reason however to place all the poles of a 10thorder filter at one location Better pole placement will improve our filters magnitude response One strategy discussed in Sec 410 is to place a wall of poles opposite the passband frequencies A semicircular wall of poles leads to the Butterworth family of filters and a semielliptical shape leads to the Chebyshev family of filters Butterworth filters are considered first To begin notice that a transfer function Hs with real coefficients has a squared magnitude response given by Hjω2 HjωHjω HjωHjω HsHssjω Thus half the poles of Hjω2 correspond to the filter Hs and the other half correspond to Hs Filters that are both stable and causal require Hs to include only lefthalfplane poles The squared magnitude response of a Butterworth filter is HBWjω2 1 1 jωjωc2N This function has the same appealing characteristics as the firstorder RC filter a gain that is unity at ω 0 and monotonically decreases to zero as ω By construction the halfpower gain 04LathiC04 2017925 1946 page 460 131 460 CHAPTER 4 CONTINUOUSTIME SYSTEM ANALYSIS 2 15 1 05 0 05 1 15 2 2 15 1 05 0 05 1 15 2 Imaginary 3 104 Real 3 104 Figure 465 Roots of HBWjω2 for N 10 and ωc 30002π occurs at ωc Perhaps most importantly however the first 2N 1 derivatives of HBWjω with respect to ω are zero at ω 0 Put another way the passband is constrained to be very flat for low frequencies For this reason Butterworth filters are sometimes called maximally flat filters As discussed in Sec B7 the roots of minus 1 must lie equally spaced on a circle centered at the origin Thus the 2N poles of HBWjω2 naturally lie equally spaced on a circle of radius ωc centered at the origin Figure 465 displays the 20 poles corresponding to the case N 10 and ωc 30002π rads An Nthorder Butterworth filter that is both causal and stable uses the N lefthalfplane poles of HBWjω2 To design a 10thorder Butterworth filter we first compute the 20 poles of HBWjω2 N10 poles roots1jomegac2Nzeros12N11 The find command is a powerful and useful function that returns the indices of a vectors nonzero elements Combined with relational operators the find command allows us to extract the 10 lefthalfplane roots that correspond to the poles of our Butterworth filter BWpoles polesfindrealpoles0 To compute the magnitude response these roots are converted to coefficient vector A A polyBWpoles A AAend HmagBW absCH4MP1BAf2pi plotfabsf2piomegackfHmagBWk axis0 20000 005 105 xlabelf Hz ylabelHj2pi f legendIdealTenthorder Butterworthlocationbest The magnitude response plot of the Butterworth filter is shown in Fig 466 The Butterworth response closely approximates the brickwall function and provides excellent filter characteristics flat passband rapid transition to the stopband and excellent stopband attenuation 40 dB at 5 kHz 04LathiC04 2017925 1946 page 462 133 462 CHAPTER 4 CONTINUOUSTIME SYSTEM ANALYSIS xt yt R2 C1 R1 C2 Figure 467 SallenKey filter stage provides a measure of the peakedness of the response HighQ filters have poles close to the ω axis which boost the magnitude response near those frequencies Although many ways exist to determine suitable component values a simple method is to assign R1 a realistic value and then let R2 R1 C1 2Qω0R1 and C2 12Qω0R2 Butterworth poles are a distance ωc from the origin so ω0 ωc For our 10thorder Butterworth filter the angles ψ are regularly spaced at 9 27 45 63 and 81 degrees MATLAB program CH4MP2 automates the task of computing component values and magnitude responses for each stage CH4MP2m Chapter 4 MATLAB Program 2 Script Mfile computes SallenKey component values and magnitude responses for each of the five cascaded secondorder filter sections omega0 30002pi Filter cutoff frequency psi 9 27 45 63 81pi180 Butterworth pole angles f linspace06000200 Frequency range for magnitude response calculations HmagSK zeros5200 Preallocate array for magnitude responses for stage 15 Q 12cospsistage Compute Q for current stage Compute and display filter components to the screen dispStage num2strstage Q num2strQ R1 R2 num2str56000 C1 num2str2Qomega056000 C2 num2str12Qomega056000 B omega02 A 1 omega0Q omega02 Compute filter coefficients HmagSKstage absCH4MP1BA2pif Compute magnitude response end plotfHmagSKkfprodHmagSKk xlabelf Hz ylabelMagnitude Response The disp command displays a character string to the screen Character strings must be enclosed in single quotation marks The num2str command converts numbers to character strings and facilitates the formatted display of information The prod command multiplies along the columns of a matrix it computes the total magnitude response as the product of the magnitude responses of the five stages Executing the program produces the following output CH4MP2 Stage 1 Q 050623 R1 R2 56000 C1 95916e10 C2 93569e10 Stage 2 Q 056116 R1 R2 56000 C1 10632e09 C2 8441e10 Stage 3 Q 070711 R1 R2 56000 C1 13398e09 C2 66988e10 Stage 4 Q 11013 R1 R2 56000 C1 20867e09 C2 43009e10 Stage 5 Q 31962 R1 R2 56000 C1 60559e09 C2 1482e10 04LathiC04 2017925 1946 page 463 134 412 MATLAB ContinuousTime Filters 463 0 1000 2000 3000 4000 5000 6000 f Hz 0 05 1 15 2 25 3 35 Magnitude Response Figure 468 Magnitude responses for SallenKey filter stages Since all the component values are practical this filter is possible to implement Figure 468 displays the magnitude responses for all five stages solid lines The total response dotted line confirms a 10thorder Butterworth response Stage 5 which has the largest Q and implements the pair of conjugate poles nearest the ω axis is the most peaked response Stage 1 which has the smallest Q and implements the pair of conjugate poles furthest from the ω axis is the least peaked response In practice it is best to order highQ stages last this reduces the risk that the high gains will saturate the filter hardware 4124 Chebyshev Filters Like an orderN Butterworth lowpass filter LPF an orderN Chebyshev LPF is an allpole filter that possesses many desirable characteristics Compared with an equalorder Butterworth filter the Chebyshev filter achieves better stopband attenuation and reduced transition bandwidth by allowing an adjustable amount of ripple within the passband The squared magnitude response of a Chebyshev filter is HCjω2 1 1 ϵ2C2 Nωωc where ϵ controls the passband ripple CNωωc is a degreeN Chebyshev polynomial and ωc is the radian cutoff frequency Several characteristics of Chebyshev LPFs are noteworthy An orderN Chebyshev LPF is equiripple in the passband ω ωc has a total of N maxima and minima over 0 ω ωc and is monotonic decreasing in the stopband ω ωc 04LathiC04 2017925 1946 page 464 135 464 CHAPTER 4 CONTINUOUSTIME SYSTEM ANALYSIS In the passband the maximum gain is 1 and the minimum gain is 1 1 ϵ2 For oddvalued N Hj0 1 For evenvalued N HCj0 1 1 ϵ2 Ripple is controlled by setting ϵ 10R10 1 where R is the allowable passband ripple expressed in decibels Reducing ϵ adversely affects filter performance see Prob 41210 Unlike Butterworth filters the cutoff frequency ωc rarely specifies the 3 dB point For ϵ 1 HCjωc2 11ϵ2 05 The cutoff frequency ωc simply indicates the frequency after which HCjω 1 1 ϵ2 The Chebyshev polynomial CNx is defined as CNx cosN cos1x coshN cosh1x In this form it is difficult to verify that CNx is a degreeN polynomial in x A recursive form of CNx makes this fact more clear see Prob 41213 CNx 2xCN1x CN2x With C0x 1 and C1x x the recursive form shows that any CN is a linear combination of degreeN polynomials and is therefore a degreeN polynomial itself For N 2 MATLAB program CH4MP3 generates the N 1 coefficients of Chebyshev polynomial CNx function CN CH4MP3N CH4MP3m Chapter 4 MATLAB Program 3 Function Mfile computes Chebyshev polynomial coefficients using the recursion relation CNx 2xCN1x CN2x INPUTS N degree of Chebyshev polynomial OUTPUTS CN vector of Chebyshev polynomial coefficients CNm2 1 CNm1 1 0 Initial polynomial coefficients for t 2N CN 2conv1 0CNm1zeros1lengthCNm1lengthCNm21CNm2 CNm2 CNm1 CNm1 CN end As examples consider C2x 2xC1x C0x 2xx 1 2x2 1 and C3x 2xC2x C1x 2x2x2 1 x 4x3 3x CH4MP3 easily confirms these cases CH4MP32 ans 2 0 1 CH4MP33 ans 4 0 3 0 Since CNωωc is a degreeN polynomial HCjω2 is an allpole rational function with 2N finite poles Similar to the Butterworth case the N poles specifying a causal and stable Chebyshev filter can be found by selecting the N lefthalfplane roots of 1 ϵ2C2 Nsjωc Root locations and dc gain are sufficient to specify a Chebyshev filter for a given N and ϵ To demonstrate consider the design of an order8 Chebyshev filter with cutoff frequency fc 1 kHz and allowable passband ripple R 1 dB First filter parameters are specified 04LathiC04 2017925 1946 page 465 136 412 MATLAB ContinuousTime Filters 465 omegac 2pi1000 R 1 N 8 epsilon sqrt10R101 The coefficients of CNsjωc are obtained with the help of CH4MP3 and then the coefficients of 1 ϵ2C2 Nsjωc are computed by using convolution to perform polynomial multiplication CN CH4MP3N11jomegacN10 CP epsilon2convCNCN CPend CPend1 Next the polynomial roots are found and the lefthalfplane poles are retained and plotted poles rootsCP i findrealpoles0 Cpoles polesi plotrealCpolesimagCpoleskx axis equal axisomegac11 11 11 11 xlabelReal ylabelImaginary As shown in Fig 469 the roots of a Chebyshev filter lie on an ellipse see Prob 41214 5000 0 5000 Real 6000 4000 2000 0 2000 4000 6000 Imaginary Figure 469 Polezero plot for an order8 Chebyshev LPF with fc 1 kHz and R 1 dB To compute the filters magnitude response the poles are expanded into a polynomial the dc gain is set based on the even value of N and CH4MP1 is used A polyCpoles B Aendsqrt1epsilon2 omega linspace02pi20002001 HC CH4MP1BAomega plotomega2piabsHCk axis0 2000 0 11 xlabelf Hz ylabelHCj2pi f E A Guillemin demonstrates a wonderful relationship between the Chebyshev ellipse and the Butterworth circle in his book Synthesis of Passive Networks Wiley New York 1957 04LathiC04 2017925 1946 page 467 138 413 Summary 467 equations Therefore solving these integrodifferential equations reduces to solving algebraic equations The Laplace transform method cannot be used for timevaryingparameter systems or for nonlinear systems in general The transfer function Hs of an LTIC system is the Laplace transform of its impulse response It may also be defined as a ratio of the Laplace transform of the output to the Laplace transform of the input when all initial conditions are zero system in zero state If Xs is the Laplace transform of the input xt and Ys is the Laplace transform of the corresponding output yt when all initial conditions are zero then Ys XsHs For an LTIC system described by an Nthorder differential equation QDyt PDxt the transfer function Hs PsQs Like the impulse response ht the transfer function Hs is also an external description of the system Electrical circuit analysis can also be carried out by using a transformed circuit method in which all signals voltages and currents are represented by their Laplace transforms all elements by their impedances or admittances and initial conditions by their equivalent sources initial condition generators In this method a network can be analyzed as if it were a resistive circuit Large systems can be depicted by suitably interconnected subsystems represented by blocks Each subsystem being a smaller system can be readily analyzed and represented by its inputoutput relationship such as its transfer function Analysis of large systems can be carried out with the knowledge of inputoutput relationships of its subsystems and the nature of interconnection of various subsystems LTIC systems can be realized by scalar multipliers adders and integrators A given transfer function can be synthesized in many different ways such as canonic cascade and parallel Moreover every realization has a transpose which also has the same transfer function In practice all the building blocks scalar multipliers adders and integrators can be obtained from operational amplifiers The system response to an everlasting exponential est is also an everlasting exponential Hsest Consequently the system response to an everlasting exponential ejωt is Hjωejωt Hence Hjω is the frequency response of the system For a sinusoidal input of unit amplitude and having frequency ω the system response is also a sinusoid of the same frequency ω with amplitude Hjω and its phase is shifted by Hjω with respect to the input sinusoid For this reason Hjω is called the amplitude response gain and Hjω is called the phase response of the system Amplitude and phase response of a system indicate the filtering characteristics of the system The general nature of the filtering characteristics of a system can be quickly determined from a knowledge of the location of poles and zeros of the system transfer function Most of the input signals and practical systems are causal Consequently we are required most of the time to deal with causal signals When all signals must be causal the Laplace transform analysis is greatly simplified the region of convergence of a signal becomes irrelevant to the analysis process This special case of the Laplace transform which is restricted to causal signals is called the unilateral Laplace transform Much of the chapter deals with this variety of Laplace transform Section 411 discusses the general Laplace transform the bilateral Laplace transform which can handle causal and noncausal signals and systems In the bilateral transform the inverse transform of Xs is not unique but depends on the region of convergence of Xs Thus the region of convergence plays a very crucial role in the bilateral Laplace transform 04LathiC04 2017925 1946 page 470 141 470 CHAPTER 4 CONTINUOUSTIME SYSTEM ANALYSIS t t t xt a gt b T0 2T0 3T0 pt c 1 2 8 10 16 18 24 Figure P429 4211 a Find the Laplace transform of the pulses in Fig 42 by using only the timedifferent iation property the timeshifting property and the fact that δt 1 b In Ex 49 the Laplace transform of xt is found by finding the Laplace transform of d2xdt2 Find the Laplace transform of xt in that example by finding the Laplace transform of dxdt and using Table 41 if necessary 4212 Determine the inverse unilateral Laplace trans form of Xs 1 es3 s2 s 1s 2 4213 Since 13 is such a lucky number determine the inverse Laplace transform of Xs 1s 113 given region of convergence σ 1 Hint What is the nth derivative of 1s a 4214 It is difficult to compute the Laplace transform Xs of signal xt 1 t ut by using direct integration Instead properties provide a simpler method a Use Laplace transform properties to express the Laplace transform of txt in terms of the unknown quantity Xs b Use the definition to determine the Laplace transform of yt txt c Solve for Xs by using the two pieces from a and b Simplify your answer 431 Use the Laplace transform to solve the following differential equations a D2 3D 2yt Dxt if y0 y0 0 and xt ut b D2 4D 4yt D 1xt if y0 2 y0 1 and xt etut c D2 6D25yt D2xt if y0 y0 1 and xt 25ut 432 Solve the differential equations in Prob 431 using the Laplace transform In each case deter mine the zeroinput and zerostate components of the solution 433 Consider a causal LTIC system described by the differential equation 2yt 6yt xt 4xt a Using transformdomain techniques deter mine the ZIR yzirt if y0 3 b Using transformdomain techniques deter mine the ZSR yzsrt to the input xt eδt π 434 Consider a causal LTIC system described by the differential equation yt 3yt 2yt 2xt xt 04LathiC04 2017925 1946 page 478 149 478 CHAPTER 4 CONTINUOUSTIME SYSTEM ANALYSIS Fig P454b consider three cases a K 10 b K 50 and c K 48 461 Realize Hs ss 2 s 1s 3s 4 by canonic direct series and parallel forms 462 Realize the transfer function in Prob 461 by using the transposed form of the realizations found in Prob 461 463 Repeat Prob 461 for a Hs 3ss 2 s 1s2 2s 2 b Hs 2s 4 s 2s2 4 464 Realize the transfer functions in Prob 463 by using the transposed form of the realizations found in Prob 463 465 Repeat Prob 461 for Hs 2s 3 5ss 22s 3 466 Realize the transfer function in Prob 465 by using the transposed form of the realizations found in Prob 465 467 Repeat Prob 461 for Hs ss 1s 2 s 5s 6s 8 468 Realize the transfer function in Prob 467 by using the transposed form of the realizations found in Prob 467 469 Repeat Prob 461 for Hs s3 s 12s 2s 3 4610 Realize the transfer function in Prob 469 by using the transposed form of the realizations found in Prob 469 4611 Repeat Prob 461 for Hs s3 s 1s2 4s 13 4612 Realize the transfer function in Prob 4611 by using the transposed form of the realizations found in Prob 4611 4613 Draw a TDFII block realization of a causal LTIC system with transfer function Hs s2js2j sjsjs2 Give two reasons why TDFII tends to be a good structure 4614 Consider a causal LTIC system with transfer function Hs s2js2js3js3j 9s1s2s1js1j a Realize Hs using a single fourthorder real TDFII structure Is this block realization unique Explain b Realize Hs using a cascade of second order real DFII structures Is this block realization unique Explain c Realize Hs using a parallel connection of secondorder real DFI structures Is this block realization unique Explain 4615 In this problem we show how a pair of complex conjugate poles may be realized by using a cascade of two firstorder transfer functions and feedback Show that the transfer functions of the block diagrams in Figs P4615a and P4615b are a Has 1 s a2 b2 1 s2 2as a2 b2 b Hbs s a s a2 b2 s a s2 2as a2 b2 Hence show that the transfer function of the block diagram in Fig P4615c is c Hcs As B s a2 b2 As B s2 2as a2 b2 4616 Show opamp realizations of the following transfer functions a 10 s 5 b 10 s 5 c s 2 s 5 04LathiC04 2017925 1946 page 480 151 480 CHAPTER 4 CONTINUOUSTIME SYSTEM ANALYSIS c To decrease the bandwidth of this system we use positive feedback with Hs 09 as illustrated in Fig P471c Show that the 3 dB bandwidth of this system is ωc10 What is the dc gain d The system gain at dc times its 3 dB bandwidth is the gainbandwidth product of a system Show that this product is the same for all the three systems in Fig P471 This result shows that if we increase the bandwidth the gain decreases and vice versa 481 Suppose an engineer builds a controllable observable LTIC system with transfer function Hs s24 2s24s4 a By direct calculation compute the magni tude response at frequencies ω 0 1 2 3 5 10 and Use these calculations to roughly sketch the magnitude response over 0 ω 10 b To test the system the engineer connects a signal generator to the system in hopes to measure the magnitude response using a standard oscilloscope What type of signal should the engineer input into the system to make the measurements How should the engineer make the measurements Pro vide sufficient detail to fully justify your answers c Suppose the engineer accidentally con structs the system H1s 1 Hs 2s24s4 s24 What impact will this mistake have on his tests 482 For an LTIC system described by the transfer function Hs s 2 s2 5s 4 find the response to the following everlasting sinusoidal inputs a 5cos2t 30 b 10sin2t 45 c 10cos3t 40 Observe that these are everlasting sinusoids 483 For an LTIC system described by the transfer function Hs s 3 s 22 find the steadystate system response to the following inputs a 10ut b cos2t 60ut c sin3t 45ut d ej3tut 484 For an allpass filter specified by the transfer function Hs s 10 s 10 find the system response to the following ever lasting inputs a ejωt b cosωt θ c cos t d sin 2t e cos 10t f cos 100t Comment on the filter response 485 The polezero plot of a secondorder system Hs is shown in Fig P485 The dc response of this system is minus 1 Hj0 1 a Letting Hs ks2 b1s b2s2 a1s a2 determine the constants k b1 b2 a1 and a2 b What is the output yt of this system in response to the input xt 4 cost2 π3 2 15 1 05 0 s v 05 1 15 2 2 15 1 05 0 05 1 15 2 Figure P485 486 Consider a CT system described by D1D 2yt xt 1 Notice that this differential equation is in terms of xt 1 not xt a Determine the output yt given input xt 1 04LathiC04 2017925 1946 page 481 152 Problems 481 b Determine the output yt given input xt cost 487 An LTIC system has transfer function Hs 4s s22s37 4s s16js16j Determine the steadystate output in response to input xt 1 3ej6tπ3u6t π3 491 Suppose a real firstorder lowpass system Hs has unity gain in the passband one finite pole at s 2 and one finite zero at an unspecified location a Determine the location of the system zero so that the filter achieves 40 dB of stop band attenuation Sketch the corresponding straightline Bode approximation of the system magnitude response b Determine the location of the system zero so that the filter achieves 30 dB of stop band attenuation Sketch the corresponding straightline Bode approximation of the system magnitude response 492 Repeat Prob 491 for a highpass rather than a lowpass system 493 Repeat Prob 491 for a secondorder system that has a pair of repeated poles and a pair of repeated zeros 494 Sketch Bode plots for the following transfer functions a ss 100 s 2s 20 b s 10s 20 s2s 100 c s 10s 200 s 202s 1000 495 Repeat Prob 494 for a s2 s 1s2 4s 16 b s s 1s2 1414s 100 c s 10 ss2 1414s 100 496 Using the lowest order possible determine a system function Hs with realvalued roots that matches the frequency response in Fig P496 Verify your answer with MATLAB 497 A graduate student recently implemented an analog phase lock loop PLL as part of his the sis His PLL consists of four basic components a phasefrequency detector a charge pump a loop filter and a voltagecontrolled oscillator This problem considers only the loop filter which is shown in Fig P497a The loop filter input is the current xt and the output is the voltage yt a Derive the loop filters transfer function Hs Express Hs in standard form b Figure P497b provides four possible fre quency response plots labeled A through D Each loglog plot is drawn to the same scale and line slopes are either 20 dBdecade 0 dBdecade or 20 dBdecade Clearly identify which plots if any could repre sent the loop filter c Holding the other components constant what is the general effect of increasing the resistance R on the magnitude response for lowfrequency inputs 101 100 101 102 103 104 10 5 0 5 10 15 20 25 30 35 40 v rads Hjv dB Bode approximation True H jv Figure P496 04LathiC04 2017925 1946 page 482 153 482 CHAPTER 4 CONTINUOUSTIME SYSTEM ANALYSIS d Holding the other components constant what is the general effect of increasing the resistance R on the magnitude response for highfrequency inputs A B C D b xt yt a R C1 C2 Figure P497 4101 A causal LTIC system Hs 2s4js4j s12js12j has input xt 1 2cos2t 3sin4t π3 4cos10t Below perform accurate cal culations at ω 0 2 4 and 10 a Using the graphical method of Sec 4101 accurately sketch the magnitude response Hjω over 10 ω 10 b Using the graphical method of Sec 4101 accurately sketch the phase response Hjω over 10 ω 10 c Approximate the system output yt in response to the input xt 4102 The polezero plot of a secondorder system Hs is shown in Fig P4102 The dc response of this system is minus 2 Hj0 2 a Letting Hs k s2b1sb2 s2a1sa2 determine the constants k b1 b2 a1 and a2 b Using the graphical method of Sec 4101 handsketch the magnitude response Hjω over 10 ω 10 Verify your sketch with MATLAB c Using the graphical method of Sec 4101 handsketch the phase response Hjω over 10 ω 10 Verify your sketch with MATLAB d What is the output yt in response to input xt 3 cos3tπ3 sin4tπ8 Re Im 1 4 3 3 4 Figure P4102 4103 Using the graphical method of Sec 4101 draw a rough sketch of the amplitude and phase responses of an LTIC system described by the transfer function Hs s2 2s 50 s2 2s 50 s 1 j7s 1 j7 s 1 j7s 1 j7 What kind of filter is this 4104 Using the graphical method of Sec 4101 draw a rough sketch of the amplitude and phase responses of LTIC systems whose polezero plots are shown in Fig P4104 04LathiC04 2017925 1946 page 486 157 486 CHAPTER 4 CONTINUOUSTIME SYSTEM ANALYSIS xt R C R R10 C R R yt R2 R26 Figure P4123 xt yt R2 C1 R1 C2 Figure P4124 4124 Design an order12 Butterworth lowpass filter with a cutoff frequency of ωc 2π5000 by completing the following a Locate and plot the filters poles and zeros in the complex plane Plot the correspond ing magnitude response HLPjω to verify proper design b Setting all resistor values to 100000 deter mine the capacitor values to implement the filter using a cascade of six secondorder SallenKey circuit sections The form of a SallenKey stage is shown in Fig P4124 On a single plot plot the magnitude response of each section as well as the over all magnitude response Identify the poles that correspond to each sections magnitude response curve Are the capacitor values realistic 4125 Rather than a Butterworth filter repeat Prob 4124 for a Chebyshev LPF with R 3 dB of passband ripple Since each SallenKey stage is constrained to have unity gain at dc an overall gain error of 1 1 ϵ2 is acceptable 4126 An analog lowpass filter with cutoff frequency ωc can be transformed into a highpass filter with cutoff frequency ωc by using an RCCR transformation rule each resistor Ri is replaced by a capacitor C i 1Riωc and each capacitor Ci is replaced by a resistor R i 1Ciωc Use this rule to design an order8 Butterworth highpass filter with ωc 2π4000 by completing the following a Design an order8 Butterworth lowpass filter with ωc 2π4000 by using four secondorder SallenKey circuit stages the form of which is shown in Fig P4124 Give resistor and capacitor values for each stage Choose the resistors so that the RCCR transformation will result in 1 nF capacitors At this point are the component values realistic b Draw an RCCR transformed SallenKey circuit stage Determine the transfer func tion Hs of the transformed stage in terms of the variables R 1 R 2 C 1 and C 2 c Transform the LPF designed in part a by using an RCCR transformation Give the resistor and capacitor values for each stage Are the component values realistic Using Hs derived in part b plot the magnitude response of each section as well 04LathiC04 2017925 1946 page 487 158 Problems 487 as the overall magnitude response Does the overall response look like a highpass Butterworth filter Plot the HPF system poles and zeros in the complex s plane How do these locations compare with those of the Butterworth LPF 4127 Repeat Prob 4126 using ωc 2π1500 and an order16 filter That is eight secondorder stages need to be designed 4128 Rather than a Butterworth filter repeat Prob 4126 for a Chebyshev LPF with R 3 dB of passband ripple Since each transformed SallenKey stage is constrained to have unity gain at ω an overall gain error of 1 1 ϵ2 is acceptable 4129 The MATLAB signalprocessing toolbox func tion butter helps design analog Butterworth filters Use MATLAB help to learn how butter works For each of the following cases design the filter plot the filters poles and zeros in the complex s plane and plot the decibel magnitude response 20log10 Hjω a Design a sixthorder analog lowpass filter with ωc 2π3500 b Design a sixthorder analog highpass filter with ωc 2π3500 c Design a sixthorder analog bandpass filter with a passband between 2 and 4 kHz d Design a sixthorder analog bandstop filter with a stopband between 2 and 4 kHz 41210 The MATLAB signalprocessing toolbox func tion cheby1 helps design analog Chebyshev type I filters A Chebyshev type I filter has a passband ripple and a smooth stopband Set ting the passband ripple to Rp 3 dB repeat Prob 4129 using the cheby1 command With all other parameters held constant what is the general effect of reducing Rp the allowable passband ripple 41211 The MATLAB signalprocessing toolbox func tion cheby2 helps design analog Chebyshev type II filters A Chebyshev type II filter has a smooth passband and ripple in the stopband Setting the stopband ripple Rs 20 dB down repeat Prob 4129 using the cheby2 command With all other parameters held constant what is the general effect of increasing Rs the minimum stopband attenuation 41212 The MATLAB signalprocessing toolbox func tion ellip helps design analog elliptic filters An elliptic filter has ripple in both the passband and the stopband Setting the passband ripple to Rp 3 dB and the stopband ripple Rs 20 dB down repeat Prob 4129 using the ellip command 41213 Using the definition CNx coshN cosh1x prove the recursive relation CNx 2xCN1x CN2x 41214 Prove that the poles of a Chebyshev filter which are located at pk ωc sinhξsinφk jωc coshξcosφk lie on an ellipse Hint The equation of an ellipse in the xy plane is xa2 yb2 1 where constants a and b define the major and minor axes of the ellipse 05LathiC05 2017925 1554 page 495 8 51 The zTransform 495 ANSWERS a Xz z5 z4 z3 z2 z 1 z9 or z z 1z4 z10 b z32z 172 z2 2z 2 n 1 4 5 6 7 8 9 0 xn Figure 53 Signal for Drill 51a 511 Inverse Transform by Partial Fraction Expansion and Tables As in the Laplace transform we shall avoid the integration in the complex plane required to find the inverse ztransform Eq 52 by using the unilateral transform table Table 51 Many of the transforms Xz of practical interest are rational functions ratio of polynomials in z which can be expressed as a sum of partial fractions whose inverse transforms can be readily found in a table of transform The partial fraction method works because for every transformable xn defined for n 0 there is a corresponding unique Xz defined for z r0 where r0 is some constant and vice versa EXAMPLE 53 Inverse zTransform by Partial Fraction Expansion Find the inverse ztransforms of a 8z 19 z 2z 3 b z2z2 11z 12 z 1z 23 c 2z3z 17 z 1z2 6z 25 a Expanding Xz into partial fractions yields Xz 8z 19 z 2z 3 3 z 2 5 z 3 05LathiC05 2017925 1554 page 510 23 510 CHAPTER 5 DISCRETETIME SYSTEM ANALYSIS USING THE ZTRANSFORM 53 zTRANSFORM SOLUTION OF LINEAR DIFFERENCE EQUATIONS The timeshifting leftshift or rightshift property has set the stage for solving linear difference equations with constant coefficients As in the case of the Laplace transform with differential equations the ztransform converts difference equations into algebraic equations that are readily solved to find the solution in the z domain Taking the inverse ztransform of the zdomain solution yields the desired timedomain solution The following examples demonstrate the procedure EXAMPLE 55 zTransform Solution of a Linear Difference Equation Solve yn 2 5yn 1 6yn 3xn 1 5xn if the initial conditions are y1 116 y2 3736 and the input xn 2nun As we shall see difference equations can be solved by using the rightshift or the left shift property Because the difference equation here is in advance form the use of the leftshift property in Eq 516 may seem appropriate for its solution Unfortunately this leftshift property requires a knowledge of auxiliary conditions y0 y1 yN 1 rather than of the initial conditions y1 y2 yn which are generally given This difficulty can be overcome by expressing the difference equation in delay form obtained by replacing n with n 2 and then using the rightshift property The resulting delayform difference equation is yn 5yn 1 6yn 2 3xn 1 5xn 2 521 We now use the rightshift property to take the ztransform of this equation But before proceeding we must be clear about the meaning of a term like yn 1 here Does it mean yn 1un 1 or yn 1un In any equation we must have some time reference n 0 and every term is referenced from this instant Hence ynk means ynkun Remember also that although we are considering the situation for n 0 yn is present even before n 0 in the form of initial conditions Now ynun Yz yn 1un 1 z Yz y1 1 z Yz 11 6 yn 2un 1 z2 Yz 1 z y1 y2 1 z2 Yz 11 6z 37 36 Noting that for causal input xn x1 x2 xn 0 Another approach is to find y0 y1 y2 yN 1 from y1 y2 yn iteratively as in Sec 351 and then apply the leftshift property to the advanceform difference equation 05LathiC05 2017925 1554 page 516 29 516 CHAPTER 5 DISCRETETIME SYSTEM ANALYSIS USING THE ZTRANSFORM Figure 56 The transformed representation of an LTID system representing all signals by their ztransforms and all system components or elements by their transfer functions as shown in Fig 56b The result Yz HzXz greatly facilitates derivation of the system response to a given input We shall demonstrate this assertion by an example EXAMPLE 56 Transfer Function to Find the ZeroState Response Find the response yn of an LTID system described by the difference equation yn 2 yn 1 016yn xn 1 032xn or E2 E 016yn E 032xn for the input xn 2nun and with all the initial conditions zero system in the zero state From the difference equation we find Hz Pz Qz z 032 z2 z 016 For the input xn 2nun 21nun 05nun Xz z z 05 and Yz XzHz zz 032 z2 z 016z 05 05LathiC05 2017925 1554 page 519 32 54 System Realization 519 3 An LTID system is marginally stable if and only if there are no poles of Hz outside the unit circle and there are some simple poles on the unit circle DRILL 514 Transfer Function to Determine Stability Show that an accumulator whose impulse response is hn un is marginally stable but BIBOunstable 533 Inverse Systems If Hz is the transfer function of a system S then Si its inverse system has a transfer function Hiz given by Hiz 1 Hz This follows from the fact the inverse system Si undoes the operation of S Hence if Hz is placed in cascade with Hiz the transfer function of the composite system identity system is unity For example an accumulator whose transfer function is Hz zz 1 and a backward difference system whose transfer function is Hiz z 1z are inverse of each other Similarly if Hz z 04 z 07 its inverse system transfer function is Hiz z 07 z 04 as required by the property HzHiz 1 Hence it follows that hn hin δn DRILL 515 Inverse Systems Find the impulse responses of an accumulator and a firstorder backward difference system Show that the convolution of the two impulse responses yields δn 54 SYSTEM REALIZATION Because of the similarity between LTIC and LTID systems conventions for block diagrams and rules of interconnection for LTID are identical to those for continuoustime LTIC systems It is not necessary to rederive these relationships We shall merely restate them to refresh the readers memory 05LathiC05 2017925 1554 page 521 34 54 System Realization 521 system is H1z H2z For a feedback system as in Fig 418d the transfer function is Gz1 GzHz We now consider a systematic method for realization or simulation of an arbitrary Nthorder LTID transfer function Since realization is basically a synthesis problem there is no unique way of realizing a system A given transfer function can be realized in many different ways We present here the two forms of direct realization Each of these forms can be executed in several other ways such as cascade and parallel Furthermore a system can be realized by the transposed version of any known realization of that system This artifice doubles the number of system realizations A transfer function Hz can be realized by using time delays along with adders and multipliers We shall consider a realization of a general Nthorder causal LTID system whose transfer function is given by Hz b0zN b1zN1 bN1z bN zN a1zN1 aN1z aN 529 This equation is identical to the transfer function of a general Nthorder proper LTIC system given in Eq 436 The only difference is that the variable z in the former is replaced by the variable s in the latter Hence the procedure for realizing an LTID transfer function is identical to that for the LTIC transfer function with the basic element 1s integrator replaced by the element 1z unit delay The reader is encouraged to follow the steps in Sec 46 and rederive the results for the LTID transfer function in Eq 529 Here we shall merely reproduce the realizations from Sec 46 with integrators 1s replaced by unit delays 1z The direct form I DFI is shown in Fig 58a the canonic direct form DFII is shown in Fig 58b and the transpose of canonic direct is shown in Fig 58c The DFII and its transpose are canonic because they require N delays which is the minimum number needed to implement the Nthorder LTID transfer function in Eq 529 In contrast the form DFI is a noncanonic because it generally requires 2N delays The DFII realization in Fig 58b is also called a canonic direct form EXAMPLE 58 Canonical Realizations of Transfer Functions Find the canonic direct and the transposed canonic direct realizations of the following transfer functions a 2 z 5 b 4z 28 z 1 c z z 7 and d 4z 28 z2 6z 5 All four of these transfer functions are special cases of Hz in Eq 529 a Hz 2 z 5 For this case the transfer function is of the first order N 1 therefore we need only one delay for its realization The feedback and feedforward coefficients are a1 5 and b0 0 b1 2 05LathiC05 2017925 1554 page 547 60 57 Digital Processing of Analog Signals 547 DRILL 521 Highpass Filter by PoleZero Placement Use the graphical argument to show that a filter with transfer function Hz z 09 z acts like a highpass filter Make a rough sketch of the amplitude response 57 DIGITAL PROCESSING OF ANALOG SIGNALS An analog meaning continuoustime signal can be processed digitally by sampling the analog signal and processing the samples by a digital meaning discretetime processor The output of the processor is then converted back to analog signal as shown in Fig 524a We saw some simple cases of such processing in Exs 38 39 514 and 515 In this section we shall derive a criterion for designing such a digital processor for a general LTIC system Suppose that we wish to realize an equivalent of an analog system with transfer function Has shown in Fig 524b Let the digital processor transfer function in Fig 524a that realizes this desired Has be Hz In other words we wish to make the two systems in Fig 524 equivalent at least approximately By equivalence we mean that for a given input xt the systems in Fig 524 yield the same output yt Therefore ynT the samples of the output in Fig 524b are identical to yn the output of Hz in Fig 524a xn xt yn yt xt yt Continuous to discrete CD Discretetime system Hz Discrete to continuous DC a a b Has b Has Figure 524 Analog filter realization with a digital filter 05LathiC05 2017925 1554 page 573 86 510 MATLAB DiscreteTime IIR Filters 573 1 05 0 05 1 Real 1 05 0 05 1 Imag Figure 532 Polezero plot computed by using roots CH5MP5m Chapter 5 MATLAB Program 5 Script Mfile designs a 180thorder Butterworth lowpass discretetime filter with cutoff Omegac 06pi using 90 cascaded secondorder filter sections omega0 1 Use normalized cutoff frequency for analog prototype psi 05190pi180 Butterworth pole angles Omegac 06pi Discretetime cutoff frequency Omega linspace0pi1000 Frequency range for magnitude response Hmag zeros901000 p zeros1180 z zeros1180 Preallocation for stage 190 Q 12cospsistage Compute Q for stage B omega02 A 1 omega0Q omega02 Compute stage coefficients B1A1 CH5MP4BA2omega0tan06pi2 Transform stage to DT pstage21stage2 rootsA1 Compute zdomain poles for stage zstage21stage2 rootsB1 Compute zdomain zeros for stage Hmagstage absCH5MP1B1A1Omega Compute stage mag response end ucirc expjlinspace02pi200 Compute unit circle for polezero plot figure plotrealpimagpkxrealzimagzokrealucircimagucirck axis equal xlabelReal ylabelImag figure plotOmegaprodHmagk axis0 pi 005 105 xlabelOmega rad ylabelMagnitude Response The figure command preceding each plot command opens a separate window for each plot The filters polezero plot is shown in Fig 533 along with the unit circle for reference All 180 zeros of the cascaded design are properly located at minus 1 The wall of poles provides an amazing approximation to the desired brickwall response as shown by the magnitude response in Fig 534 It is virtually impossible to realize such highorder designs with continuoustime filters which adds another reason for the popularity of discretetime filters Still the design is not 05LathiC05 2017925 1554 page 574 87 574 CHAPTER 5 DISCRETETIME SYSTEM ANALYSIS USING THE ZTRANSFORM 1 05 0 05 1 Real 08 06 04 02 0 02 04 06 08 Imag Figure 533 Polezero plot for 180thorder discretetime Butterworth filter 0 05 1 15 2 25 3 Ω rad 0 02 04 06 08 1 Magnitude Response Figure 534 Magnitude response for a 180thorder discretetime Butterworth filter trivial even functions from the MATLAB signalprocessing toolbox fail to properly design such a highorder discretetime Butterworth filter 511 SUMMARY In this chapter we discussed the analysis of linear timeinvariant discretetime LTID systems by means of the ztransform The ztransform changes the difference equations of LTID systems into algebraic equations Therefore solving these difference equations reduces to solving algebraic equations The transfer function Hz of an LTID system is equal to the ratio of the ztransform of the output to the ztransform of the input when all initial conditions are zero Therefore if Xz is the ztransform of the input xn and Yz is the ztransform of the corresponding output yn 05LathiC05 2017925 1554 page 575 88 Problems 575 when all initial conditions are zero then Yz HzXz For an LTID system specified by the difference equation QEyn PExn the transfer function Hz PzQz Moreover Hz is the ztransform of the system impulse response hn We showed in Ch 3 that the system response to an everlasting exponential zn is Hzzn We may also view the ztransform as a tool that expresses a signal xn as a sum of exponentials of the form zn over a continuum of the values of z Using the fact that an LTID system response to zn is Hzzn we find the system response to xn as a sum of the systems responses to all the components of the form zn over the continuum of values of z LTID systems can be realized by scalar multipliers adders and time delays A given transfer function can be synthesized in many different ways We discussed canonical transposed canonical cascade and parallel forms of realization The realization procedure is identical to that for continuoustime systems with 1s integrator replaced by 1z unit delay The majority of the input signals and practical systems are causal Consequently we are required to deal with causal signals most of the time Restricting all signals to the causal type greatly simplifies ztransform analysis the ROC of a signal becomes irrelevant to the analysis process This special case of ztransform which is restricted to causal signals is called the unilateral ztransform Much of the chapter deals with this transform Section 58 discusses the general variety of the ztransform bilateral ztransform which can handle causal and noncausal signals and systems In the bilateral transform the inverse transform of Xz is not unique but depends on the ROC of Xz Thus the ROC plays a crucial role in the bilateral ztransform In Sec 59 we showed that discretetime systems can be analyzed by the Laplace transform as if they were continuoustime systems In fact we showed that the ztransform is the Laplace transform with a change in variable REFERENCES 1 Lyons R G Understanding Digital Signal Processing AddisonWesley Reading MA 1997 2 Oppenheim A V and R W Schafer DiscreteTime Signal Processing 2nd ed PrenticeHall Upper Saddle River NJ 1999 3 Mitra S K Digital Signal Processing 2nd ed McGrawHill New York 2001 PROBLEMS 511 Using the definition compute the ztransform of xn 1nun un 8 Sketch the poles and zeros of Xz in the z plane No calculator is needed to do this problem 512 Determine the unilateral ztransform Xz of the signal xn shown in Fig P512 As the picture suggests xn 3 for all n 9 and xn 0 for all n 3 513 a A causal signal has ztransform given by Xz z2 z31 Determine the timedomain signal xn and sketch xn over 4 n 11 Hint No complex arithmetic is needed to solve this problem yn n 3 1 1 3 15 10 5 5 Figure P512 05LathiC05 2017925 1554 page 579 92 Problems 579 a Use transformdomain techniques to determine the zerostate response yzsrn to input xn 3un 5 b Use transformdomain techniques to determine the zeroinput response yzirn given yzir2 yzir1 1 537 a Find the output yn of an LTID system specified by the equation 2yn 2 3yn 1 yn 4xn 2 3xn 1 for input xn 4nun and initial conditions y1 0 and y2 1 b Find the zeroinput and the zerostate components of the response c Find the transient and the steadystate components of the response 538 Solve Prob 537 if initial conditions y1 and y2 are instead replaced with auxiliary conditions y0 32 and y1 354 539 a Solve 4yn 2 4yn 1 yn xn 1 with y1 0 y2 1 and xn un b Find the zeroinput and the zerostate components of the response c Find the transient and the steadystate components of the response 5310 Solve yn 2 3yn 1 2yn xn 1 if y1 2 y2 3 and xn 3nun 5311 Solve yn 2 2yn 1 2yn xn with y1 1 y2 0 and xn un 5312 Consider a causal LTID system described as Hz 21z21 16z2 1 4 z 3 8 a Determine the standard delayform differ ence equation description of this system b Using transformdomain techniques deter mine the system impulse response hn c Using transformdomain techniques determine yzirn given y1 16 and y2 8 5313 Consider a causal LTID system described as yn 5 6yn 1 1 6yn 2 3 2xn 1 3 2xn 2 a Determine the standardform system transfer function Hz and sketch the system polezero plot b Using transformdomain techniques determine yzirn given y1 2 and y2 2 5314 Solve yn2yn12yn2 xn12xn2 with y0 0 y1 1 and xn enun 5315 A system with impulse response hn 213nun 1 produces an output yn 2nun 1 Determine the corresponding input xn 5316 A professor recently received an unexpected 10 a futile bribe attached to a test Being the savvy investor that she is the professor decides to invest the 10 into a savings account that earns 05 interest compounded monthly 617 APY Furthermore she decides to supplement this initial investment with an additional 5 deposit made every month beginning the month immediately following her initial investment a Model the professors savings account as a constant coefficient linear difference equation Designate yn as the account balance at month n where n 0 corresponds to the first month that interest is awarded and that her 5 deposits begin b Determine a closedform solution for yn That is you should express yn as a function only of n c If we consider the professors bank account as a system what is the system impulse response hn What is the system transfer function Hz d Explain this fact if the input to the professors bank account is the everlasting exponential xn 1n 1 then the output is not yn 1nH1 H1 5317 Sally deposits 100 into her savings account on the first day of every month except for each December when she uses her money to buy 05LathiC05 2017925 1554 page 592 105 592 CHAPTER 5 DISCRETETIME SYSTEM ANALYSIS USING THE ZTRANSFORM type I filters A Chebyshev type I filter has passband ripple and smooth stopband Setting the passband ripple to Rp 3 dB repeat Prob 51012 using the cheby1 command With all other parameters held constant what is the general effect of reducing Rp the allowable passband ripple 51014 The MATLAB signalprocessing toolbox function cheby2 helps design digital Chebyshev type II filters A Chebyshev type II filter has smooth passband and ripple in the stopband Setting the stopband ripple Rs 20 dB down repeat Prob 51012 using the cheby2 command With all other parameters held constant what is the general effect of increasing Rs the minimum stopband attenuation 51015 The MATLAB signalprocessing toolbox function ellip helps design digital elliptic filters An elliptic filter has ripple in both the passband and the stopband Setting the passband ripple to Rp 3 dB and the stopband ripple Rs 20 dB down repeat Prob 51012 using the ellip command 06LathiC06 2017925 1554 page 593 1 C H A P T E R CONTINUOUSTIME SIGNAL ANALYSIS THE FOURIER SERIES 6 Electrical engineers instinctively think of signals in terms of their frequency spectra and think of systems in terms of their frequency response Most teenagers know about the audible portion of audio signals having a bandwidth of about 20 kHz and the need for goodquality speakers to respond up to 20 kHz This is basically thinking in the frequency domain In Chs 4 and 5 we discussed extensively the frequencydomain representation of systems and their spectral response system response to signals of various frequencies In Chs 6 through 9 we discuss spectral representation of signals where signals are expressed as a sum of sinusoids or exponentials Actually we touched on this topic in Chs 4 and 5 Recall that the Laplace transform of a continuoustime signal is its spectral representation in terms of exponentials or sinusoids of complex frequencies Similarly the ztransform of a discretetime signal is its spectral representation in terms of discretetime exponentials However in the earlier chapters we were concerned mainly with system representation the spectral representation of signals was incidental to the system analysis Spectral analysis of signals is an important topic in its own right and now we turn to this subject In this chapter we show that a periodic signal can be represented as a sum of sinusoids or exponentials of various frequencies These results are extended to aperiodic signals in Ch 7 and to discretetime signals in Ch 9 The fascinating subject of sampling of continuoustime signals is discussed in Ch 8 leading to AD analogtodigital and DA conversion Chapter 8 forms the bridge between the continuoustime and the discretetime worlds 61 PERIODIC SIGNAL REPRESENTATION BY TRIGONOMETRIC FOURIER SERIES As seen in Sec 133 Eq 17 a periodic signal xt with period T0 Fig 61 has the property xt xt T0 for all t The smallest value of T0 that satisfies this periodicity condition is the fundamental period of xt As argued in Sec 133 this equation implies that xt starts at and continues to Moreover the area under a periodic signal xt over any interval of duration T0 is the same that is for any 593 06LathiC06 2017925 1554 page 601 9 61 Periodic Signal Representation by Trigonometric Fourier Series 601 PLOTTING FOURIER SERIES SPECTRA USING MATLAB MATLAB is well suited to compute and plot Fourier series spectra The results in Fig 63 which plot Cn and θn as functions of n match Figs 62b and 62c which plot Cn and θn as functions of ω nω0 2n Plots of an and bn are similarly simple to generate n 010 thetan atan4n Cnn0 0504 Cnn0 05042sqrt116nn02 subplot121 stemnCnk axis5 105 0 6 xlabeln ylabelCn subplot122 stemnthetank axis5 105 16 0 xlabeln ylabel hetan 10 n 0 02 04 06 Cn 0 2 4 6 8 0 2 4 6 8 10 n 15 1 05 0 θn Figure 63 Fourier Series spectra for Ex 61 using MATLAB The amplitude and phase spectra for xt in Figs 62b and 62c tell us at a glance the frequency composition of xt that is the amplitudes and phases of various sinusoidal components of xt Knowing the frequency spectra we can reconstruct xt as shown on the righthand side of Eq 611 Therefore the frequency spectra Figs 62b 62c provide an alternative descriptionthe frequencydomain description of xt The timedomain description of xt is shown in Fig 62a A signal therefore has a dual identity the timedomain identity xt and the frequencydomain identity Fourier spectra The two identities complement each other taken together they provide a better understanding of a signal An interesting aspect of Fourier series is that whenever there is a jump discontinuity in xt the series at the point of discontinuity converges to an average of the lefthand and righthand limits of xt at the instant of discontinuity In the present example for instance xt is discontinuous at t 0 with x0 1 and x0 xπ eπ2 0208 The corresponding Fourier series converges to a value 1 02082 0604 at t 0 This is easily verified from Eq 611 by setting t 0 This behavior of the Fourier series is dictated by its convergence in the mean discussed later in Secs 62 and 65 06LathiC06 2017925 1554 page 611 19 61 Periodic Signal Representation by Trigonometric Fourier Series 611 JeanBaptisteJoseph Fourier and Napoleon Napoleon was the first modern ruler with a scientific education and he was one of the rare persons who are equally comfortable with soldiers and scientists The age of Napoleon was one of the most fruitful in the history of science Napoleon liked to sign himself as member of Institut de France a fraternity of scientists and he once expressed to Laplace his regret that force of circumstances has led me so far from the career of a scientist 2 Many great figures in science and mathematics including Fourier and Laplace were honored and promoted by Napoleon In 1798 he took a group of scientists artists and scholarsFourier among themon his Egyptian expedition with the promise of an exciting and historic union of adventure and research Fourier proved to be a capable administrator of the newly formed Institut dÉgypte which incidentally was responsible for the discovery of the Rosetta Stone The inscription on this stone in two languages and three scripts hieroglyphic demotic and Greek enabled Thomas Young and JeanFrançois Champollion a protégé of Fourier to invent a method of translating hieroglyphic writings of ancient Egyptthe only significant result of Napoleons Egyptian expedition Back in France in 1801 Fourier briefly served in his former position as professor of mathematics at the École Polytechnique in Paris In 1802 Napoleon appointed him the prefect of Isère with its headquarters in Grenoble a position in which Fourier served with distinction Fourier was named Baron of the Empire by Napoleon in 1809 Later when Napoleon was exiled to Elba his route was to take him through Grenoble Fourier had the route changed to avoid meeting Napoleon which would have displeased Fouriers new master King Louis XVIII Within a year Napoleon escaped from Elba and returned to France At Grenoble Fourier was brought before him in chains Napoleon scolded Fourier for his ungrateful behavior but reappointed him the prefect of Rhône at Lyons Within four months Napoleon was defeated at Waterloo and was exiled to St Helena where he died in 1821 Fourier once again was in disgrace as a Bonapartist and had 06LathiC06 2017925 1554 page 617 25 62 Existence and Convergence of the Fourier Series 617 EXAMPLE 65 SquareWave Synthesis by Truncated Fourier Series Using MATLAB Use MATLAB to synthesize and plot the square wave of Fig 68a using a Fourier series that is truncated to the 19th harmonic The result should match Fig 68e To synthesize the waveform we use the Fourier series of Eq 613 x t 10modtpi22pipi t linspace2pi2pi10001 x19 05onessizet for n119 x19 x192pinsinpin2cosnt end plottx19k axis2pi 2pi 02 12 xlabelt ylabelx19t As expected the result of Fig 69 matches Fig 68e 6 4 2 0 2 4 6 t 0 05 1 x19t Figure 69 Using MATLAB to synthesize a square wave via truncated Fourier series PHASE SPECTRUM THE WOMAN BEHIND A SUCCESSFUL MAN The role of the amplitude spectrum in shaping the waveform xt is quite clear However the role of the phase spectrum in shaping this waveform is less obvious Yet the phase spectrum like the woman behind a successful man plays an equally important role in waveshaping We can explain this role by considering a signal xt that has rapid changes such as jump discontinuities To synthesize an instantaneous change at a jump discontinuity the phases of the various sinusoidal components in its spectrum must be such that all or most of the harmonic components will have Or to keep up with the times the man behind a successful woman 06LathiC06 2017925 1554 page 619 27 62 Existence and Convergence of the Fourier Series 619 FOURIER SYNTHESIS OF DISCONTINUOUS FUNCTIONS THE GIBBS PHENOMENON Figure 68 showed the square function xt and its approximation by a truncated trigonometric Fourier series that includes only the first N harmonics for N 1 3 5 and 19 The plot of the truncated series approximates closely the function xt as N increases and we expect that the series will converge exactly to xt as N Yet the curious fact as seen from Fig 68 is that even for large N the truncated series exhibits an oscillatory behavior and an overshoot approaching a value of about 9 in the vicinity of the discontinuity at the nearest peak of oscillation Regardless of the value of N the overshoot remains at about 9 Such strange behavior certainly would undermine anyones faith in the Fourier series In fact this behavior puzzled many scholars at the turn of the century Josiah Willard Gibbs an eminent mathematical physicist who was the inventor of vector analysis gave a mathematical explanation of this behavior now called the Gibbs phenomenon We can reconcile the apparent aberration in the behavior of the Fourier series by observing from Fig 68 that the frequency of oscillation of the synthesized signal is Nf0 so the width of the spike with 9 overshoot is approximately 12Nf0 As we increase N the frequency of oscillation increases and the spike width 12Nf0 diminishes As N the error power 0 because the error consists mostly of the spikes whose widths 0 Therefore as N the corresponding Fourier series differs from xt by about 9 at the immediate left and right of the points of discontinuity and yet the error power 0 The reason for all this confusion is that in this case the Fourier series converges in the mean When this happens all we promise is that the error energy over one period 0 as N Thus the series may differ from xt at some points and yet have the error signal power zero as verified earlier Note that the series in this case also converges pointwise at all points except the points of discontinuity It is precisely at the discontinuities that the series differs from xt by 9 When we use only the first N terms in the Fourier series to synthesize a signal we are abruptly terminating the series giving a unit weight to the first N harmonics and zero weight to all the remaining harmonics beyond N This abrupt termination of the series causes the Gibbs phenomenon in synthesis of discontinuous functions Section 78 offers more discussion on the Gibbs phenomenon its ramifications and cure The Gibbs phenomenon is present only when there is a jump discontinuity in xt When a continuous function xt is synthesized by using the first N terms of the Fourier series the synthesized function approaches xt for all t as N No Gibbs phenomenon appears This can be seen in Fig 611 which shows one cycle of a continuous periodic signal being synthesized from the first 19 harmonics Compare the similar situation for a discontinuous signal in Fig 68 DRILL 63 Rate of Spectral Decay By inspection of signals in Figs 62a 67a and 67b determine the asymptotic rate of decay of their amplitude spectra There is also an undershoot of 9 at the other side at t π2 of the discontinuity Actually at discontinuities the series converges to a value midway between the values on either side of the discontinuity The 9 overshoot occurs at t π2 and 9 undershoot occurs at t π2 06LathiC06 2017925 1554 page 620 28 620 CHAPTER 6 CONTINUOUSTIME SIGNAL ANALYSIS THE FOURIER SERIES 0 t 1 Figure 611 Fourier synthesis of a continuous signal using first 19 harmonics ANSWERS 1n1n2 and 1n respectively A HISTORICAL NOTE ON THE GIBBS PHENOMENON Normally speaking troublesome functions with strange behavior are invented by mathematicians we rarely see such oddities in practice In the case of the Gibbs phenomenon however the tables were turned A rather puzzling behavior was observed in a mundane object a mechanical wave synthesizer and then wellknown mathematicians of the day were dispatched on the scent of it to discover its hideout Albert Michelson of MichelsonMorley fame was an intense practical man who developed ingenious physical instruments of extraordinary precision mostly in the field of optics His harmonic analyzer developed in 1898 could compute the first 80 coefficients of the Fourier series of a signal xt specified by any graphical description The instrument could also be used as a harmonic synthesizer which could plot a function xt generated by summing the first 80 harmonics Fourier components of arbitrary amplitudes and phases This analyzer therefore had the ability of selfchecking its operation by analyzing a signal xt and then adding the resulting 80 components to see whether the sum yielded a close approximation of xt Michelson found that the instrument checked very well with most of signals analyzed However when he tried a discontinuous function such as a square wave a curious behavior was observed The sum of 80 components showed oscillatory behavior ringing with an overshoot of 9 in the vicinity of the points of discontinuity Moreover this behavior was a constant feature regardless of the number of terms added A larger number of terms made the oscillations proportionately faster but regardless of the number of terms added the overshoot remained 9 This puzzling behavior caused Michelson to suspect some mechanical defect in his synthesizer He wrote about his observation in a letter to Nature December 1898 Josiah Willard Gibbs who was a professor at Yale investigated and clarified this behavior for a sawtooth periodic signal in a letter to Nature 7 Later in 1906 Bôcher generalized the result for any function with discontinuity 8 Actually it was a periodic sawtooth signal 06LathiC06 2017925 1554 page 626 34 626 CHAPTER 6 CONTINUOUSTIME SIGNAL ANALYSIS THE FOURIER SERIES 5 n 0 02 04 06 Dn 5 0 5 0 5 n 2 0 2 Dn rad Figure 613 Exponential Fourier series spectra for Ex 67 WHAT IS A NEGATIVE FREQUENCY The existence of the spectrum at negative frequencies is somewhat disturbing because by definition the frequency number of repetitions per second is a positive quantity How do we interpret a negative frequency We can use a trigonometric identity to express a sinusoid of a negative frequency ω0 as cosω0t θ cosω0t θ This equation clearly shows that the frequency of a sinusoid cosω0t θ is ω0 which is a positive quantity The same conclusion is reached by observing that ejω0t cos ω0t jsin ω0t Thus the frequency of exponentials ejω0t is indeed ω0 How do we then interpret the spectral plots for negative values of ω A more satisfying way of looking at the situation is to say that exponential spectra are a graphical representation of coefficients Dn as a function of ω Existence of the spectrum at ω nω0 is merely an indication that an exponential component ejnω0t exists in the series We know that a sinusoid of frequency nω0 can be expressed in terms of a pair of exponentials ejnω0t and ejnω0t We see a close connection between the exponential spectra in Fig 612 and the spectra of the corresponding trigonometric Fourier series for xt Figs 62b 62c Equation 622 explains the reason for the close connection for real xt between the trigonometric spectra Cn and θn with exponential spectra Dn and Dn The dc components D0 and C0 are identical in both spectra Moreover the exponential amplitude spectrum Dn is half the trigonometric amplitude spectrum Cn for n 1 The exponential angle spectrum Dn is identical to the trigonometric phase spectrum θn for n 0 We can therefore produce the exponential spectra merely by inspection of trigonometric spectra and vice versa The following example demonstrates this feature 06LathiC06 2017925 1554 page 635 43 63 Exponential Fourier Series 635 633 Properties of the Fourier Series As with the Laplace and ztransforms the Fourier series has a variety of properties that can simplify work and help provide a more intuitive understanding of signals Table 62 provides the most important properties of the Fourier series for a periodic signal xt and its spectrum Dn Properties that involve two signals require that the two signals have a common fundamental frequency ω0 While not given here the proofs of these properties are straightforward and parallel the proofs of the Fourier transform properties given in Ch 7 To demonstrate the utility of Fourier series properties let us consider an example where we use a selection of properties to simplify the work of finding a piecewise polynomial signals spectrum TABLE 62 Selected Fourier Series Properties Operation xt Dn Scalar multiplication kxt kDn Addition x1t x2t D1n D2n x1t x2t require same ω0 Conjugation xt D n Reversal xt Dn Time shifting xt t0 Dnejnω0t0 Frequency shifting xtejn0ω0t Dnn0 Frequency convolution x1tx2t D1n D2n x1t x2t require same ω0 Time differentiation dkxt dtk jnω0kDn EXAMPLE 611 Using Fourier Series Properties Use properties rather than integration to compute the exponential Fourier series coefficients Dn of the triangular signal xt shown in Fig 64 Verify the correctness of Dn for A 1 by synthesizing xt with a suitable truncation of Eq 619 From Fig 64 we see that xt is a piecewise linear function that is T0 2 periodic To compute Dn directly using Eq 619 would therefore require tedious integration by parts Fortunately we can compute Dn without integration by instead using Fourier series properties First however we must compute the dc component D0 separately from other Dn By simple inspection of Fig 64 we see that xt has no dc component so D0 0 To determine the remaining Dn we begin by noting that xt has a constant slope of either 2A or 2A Thus differentiating xt once yields a square wave with amplitudes 2A Here differentiation reduces xt from a piecewise linear to a piecewise constant function 06LathiC06 2017925 1554 page 641 49 65 Generalized Fourier Series Signals as Vectors 641 DUAL PERSONALITY OF A SIGNAL The discussion so far shows that a periodic signal has a dual personalitythe time domain and the frequency domain It can be described by its waveform or by its Fourier spectra The time and frequencydomain descriptions provide complementary insights into a signal For indepth perspective we need to understand both these identities It is important to learn to think of a signal from both perspectives In the next chapter we shall see that aperiodic signals also have this dual personality Moreover we shall show that even LTI systems have this dual personality which offers complementary insights into the system behavior LIMITATIONS OF THE FOURIER SERIES METHOD OF ANALYSIS We have developed here a method of representing a periodic signal as a weighted sum of everlasting exponentials whose frequencies lie along the ω axis in the s plane This representation Fourier series is valuable in many applications However as a tool for analyzing linear systems it has serious limitations and consequently has limited utility for the following reasons 1 The Fourier series can be used only for periodic inputs All practical inputs are aperiodic remember that a periodic signal starts at t 2 The Fourier methods can be applied readily to BIBOstable or asymptotically stable systems It cannot handle unstable or even marginally stable systems The first limitation can be overcome by representing aperiodic signals in terms of everlasting exponentials This representation can be achieved through the Fourier integral which may be considered to be an extension of the Fourier series We shall therefore use the Fourier series as a steppingstone to the Fourier integral developed in the next chapter The second limitation can be overcome by using exponentials est where s is not restricted to the imaginary axis but is free to take on complex values This generalization leads to the Laplace integral discussed in Ch 4 the Laplace transform 65 GENERALIZED FOURIER SERIES SIGNALS AS VECTORS We now consider a very general approach to signal representation with farreaching consequences There is a perfect analogy between signals and vectors the analogy is so strong that the term analogy understates the reality Signals are not just like vectors Signals are vectors A vector can be represented as a sum of its components in a variety of ways depending on the choice of coordinate system A signal can also be represented as a sum of its components in a variety of ways Let us begin with some basic vector concepts and then apply these concepts to signals This section closely follows the material from the authors earlier book 10 Omission of this section will not cause any discontinuity in understanding the rest of the book Derivation of Fourier series through the signalvector analogy provides an interesting insight into signal representation and other topics such as signal correlation data truncation and signal detection 06LathiC06 2017925 1554 page 642 50 642 CHAPTER 6 CONTINUOUSTIME SIGNAL ANALYSIS THE FOURIER SERIES 651 Component of a Vector A vector is specified by its magnitude and its direction We shall denote all vectors by boldface For example x is a certain vector with magnitude or length x For the two vectors x and y shown in Fig 621 we define their dot inner or scalar product as x y xycos θ where θ is the angle between these vectors Using this definition we can express x the length of a vector x as x2 x x Let the component of x along y be cy as depicted in Fig 621 Geometrically the component of x along y is the projection of x on y and is obtained by drawing a perpendicular from the tip of x on the vector y as illustrated in Fig 621 What is the mathematical significance of a component of a vector along another vector As seen from Fig 621 the vector x can be expressed in terms of vector y as x cy e However this is not the only way to express x in terms of y From Fig 622 which shows two of the infinite other possibilities we have x c1y e1 c2y e2 In each of these three representations x is represented in terms of y plus another vector called the error vector If we approximate x by cy x cy the error in the approximation is the vector e x cy Similarly the errors in approximations in these drawings are e1 Fig 622a and e2 Fig 622b What is unique about the approximation in Fig 621 is that the error vector is the smallest We can now define mathematically the component of a vector x along vector y to be cy where c is chosen to minimize the length of the error vector e x cy Now the length of the component of x along y is xcosθ But it is also cy as seen from Fig 621 Therefore cy xcosθ Multiplying both sides by y yields cy2 xycos θ x y x cy y e u Figure 621 Component projection of a vector along another vector 06LathiC06 2017925 1554 page 660 68 660 CHAPTER 6 CONTINUOUSTIME SIGNAL ANALYSIS THE FOURIER SERIES this purpose we need samples of xt over one period starting at t 0 In this algorithm it is also preferable although not necessary that N0 be a power of 2 ie N0 2m where m is an integer EXAMPLE 616 Numerical Computation of Fourier Spectra Numerically compute and then plot the exponential Fourier spectra for the periodic signal in Fig 62a Ex 61 The samples of xt start at t 0 and the last N0th sample is at t T0 T At the points of discontinuity the sample value is taken as the average of the values of the function on two sides of the discontinuity Thus the sample at t 0 is not 1 but eπ2 12 0604 To determine N0 we require that Dn for n N02 be negligible Because xt has a jump discontinuity Dn decays rather slowly as 1n Hence a choice of N0 200 is acceptable because the N02nd 100th harmonic is about 1 of the fundamental However we also require N0 to be a power of 2 Hence we shall take N0 256 28 First the basic parameters are established T0 pi N0 256 T T0N0 t 0TTN01 x expt2 x1 exppi212 Next the DFT computed by means of the fft function is used to approximate the exponential Fourier spectra up to n N02 To facilitate comparison with previous plots of Dn we only plot the results over 5 n 5 Dn fftxN0 n N02N021 clf subplot121 stemnabsfftshiftDnk axis5 5 0 6 xlabeln ylabelDn subplot122 stemnanglefftshiftDnk axis5 5 2 2 xlabeln ylabelangle Dn rad As shown in Fig 628 the resulting approximation is visually indistinguishable from the true Fourier series spectra shown in Fig 612 or Fig 613 n 0 02 04 06 Dn 5 5 0 5 0 5 n 2 0 2 Dn rad Figure 628 Numerical approximation of exponential Fourier series spectra using the DFT 06LathiC06 2017925 1554 page 662 70 662 CHAPTER 6 CONTINUOUSTIME SIGNAL ANALYSIS THE FOURIER SERIES t time vector for xN Define FS coefficients for signal xt D n 12pinexp1jnA1nA 1jexp1jnpi Construct truncated FS approximation of xt using N harmonics t linspacepi42pipi410000 Time vector exceeds one period xN 2piA4pionessizet Compute dc term for n 1N Compute N remaining terms xN xNrealDnexp1jnt conjDnexp1jnt end Although theoretically not required the real command ensures that small computer roundoff errors do not cause a complexvalued result Using program CH6MP1 with A π2 and N 20 Fig 629 compares xt and x20t A pi2 x20t CH6MP1A20 plottx20ktxtAk axispi42pipi40111 xlabelt ylabelx20t As expected the falling edge is accompanied by the overshoot that is characteristic of the Gibbs phenomenon Increasing N to 100 as shown in Fig 630 improves the approximation but does not reduce the overshoot x100t CH6MP1A100 plottx100ktxtAk axispi42pipi40111 xlabelt ylabelx100t Reducing A to π64 produces a curious result For N 20 both the rising and falling edges are accompanied by roughly 9 of overshoot as shown in Fig 631 As the number of terms is increased overshoot persists only in the vicinity of jump discontinuities For xNt increasing N decreases the overshoot near the rising edge but not near the falling edge Remember that it is a 0 1 2 3 4 5 6 7 t 0 02 04 06 08 1 x20t Figure 629 Comparison of x20t and xt when A π2 06LathiC06 2017925 1554 page 663 71 67 MATLAB Fourier Series Applications 663 0 1 2 3 4 5 6 7 t 0 02 04 06 08 1 x100 t Figure 630 Comparison of x100t and xt when A π2 0 1 2 3 4 5 6 7 t 0 02 04 06 08 1 x20t Figure 631 Comparison of x20t and xt when A π64 0 1 2 3 4 5 6 7 t 0 02 04 06 08 1 x100 t Figure 632 Comparison of x100t and xt when A π64 true jump discontinuity that causes the Gibbs phenomenon A continuous signal no matter how sharply it rises can always be represented by a Fourier series at every point within any small error by increasing N This is not the case when a true jump discontinuity is present Figure 632 illustrates this behavior using N 100 06LathiC06 2017925 1554 page 665 73 67 MATLAB Fourier Series Applications 665 001 0008 0006 0004 0002 0 0002 0004 0006 0008 001 t sec 5 0 5 10 15 20 mt volts Figure 633 Test signal mt with θn 0 As with any computer MATLAB cannot generate truly random numbers Rather it generates pseudorandom numbers Pseudorandom numbers are deterministic sequences that appear to be random The particular sequence of numbers that is realized depends entirely on the initial state of the pseudorandom number generator Setting the generators initial state to a known value allows a random experiment with reproducible results The command rng0 initializes the state of the pseudorandom number generator to a known condition of zero and the MATLAB command randab generates an abyb matrix of pseudorandom numbers that are uniformly distributed over the interval 01 Radian phases occupy the wider interval 02π so the results from rand need to be appropriately scaled rng0 thetarand0 2pirandN1 Next we recompute and plot mt using the randomly chosen θn mrand0 mthetarand0tomega plottmrand0k axis0010011010 xlabelt sec ylabelmt volts setgcaytickminmrand0maxmrand0 grid on For a vector input the min and max commands return the minimum and maximum values of the vector Using these values to set y axis tick marks makes it easy to identify the extreme values of the mt As seen from Fig 634 the maximum amplitude is now 76307 which is significantly smaller than the maximum of 20 when θn 0 Randomly chosen phases suffer a fatal fault there is little guarantee of optimal performance For example repeating the experiment with rng5 produces a maximum magnitude of 82399 volts as shown in Fig 635 This value is significantly higher than the previous maximum of 76307 volts Clearly it is better to replace a random solution with an optimal solution What constitutes optimal Many choices exist but desired signal criteria naturally suggest that optimal phases minimize the maximum magnitude of mt over all t To find these optimal phases MATLABs fminsearch command is useful First the function to be minimized called the objective function is defined maxmagm thetatomega maxabssumcosomegatthetaonessizet 06LathiC06 2017925 1554 page 666 74 666 CHAPTER 6 CONTINUOUSTIME SIGNAL ANALYSIS THE FOURIER SERIES 001 0008 0006 0004 0002 0 0002 0004 0006 0008 001 t sec 74460 76307 mt volts Figure 634 Test signal mt with random θn found by using rng0 001 0008 0006 0004 0002 0 0002 0004 0006 0008 001 t sec 82399 78268 mt volts Figure 635 Test signal mt with random θn found by using randstate1 The anonymous function argument order is important fminsearch uses the first input argument as the variable of minimization To minimize over θ as desired θ must be the first argument of the objective function maxmagm Next the time vector is shortened to include only one period of mt t linspace0001401 A full period ensures that all values of mt are considered the short length of t helps ensure that functions execute quickly An initial value of θ is randomly chosen to begin the search rng0 thetainit 2pirandN1 thetaopt fminsearchmaxmagmthetainittomega Notice that fminsearch finds the minimizer to maxmagm over θ by using an initial value thetainit Most numerical minimization techniques are capable of finding only local minima and fminsearch is no exception As a result fminsearch does not always produce a unique solution The empty square brackets indicate no special options are requested and the remaining ordered arguments are secondary inputs for the objective function Full format details for fminsearch are available from MATLABs help facilities 06LathiC06 2017925 1554 page 667 75 68 Summary 667 001 0008 0006 0004 0002 0 0002 0004 0006 0008 001 t sec 53632 53414 mt volts Figure 636 Test signal mt with optimized phases Figure 636 shows the phaseoptimized test signal The maximum magnitude is reduced to a value of 53632 volts which is a significant improvement over the original peak of 20 volts Although the signals shown in Figs 633 through 636 look different they all possess the same magnitude spectra The signals differ only in phase spectra It is interesting to investigate the similarities and differences of these signals in ways other than graphs and mathematics For example is there an audible difference between the signals For computers equipped with sound capability the MATLAB sound command can be used to find out Fs 8000 t 01Fs2 Two second records at a sampling rate of 8kHz soundmthetatomega20Fs Play scaled mt constructed using zero phases Since the sound command clips magnitudes that exceed 1 the input vector is scaled by 120 to avoid clipping and the resulting sound distortion The signals using other phase assignments are created and played in a similar fashion How well does the human ear discern the differences in phase spectra If you are like most people you will not be able to discern any differences in how these waveforms sound 68 SUMMARY In this chapter we showed how a periodic signal can be represented as a sum of sinusoids or exponentials If the frequency of a periodic signal is f0 then it can be expressed as a weighted sum of a sinusoid of frequency f0 and its harmonics the trigonometric Fourier series We can reconstruct the periodic signal from a knowledge of the amplitudes and phases of these sinusoidal components amplitude and phase spectra If a periodic signal xt has an even symmetry its Fourier series contains only cosine terms including dc In contrast if xt has an odd symmetry its Fourier series contains only sine terms If xt has neither type of symmetry its Fourier series contains both sine and cosine terms At points of discontinuity the Fourier series for xt converges to the mean of the values of xt on either side of the discontinuity For signals with discontinuities the Fourier series converges in the mean and exhibits Gibbs phenomenon at the points of discontinuity The amplitude spectrum of the Fourier series for a periodic signal xt with jump discontinuities decays slowly as 1n with frequency We need a large number of terms in the Fourier series to approximate xt within 06LathiC06 2017925 1554 page 668 76 668 CHAPTER 6 CONTINUOUSTIME SIGNAL ANALYSIS THE FOURIER SERIES a given error In contrast the amplitude spectrum of a smoother periodic signal decays faster with frequency and we require a smaller number of terms in the series to approximate xt within a given error A sinusoid can be expressed in terms of exponentials Therefore the Fourier series of a periodic signal can also be expressed as a sum of exponentials the exponential Fourier series The exponential form of the Fourier series and the expressions for the series coefficients are more compact than those of the trigonometric Fourier series Also the response of LTIC systems to an exponential input is much simpler than that for a sinusoidal input Moreover the exponential form of representation lends itself better to mathematical manipulations than does the trigonometric form This includes the establishment of useful Fourier series properties that simplify work and help provide a more intuitive understanding of signals For these reasons the exponential form of the series is preferred in modern practice in the areas of signals and systems The plots of amplitudes and angles of various exponential components of the Fourier series as functions of the frequency are the exponential Fourier spectra amplitude and angle spectra of the signal Because a sinusoid cosω0t can be represented as a sum of two exponentials ejω0t and ejω0t the frequencies in the exponential spectra range from ω to By definition frequency of a signal is always a positive quantity Presence of a spectral component of a negative frequency nω0 merely indicates that the Fourier series contains terms of the form ejnω0t The spectra of the trigonometric and exponential Fourier series are closely related and one can be found by the inspection of the other In Sec 65 we discuss a method of representing signals by the generalized Fourier series of which the trigonometric and exponential Fourier series are special cases Signals are vectors in every sense Just as a vector can be represented as a sum of its components in a variety of ways depending on the choice of the coordinate system a signal can be represented as a sum of its components in a variety of ways of which the trigonometric and exponential Fourier series are only two examples Just as we have vector coordinate systems formed by mutually orthogonal vectors we also have signal coordinate systems basis signals formed by mutually orthogonal signals Any signal in this signal space can be represented as a sum of the basis signals Each set of basis signals yields a particular Fourier series representation of the signal The signal is equal to its Fourier series not in the ordinary sense but in the special sense that the energy of the difference between the signal and its Fourier series approaches zero This allows for the signal to differ from its Fourier series at some isolated points REFERENCES 1 Bell E T Men of Mathematics Simon Schuster New York 1937 2 Durant W and Durant A The Age of Napoleon Part XI in The Story of Civilization Series Simon Schuster New York 1975 3 Calinger R Classics of Mathematics 4th ed Moore Publishing Oak Park IL 1982 4 Lanczos C Discourse on Fourier Series Oliver Boyd London 1966 5 Körner T W Fourier Analysis Cambridge University Press Cambridge UK 1989 6 Guillemin E A Theory of Linear Physical Systems Wiley New York 1963 7 Gibbs W J Nature vol 59 p 606 April 1899 8 Bôcher M Annals of Mathematics vol 7 no 2 1906 07LathiC07 2017925 1917 page 680 1 C H A P T E R CONTINUOUSTIME SIGNAL ANALYSIS THE FOURIER TRANSFORM 7 We can analyze linear systems in many different ways by taking advantage of the property of linearity whereby the input is expressed as a sum of simpler components The system response to any complex input can be found by summing the systems response to these simpler components of the input In timedomain analysis we separated the input into impulse components In the frequencydomain analysis in Ch 4 we separated the input into exponentials of the form est the Laplace transform where the complex frequency s σ jω The Laplace transform although very valuable for system analysis proves somewhat awkward for signal analysis where we prefer to represent signals in terms of exponentials ejωt instead of est This is accomplished by the Fourier transform In a sense the Fourier transform may be considered to be a special case of the Laplace transform with s jω Although this view is true most of the time it does not always hold because of the nature of convergence of the Laplace and Fourier integrals In Ch 6 we succeeded in representing periodic signals as a sum of everlasting sinusoids or exponentials of the form ejωt The Fourier integral developed in this chapter extends this spectral representation to aperiodic signals 71 APERIODIC SIGNAL REPRESENTATION BY THE FOURIER INTEGRAL Applying a limiting process we now show that an aperiodic signal can be expressed as a continuous sum integral of everlasting exponentials To represent an aperiodic signal xt such as the one depicted in Fig 71a by everlasting exponentials let us construct a new periodic signal xT0t formed by repeating the signal xt at intervals of T0 seconds as illustrated in Fig 71b The period T0 is made long enough to avoid overlap between the repeating pulses The periodic signal xT0t can be represented by an exponential Fourier series If we let T0 the pulses in the periodic signal repeat after an infinite interval and therefore lim T0xT0t xt 680 07LathiC07 2017125 2004 page 701 22 73 Some Properties of the Fourier Transform 701 The Fourier transform is given by ut 1 jω πδω Clearly Xjω Xω in this case To understand this puzzle consider the fact that we obtain Xjω by setting s jω in Eq 724 This implies that the integral on the righthand side of Eq 724 converges for s jω meaning that s jω the imaginary axis lies in the ROC for Xs The general rule is that only when the ROC for Xs includes the ω axis does setting s jω in Xs yield the Fourier transform Xω that is Xjω Xω This is the case of absolutely integrable xt If the ROC of Xs excludes the ω axis Xjω Xω This is the case for exponentially growing xt and also xt that is constant or is oscillating with constant amplitude The reason for this peculiar behavior has something to do with the nature of convergence of the Laplace and the Fourier integrals when xt is not absolutely integrable This discussion shows that although the Fourier transform may be considered as a special case of the Laplace transform we need to circumscribe such a view This fact can also be confirmed by noting that a periodic signal has the Fourier transform but the Laplace transform does not exist 73 SOME PROPERTIES OF THE FOURIER TRANSFORM We now study some of the important properties of the Fourier transform and their implications as well as applications We have already encountered two important properties linearity Eq 715 and the conjugation property Eq 711 Before embarking on this study we shall explain an important and pervasive aspect of the Fourier transform the timefrequency duality To explain this point consider the unit step function and its transforms Both the Laplace and the Fourier transform synthesize xt using everlasting exponentials of the form est The frequency s can be anywhere in the complex plane for the Laplace transform but it must be restricted to the ω axis in the case of the Fourier transform The unit step function is readily synthesized in the Laplace transform by a relatively simple spectrum Xs 1s in which the frequencies s are chosen in the RHP the region of convergence for ut is Re s 0 In the Fourier transform however we are restricted to values of s on the ω axis only The function ut can still be synthesized by frequencies along the ω axis but the spectrum is more complicated than it is when we are free to choose the frequencies in the RHP In contrast when xt is absolutely integrable the region of convergence for the Laplace transform includes the ω axis and we can synthesize xt by using frequencies along the ω axis in both transforms This leads to Xjω Xω We may explain this concept by an example of two countries X and Y Suppose these countries want to construct similar dams in their respective territories Country X has financial resources but not much manpower In contrast Y has considerable manpower but few financial resources The dams will still be constructed in both countries although the methods used will be different Country X will use expensive but efficient equipment to compensate for its lack of manpower whereas Y will use the cheapest possible equipment in a laborintensive approach to the project Similarly both Fourier and Laplace integrals converge for ut but the makeup of the components used to synthesize ut will be very different for two cases because of the constraints of the Fourier transform which are not present for the Laplace transform 07LathiC07 2017925 1917 page 708 29 708 CHAPTER 7 CONTINUOUSTIME SIGNAL ANALYSIS THE FOURIER TRANSFORM t t0 t Figure 722 Physical explanation of the timeshifting property cosωt delayed by t0 is given by cos ωt t0 cosωt ωt0 Therefore a time delay t0 in a sinusoid of frequency ω manifests as a phase delay of ωt0 This is a linear function of ω meaning that higherfrequency components must undergo proportionately higher phase shifts to achieve the same time delay This effect is depicted in Fig 722 with two sinusoids the frequency of the lower sinusoid being twice that of the upper The same time delay t0 amounts to a phase shift of π2 in the upper sinusoid and a phase shift of π in the lower sinusoid This verifies the fact that to achieve the same time delay higherfrequency sinusoids must undergo proportionately higher phase shifts The principle of linear phase shift is very important and we shall encounter it again in distortionless signal transmission and filtering applications EXAMPLE 713 Fourier Transform TimeShifting Property Use the timeshifting property to find the Fourier transform of eatt0 This function shown in Fig 723a is a timeshifted version of eat depicted in Fig 721a From Eqs 728 and 729 we have eatt0 2a a2 ω2 ejωt0 The spectrum of eatt0 Fig 723b is the same as that of eat Fig 721b except for an added phase shift of ωt0 07LathiC07 2017925 1917 page 714 35 714 CHAPTER 7 CONTINUOUSTIME SIGNAL ANALYSIS THE FOURIER TRANSFORM Old is gold but sometimes it is fools gold by using modulation whereby each radio station is assigned a distinct carrier frequency Each station transmits a modulated signal This procedure shifts the signal spectrum to its allocated band which is not occupied by any other station A radio receiver can pick up any station by tuning to the band of the desired station The receiver must now demodulate the received signal undo the effect of modulation Demodulation therefore consists of another spectral shift required to restore the signal to its original band Note that both modulation and demodulation implement spectral shifting consequently demodulation operation is similar to modulation see Sec 77 This method of transmitting several signals simultaneously over a channel by sharing its frequency band is known as frequencydivision multiplexing FDM 2 For effective radiation of power over a radio link the antenna size must be of the order of the wavelength of the signal to be radiated Audio signal frequencies are so low wavelengths are so large that impracticably large antennas would be required for radiation Here shifting the spectrum to a higher frequency a smaller wavelength by modulation solves the problem CONVOLUTION The timeconvolution property and its dual the frequencyconvolution property state that if x1t X1ω and x2t X2ω then x1tx2t X1ωX2ω time convolution 733 07LathiC07 2017925 1917 page 720 41 720 CHAPTER 7 CONTINUOUSTIME SIGNAL ANALYSIS THE FOURIER TRANSFORM Fourier series to verify spectrum correctness Let us demonstrate the idea for the current example with τ 1 To begin we represent Xω τ 2sinc2ωτ4 using an anonymous function in MATLAB Since MATLAB computes sincx as sinπxπx we must scale the input by 1π to match the notation of sinc in this book tau 1 X omega tau2sincomegatau4pi2 For our periodic replication let us pick T0 2 which is comfortably wide enough to accommodate our τ 1width function without overlap We use Eq 75 to define the needed Fourier series coefficients Dn T0 2 omega0 2piT0 D n Xnomega0T0 Let us use 25 harmonics to synthesize the periodic replication x25t of our triangular signal xt To begin waveform synthesis we set the dc portion of the signal t T0001T0 x25 D0onessizet To add the desired 25 harmonics we enter a loop for 1 n 25 and add in the Dn and Dn terms Although the result should be real small roundoff errors cause the reconstruction to be complex These small imaginary parts are removed by using the real command for n 125 x25 x25realDnexp1jomega0ntDnexp1jomega0nt end Lastly we plot the resulting truncated Fourier series synthesis of xt plottx25k xlabelt ylabelx25t Since the synthesized waveform shown in Fig 728 closely matches a 2periodic replication of the triangle wave in Fig 727a we have high confidence that both the computed Dn and by extension the Fourier spectrum Xω are correct 2 15 1 05 0 05 1 15 2 t 0 05 1 x25t Figure 728 Synthesizing a 2periodic replication of xt using a truncated Fourier series 07LathiC07 2017925 1917 page 721 42 74 Signal Transmission Through LTIC Systems 721 DRILL 79 Fourier Transform TimeDifferentiation Property Use the timedifferentiation property to find the Fourier transform of rect tτ 74 SIGNAL TRANSMISSION THROUGH LTIC SYSTEMS If xt and yt are the input and output of an LTIC system with impulse response ht then as demonstrated in Eq 735 Yω HωXω This equation does not apply to asymptotically unstable systems because ht for such systems is not Fourier transformable It applies to BIBOstable as well as most of the marginally stable systems Similarly this equation does not apply if xt is not Fourier transformable In Ch 4 we saw that the Laplace transform is more versatile and capable of analyzing all kinds of LTIC systems whether stable unstable or marginally stable Laplace transform can also handle exponentially growing inputs In comparison to the Laplace transform the Fourier transform in system analysis is not just clumsier but also very restrictive Hence the Laplace transform is preferable to the Fourier transform in LTIC system analysis We shall not belabor the application of the Fourier transform to LTIC system analysis We consider just one example here EXAMPLE 718 Fourier Transform to Determine the ZeroState Response Use the Fourier transform to find the zerostate response of a stable LTIC system with frequency response Hs 1 s 2 and the input is xt etut Stability implies that the region of convergence of Hs includes the ω axis In this case Xω 1 jω 1 For marginally stable systems if the input xt contains a finiteamplitude sinusoid of the systems natural frequency which leads to resonance the output is not Fourier transformable It does however apply to marginally stable systems if the input does not contain a finiteamplitude sinusoid of the systems natural frequency 07LathiC07 2017925 1917 page 725 46 74 Signal Transmission Through LTIC Systems 725 component is delayed by td seconds This results in the output equal to G0 times the input delayed by td seconds Because each spectral component is attenuated by the same factor G0 and delayed by exactly the same amount td the output signal is an exact replica of the input except for attenuating factor G0 and delay td For distortionless transmission we require a linear phase characteristic The phase is not only a linear function of ω it should also pass through the origin ω 0 In practice many systems have a phase characteristic that may be only approximately linear A convenient way of judging phase linearity is to plot the slope of Hω as a function of frequency This slope which is constant for an ideal linear phase ILP system is a function of ω in the general case and can be expressed as tgω d dω Hω 740 If tgω is constant all the components are delayed by the same time interval tg But if the slope is not constant the time delay tg varies with frequency This variation means that different frequency components undergo different amounts of time delay and consequently the output waveform will not be a replica of the input waveform As we shall see tgω plays an important role in bandpass systems and is called the group delay or envelope delay Observe that constant td Eq 739 implies constant tg Note that Hω φ0 ωtg also has a constant tg Thus constant group delay is a more relaxed condition It is often thought erroneously that flatness of amplitude response Hω alone can guarantee signal quality However a system that has a flat amplitude response may yet distort a signal beyond recognition if the phase response is not linear td not constant THE NATURE OF DISTORTION IN AUDIO AND VIDEO SIGNALS Generally speaking the human ear can readily perceive amplitude distortion but is relatively insensitive to phase distortion For the phase distortion to become noticeable the variation in delay variation in the slope of Hω should be comparable to the signal duration or the physically perceptible duration in case the signal itself is long In the case of audio signals each spoken syllable can be considered to be an individual signal The average duration of a spoken syllable is of a magnitude of the order of 001 to 01 second Audio systems may have nonlinear phases yet no noticeable signal distortion results because in practical audio systems maximum variation in the slope of Hω is only a small fraction of a millisecond This is the real truth underlying the statement that the human ear is relatively insensitive to phase distortion 3 As a result the manufacturers of audio equipment make available only Hω the amplitude response characteristic of their systems For video signals in contrast the situation is exactly the opposite The human eye is sensitive to phase distortion but is relatively insensitive to amplitude distortion Amplitude distortion in television signals manifests itself as a partial destruction of the relative halftone values of the resulting picture but this effect is not readily apparent to the human eye Phase distortion nonlinear phase on the other hand causes different time delays in different picture elements The result is a smeared picture and this effect is readily perceived by the human eye Phase distortion is also very important in digital communication systems because the nonlinear phase characteristic of a channel causes pulse dispersion spreading out which in turn causes pulses to interfere with neighboring pulses Such interference between pulses can cause an error in the pulse amplitude at the receiver a binary 1 may read as 0 and vice versa 07LathiC07 2017925 1917 page 727 48 74 Signal Transmission Through LTIC Systems 727 spectrum ˆYω is given by ˆYω HωˆZω HωXω ωc Recall that the bandwidth of Xω is W so that the bandwidth of Xω ωc is 2W centered at ωc Over this range Hω is given by Eq 741 Hence ˆYω G0Xω ωcejφ0ωtg G0ejφ0Xω ωceωtg Use of Eqs 729 and 730 yields ˆyt as ˆyt G0ejφ0xt tgejωcttg G0xt tgejωcttgφ0 This is the system response to input ˆzt xtejωct which is a complex signal We are really interested in finding the response to the input zt xtcosωct which is the real part of ˆzt xtejωct Hence we use Eq 231 to obtain yt the system response to the input zt xtcosωct as yt G0xt tgcosωct tg φ0 742 where tg the group or envelope delay is the negative slope of Hω at ωc The output yt is basically the delayed input zt tg except that the output carrier acquires an extra phase φ0 The output envelope xt tg is the delayed version of the input envelope xt and is not affected by extra phase φ0 of the carrier In a modulated signal such as xtcosωct the information generally resides in the envelope xt Hence the transmission is considered to be distortionless if the envelope xt remains undistorted Most practical systems satisfy Eq 741 at least over a very small band Figure 730b shows a typical case in which this condition is satisfied for a small band W centered at frequency ωc A system in Eq 741 is said to have a generalized linear phase GLP as illustrated in Fig 730 The ideal linear phase ILP characteristics is shown in Fig 729 For distortionless transmission of bandpass signals the system need satisfy Eq 741 only over the bandwidth of the bandpass signal Caution Recall that the phase response associated with the amplitude response may have jump discontinuities when the amplitude response goes negative Jump discontinuities also arise because of the use of the principal value for phase Under such conditions to compute the group delay Eq 740 we should ignore the jump discontinuities Equation 742 can also be expressed as yt Goxt tgcosωct tph where tph called the phase delay at ωc is given by tphωc ωctg φ0ωc Generally tph varies with ω and we can write tphω ωtg φ0 ω Recall also that tg itself may vary with ω 07LathiC07 2017925 1917 page 728 49 728 CHAPTER 7 CONTINUOUSTIME SIGNAL ANALYSIS THE FOURIER TRANSFORM EXAMPLE 719 Distortionless Bandpass Transmission a A signal zt shown in Fig 731b is given by zt xtcosωct where ωc 2000π The pulse xt Fig 731a is a lowpass pulse of duration 01 second and has a bandwidth of about 10 Hz This signal is passed through a filter whose frequency response is shown in Fig 731c shown only for positive ω Find and sketch the filter output yt b Find the filter response if ωc 4000π a The spectrum Zω is a narrow band of width 20 Hz centered at frequency f0 1 kHz The gain at the center frequency 1 kHz is 2 The group delay which is the negative of the slope of the phase plot can be found by drawing tangents at ωc as shown in Fig 731c The negative of the slope of the tangent represents tg and the intercept along the vertical axis by the tangent represents φ0 at that frequency From the tangents at ωc we find tg the group delay as tg 24π 04π 2000π 103 The vertical axis intercept is φ0 04π Hence by using Eq 742 with gain G0 2 we obtain yt 2xt tgcosωct tg 04π ωc 2000π tg 103 Figure 731d shows the output yt which consists of the modulated pulse envelope xt delayed by 1 ms and the phase of the carrier changed by 04π The output shows no distortion of the envelope xt only the delay The carrier phase change does not affect the shape of envelope Hence the transmission is considered distortionless b Figure 731c shows that when ωc 4000π the slope of Hω is zero so that tg 0 Also the gain G0 15 and the intercept of the tangent with the vertical axis is φ0 31π Hence yt 15xtcosωct 31π This too is a distortionless transmission for the same reasons as for case a 07LathiC07 2017925 1917 page 732 53 732 CHAPTER 7 CONTINUOUSTIME SIGNAL ANALYSIS THE FOURIER TRANSFORM 0 t hˆt td Figure 734 Approximate realization of an ideal lowpass filter by truncation of its impulse response 01 ms would be a reasonable choice The truncation operation cutting the tail of ht to make it causal however creates some unsuspected problems We discuss these problems and their cure in Sec 78 In practice we can realize a variety of filter characteristics that approach the ideal Practical realizable filter characteristics are gradual without jump discontinuities in amplitude response DRILL 711 The Unrealizable Gaussian Response Show that a filter with Gaussian frequency response Hω eαω2 is unrealizable Demonstrate this fact in two ways first by showing that its impulse response is noncausal and then by showing that Hω violates the PaleyWiener criterion Hint Use pair 22 in Table 71 THINKING IN THE TIME AND FREQUENCY DOMAINS A TWODIMENSIONAL VIEW OF SIGNALS AND SYSTEMS Both signals and systems have dual personalities the time domain and the frequency domain For a deeper perspective we should examine and understand both these identities because they offer complementary insights An exponential signal for instance can be specified by its timedomain description such as e2tut or by its Fourier transform its frequencydomain description 1jω 2 The timedomain description depicts the waveform of a signal The frequencydomain description portrays its spectral composition relative amplitudes of its sinusoidal or exponential components and their phases For the signal e2t for instance the timedomain description portrays the exponentially decaying signal with a time constant 05 The frequencydomain description characterizes it as a lowpass signal which can be synthesized by sinusoids with amplitudes decaying with frequency roughly as 1ω An LTIC system can also be described or specified in the time domain by its impulse response ht or in the frequency domain by its frequency response Hω In Sec 26 we studied intuitive insights in the system behavior offered by the impulse response which consists of characteristic modes of the system By purely qualitative reasoning we saw that the system responds well to signals that are similar to the characteristic modes and responds poorly to signals that are very different from those modes We also saw that the shape of the impulse response ht determines the system time constant speed of response and pulse dispersion spreading which in turn determines the rate of pulse transmission The frequency response Hω specifies the system response to exponential or sinusoidal input of various frequencies This is precisely the filtering characteristic of the system 07LathiC07 2017925 1917 page 736 57 736 CHAPTER 7 CONTINUOUSTIME SIGNAL ANALYSIS THE FOURIER TRANSFORM This result indicates that the spectral components of xt in the band from 0 dc to 12706a rads 202a Hz contribute 95 of the total signal energy all the remaining spectral components in the band from 12706a rads to contribute only 5 of the signal energy DRILL 712 Signal Energy and Parsevals Theorem Use Parsevals theorem to show that the energy of the signal xt 2at2 a2 is 2πa Hint Find Xω using pair 3 of Table 71 and the duality property THE ESSENTIAL BANDWIDTH OF A SIGNAL The spectra of all practical signals extend to infinity However because the energy of any practical signal is finite the signal spectrum must approach 0 as ω Most of the signal energy is contained within a certain band of B Hz and the energy contributed by the components beyond B Hz is negligible We can therefore suppress the signal spectrum beyond B Hz with little effect on the signal shape and energy The bandwidth B is called the essential bandwidth of the signal The criterion for selecting B depends on the error tolerance in a particular application We may for example select B to be that band which contains 95 of the signal energy This figure may be higher or lower than 95 depending on the precision needed Using such a criterion we can determine the essential bandwidth of a signal The essential bandwidth B for the signal eatut using 95 energy criterion was determined in Ex 720 to be 202a Hz Suppression of all the spectral components of xt beyond the essential bandwidth results in a signal ˆxt which is a close approximation of xt If we use the 95 criterion for the essential bandwidth the energy of the error the difference xt ˆxt is 5 of Ex 77 APPLICATION TO COMMUNICATIONS AMPLITUDE MODULATION Modulation causes a spectral shift in a signal and is used to gain certain advantages mentioned in our discussion of the frequencyshifting property Broadly speaking there are two classes of modulation amplitude linear modulation and angle nonlinear modulation In this section we shall discuss some practical forms of amplitude modulation For lowpass signals the essential bandwidth may also be defined as a frequency at which the value of the amplitude spectrum is a small fraction about 1 of its peak value In Ex 720 for instance the peak value which occurs at ω 0 is 1a 07LathiC07 2017925 1917 page 738 59 738 CHAPTER 7 CONTINUOUSTIME SIGNAL ANALYSIS THE FOURIER TRANSFORM then mtcos ωct 1 2 Mω ωc Mω ωc 748 Recall that Mω ωc is Mωshifted to the right by ωc and Mω ωc is Mωshifted to the left by ωc Thus the process of modulation shifts the spectrum of the modulating signal to the left and the right by ωc Note also that if the bandwidth of mt is B Hz then as indicated in Fig 736c the bandwidth of the modulated signal is 2B Hz We also observe that the modulated signal spectrum centered at ωc is composed of two parts a portion that lies above ωc known as the upper sideband USB and a portion that lies below ωc known as the lower sideband LSB Similarly the spectrum centered at ωc has upper and lower sidebands This form of modulation is called double sideband DSB modulation for the obvious reason The relationship of B to ωc is of interest Figure 736c shows that ωc 2πB to avoid the overlap of the spectra centered at ωc If ωc 2πB the spectra overlap and the information of mt are lost in the process of modulation a loss that makes it impossible to get back mt from the modulated signal mtcos ωct EXAMPLE 721 DoubleSideband SuppressedCarrier Modulation For a baseband signal mt cos ωmt find the DSBSC signal and sketch its spectrum Identify the upper and lower sidebands We shall work this problem in the frequency domain as well as the time domain to clarify the basic concepts of DSBSC modulation In the frequencydomain approach we work with the signal spectra The spectrum of the baseband signal mt cos ωmt is given by Mω πδω ωm δω ωm The spectrum consists of two impulses located at ωm as depicted in Fig 737a The DSBSC modulated spectrum as indicated by Eq 748 is the baseband spectrum in Fig 737a shifted to the right and the left by ωc times 05 as depicted in Fig 737b This spectrum consists of impulses at ωc ωm and ωc ωm The spectrum beyond ωc is the upper sideband USB and the one below ωc is the lower sideband LSB Observe that the DSBSC spectrum does not have as a component the carrier frequency ωc This is why the term doublesideband suppressed carrier DSBSC is used for this type of modulation Practical factors may impose additional restrictions on ωc For instance in broadcast applications a radiating antenna can radiate only a narrow band without distortion This restriction implies that avoiding distortion caused by the radiating antenna calls for ωc2πB 1 The broadcast band AM radio for instance with B 5 kHz and the band of 5501600 kHz for carrier frequency gives a ratio of ωc2πB roughly in the range of 100300 07LathiC07 2017925 1917 page 742 63 742 CHAPTER 7 CONTINUOUSTIME SIGNAL ANALYSIS THE FOURIER TRANSFORM 772 Amplitude Modulation AM For the suppressedcarrier scheme just discussed a receiver must generate a carrier in frequency and phase synchronism with the carrier at a transmitter that may be located hundreds or thousands of miles away This situation calls for a sophisticated receiver which could be quite costly The other alternative is for the transmitter to transmit a carrier A cosωct along with the modulated signal mt cosωct so that there is no need to generate a carrier at the receiver In this case the transmitter needs to transmit much larger power a rather expensive procedure In pointtopoint communications where there is one transmitter for each receiver substantial complexity in the receiver system can be justified provided there is a large enough saving in expensive highpower transmitting equipment On the other hand for a broadcast system with a multitude of receivers for each transmitter it is more economical to have one expensive highpower transmitter and simpler less expensive receivers The second option transmitting a carrier along with the modulated signal is the obvious choice in this case This is amplitude modulation AM in which the transmitted signal ϕAMt is given by ϕAMt Acos ωct mtcosωct A mtcosωct 750 Recall that the DSBSC signal is mt cos ωct From Eq 750 it follows that the AM signal is identical to the DSBSC signal with Amt as the modulating signal instead of mt Therefore to sketch ϕAMt we sketch A mt and A mt as the envelopes and fill in between with the sinusoid of the carrier frequency Two cases are considered in Fig 739 In the first case A is large enough so that A mt 0 is nonnegative for all values of t In the second case A is not large enough to satisfy this condition In the first case the envelope Fig 739d has the same shape as mt although riding on a dc of magnitude A In the second case the envelope shape is not mt for some parts get rectified Fig 739e Thus we can detect the desired signal mt by detecting the envelope in the first case In the second case such a detection is not possible We shall see that envelope detection is an extremely simple and inexpensive operation which does not require generation of a local carrier for the demodulation But as just noted the envelope of AM has the information about mt only if the AM signal Amtcos ωct satisfies the condition A mt 0 for all t Thus the condition for envelope detection of an AM signal is A mt 0 for allt 751 If mp is the peak amplitude positive or negative of mt then Eq 751 is equivalent to A mp Thus the minimum carrier amplitude required for the viability of envelope detection is mp This point is clearly illustrated in Fig 739 We define the modulation index μ as μ mp A 752 where A is the carrier amplitude Note that mp is a constant of the signal mt Because A mp and because there is no upper bound on A it follows that 0 μ 1 07LathiC07 2017925 1917 page 743 64 77 Application to Communications Amplitude Modulation 743 Figure 739 An AM signal a for two values of A b c and the respective envelopes d e as the required condition for the viability of demodulation of AM by an envelope detector When A mp Eq 752 shows that μ 1 overmodulation shown in Fig 739e In this case the option of envelope detection is no longer viable We then need to use synchronous demodulation Note that synchronous demodulation can be used for any value of μ see Prob 777 The envelope detector which is considerably simpler and less expensive than the synchronous detector can be used only when μ 1 EXAMPLE 723 Amplitude Modulation Sketch ϕAMt for modulation indices of μ 05 50 modulation and μ 1 100 modulation when mt Bcos ωmt This case is referred to as tone modulation because the modulating signal is a pure sinusoid or tone 07LathiC07 2017925 1917 page 747 68 77 Application to Communications Amplitude Modulation 747 as singlesideband SSB transmission which requires only half the bandwidth of the DSB signal Thus we transmit only the upper sidebands Fig 742c or only the lower sidebands Fig 742d An SSB signal can be coherently synchronously demodulated For example multiplication of a USB signal Fig 742c by 2cos ωct shifts its spectrum to the left and to the right by ωc yielding the spectrum in Fig 742e Lowpass filtering of this signal yields the desired baseband signal The case is similar with an LSB signal Hence demodulation of SSB signals is identical to that of DSBSC signals and the synchronous demodulator in Fig 738a can demodulate SSB signals Note that we are talking of SSB signals without an additional carrier Hence they are suppressedcarrier signals SSBSC EXAMPLE 724 SingleSideband Modulation Find the USB upper sideband and LSB lower sideband signals when mt cos ωmt Sketch their spectra and show that these SSB signals can be demodulated using the synchronous demodulator in Fig 738a The DSBSC signal for this case is ϕDSBSCt mtcos ωct cos ωmtcos ωct 1 2cosωc ωmt cosωc ωmt As pointed out in Ex 721 the terms 12cosωc ωmt and 12cosωc ωmt represent the upper and lower sidebands respectively The spectra of the upper and lower sidebands are given in Figs 743a and 743b Observe that these spectra can be obtained from the DSBSC spectrum in Fig 737b by using a proper filter to suppress the undesired sidebands For instance the USB signal in Fig 743a can be obtained by passing the DSBSC signal Fig 737b through a highpass filter of cutoff frequency ωc Similarly the LSB signal in Fig 743b can be obtained by passing the DSBSC signal through a lowpass filter of cutoff frequency ωc If we apply the LSB signal 12cosωc ωmt to the synchronous demodulator in Fig 738a the multiplier output is et 1 2 cosωc ωmtcos ωct 1 4cos ωmt cos2ωc ωmt The term 14cos2ωcωmt is suppressed by the lowpass filter producing the desired output 14cos ωmt which is mt4 The spectrum of this term is πδω ωmδω ωm4 as depicted in Fig 743c In the same way we can show that the USB signal can be demodulated by the synchronous demodulator In the frequency domain demodulation multiplication by cos ωct amounts to shifting the LSB spectrum Fig 743b to the left and the right by ωc times 05 and then suppressing the high frequency as illustrated in Fig 743c The resulting spectrum represents the desired signal 14mt 07LathiC07 2017925 1917 page 749 70 78 Data Truncation Window Functions 749 200 3200 0 fHz Relative power Figure 744 Voice spectrum at low frequencies around ω 0 SSB techniques cause considerable distortion Such is the case with video signals Consequently for video signals instead of SSB we use another technique the vestigial sideband VSB which is a compromise between SSB and DSB It inherits the advantages of SSB and DSB but avoids their disadvantages at a cost of slightly increased bandwidth VSB signals are relatively easy to generate and their bandwidth is only slightly typically 25 greater than that of SSB signals In VSB signals instead of rejecting one sideband completely as in SSB we accept a gradual cutoff from one sideband 4 774 FrequencyDivision Multiplexing Signal multiplexing allows transmission of several signals on the same channel Later in Ch 8 Sec 822 we shall discuss timedivision multiplexing TDM where several signals timeshare the same channel such as a cable or an optical fiber In frequencydivision multiplexing FDM the use of modulation as illustrated in Fig 745 makes several signals share the band of the same channel Each signal is modulated by a different carrier frequency The various carriers are adequately separated to avoid overlap or interference between the spectra of various modulated signals These carriers are referred to as subcarriers Each signal may use a different kind of modulation for example DSBSC AM SSBSC VSBSC or even other forms of modulation not discussed here such as FM frequency modulation or PM phase modulation The modulatedsignal spectra may be separated by a small guard band to avoid interference and to facilitate signal separation at the receiver When all the modulated spectra are added we have a composite signal that may be considered to be a new baseband signal Sometimes this composite baseband signal may be used to further modulate a highfrequency radio frequency or RF carrier for the purpose of transmission At the receiver the incoming signal is first demodulated by the RF carrier to retrieve the composite baseband which is then bandpassfiltered to separate the modulated signals Then each modulated signal is individually demodulated by an appropriate subcarrier to obtain all the basic baseband signals 78 DATA TRUNCATION WINDOW FUNCTIONS We often need to truncate data in diverse situations from numerical computations to filter design For example if we need to compute numerically the Fourier transform of some signal say etut we will have to truncate the signal etut beyond a sufficiently large value of t typically five time constants and above The reason is that in numerical computations we have to deal with 07LathiC07 2017925 1917 page 751 72 78 Data Truncation Window Functions 751 by adding the first n harmonics and truncating all the higher harmonics These examples show that data truncation can occur in both time and frequency domains On the surface truncation appears to be a simple problem of cutting off the data at a point at which values are deemed to be sufficiently small Unfortunately this is not the case Simple truncation can cause some unsuspected problems WINDOW FUNCTIONS Truncation operation may be regarded as multiplying a signal of a large width by a window function of a smaller finite width Simple truncation amounts to using a rectangular window wRt shown later in Fig 748a in which we assign unit weight to all the data within the window width t T2 and assign zero weight to all the data lying outside the window t T2 It is also possible to use a window in which the weight assigned to the data within the window may not be constant In a triangular window wTt for example the weight assigned to data decreases linearly over the window width shown later in Fig 748b Consider a signal xt and a window function wt If xt Xω and wt Wω and if the windowed function xwt Xwω then xwt xtwt and Xwω 1 2π XωWω According to the width property of convolution it follows that the width of Xwω equals the sum of the widths of Xω and Wω Thus truncation of a signal increases its bandwidth by the amount of bandwidth of wt Clearly the truncation of a signal causes its spectrum to spread or smear by the amount of the bandwidth of wt Recall that the signal bandwidth is inversely proportional to the signal duration width Hence the wider the window the smaller its bandwidth and the smaller the spectral spreading This result is predictable because a wider window means that we are accepting more data closer approximation which should cause smaller distortion smaller spectral spreading Smaller window width poorer approximation causes more spectral spreading more distortion In addition since Wω is really not strictly bandlimited and its spectrum 0 only asymptotically the spectrum of Xwω 0 asymptotically also at the same rate as that of Wω even if Xω is in fact strictly bandlimited Thus windowing causes the spectrum of Xω to spread into the band where it is supposed to be zero This effect is called leakage The following example clarifies these twin effects of spectral spreading and leakage Let us consider xt cos ω0t and a rectangular window wRt recttT illustrated in Fig 746b The reason for selecting a sinusoid for xt is that its spectrum consists of spectral lines of zero width Fig 746a Hence this choice will make the effect of spectral spreading and leakage easily discernible The spectrum of the truncated signal xwt is the convolution of the two impulses of Xω with the sinc spectrum of the window function Because the convolution of any function with an impulse is the function itself shifted at the location of the impulse the resulting spectrum of the truncated signal is 12π times the two sinc pulses at ω0 as depicted in Fig 746c also see Fig 726 Comparison of spectra Xω and Xwω reveals the effects of truncation These are 1 The spectral lines of Xω have zero width But the truncated signal is spread out by 2πT about each spectral line The amount of spread is equal to the width of the mainlobe of the window spectrum One effect of this spectral spreading or smearing is that if xt has two spectral components of frequencies differing by less than 4πT rads 2T Hz they 07LathiC07 2017925 1917 page 753 74 78 Data Truncation Window Functions 753 other hand the truncated signal spectrum Xwω is zero nowhere because of the sidelobes These sidelobes decay asymptotically as 1ω Thus the truncation causes spectral leakage in the band where the spectrum of the signal xt is zero The peak sidelobe magnitude is 0217 times the mainlobe magnitude 133 dB below the peak mainlobe magnitude Also the sidelobes decay at a rate 1ω which is 6 dBoctave or 20 dBdecade This is the sidelobes rolloff rate We want smaller sidelobes with a faster rate of decay high rolloff rate Figure 746d which plots WRω as a function of ω clearly shows the mainlobe and sidelobe features with the first sidelobe amplitude 133 dB below the mainlobe amplitude and the sidelobes decaying at a rate of 6 dBoctave or 20 dBdecade So far we have discussed the effect on the signal spectrum of signal truncation truncation in the time domain Because of the timefrequency duality the effect of spectral truncation truncation in frequency domain on the signal shape is similar REMEDIES FOR SIDE EFFECTS OF TRUNCATION For better results we must try to minimize the twin side effects of truncations spectral spreading mainlobe width and leakage sidelobe Let us consider each of these ills 1 The spectral spread mainlobe width of the truncated signal is equal to the bandwidth of the window function wt We know that the signal bandwidth is inversely proportional to the signal width duration Hence to reduce the spectral spread mainlobe width we need to increase the window width 2 To improve the leakage behavior we must search for the cause of the slow decay of sidelobes In Ch 6 we saw that the Fourier spectrum decays as 1ω for a signal with jump discontinuity decays as 1ω2 for a continuous signal whose first derivative is discontinuous and so on Smoothness of a signal is measured by the number of continuous derivatives it possesses The smoother the signal the faster the decay of its spectrum Thus we can achieve a given leakage behavior by selecting a suitably smooth tapered window 3 For a given window width the remedies for the two effects are incompatible If we try to improve one the other deteriorates For instance among all the windows of a given width the rectangular window has the smallest spectral spread mainlobe width but its sidelobes have high level and they decay slowly A tapered smooth window of the same width has smaller and faster decaying sidelobes but it has a wider mainlobe But we can compensate for the increased mainlobe width by widening the window Thus we can remedy both the side effects of truncation by selecting a suitably smooth window of sufficient width There are several wellknown taperedwindow functions such as Bartlett triangular Hanning von Hann Hamming Blackman and Kaiser which truncate the data gradually These This result was demonstrated for periodic signals However it applies to aperiodic signals also This is because we showed in the beginning of this chapter that if xT0t is a periodic signal formed by periodic extension of an aperiodic signal xt then the spectrum of xT0t is 1T0 times the samples of Xω Thus what is true of the decay rate of the spectrum of xT0t is also true of the rate of decay of Xω A tapered window yields a higher mainlobe width because the effective width of a tapered window is smaller than that of the rectangular window see Sec 262 Eq 247 for the definition of effective width Therefore from the reciprocity of the signal width and its bandwidth it follows that the rectangular window mainlobe is narrower than a tapered window 07LathiC07 2017925 1917 page 755 76 79 MATLAB Fourier Transform Topics 755 triangle window also called the Fejer or Cesaro is inferior in all respects to the Hanning window For this reason it is rarely used in practice Hanning is preferred over Hamming in spectral analysis because it has faster sidelobe decay For filtering applications on the other hand the Hamming window is chosen because it has the smallest sidelobe magnitude for a given mainlobe width The Hamming window is the most widely used generalpurpose window The Kaiser window which uses I0α the modified zeroorder Bessel function is more versatile and adjustable Selecting a proper value of α 0 α 10 allows the designer to tailor the window to suit a particular application The parameter α controls the mainlobesidelobe tradeoff When α 0 the Kaiser window is the rectangular window For α 54414 it is the Hamming window and when α 8885 it is the Blackman window As α increases the mainlobe width increases and the sidelobe level decreases 781 Using Windows in Filter Design We shall design an ideal lowpass filter of bandwidth W rads with frequency response Hω as shown in Fig 748e or Fig 748f For this filter the impulse response ht WπsincWt Fig 748c is noncausal and therefore unrealizable Truncation of ht by a suitable window Fig 748a makes it realizable although the resulting filter is now an approximation to the desired ideal filter We shall use a rectangular window wRt and a triangular Bartlett window wTt to truncate ht and then examine the resulting filters The truncated impulse responses hRt htwRt and hTt htwTt are depicted in Fig 748d Hence the windowed filter frequency response is the convolution of Hω with the Fourier transform of the window as illustrated in Figs 748e and 748f We make the following observations 1 The windowed filter spectra show spectral spreading at the edges and instead of a sudden switch there is a gradual transition from the passband to the stopband of the filter The transition band is smaller 2πT rads for the rectangular case than for the triangular case 4πT rads 2 Although Hω is bandlimited the windowed filters are not But the stopband behavior of the triangular case is superior to that of the rectangular case For the rectangular window the leakage in the stopband decreases slowly as 1ω in comparison to that of the triangular window as 1ω2 Moreover the rectangular case has a higher peak sidelobe amplitude than that of the triangular window 79 MATLAB FOURIER TRANSFORM TOPICS MATLAB is useful for investigating a variety of Fourier transform topics In this section a rectangular pulse is used to investigate the scaling property Parsevals theorem essential bandwidth and spectral sampling Kaiser window functions are also investigated In addition to truncation we need to delay the truncated function by T2 to render it causal However the time delay only adds a linear phase to the spectrum without changing the amplitude spectrum Thus to simplify our discussion we shall ignore the delay 07LathiC07 2017925 1917 page 757 78 79 MATLAB Fourier Transform Topics 757 791 The Sinc Function and the Scaling Property As shown in Ex 72 the Fourier transform of xt recttτ is Xω τ sincωτ2 To represent Xω in MATLAB a sinc function is first required As an alternative to the signal processing toolbox function sinc which computes sincx as sinπxπx we create our own function that follows the conventions of this book and defines sincx sinxx function y CH7MP1x CH7MP1m Chapter 7 MATLAB Program 1 Function Mfile computes the sinc function y sinxx yx0 1 yx0 sinxx0xx0 The computational simplicity of sinc x sinxx is somewhat deceptive sin00 results in a dividebyzero error Thus program CH7MP1 assigns sinc 0 1 and computes the remaining values according to the definition Notice that CH7MP1 cannot be directly replaced by an anonymous function Anonymous functions cannot have multiple lines or contain certain commands such as if or for Mfiles however can be used to define an anonymous function For example we can represent Xω as an anonymous function that is defined in terms of CH7MP1 X omegatau tauCH7MP1omegatau2 Once we have defined Xω it is simple to investigate the effects of scaling the pulse width τ Consider the three cases τ 10 τ 05 and τ 20 omega linspace4pi4pi200 plotomegaXomega1komegaXomega05komegaXomega2k grid axis tight xlabelomega ylabelXomega legendBaseline au 1Compressed au 05 Expanded au 20 Figure 749 confirms the reciprocal relationship between signal duration and spectral bandwidth time compression causes spectral expansion and time expansion causes spectral compression Additionally spectral amplitudes are directly related to signal energy As a signal is compressed signal energy and thus spectral magnitude decrease The opposite effect occurs when the signal is expanded 10 5 ω 0 5 10 0 1 2 Xω τ 1 τ 05 τ 20 Figure 749 Spectra Xω τ sincωτ2 for τ 10 τ 05 and τ 20 07LathiC07 2017925 1917 page 759 80 79 MATLAB Fourier Transform Topics 759 W Wstep end EW 12piquadXsquaredWWtau relerr E EWE end Although this guessandcheck method is not the most efficient it is relatively simple to understand CH7MP2 sensibly adjusts W until the relative error is within tolerance The number of iterations needed to converge to a solution depends on a variety of factors and is not known beforehand The while command is ideal for such situations while expression statements end While the expression is true the statements are continually repeated To demonstrate CH7MP2 consider the 90 essential bandwidth W for a pulse of 1 second duration Typing WEWCH7MP21090001 returns an essential bandwidth W 53014 that contains 8997 of the energy Reducing the error tolerance improves the estimate CH7MP2109000005 returns an essential bandwidth W 53321 that contains 9000 of the energy These essential bandwidth calculations are consistent with estimates presented after Ex 72 793 Spectral Sampling Consider a signal with finite duration τ A periodic signal xT0t is constructed by repeating xt every T0 seconds where T0 τ From Eq 75 we can write the Fourier series coefficients of xT0t as Dn 1T0Xn2πT0 Put another way the Fourier series coefficients are obtained by sampling the spectrum Xω By using spectral sampling it is simple to determine the Fourier series coefficients for an arbitrary dutycycle squarepulse periodic signal The square pulse xt recttτ has spectrum Xω τ sincωτ2 Thus the nth Fourier coefficient of the periodic extension xT0t is Dn τT0sincnπτT0 As in Ex 64 τ π and T0 2π provide a squarepulse periodic signal The Fourier coefficients are determined by tau pi T0 2pi n 010 Dn tauT0MS7P1npitauT0 stemnDn xlabeln ylabelDn axis05 105 02 055 The results shown in Fig 750 agree with Fig 66b Doubling the period to T0 4π effectively doubles the density of spectral samples and halves the spectral amplitude as shown in Fig 751 As T0 increases the spectral sampling becomes progressively finer while the amplitude becomes infinitesimal An evolution of the Fourier series toward the Fourier integral is seen by allowing the period T0 to become large Figure 752 shows the result for T0 40π If T0 τ the signal xT0 is a constant and the spectrum should concentrate energy at dc In this case the sinc function is sampled at the zero crossings and Dn 0 for all n not equal to 0 Only the sample corresponding to n 0 is nonzero indicating a dc signal as expected It is a simple matter to modify the previous code to verify this case 07LathiC07 2017925 1917 page 762 83 762 CHAPTER 7 CONTINUOUSTIME SIGNAL ANALYSIS THE FOURIER TRANSFORM 06 04 02 0 02 04 t 0 05 1 wKt Rectangular Hamming Blackman Figure 753 Specialcase unitduration Kaiser windows Figure 753 shows the three specialcase unitduration Kaiser windows generated by t 0600106 T 1 plottCH7MP3tTrktCH7MP3tThamktCH7MP3tTbk axis06 06 1 11 xlabelt ylabelwKt legendRectangularHammingBlackmanLocationEastOutside 710 SUMMARY In Ch 6 we represented periodic signals as a sum of everlasting sinusoids or exponentials Fourier series In this chapter we extended this result to aperiodic signals which are represented by the Fourier integral instead of the Fourier series An aperiodic signal xt may be regarded as a periodic signal with period T0 so that the Fourier integral is basically a Fourier series with a fundamental frequency approaching zero Therefore for aperiodic signals the Fourier spectra are continuous This continuity means that a signal is represented as a sum of sinusoids or exponentials of all frequencies over a continuous frequency interval The Fourier transform Xω therefore is the spectral density per unit bandwidth in hertz An everpresent aspect of the Fourier transform is the duality between time and frequency which also implies duality between the signal xt and its transform Xω This duality arises because of nearsymmetrical equations for direct and inverse Fourier transforms The duality principle has farreaching consequences and yields many valuable insights into signal analysis The scaling property of the Fourier transform leads to the conclusion that the signal bandwidth is inversely proportional to signal duration signal width Time shifting of a signal does not change its amplitude spectrum but it does add a linear phase component to its spectrum Multiplication of a signal by an exponential ejω0t shifts the spectrum to the right by ω0 In practice spectral shifting is achieved by multiplying a signal by a sinusoid such as cosω0t rather than the exponential ejω0t This process is known as amplitude modulation Multiplication of two signals results in convolution of their spectra whereas convolution of two signals results in multiplication of their spectra For an LTIC system with the frequency response Hω the input and output spectra Xω and Yω are related by the equation Yω XωHω This is valid only for asymptotically stable systems It also applies to marginally stable systems if the input does not contain a finiteamplitude 08LathiC08 2017925 1554 page 776 1 C H A P T E R SAMPLING THE BRIDGE FROM CONTINUOUS TO DISCRETE 8 A continuoustime signal can be processed by applying its samples through a discretetime system For this purpose it is important to maintain the signal sampling rate high enough to permit the reconstruction of the original signal from these samples without error or with an error within a given tolerance The necessary quantitative framework for this purpose is provided by the sampling theorem derived in Sec 81 Sampling theory is the bridge between the continuoustime and discretetime worlds The information inherent in a sampled continuoustime signal is equivalent to that of a discretetime signal A sampled continuoustime signal is a sequence of impulses while a discretetime signal presents the same information as a sequence of numbers These are basically two different ways of presenting the same data Clearly all the concepts in the analysis of sampled signals apply to discretetime signals We should not be surprised to see that the Fourier spectra of the two kinds of signal are also the same within a multiplicative constant 81 THE SAMPLING THEOREM We now show that a real signal whose spectrum is bandlimited to B Hz Xω 0 for ω 2πB can be reconstructed exactly without any error from its samples taken uniformly at a rate fs 2B samples per second In other words the minimum sampling frequency is fs 2B Hz To prove the sampling theorem consider a signal xt Fig 81a whose spectrum is bandlimited to B Hz Fig 81b For convenience spectra are shown as functions of ω as well as of f hertz Sampling xt at a rate of fs Hz fs samples per second can be accomplished by multiplying xt by an impulse train δTt Fig 81c consisting of unit impulses repeating periodically every T seconds where T 1fs The schematic of a sampler is shown in Fig 81d The resulting sampled signal xt is shown in Fig 81e The sampled signal consists of impulses The theorem stated here and proved subsequently applies to lowpass signals A bandpass signal whose spectrum exists over a frequency band fc B2 f fc B2 has a bandwidth of B Hz Such a signal is uniquely determined by 2B samples per second In general the sampling scheme is a bit more complex in this case It uses two interlaced sampling trains each at a rate of B samples per second See for example 1 The spectrum Xω in Fig 81b is shown as real for convenience However our arguments are valid for complex Xω as well 776 08LathiC08 2017925 1554 page 781 6 81 The Sampling Theorem 781 from Xω using an ideal lowpass filter of bandwidth 5 Hz Fig 82f Finally in the last case of oversampling sampling rate 20 Hz the spectrum Xω consists of nonoverlapping repetitions of 1TXω repeating every 20 Hz with empty bands between successive cycles Fig 82h Hence Xω can be recovered from Xω by using an ideal lowpass filter or even a practical lowpass filter shown dashed in Fig 82h DRILL 81 Nyquist Sampling Find the Nyquist rate and the Nyquist sampling interval for the signals sinc100πt and sinc100πt sinc50πt ANSWERS The Nyquist sampling interval is 001 s and the Nyquist sampling rate is 100 Hz for both signals FOR SKEPTICS ONLY Rare is the reader who at first encounter is not skeptical of the sampling theorem It seems impossible that Nyquist samples can define the one and the only signal that passes through those sample values We can easily picture infinite number of signals passing through a given set of samples However among all these infinite number of signals only one has the minimum bandwidth B 12T Hz where T is the sampling interval See Prob 8215 To summarize for a given set of samples taken at a rate fs Hz there is only one signal of bandwidth B fs2 that passes through those samples All other signals that pass through those samples have bandwidth higher than fs2 and the samples are subNyquist rate samples for those signals 811 Practical Sampling In proving the sampling theorem we assumed ideal samples obtained by multiplying a signal xt by an impulse train that is physically unrealizable In practice we multiply a signal xt by a train of pulses of finite width depicted in Fig 83c The sampler is shown in Fig 83d The sampled signal xt is illustrated in Fig 83e We wonder whether it is possible to recover or reconstruct xt from this xt Surprisingly the answer is affirmative provided the sampling rate is not below the Nyquist rate The signal xt can be recovered by lowpass filtering xt as if it were sampled by impulse train The filter should have a constant gain between 0 and 5 Hz and zero gain beyond 10 Hz In practice the gain beyond 10 Hz can be made negligibly small but not zero 08LathiC08 2017925 1554 page 788 13 788 CHAPTER 8 SAMPLING THE BRIDGE FROM CONTINUOUS TO DISCRETE where the sampling interval T is the Nyquist interval for xt that is T 12B Because we are given the Nyquist sample values we use the interpolation formula of Eq 86 to construct xt from its samples Since all but one of the Nyquist samples are zero only one term corresponding to n 0 in the summation on the righthand side of Eq 86 survives Thus xt sinc2πBt This signal is illustrated in Fig 86b Observe that this is the only signal that has a bandwidth B Hz and the sample values x0 1 and xnT 0n 0 No other signal satisfies these conditions 821 Practical Difficulties in Signal Reconstruction Consider the signal reconstruction procedure illustrated in Fig 87a If xt is sampled at the Nyquist rate fs 2B Hz the spectrum Xω consists of repetitions of Xω without any gap between successive cycles as depicted in Fig 87b To recover xt from xt we need to pass the sampled signal xt through an ideal lowpass filter shown dotted in Fig 87b As seen in Sec 75 such a filter is unrealizable it can be closely approximated only with infinite time delay in the response In other words we can recover the signal xt from its samples with infinite time delay A practical solution to this problem is to sample the signal at a rate higher than the Nyquist rate fs 2B or ωs 4πB The result is Xω consisting of repetitions of Xω with a finite bandgap between successive cycles as illustrated in Fig 87c Now we can recover Xω from Xω using a lowpass filter with a gradual cutoff characteristic shown dotted in Fig 87c But even in this case if the unwanted spectrum is to be suppressed the filter gain must be zero beyond some frequency see Fig 87c According to the PaleyWiener criterion Eq 743 it is impossible to realize even this filter The only advantage in this case is that the required filter can be closely approximated with a smaller time delay All this means that it is impossible in practice to recover a bandlimited signal xt exactly from its samples even if the sampling rate is higher than the Nyquist rate However as the sampling rate increases the recovered signal approaches the desired signal more closely THE TREACHERY OF ALIASING There is another fundamental practical difficulty in reconstructing a signal from its samples The sampling theorem was proved on the assumption that the signal xt is bandlimited All practical signals are timelimited that is they are of finite duration or width We can demonstrate see Prob 8220 that a signal cannot be timelimited and bandlimited simultaneously If a signal is timelimited it cannot be bandlimited and vice versa but it can be simultaneously nontimelimited and nonbandlimited Clearly all practical signals which are necessarily timelimited are nonbandlimited as shown in Fig 88a they have infinite bandwidth and the spectrum Xω consists of overlapping cycles of Xω repeating every fs Hz the sampling frequency as 08LathiC08 2017925 1554 page 789 14 82 Signal Reconstruction 789 c 2pB vs vs b v v a Ideal lowpass filter cutoff B Hz Sampler dTt xt xt Xv Xv xt Figure 87 a Signal reconstruction from its samples b Spectrum of a signal sampled at the Nyquist rate c Spectrum of a signal sampled above the Nyquist rate illustrated in Fig 88b Because of infinite bandwidth in this case the spectral overlap is unavoidable regardless of the sampling rate Sampling at a higher rate reduces but does not eliminate overlapping between repeating spectral cycles Because of the overlapping tails Xω no longer has complete information about Xω and it is no longer possible even theoretically to recover xt exactly from the sampled signal xt If the sampled signal is passed through an ideal lowpass filter of cutoff frequency fs2 Hz the output is not Xω but Xaω Fig 88c which is a version of Xω distorted as a result of two separate causes 1 The loss of the tail of Xω beyond f fs2 Hz 2 The reappearance of this tail inverted or folded onto the spectrum Note that the spectra cross at frequency fs2 12T Hz This frequency is called the folding frequency Figure 88b shows that from the infinite number of repeating cycles only the neighboring spectral cycles overlap This is a somewhat simplified picture In reality all the cycles overlap and interact with every other cycle because of the infinite width of all practical signal spectra Fortunately all practical spectra also must decay at higher frequencies This results in insignificant amount of interference from cycles other than the immediate neighbors When such an assumption is not justified aliasing computations become little more involved 08LathiC08 2017925 1554 page 791 16 82 Signal Reconstruction 791 The spectrum may be viewed as if the lost tail is folding back onto itself at the folding frequency For instance a component of frequency fs2 fz shows up as or impersonates a component of lower frequency fs2 fz in the reconstructed signal Thus the components of frequencies above fs2 reappear as components of frequencies below fs2 This tail inversion known as spectral folding or aliasing is shown shaded in Fig 88b and also in Fig 88c In the process of aliasing not only are we losing all the components of frequencies above the folding frequency fs2 Hz but these very components reappear aliased as lowerfrequency components as shown in Figs 88b and 88c Such aliasing destroys the integrity of the frequency components below the folding frequency fs2 as depicted in Fig 88c The aliasing problem is analogous to that of an army with a platoon that has secretly defected to the enemy side The platoon is however ostensibly loyal to the army The army is in double jeopardy First the army has lost this platoon as a fighting force In addition during actual fighting the army will have to contend with sabotage by the defectors and will have to find another loyal platoon to neutralize the defectors Thus the army has lost two platoons in nonproductive activity DEFECTORS ELIMINATED THE ANTIALIASING FILTER If you were the commander of the betrayed army the solution to the problem would be obvious As soon as the commander got wind of the defection he would incapacitate by whatever means the defecting platoon before the fighting begins This way he loses only one the defecting platoon This is a partial solution to the double jeopardy of betrayal and sabotage a solution that partly rectifies the problem and cuts the losses to half We follow exactly the same procedure The potential defectors are all the frequency components beyond the folding frequency fs2 12T Hz We should eliminate suppress these components from xt before sampling xt Such suppression of higher frequencies can be accomplished by an ideal lowpass filter of cutoff fs2 Hz as shown in Fig 88d This is called the antialiasing filter Figure 88d also shows that antialiasing filtering is performed before sampling Figure 88e shows the sampled signal spectrum dotted and the reconstructed signal Xaaω when an antialiasing scheme is used An antialiasing filter essentially bandlimits the signal xt to fs2 Hz This way we lose only the components beyond the folding frequency fs2 Hz These suppressed components now cannot reappear to corrupt the components of frequencies below the folding frequency Clearly use of an antialiasing filter results in the reconstructed signal spectrum Xaaω Xω for f fs2 Thus although we lost the spectrum beyond fs2 Hz the spectrum for all the frequencies below fs2 remains intact The effective aliasing distortion is cut in half owing to elimination of folding We stress again that the antialiasing operation must be performed before the signal is sampled An antialiasing filter also helps to reduce noise Noise generally has a wideband spectrum and without antialiasing the aliasing phenomenon itself will cause the noise lying outside the desired band to appear in the signal band Antialiasing suppresses the entire noise spectrum beyond frequency fs2 The antialiasing filter being an ideal filter is unrealizable In practice we use a steep cutoff filter which leaves a sharply attenuated spectrum beyond the folding frequency fs2 08LathiC08 2017925 1554 page 793 18 82 Signal Reconstruction 793 This discussion again shows that sampling a sinusoid of frequency f aliasing can be avoided if the sampling rate fs 2f Hz 0 f fs 2 or 0 ω π T Violating this condition leads to aliasing implying that the samples appear to be those of a lowerfrequency signal Because of this loss of identity it is impossible to reconstruct the signal faithfully from its samples GENERAL CONDITION FOR ALIASING IN SINUSOIDS We can generalize the foregoing result by showing that samples of a sinusoid of frequency f0 are identical to those of a sinusoid of frequency f0 mfs Hz integer m where fs is the sampling frequency The samples of cos2πf0 mfst are cos 2πf0 mfsnT cos2πf0nT 2πmn cos 2πf0nT The result follows because mn is an integer and fsT 1 This result shows that sinusoids of frequencies that differ by an integer multiple of fs result in identical set of samples In other words samples of sinusoids separated by frequency fs Hz are identical This implies that samples of sinusoids in any frequency band of fs Hz are unique that is no two sinusoids in that band have the same samples when sampled at a rate fs Hz For instance frequencies in the band from fs2 to fs2 have unique samples at the sampling rate fs This band is called the fundamental band Recall also that fs2 is the folding frequency From the discussion thus far we conclude that if a continuoustime sinusoid of frequency f Hz is sampled at a rate of fs Hz sampless the resulting samples would appear as samples of a continuoustime sinusoid of frequency fa in the fundamental band where fa f mfs fs 2 fa fs 2 m an integer 87 The frequency fa lies in the fundamental band from fs2 to fs2 Figure 89a shows the plot of fa versus f where f is the actual frequency and fa is the corresponding fundamental band frequency whose samples are identical to those of the sinusoid of frequency f when the sampling rate is fs Hz Recall however that the sign change of a frequency does not alter the actual frequency of the waveform This is because cosωat θ cosωat θ Clearly the apparent frequency of a sinusoid of frequency fa is also fa However its phase undergoes a sign change This means the apparent frequency of any sampled sinusoid lies in the range from 0 to fs2 Hz To summarize if a continuoustime sinusoid of frequency f Hz is sampled at a rate of fs Hz samplessecond the resulting samples would appear as samples of a continuoustime sinusoid of frequency fa that lies in the band from 0 to fs2 According to Eq 87 fa f mfs fa fs 2 m an integer 08LathiC08 2017925 1554 page 796 21 796 CHAPTER 8 SAMPLING THE BRIDGE FROM CONTINUOUS TO DISCRETE d Here f 2400 Hz can be expressed as 2400 400 2 1000 so that fa 400 Hence the aliased frequency is 400 Hz and there is no sign change for the phase The apparent sinusoid is cos2πft θ with f 400 We could have found these answers directly from Fig 89b For example for case b we read fa 400 corresponding to f 600 Moreover f 600 lies in the shaded belt Hence there is a phase sign change DRILL 83 A Case of Identical Sampled Sinusoids Show that samples of 90 Hz and 110 Hz sinusoids of the form cosωt are identical when sampled at a rate 200 Hz DRILL 84 Apparent Frequency of Sampled Sinusoids A sinusoid of frequency f0 Hz is sampled at a rate of 100 Hz Determine the apparent frequency of the samples if f0 is a 40 Hz b 60 Hz c 140 Hz and d 160 Hz ANSWERS All four cases have an apparent frequency of 40 Hz 822 Some Applications of the Sampling Theorem The sampling theorem is very important in signal analysis processing and transmission because it allows us to replace a continuoustime signal with a discrete sequence of numbers Processing a continuoustime signal is therefore equivalent to processing a discrete sequence of numbers Such processing leads us directly into the area of digital filtering In the field of communication the transmission of a continuoustime message reduces to the transmission of a sequence of numbers by means of pulse trains The continuoustime signal xt is sampled and sample values are used to modify certain parameters of a periodic pulse train We may vary the amplitudes Fig 811b widths Fig 811c or positions Fig 811d of the pulses in proportion to the sample values of the signal xt Accordingly we may have pulseamplitude modulation PAM pulsewidth modulation PWM or pulseposition modulation PPM The most important form of pulse modulation today is pulsecode modulation PCM discussed in Sec 83 in connection with Fig 814b In all these cases instead of transmitting xt we transmit the corresponding pulsemodulated signal At the receiver we read the information of the pulsemodulated signal and reconstruct the analog signal xt 08LathiC08 2017925 1554 page 797 22 82 Signal Reconstruction 797 t t t t a b c Pulse locations are the same but their widths change d Pulse widths are the same but their locations change xt Figure 811 Pulsemodulated signals a The signal b The PAM signal c The PWM PDM signal d The PAM signal One advantage of using pulse modulation is that it permits the simultaneous transmission of several signals on a timesharing basistimedivision multiplexing TDM Because a pulsemodulated signal occupies only a part of the channel time we can transmit several pulsemodulated signals on the same channel by interweaving them Figure 812 shows the TDM of two PAM signals In this manner we can multiplex several signals on the same channel by reducing pulse widths Digital signals also offer an advantage in the area of communications where signals must travel over distances Transmission of digital signals is more rugged than that of analog signals because digital signals can withstand channel noise and distortion much better as long as the noise Another method of transmitting several baseband signals simultaneously is frequencydivision multiplexing FDM discussed in Sec 774 In FDM various signals are multiplexed by sharing the channel bandwidth The spectrum of each message is shifted to a specific band not occupied by any other signal The information of various signals is located in nonoverlapping frequency bands of the channel Fig 745 In a way TDM and FDM are duals of each other 08LathiC08 2017925 1554 page 805 30 85 Numerical Computation of the Fourier Transform 805 85 NUMERICAL COMPUTATION OF THE FOURIER TRANSFORM THE DISCRETE FOURIER TRANSFORM Numerical computation of the Fourier transform of xt requires sample values of xt because a digital computer can work only with discrete data sequence of numbers Moreover a computer can compute Xω only at some discrete values of ω samples of Xω We therefore need to relate the samples of Xω to samples of xt This task can be accomplished by using the results of the two sampling theorems developed in Secs 81 and 84 We begin with a timelimited signal xt Fig 816a and its spectrum Xω Fig 816b Since xt is timelimited Xω is nonbandlimited For convenience we shall show all spectra as functions of the frequency variable f in hertz rather than ω According to the sampling theorem the spectrum Xω of the sampled signal xt consists of Xω repeating every fs Hz where fs 1T as depicted in Fig 816d In the next step the sampled signal in Fig 816c is repeated periodically every T0 seconds as illustrated in Fig 816e According to the spectral sampling theorem such an operation results in sampling the spectrum at a rate of T0 samplesHz This sampling rate means that the samples are spaced at f0 1T0 Hz as depicted in Fig 816f The foregoing discussion shows that when a signal xt is sampled and then periodically repeated the corresponding spectrum is also sampled and periodically repeated Our goal is to relate the samples of xt to the samples of Xω NUMBER OF SAMPLES One interesting observation from Figs 816e and 816f is that N0 the number of samples of the signal in Fig 816e in one period T0 is identical to N 0 the number of samples of the spectrum in Fig 816f in one period fs To see this we notice that N0 T0 T N 0 fs f0 fs 1 T and f0 1 T0 810 Using these relations we see that N0 T0 T fs f0 N 0 ALIASING AND LEAKAGE IN NUMERICAL COMPUTATION Figure 816f shows the presence of aliasing in the samples of the spectrum Xω This aliasing error can be reduced as much as desired by increasing the sampling frequency fs decreasing the sampling interval T 1fs The aliasing can never be eliminated for timelimited xt however because its spectrum Xω is nonbandlimited Had we started with a signal having a bandlimited spectrum Xω there would be no aliasing in the spectrum in Fig 816f Unfortunately such a signal is nontimelimited and its repetition in Fig 816e would result in signal overlapping aliasing in the time domain In this case we shall have to contend with errors in signal There is a multiplying constant 1T for the spectrum in Fig 816d see Eq 82 but this is irrelevant to our discussion here 08LathiC08 2017925 1554 page 811 36 85 Numerical Computation of the Fourier Transform 811 ZERO PADDING DOES NOT IMPROVE ACCURACY OR RESOLUTION Actually we are not observing Xω through a picket fence We are observing a distorted version of Xω resulting from the truncation of xt Hence we should keep in mind that even if the fence were transparent we would see a reality distorted by aliasing Seeing through the picket fence just gives us an imperfect view of the imperfectly represented reality Zero padding only allows us to look at more samples of that imperfect reality It can never reduce the imperfection in what is behind the fence The imperfection which is caused by aliasing can be lessened only by reducing the sampling interval T Observe that reducing T also increases N0 the number of samples and is like increasing the number of pickets while reducing their width But in this case the reality behind the fence is also better dressed and we see more of it EXAMPLE 87 Number of Samples and Frequency Resolution A signal xt has a duration of 2 ms and an essential bandwidth of 10 kHz It is desirable to have a frequency resolution of 100 Hz in the DFT f0 100 Determine N0 To have f0 100 Hz the effective signal duration T0 must be T0 1 f0 1 100 10 ms Since the signal duration is only 2 ms we need zero padding over 8 ms Also B 10000 Hence fs 2B 20000 and T 1fs 50 µs Furthermore N0 fs f0 20000 100 200 The fast Fourier transform FFT algorithm discussed later see Sec 86 is used to compute DFT where it proves convenient although not necessary to select N0 as a power of 2 that is N0 2n n integer Let us choose N0 256 Increasing N0 from 200 to 256 can be used to reduce aliasing error by reducing T to improve resolution by increasing T0 using zero padding or a combination of both Reducing Aliasing Error We maintain the same T0 so that f0 100 Hence fs N0f0 256 100 25600 and T 1 fs 39µs Thus increasing N0 from 200 to 256 permits us to reduce the sampling interval T from 50 µs to 39 µs while maintaining the same frequency resolution f0 100 Improving Resolution Here we maintain the same T 50 µs which yields T0 N0T 25650 106 128 ms and f0 1 T0 78125 Hz 08LathiC08 2017925 1554 page 812 37 812 CHAPTER 8 SAMPLING THE BRIDGE FROM CONTINUOUS TO DISCRETE Thus increasing N0 from 200 to 256 can improve the frequency resolution from 100 to 78125 Hz while maintaining the same aliasing error T 50 µs Combination of Reducing Aliasing Error and Improving Resolution To simultaneously reduce alias error and improve resolution we could choose T 45 µs and T0 115 ms so that f0 8696 Hz Many other combinations exist as well EXAMPLE 88 DFT to Compute the Fourier Transform of an Exponential Use the DFT to compute samples of the Fourier transform of e2tut Plot the resulting Fourier spectra We first determine T and T0 The Fourier transform of e2tut is 1jω 2 This lowpass signal is not bandlimited In Sec 76 we used the energy criterion to compute the essential bandwidth of a signal Here we shall present a simpler but workable alternative to the energy criterion The essential bandwidth of a signal will be taken as the frequency at which Xω drops to 1 of its peak value see the footnote on page 736 In this case the peak value occurs at ω 0 where X0 05 Observe that Xω 1 ω2 4 1 ω ω 2 Also 1 of the peak value is 001 05 0005 Hence the essential bandwidth B is at ω 2πB where Xω 1 2πB 0005 B 100 π Hz and from Eq 816 T 1 2B π 200 0015708 Had we used 1 energy criterion to determine the essential bandwidth following the procedure in Ex 720 we would have obtained B 2026 Hz which is somewhat smaller than the value just obtained by using the 1 amplitude criterion The second issue is to determine T0 Because the signal is not timelimited we have to truncate it at T0 such that xT0 1 A reasonable choice would be T0 4 because x4 e8 0000335 1 The result is N0 T0T 2546 which is not a power of 2 Hence we choose T0 4 and T 0015625 164 yielding N0 256 which is a power of 2 Note that there is a great deal of flexibility in determining T and T0 depending on the accuracy desired and the computational capacity available We could just as well have chosen T 003125 yielding N0 128 although this choice would have given a slightly higher aliasing error 08LathiC08 2017925 1554 page 814 39 814 CHAPTER 8 SAMPLING THE BRIDGE FROM CONTINUOUS TO DISCRETE In this example we knew Xω beforehand hence we could make intelligent choices for B or the sampling frequency fs In practice we generally do not know Xω beforehand In fact that is the very thing we are trying to determine In such a case we must make an intelligent guess for B or fs from circumstantial evidence We should then continue reducing the value of T and recomputing the transform until the result stabilizes within the desired number of significant digits USING MATLAB TO COMPUTE AND PLOT THE RESULTS Let us now use MATLAB to confirm the results of this example First parameters are defined and MATLABs fft command is used to compute the DFT T0 4 N0 256 T T0N0 t 0TTN01 x Texp2t x1 x12 Xr fftx r N02N021 omegar r2piT0 The true Fourier transform is also computed for comparison omega linspacepiTpiT5001 X 1jomega2 For clarity we display spectrum over a restricted frequency range subplot121 stemomegarfftshiftabsXrk lineomegaabsXcolor0 0 0 axis001 44 001 051 xlabelomega ylabelXomega subplot122 stemomegarfftshiftangleXrk lineomegaangleXcolor0 0 0 axis001 44 pi2001 001 xlabelomega ylabelangle Xomega The results shown in Fig 818 match the earlier results shown in Fig 817 0 01 02 03 04 05 Xω 0 10 20 30 40 ω ω 0 10 20 30 40 15 1 05 0 Xω Figure 818 MATLABcomputed DFT of an exponential signal e2tut 08LathiC08 2017925 1554 page 815 40 85 Numerical Computation of the Fourier Transform 815 EXAMPLE 89 DFT to Compute the Fourier Transform of a Rectangular Pulse Use the DFT to compute the Fourier transform of 8rectt This gate function and its Fourier transform are illustrated in Figs 819a and 819b To determine the value of the sampling interval T we must first decide on the essential bandwidth B In Fig 819b we see that Xω decays rather slowly with ω Hence the essential bandwidth B is rather large For instance at B 155 Hz 9739 rads Xω 01643 which is about 2 of the peak at X0 Hence the essential bandwidth is well above 16 Hz if we use the 1 of the peak amplitude criterion for computing the essential bandwidth However we shall deliberately take B 4 for two reasons to show the effect of aliasing and because the use of B 4 would give an enormous number of samples which could not be conveniently displayed on the page without losing sight of the essentials Thus we shall intentionally accept approximation to graphically clarify the concepts of the DFT The choice of B 4 results in the sampling interval T 12B 18 Looking again at the spectrum in Fig 819b we see that the choice of the frequency resolution f0 14 Hz is reasonable Such a choice gives us four samples in each lobe of Xω In this case T0 1f0 4 seconds and N0 T0T 32 The duration of xt is only 1 second We must repeat it every 4 seconds T0 4 as depicted in Fig 819c and take samples every 18 second This choice yields 32 samples N0 32 Also xn TxnT 1 8xnT Since xt 8rect t the values of xn are 1 0 or 05 at the points of discontinuity as illustrated in Fig 819c where xn is depicted as a function of t as well as n for convenience In the derivation of the DFT we assumed that xt begins at t 0 Fig 816a and then took N0 samples over the interval 0 T0 In the present case however xt begins at 12 This difficulty is easily resolved when we realize that the DFT obtained by this procedure is actually the DFT of xn repeating periodically every T0 seconds Figure 819c clearly indicates that periodic repeating the segment of xn over the interval from 2 to 2 seconds yields the same signal as the periodic repeating the segment of xn over the interval from 0 to 4 seconds Hence the DFT of the samples taken from 2 to 2 seconds is the same as that of the samples taken from 0 to 4 seconds Therefore regardless of where xt starts we can always take the samples of xt and its periodic extension over the interval from 0 to T0 In the present example the 32 sample values are xn 1 0 n 3 and 29 n 31 0 5 n 27 05 n 428 08LathiC08 2017925 1554 page 816 41 816 CHAPTER 8 SAMPLING THE BRIDGE FROM CONTINUOUS TO DISCRETE Figure 819 Discrete Fourier transform of a gate pulse 08LathiC08 2017925 1554 page 818 43 818 CHAPTER 8 SAMPLING THE BRIDGE FROM CONTINUOUS TO DISCRETE xlabelomega ylabelXomega axis tight The result shown in Fig 820 matches the earlier result shown in Fig 819d The DFT approximation does not perfectly follow the true Fourier transform especially at high frequencies because the parameter B is deliberately set too small 25 20 15 10 5 ω 0 5 10 15 20 25 0 2 4 6 8 Xω Figure 820 MATLABcomputed DFT of a gate pulse 851 Some Properties of the DFT The discrete Fourier transform is basically the Fourier transform of a sampled signal repeated periodically Hence the properties derived earlier for the Fourier transform apply to the DFT as well LINEARITY If xn Xr and gn Gr then a1xn a2gn a1Xr a2Gr The proof is trivial CONJUGATE SYMMETRY From the conjugation property xt Xω we have x n X r From this equation and the timereversal property we obtain x n X r 08LathiC08 2017925 1554 page 823 48 85 Numerical Computation of the Fourier Transform 823 that Hr must be repeated every 8 Hz or 16π rads see Fig 822c The resulting 32 samples of Hr over 0 ω 16π are as follows Hr 1 0 r 7 and 25 r 31 0 9 r 23 05 r 824 We multiply Xr with Hr The desired output signal samples yn are found by taking the inverse DFT of XrHr The resulting output signal is illustrated in Fig 822d It is quite simple to verify the results of this filtering example using MATLAB First parameters are defined and MATLABs fft command is used to compute the DFT of xn T0 4 N0 32 T T0N0 n 0N01 r n xn ones14 05 zeros123 05 ones13 Xr fftxn The DFT of the filters output is just the product of the filter response Hr and the input DFT Xr The output yn is obtained using the ifft command and then plotted Hr ones18 05 zeros115 05 ones17 Yr HrXr yn ifftYr clf stemnrealynk xlabeln ylabelyn axis0 31 1 11 The result shown in Fig 823 matches the earlier result shown in Fig 822d Recall this DFTbased approach shows the samples yn of the filter output yt sampled in this case at a rate T 1 8 over 0 n N0 1 31 when the input pulse xt is periodically replicated to form samples xn see Fig 819c 0 5 10 15 20 25 30 n 0 02 04 06 08 1 yn Figure 823 Using MATLAB and the DFT to determine filter output 08LathiC08 2017925 1554 page 827 52 87 MATLAB The Discrete Fourier Transform 827 Thus an N0point DFT can be computed by combining the two N02point DFTs as in Eq 827 These equations can be represented conveniently by the signal flow graph depicted in Fig 824 This structure is known as a butterfly Figure 825a shows the implementation of Eq 824 for the case of N0 8 The next step is to compute the N02point DFTs Gr and Hr We repeat the same procedure by dividing gn and hn into two N04point sequences corresponding to the even and oddnumbered samples Then we continue this process until we reach the onepoint DFT These steps for the case of N0 8 are shown in Figs 825a 825b and 825c Figure 825c shows that the twopoint DFTs require no multiplication To count the number of computations required in the first step assume that Gr and Hr are known Equation 827 clearly shows that to compute all the N0 points of the Xr we require N0 complex additions and N02 complex multiplications corresponding to Wr N0Hr In the second step to compute the N02point DFT Gr from the N04point DFT we require N02 complex additions and N04 complex multiplications We require an equal number of computations for Hr Hence in the second step there are N0 complex additions and N02 complex multiplications The number of computations required remains the same in each step Since a total of log2 N0 steps is needed to arrive at a onepoint DFT we require conservatively a total of N0 log2 N0 complex additions and N02log2 N0 complex multiplications to compute the N0point DFT Actually as Fig 825c shows many multiplications are multiplications by 1 or 1 which further reduces the number of computations The procedure for obtaining IDFT is identical to that used to obtain the DFT except that WN0 ej2πN0 instead of ej2πN0 in addition to the multiplier 1N0 Another FFT algorithm the decimationinfrequency algorithm is similar to the decimationintime algorithm The only difference is that instead of dividing xn into two sequences of even and oddnumbered samples we divide xn into two sequences formed by the first N02 and the last N02 samples proceeding in the same way until a singlepoint DFT is reached in log2 N0 steps The total number of computations in this algorithm is the same as that in the decimationintime algorithm 87 MATLAB THE DISCRETE FOURIER TRANSFORM As an idea the discrete Fourier transform DFT has been known for hundreds of years Practical computing devices however are responsible for bringing the DFT into common use MATLAB is capable of DFT computations that would have been impractical just a few decades ago 871 Computing the Discrete Fourier Transform The MATLAB command fftx computes the DFT of a vector x that is defined over 0 n N0 1 Problem 871 considers how to scale the DFT to accommodate signals that do not begin at n 0 As its name suggests the function fft uses the computationally more efficient fast Fourier transform algorithm when it is appropriate to do so The inverse DFT is easily computed by using the ifft function Actually N02 is a conservative figure because some multiplications corresponding to the cases of Wr N0 1j and so on are eliminated 08LathiC08 2017925 1554 page 828 53 828 CHAPTER 8 SAMPLING THE BRIDGE FROM CONTINUOUS TO DISCRETE To illustrate MATLABs DFT capabilities consider 50 points of a 10 Hz sinusoid sampled at fs 50 Hz and scaled by T 1fs T 150 N0 50 n 0N01 x Tcos2pi10nT In this case the vector x contains exactly 10 cycles of the sinusoid The fft command computes the DFT X fftx Since the DFT is both discrete and periodic fft needs to return only the N0 discrete values contained in the single period 0 f fs While Xr can be plotted as a function of r it is more convenient to plot the DFT as a function of frequency f A frequency vector in hertz is created by using N0 and T f 0N01TN0 stemfabsXk axis0 50 005 055 xlabelf Hz ylabelXf As expected Fig 826 shows content at a frequency of 10 Hz Since the timedomain signal is real Xf is conjugate symmetric Thus content at 10 Hz implies equal content at 10 Hz The content visible at 40 Hz is an alias of the 10 Hz content Often it is preferred to plot a DFT over the principal frequency range fs2 f fs2 The MATLAB function fftshift properly rearranges the output of fft to accomplish this task stemf1T2fftshiftabsXk axis25 25 005 055 xlabelf Hz ylabelXf When we use fftshift the conjugate symmetry that accompanies the DFT of a real signal becomes apparent as shown in Fig 827 Since DFTs are generally complexvalued the magnitude plots of Figs 826 and 827 offer only half the picture the signals phase spectrum shown in Fig 828 completes it stemf1T2fftshiftangleXk axis25 25 11pi 11pi xlabelf Hz ylabelangle Xf 0 5 10 15 20 25 30 35 40 45 50 f Hz 0 02 04 Xf Figure 826 Xf computed over 0 f 50 by using fft 08LathiC08 2017925 1554 page 830 55 830 CHAPTER 8 SAMPLING THE BRIDGE FROM CONTINUOUS TO DISCRETE 25 20 15 10 5 0 5 10 15 20 25 f Hz 0 05 1 Yf Figure 829 Yf using 50 data points 5 6 7 8 9 10 11 12 13 14 15 f Hz 0 05 1 Yzpf Figure 830 Yzpf over 5 f 15 using 50 data points padded with 550 zeros In this case the vector y contains a noninteger number of cycles Figure 829 shows the significant frequency leakage that results Also notice that since yn is not real the DFT is not conjugate symmetric In this example the discrete DFT frequencies do not include the actual 10 1 3 Hz frequency of the signal Thus it is difficult to determine the signals frequency from Fig 829 To improve the picture the signal is zeropadded to 12 times its original length yzp yzeros111lengthy Yzp fftyzp fzp 012N01T12N0 stemfzp25fftshiftabsYzpk axis25 25 005 105 xlabelf Hz ylabelYzpf Figure 830 zoomed in to 5 f 15 correctly shows the peak frequency at 10 1 3 Hz and better represents the signals spectrum It is important to keep in mind that zero padding does not increase the resolution or accuracy of the DFT To return to the picket fence analogy zero padding increases the number of pickets in our fence but cannot change what is behind the fence More formally the characteristics of the sinc function such as main beam width and sidelobe levels depend on the fixed width of the pulse not on the number of zeros that follow Adding zeros cannot change the characteristics of the sinc function and thus cannot change the resolution or accuracy of the DFT Adding zeros simply allows the sinc function to be sampled more finely 08LathiC08 2017925 1554 page 832 57 832 CHAPTER 8 SAMPLING THE BRIDGE FROM CONTINUOUS TO DISCRETE otherwise dispUnrecognized quantization method return end Several MATLAB commands require discussion First the nargin function returns the number of input arguments In this program nargin is used to ensure that a correct number of inputs is supplied If the number of inputs supplied is incorrect an error message is displayed and the function terminates If only three input arguments are detected the quantization type is not explicitly specified and the program assigns the default symmetric method As with many highlevel languages such as C MATLAB supports general switchcase structures switch switchexpr case caseexpr statements otherwise statements end CH8MP1 switches among cases of the string method In this way methodspecific parameters are easily set The command lower is used to convert a string to all lowercase characters In this way strings such as SYM Sym and sym are all indistinguishable Similar to lower the MATLAB command upper converts a string to all uppercase The floor command rounds input values to the nearest integer toward minus infinity Mathematically it computes To accommodate different types of rounding MATLAB supplies three other rounding commands ceil round and fix The ceil command rounds input values to the nearest integers toward infinity the round command rounds input values toward the nearest integer the fix command rounds input values to the nearest integer toward zero For example if x 05 05 floorx yields 1 0 ceilx yields 0 1 roundx yields 1 1 and fixx yields 0 0 Finally CH8MP1 checks and if necessary corrects large values of xq that may be outside the allowable 2B levels To verify operation CH8MP1 is used to determine the transfer characteristics of a symmetric 3bit quantizer operating over 1010 x 10000110 xsq CH8MP1x103sym plotxxsqk axis10 10 105 105 grid on xlabelQuantizer input ylabelQuantizer output Figure 831 shows the results Clearly the quantized output is limited to 2B 8 levels Zero is not a quantization level for symmetric quantizers so half of the levels occur above zero and half of the levels occur below zero In fact symmetric quantizers get their name from the symmetry in quantization levels above and below zero By changing the method in CH8MP1 from sym to asym we obtain the transfer characteristics of an asymmetric 3bit quantizer as shown in Fig 832 Again the quantized output is limited to 2B 8 levels and zero is now one of the included levels With zero as a quantization A functionally equivalent structure can be written by using if elseif and else statements 08LathiC08 2017925 1554 page 833 58 87 MATLAB The Discrete Fourier Transform 833 10 5 0 5 10 Quantizer input 10 5 0 5 10 Quantizer output Figure 831 Transfer characteristics of a symmetric 3bit quantizer 10 5 0 5 10 Quantizer input 10 5 0 5 10 Quantizer output Figure 832 Transfer characteristics of an asymmetric 3bit quantizer level we need one fewer quantization level above zero than there are levels below Not surprisingly asymmetric quantizers get their name from the asymmetry in quantization levels above and below zero There is no doubt that quantization can change a signal It follows that the spectrum of a quantized signal can also change While these changes are difficult to characterize mathematically they are easy to investigate by using MATLAB Consider a 1 Hz cosine sampled at fs 50 Hz over 1 second x cos2pinT X fftx T 150 N0 50 n 0N01 Upon quantizing by means of a 2bit asymmetric rounding quantizer both the signal and spectrum are substantially changed xaq CH8MP1x12asym Xaq fftxaq subplot221 stemnxk axis0 49 11 11 xlabelnylabelxn subplot222 stemf25fftshiftabsXk axis2525 1 26 xlabelfylabelXf subplot223 stemnxaqkaxis0 49 11 11 08LathiC08 2017925 1554 page 834 59 834 CHAPTER 8 SAMPLING THE BRIDGE FROM CONTINUOUS TO DISCRETE 0 10 20 30 40 n 1 0 1 xn 20 10 0 10 20 f 0 10 20 Xf 0 10 20 30 40 n 1 0 1 xaqn 20 10 0 10 20 f 0 10 20 Xaqf Figure 833 Signal and spectrum effects of quantization xlabelnylabelxaqn subplot224 stemf25fftshiftabsfftxaqk axis2525 1 26 xlabelfylabelXaqf The results are shown in Fig 833 The original signal xn appears sinusoidal and has pure spectral content at 1 Hz The asymmetrically quantized signal xaqn is significantly distorted The corresponding magnitude spectrum Xaqf is spread over a broad range of frequencies 88 SUMMARY A signal bandlimited to B Hz can be reconstructed exactly from its samples if the sampling rate fs 2B Hz the sampling theorem Such a reconstruction although possible theoretically poses practical problems such as the need for ideal filters which are unrealizable or are realizable only with infinite delay Therefore in practice there is always an error in reconstructing a signal from its samples Moreover practical signals are not bandlimited which causes an additional error aliasing error in signal reconstruction from its samples When a signal is sampled at a frequency fs Hz samples of a sinusoid of frequency fs2 x Hz appear as samples of a lower frequency fs2 x Hz This phenomenon in which higher frequencies appear as lower frequencies is known as aliasing Aliasing error can be reduced by bandlimiting a signal to fs2 Hz half the sampling frequency Such bandlimiting done prior to sampling is accomplished by an antialiasing filter that is an ideal lowpass filter of cutoff frequency fs2 Hz The sampling theorem is very important in signal analysis processing and transmission because it allows us to replace a continuoustime signal with a discrete sequence of numbers Processing a continuoustime signal is therefore equivalent to processing a discrete sequence of numbers This leads us directly into the area of digital filtering discretetime systems In the field 08LathiC08 2017925 1554 page 843 68 Problems 843 1 1 t t xt gt 1 1 2 a b 0 Figure P856 been computed derive a method to correct X to reflect an arbitrary starting time n n0 872 Consider a complex signal composed of two closely spaced complex exponentials x1n ej2πn30100 ej2πn33100 For each of the follow ing cases plot the lengthN DFT magnitude as a function of frequency fr where fr rN a Compute and plot the DFT of x1n using 10 samples 0 n 9 From the plot can both exponentials be identified Explain b Zeropad the signal from part a with 490 zeros and then compute and plot the 500point DFT Does this improve the pic ture of the DFT Explain c Compute and plot the DFT of x1n using 100 samples 0 n 99 From the plot can both exponentials be identified Explain d Zeropad the signal from part c with 400 zeros and then compute and plot the 500point DFT Does this improve the pic ture of the DFT Explain 873 Repeat Prob 872 using the complex signal x2n ej2πn30100 ej2πn315100 874 Consider a complex signal composed of a dc term and two complex exponentials y1n 1 ej2πn30100 05 ej2πn43100 For each of the following cases plot the lengthN DFT magnitude as a function of frequency fr where fr rN a Use MATLAB to compute and plot the DFT of y1n with 20 samples 0 n19 From the plot can the two nondc exponentials be identified Given the amplitude rela tion between the two the lowerfrequency peak should be twice as large as the higherfrequency peak Is this the case Explain b Zeropad the signal from part a to a total length of 500 Does this improve locating the two nondc exponential components Is the lowerfrequency peak twice as large as the higherfrequency peak Explain c MATLABs signalprocessing toolbox func tion window allows window functions to be easily generated Generate a length20 Han ning window and apply it to y1n Using this windowed function repeat parts a and b Comment on whether the window function helps or hinders the analysis 875 Repeat Prob 874 using the complex signal y2n 1 ej2πn30100 05ej2πn38100 876 This problem investigates the idea of zero padding applied in the frequency domain When asked plot the lengthN DFT magnitude as a function of frequency fr where fr rN a In MATLAB create a vector x that con tains one period of the sinusoid xn cosπ2n Plot the result How sinu soidal does the signal appear to be b Use the fft command to compute the DFT X of vector x Plot the magnitude of the DFT coefficients Do they make sense c Zeropad the DFT vector to a total length of 100 by inserting the appropriate number of zeros in the middle of the vector X Call this zeropadded DFT sequence Y Why are zeros inserted in the middle rather than the end Take the inverse DFT of Y and plot the result What similarities exist between the new signal y and the original signal x What are the differences between x and y What is the effect of zero padding in the frequency domain How is this type of zero padding similar to zero padding in the time domain d Derive a general modification to the pro cedure of zero padding in the frequency domain to ensure that the amplitude of the resulting timedomain signal is left unchanged 09LathiC09 2017925 1555 page 855 11 92 Aperiodic Signal Representation by Fourier Integral 855 0 5 10 15 20 25 30 r 01 0 01 02 Xr Figure 93 MATLABcomputed DTFS spectra for periodic sampled gate pulse of Ex 92 92 APERIODIC SIGNAL REPRESENTATION BY FOURIER INTEGRAL In Sec 91 we succeeded in representing periodic signals as a sum of everlasting exponentials In this section we extend this representation to aperiodic signals The procedure is identical conceptually to that used in Ch 7 for continuoustime signals Applying a limiting process we now show that an aperiodic signal xn can be expressed as a continuous sum integral of everlasting exponentials To represent an aperiodic signal xn such as the one illustrated in Fig 94a by everlasting exponential signals let us construct a new periodic signal xN0n formed by repeating the signal xn every N0 units as shown in Fig 94b The period N0 is made large enough to avoid overlap between the repeating cycles N0 2N 1 The periodic signal xN0n can be represented by an exponential Fourier series If we let N0 the signal Figure 94 Generation of a periodic signal by periodic extension of a signal xn 09LathiC09 2017925 1555 page 889 45 97 MATLAB Working with the DTFS and the DTFT 889 97 MATLAB WORKING WITH THE DTFS AND THE DTFT This section investigates various methods to compute the discretetime Fourier series DTFS Performance of these methods is assessed by using MATLABs stopwatch and profiling functions Additionally the discretetime Fourier transform DTFT is applied to the important topic of finite impulse response FIR filter design 971 Computing the DiscreteTime Fourier Series Within a scale factor the DTFS is identical to the DFT Thus methods to compute the DFT can be readily used to compute the DTFS Specifically the DTFS is the DFT scaled by 1N0 As an example consider a 50 Hz sinusoid sampled at 1000 Hz over onetenth of a second T 11000 N0 100 n 0N01 x cos2pi50nT The DTFS is obtained by scaling the DFT X fftxN0 f 0N01TN0 stemf12TfftshiftabsXk axis500 500 005 055 xlabelf Hz ylabelXf Figure 919 shows a peak magnitude of 05 at 50 Hz This result is consistent with Eulers representation cos2π50nT 1 2ej2π50nT 1 2ej2π50nT Lacking the 1N0 scale factor the DFT would have a peak amplitude 100 times larger The inverse DTFS is obtained by scaling the inverse DFT by N0 x realifftXN0 stemnxk axis0 99 11 11 xlabeln ylabelxn Figure 920 confirms that the sinusoid xn is properly recovered Although the result is theoretically real computer roundoff errors produce a small imaginary component which the real command removes 500 400 300 200 100 0 100 200 300 400 500 f Hz 0 02 04 Xf Figure 919 DTFS computed by scaling the DFT 09LathiC09 2017925 1555 page 891 47 97 MATLAB Working with the DTFS and the DTFT 891 Let us create an anonymous function to compute the N0byN0 DFT matrix WN0 Although not used here the signalprocessing toolbox function dftmtx computes the same DFT matrix although in a less obvious but more efficient fashion W N0 expj2piN00N010N01 While less efficient than FFTbased methods the matrix approach correctly computes the DTFS X WN0xN0 stemf12TfftshiftabsXk axis500 500 005 055 xlabelf Hz ylabelXf The resulting plot is indistinguishable from Fig 919 Problem 971 investigates a matrixbased approach to compute Eq 93 the inverse DTFS 972 Measuring Code Performance Writing efficient code is important particularly if the code is frequently used requires complicated operations involves large data sets or operates in real time MATLAB provides several tools for assessing code performance When properly used the profile function provides detailed statistics that help assess code performance MATLAB help thoroughly describes the use of the sophisticated profile command A simpler method of assessing code efficiency is to measure execution time and compare it with a reference The MATLAB command tic starts a stopwatch timer The toc command reads the timer Sandwiching instructions between tic and toc returns the elapsed time For example the execution time of the 100point matrixbased DTFS computation is tic WN0xN0 toc Elapsed time is 0004417 seconds Different machines operate at different speeds with different operating systems and with different background tasks Therefore elapsedtime measurements can vary considerably from machine to machine and from execution to execution For relatively simple and short events like the present case execution times can be so brief that MATLAB may report unreliable times or fail to register an elapsed time at all To increase the elapsed time and therefore the accuracy of the time measurement a loop is used to repeat the calculation tic for i1100 WN0xN0 end toc Elapsed time is 0173388 seconds This elapsed time suggests that each 100point DTFS calculation takes a little under 2 milliseconds What exactly does this mean however Elapsed time is only meaningful relative to some reference Let us see what difference occurs by precomputing the DFT matrix rather than repeatedly using our anonymous function W100 W100 tic for i1100 W100xN0 end toc Elapsed time is 0001199 seconds 09LathiC09 2017925 1555 page 897 53 97 MATLAB Working with the DTFS and the DTFT 897 0 5 10 15 20 Ω 25 30 35 40 n 02 0 02 04 hn 0 1 2 3 4 5 6 0 05 1 15 HΩ Samples Desired Actual Figure 924 Length41 FIR lowpass filter using linear phase 0 5 10 15 20 25 30 35 40 45 50 n Ω 02 0 02 hn 0 1 2 3 4 5 6 0 05 1 15 HΩ Samples Desired Actual Figure 925 Length50 FIR bandpass filter using linear phase 10LathiC10 2017925 1555 page 908 1 C H A P T E R STATESPACE ANALYSIS 10 In Sec 110 basic notions of state variables were introduced In this chapter we shall discuss state variables in more depth Most of this book deals with an external inputoutput description of systems As noted in Ch 1 such a description may be inadequate in some cases and we need a systematic way of finding a systems internal description Statespace analysis of systems meets this need In this method we first select a set of key variables called the state variables in the system Every possible signal or variable in the system at any instant t can be expressed in terms of the state variables and the inputs at that instant t If we know all the state variables as a function of t we can determine every possible signal or variable in the system at any instant with a relatively simple relationship The system description in this method consists of two parts 1 A set of equations relating the state variables to the inputs the state equation 2 A set of equations relating outputs to the state variables and the inputs the output equation The analysis procedure therefore consists of solving the state equation first and then solving the output equation The statespace description is capable of determining every possible system variable or output from knowledge of the input and the initial state conditions of the system For this reason it is an internal description of the system By its nature state variable analysis is eminently suited for multipleinput multipleoutput MIMO systems A singleinput single output SISO system is a special case of MIMO systems In addition the statespace techniques are useful for several other reasons mentioned in Sec 110 and repeated here 1 The state equations of a system provide a mathematical model of great generality that can describe not just linear systems but also nonlinear systems not just timeinvariant systems but also timevarying parameter systems not just SISO systems but also MIMO systems Indeed state equations are ideally suited for analysis synthesis and optimization of MIMO systems 2 Compact matrix notation along with powerful techniques of linear algebra greatly facilitates complex manipulations Without such features many important results of 908 10LathiC10 2017925 1555 page 915 8 102 Introduction to State Space 915 or q2 q1 2q2 Thus the two state equations are q1 25q1 5q2 10x q2 q1 2q2 Every possible output can now be expressed as a linear combination of q1 q2 and x From Fig 101 we have v1 x q1 i1 2x q1 v2 q1 i2 3q1 i3 i1 i2 q2 2x q1 3q1 q2 5q1 q2 2x i4 q2 v4 2i4 2q2 v3 q1 v4 q1 2q2 This set of equations is known as the output equation of the system It is clear from this set that every possible output at some instant t can be determined from knowledge of q1t q2t and xt the system state and the input at the instant t Once we have solved the state equations to obtain q1t and q2t we can determine every possible output for any given input xt For continuoustime systems the state equations are N simultaneous firstorder differential equations in N state variables q1 q2 qN of the form qi giq1q2 qNx1x2 xj i 12 N where x1 x2 xj are the j system inputs For a linear system these equations reduce to a simpler linear form qi ai1q1 ai2q2 aiNqN bi1x1 bi2x2 bijxj i 12 N 1014 If there are k outputs y1y2 yk the k output equations are of the form ym cm1q1 cm2q2 cmNqN dm1x1 dm2x2 dmjxj m 12 k 1015 The N simultaneous firstorder state equations are also known as the normalform equations 10LathiC10 2017925 1555 page 952 45 952 CHAPTER 10 STATESPACE ANALYSIS Using our previous calculations we have z1 z1 z2 z2 x and thus y z1 z2 Figure 1010b shows a realization of these equations Clearly each of the two modes is observable at the output but the mode corresponding to λ1 1 is not controllable USING MATLAB TO DETERMINE CONTROLLABILITY AND OBSERVABILITY As demonstrated in Ex 1011 we can use MATLABs eig function to determine the matrix P that will diagonalize A We can then use P to determine ˆB and ˆC from which we can determine the controllability and observability of a system Let us demonstrate the process for the two present systems First let us use MATLAB to compute ˆB and ˆC for the system in Fig 109a A 1 01 1 B 1 0 C 1 2 V Lambda eigA PinvV Bhat PB Chat CinvP Bhat 05000 11180 Chat 2 0 Since all the rows of ˆB are nonzero the system is controllable However one column of ˆC is zero so one mode is unobservable Next let us use MATLAB to compute ˆB and ˆC for the system in Fig 109b A 1 02 1 B 1 1 C 0 1 V Lambda eigA PinvV Bhat PB Chat CinvP Bhat 0 14142 Chat 10000 07071 One of the rows of ˆB is zero so one mode is uncontrollable Since all of the columns of ˆC are nonzero the system is observable As expected the MATLAB results confirm our earlier conclusions regarding the controllability and observability of the systems of Fig 109 10LathiC10 2017925 1555 page 953 46 107 StateSpace Analysis of DiscreteTime Systems 953 1061 Inadequacy of the Transfer Function Description of a System Example 1012 demonstrates the inadequacy of the transfer function to describe an LTI system in general The systems in Figs 109a and 109b both have the same transfer function Hs 1 s 1 Yet the two systems are very different Their true nature is revealed in Figs 1010a and 1010b respectively Both the systems are unstable but their transfer function Hs 1s 1 does not give any hint of it Moreover the systems are very different from the viewpoint of controllability and observability The system in Fig 109a is controllable but not observable whereas the system in Fig 109b is observable but not controllable The transfer function description of a system looks at a system only from the input and output terminals Consequently the transfer function description can specify only the part of the system that is coupled to the input and the output terminals From Figs 1010a and 1010b we see that in both cases only a part of the system that has a transfer function Hs 1s 1 is coupled to the input and the output terminals This is why both systems have the same transfer function Hs 1s 1 The state variable description Eqs 1058 and 1059 on the other hand contains all the information about these systems to describe them completely The reason is that the state variable description is an internal description not the external description obtained from the system behavior at external terminals Apparently the transfer function fails to describe these systems completely because the transfer functions of these systems have a common factor s1 in the numerator and denominator this common factor is canceled out in the systems in Fig 109 with a consequent loss of the information Such a situation occurs when a system is uncontrollable andor unobservable If a system is both controllable and observable which is the case with most of the practical systems the transfer function describes the system completely In such a case the internal and external descriptions are equivalent 107 STATESPACE ANALYSIS OF DISCRETETIME SYSTEMS We have shown that an Nthorder differential equation can be expressed in terms of N firstorder differential equations In the following analogous procedure we show that a general Nthorder difference equation can be expressed in terms of N firstorder difference equations Consider the ztransfer function Hz b0zN b1zN1 bN1z bN zN a1zN1 aN1z aN The input xn and the output yn of this system are related by the difference equation EN a1EN1 aN1E aNyn b0EN b1EN1 bN1E bNxn The DFII realization of this equation is illustrated in Fig 1011 10LathiC10 2017925 1555 page 963 56 108 MATLAB Toolboxes and StateSpace Analysis 963 Q simplifyQ Q 2z 6z2 2z 16z3 11z2 6z 1 2z9z2 7z 16z3 11z2 6z 1 The resulting expression is mathematically equivalent to the original but notationally more compact Since D 0 the output Yz is given by Yz CQz Y simplifyCQ Y 6z13z2 11z 26z3 11z2 6z 1 The corresponding timedomain expression is obtained by using the inverse ztransform command iztrans y iztransY y 312n 213n 12 Like ztrans the iztrans command assumes a causal signal so the result implies multiplication by a unit step That is the system output is yn 312n213n12un which is equivalent to Eq 1069 derived in Ex 1013 Continuoustime systems use inverse Laplace transforms rather than inverse ztransforms In such cases the ilaplace command therefore replaces the iztrans command Following a similar procedure it is a simple matter to compute the zeroinput response yzirn yzir iztranssimplifyCinveye2z1Aq0 yzir 2112n 813n The zerostate response is given by yzsr y yzir yzsr 613n 1812n 12 Typing iztranssimplifyCinvzeye2ABX produces the same result MATLAB plotting functions such as plot and stem do not directly support symbolic expressions By using the subs command however it is easy to replace a symbolic variable with a vector of desired values 0 5 10 15 20 25 n 115 12 125 13 135 yn Figure 1014 Output yn computed by using the symbolic math toolbox 10LathiC10 2017925 1555 page 964 57 964 CHAPTER 10 STATESPACE ANALYSIS n 025 stemnsubsynk xlabeln ylabelyn axis5 255 115 135 Figure 1014 shows the results which are equivalent to the results obtained in Ex 1013 Although there are plotting commands in the symbolic math toolbox such as ezplot that plot symbolic expression these plotting routines lack the flexibility needed to satisfactorily plot discretetime functions 1082 Transfer Functions from StateSpace Representations A systems transfer function provides a wealth of useful information From Eq 1073 the transfer function for the system described in Ex 1013 is H collectsimplifyCinvzeye2ABD H 30z 66z2 5z 1 It is also possible to determine the numerator and denominator transfer function coefficients from a statespace model by using the signalprocessing toolbox function ss2tf numden ss2tfABCD num 0 50000 10000 den 10000 08333 01667 The denominator of Hz provides the characteristic polynomial γ 2 5 6γ 1 6 Equivalently the characteristic polynomial is the determinant of zI A syms gamma charpoly subsdetzeye2Azgamma charpoly gamma2 5gamma6 16 Here the subs command replaces the symbolic variable z with the desired symbolic variable gamma The roots command does not accommodate symbolic expressions Thus the sym2poly command converts the symbolic expression into a polynomial coefficient vector suitable for the roots command rootssym2polycharpoly ans 05000 03333 Taking the inverse ztransform of Hz yields the impulse response hn h iztransH h 1812n 1213n 6kroneckerDeltan 0 As suggested by the characteristic roots the characteristic modes of the system are 12n and 13n Notice that the symbolic math toolbox represents δn as kroneckerDeltan 0 In general δn a is represented as kroneckerDeltana 0 This notation is frequently 10LathiC10 2017925 1555 page 969 62 109 Summary 969 doublesubsAnn3 ans 01389 05278 00880 03009 For continuoustime systems the matrix exponential eAt is commonly encountered The expm command can compute the matrix exponential symbolically Using the system from Ex 108 yields syms t A 12 2336 1 eAt simplifyexpmAt eAt exp9t3exp5t 85 2exp9texp5t 115 36exp9texp5t 15 exp9t8exp5t 35 This result is identical to the result computed in Ex 108 Similar to the discretetime case an identical result is obtained by typing syms s simplifyilaplaceinvseye2A For a specific t the matrix exponential is also easy to compute either through substitution or direct computation Consider the case t 3 doublesubseAtt3 ans 10e004 00369 00082 04424 00983 The command expmA3 produces the same result 109 SUMMARY An Nthorder system can be described in terms of N key variablesthe state variables of the system The state variables are not unique rather they can be selected in a variety of ways Every possible system output can be expressed as a linear combination of the state variables and the inputs Therefore the state variables describe the entire system not merely the relationship between certain inputs and outputs For this reason the state variable description is an internal description of the system Such a description is therefore the most general system description and it contains the information of the external descriptions such as the impulse response and the transfer function The state variable description can also be extended to timevarying parameter systems and nonlinear systems An external description of a system may not characterize the system completely The state equations of a system can be written directly from knowledge of the system structure from the system equations or from the block diagram representation of the system State equations consist of a set of N firstorder differential equations and can be solved by timedomain or frequencydomain transform methods Suitable procedures exist to transform one given set of state variables into another Because a set of state variables is not unique we can have an infinite variety of statespace descriptions of the same system The use of an appropriate transformation allows us to see clearly which of the system states are controllable and which are observable 10LathiC10 2017925 1555 page 974 67 11LathiIndex 2017925 1929 page 975 1 INDEX Abscissa of convergence 336 Accumulator systems 259 295 519 discretetime Fourier transform of 87576 Active circuits 38285 Adders 388 396 399 403 Addition of complex numbers 1112 of matrices 38 of sinusoids 1820 Additivity 9798 Algebra of complex numbers 515 matrix 3842 Aliasing 53638 78895 805 81112 817 834 defined 536 general condition for in sinusoids 79396 treachery of 78891 verification of in sinusoids 79293 Aliasing error 659 811 Amplitude 16 Amplitude modulation 71113 73649 762 Amplitude response 413 41617 421 424 435 43738 44042 Amplitude spectrum 598 607 61525 667 668 707 848 870 878 Analog filters 261 Analog signals 133 defined 78 digital processing of 54754 properties of 78 Analog systems 109 135 261 Analogtodigital AD conversion 799802 831 Analogtodigital converters ADC bit number 8012 bit rate 8012 Angle modulation 736 763 Angles electronic calculators in computing 811 principal value of 9 Angular acceleration 116 Angular position 116 Angular velocity 116 Antialiasing filters 537 791 834 Anticausal exponentials 86263 Anticausal signals 81 Aperiodic signals 133 discretetime Fourier integral and 85567 Fourier integral and 68089 762 properties of 7882 Apparent frequency 53436 79296 Ars Magna Cardano 25 Associative property 171 283 Asymptotic stability See Internal stability Audio signals 71314 725 746 Automatic position control system 4068 Auxiliary conditions 153 differential equation solution and 161 Backward difference system 258 295 519 56869 Bandlimited signals 533 788 792 802 Bandpass filters 44143 54244 749 88283 896 group delay and 72627 ideal 73031 88283 poles and zeros of Hs and 443 Bandstop filters 44142 445 54546 Bandwidth 628 continuoustime systems and 20810 data truncation and 75153 essential 736 75859 Fourier transform and 692 706 76263 Bartlett window 75355 Baseband signals 73740 74647 749 Basis signals 651 655 668 Basis vectors 648 Beat effect 741 Bhaskar 2 Bilateral Laplace transform 330 33537 44555 467 properties of 45155 Bilateral ztransform 431 490 55463 in discretetime system analysis 563 properties of 55960 Bilinear transformation 56970 Binary digital signals 799 Black box 95 119 120 975 11LathiIndex 2017925 1929 page 976 2 976 Index Blackman window 755 761 Block diagrams 38688 405 407 408 519 Bôcher M 62021 Bode plots 41935 constant of 421 firstorder pole and 42427 pole at the origin and 42223 secondorder pole and 42635 Bombelli Raphael 3 Bonaparte Napoleon 347 61011 Boundedinputboundedoutput BIBO stability 110 135 263 of continuoustime systems 19697 199203 22223 of discretetime systems 29899 3014 314 526 527 frequency response and 41213 internal stability relationship to 199203 3014 of the Laplace transform 37173 signal transmission and 721 steadystate response and 418 of the ztransform 518 Butterfly signal flow graph 825 Butterworth filters 440 55154 cascaded secondorder sections for Butterworth filter realization 46163 MATLAB on 45963 transformation of 57172 Canonic direct realization See Direct form II realization Cardano Gerolamo 25 Cartesian form 815 Cascade realization 394 526 920 923 Cascade systems 190 192 37273 Cascaded RC filters 46162 Causal exponentials 86162 Causal signals 81 83 134 Causal sinusoidal input in continuoustime systems 41819 in discretetime systems 527 Causal systems 1046 135 263 properties of 1046 zerostate response and 172 283 CayleyHamilton theorem 91012 Characteristic equations of continuoustime systems 15355 of discretetime systems 271 273 309 of a matrix 91012 933 Characteristic functions 192 Characteristic modes of continuoustime systems 15355 16265 167 170 196 19899 2036 of discretetime systems 27174 27879 297301 305 313 Characteristic polynomials of continuoustime systems 15356 164 166 2023 220 of discretetime systems 271 27475 279 3034 of the Laplace transform 37172 of the ztransform 518 Characteristic roots of continuoustime systems 15356 162 166 198203 206 209 21112 21417 22224 of discretetime systems 271 27375 297 299301 3035 309 314 invariance of 94243 of a matrix 911 93233 Characteristic values See Characteristic roots Characteristic vectors 910 Chebyshev filters 440 46366 Circular convolution 81920 821 Clearing fractions 2627 3233 34243 Closed Loop systems See Feedback systems Coherent demodulation See Synchronous demodulation Coefficients of Fourier series computation 59598 Column vectors 36 Commutative property of the convolution integral 170 173 181 19192 of the convolution sum 283 Compact disc CD 801 Compact form of Fourier series 59798 599 600 6047 Complex factors of Qx 29 Complex frequency 8991 Complex inputs 177 297 Complex numbers 115 54 algebra of 515 arithmetical operations for 1215 conjugates of 67 historical note 15 logarithms of 15 origins of 25 standard forms of 1415 useful identities 78 working with 1314 Complex poles 395 432 497 542 Complex roots 15456 27476 Complex signals 9495 Conjugate symmetry of the discrete Fourier transform 81819 of the discretetime Fourier transform 85859 86768 of the Fourier transform 684 703 Conjugation 684 703 Constants 54 98 100 103 130 422 Constantparameter systems See Timeinvariant systems Continuous functions 858 Continuoustime filters 45563 Continuoustime Fourier transform CTFT 867 88485 Continuoustime signals 1078 135 defined 78 discretetime systems and 238 Fourier series and 593679 Fourier transform and 678769 680775 Continuoustime systems 135 150236 analog systems compared with 261 differential equations of 161 196 213 11LathiIndex 2017925 1929 page 977 3 Index 977 discretetime systems compared with 261 external input response to 16896 frequency response of 41218 73233 internal conditions response to 15163 intuitive insights into 18990 2035 Laplace transform 330487 Periodic inputs and 637641 properties of 1078 signal transmission through 72129 stability of 196203 22223 state equations for 91516 Control systems 40412 analysis of 40612 design specifications 411 step input and 4079 Controllabilityobservability 12324 of continuoustime systems 197 2002 223 of discretetime systems 303 96568 in statespace analysis 94753 961 Convergence abscissa of 336 of Fourier series 61314 to the mean 613 614 region of See region of convergence Convolution 5079 with an impulse 283 of the bilateral ztransform 560 circular 81920 821 discretetime 31112 fast 821 886 frequency See Frequency convolution of the Fourier transform 71416 linear 821 periodic 886 time See Time convolution Convolution integral 17093 222 282 288 313 722 explanation for use 18990 graphical understanding of 17890 21720 properties of 17072 Convolution sum 28286 313 graphical procedure for 28893 properties of 28283 from a table 28586 Convolution table 17576 Cooley J W 824 Corner frequency 424 Cramers rule 2325 40 51 379 385 Critically damped systems 409 410 Cubic equations 23 58 Custom filter function 31011 Cutoff frequency 208 209 Damping coefficient 11518 Dashpots linear 115 torsional 116 Data truncations 74955 763 Decades 422 Decibels 421 Decimationinfrequency algorithm 824 827 Decimationintime algorithm 82527 Decomposition 99100 151 Delayed impulse 168 Demodulation 714 of amplitude modulation 74446 of DSBSC signals 73941 synchronous 74344 Depressed cubic equation 58 Derivative formulas 56 Descartes René 2 Detection See Demodulation Deterministic signals 82 134 Diagonal matrices 37 Difference equations 25960 26570 causality condition in 26566 classical solution of 298 differential equation kinship with 260 frequency response 532 order of 260 recursive and nonrecursive forms of 259 recursive solution of 26670 sinusoidal response of difference equation systems 528 ztransform solution of 488 51019 574 Differential equations 161 classical solution of 196 difference equation kinship with 260 Laplace transform solution of 34648 36073 Differentiators digital 25658 ideal 36971 373 41617 Digital differentiator example 25859 Digital filters 108 238 26162 Digital integrators 25859 Digital processing of analog signals 54753 Digital signals 135 79799 advantages of 26162 binary 799801 defined 78 Lary 799 properties of 78 See also Analogtodigital conversion Digital systems 109 135 261 Dirac definition of an impulse 88 134 Dirac delta train 69697 Dirac PAM 86 Direct discrete Fourier transform DFT 808 857 Direct form I DFI realization Laplace transform and 39091 394 ztransform and 521 See also Transposed direct form II realization 11LathiIndex 2017925 1929 page 978 4 978 Index Direct form II DFII realization 92025 954 965 967 Laplace transform and 391 398 ztransform and 52022 525 Direct Fourier transform 683 7023 762 Direct ztransform 488592 Dirichlet conditions 612 614 686 Discrete Fourier transform DFT 659 80523 82734 835 aliasing and leakage and 8056 applications of 82023 computing Fourier transform 81218 derivation of 80710 determining filter output 82223 direct 808 857 discretetime Fourier transform and 88586 898 inverse 808 835 857 MATLAB on 82734 picket fence effect and 807 points of discontinuity 807 properties of 81820 zero padding and 81011 82930 Discretetime complex exponentials 252 Discretetime convolution 31112 Discretetime exponentials 24749 Discretetime Fourier integral 85567 Discretetime Fourier series DTFS 84555 computation of 88586 MATLAB on 88997 of periodic gate function 85355 periodic signals and 84647 898 of sinusoids 84952 Discretetime Fourier transform DTFT 85788 of accumulator systems 87576 of anticausal exponentials 86263 of causal exponentials 86162 continuoustime Fourier transform and 88386 existence of 859 886 inverse 886 linear timeinvariant discretetime system analysis by 87980 MATLAB on 88997 physical appreciation of 859 properties of 86778 of rectangular pulses 86365 table of 860 ztransform connection with 86667 88688 898 Discretetime signals 78 79 1078 133 23753 defined 78 Fourier analysis of 845907 inherently bandlimited 533 size of 23840 useful models 24553 useful operations 24045 Discretetime systems 135 237329 classification of 26264 controllabilityobservability of 303 96568 difference equations of 25960 26570 298 discretetime Fourier transform analysis of 87883 examples of 25365 external input response to 28098 frequency response of 52638 internal conditions response to 27076 intuitive insights into 3056 properties of 1078 26465 stability of 263 298305 314 statespace analysis of 95364 ztransform analysis of 488592 Distinct factors of Qx 27 Distortionless transmission 72428 730 763 88082 bandpass systems and 72627 88182 measure of delay variation 881 Distributive property 171 283 Division of complex numbers 1214 Doublesideband suppressedcarrier DSBSC modulation 73741 742 74649 Downsampling 24344 Duality 7034 Dynamic systems 1034 13435 263 Eigenfunctions 193 Eigenvalues See Characteristic roots Eigenvectors 910 Einstein Albert 348 Electrical systems 9596 11114 Laplace transform analysis of 37385 467 state equations for 91619 Electromechanical systems 11819 Electronic calculators 811 Energy signals 67 82 134 23940 Energy spectral density 734 763 Envelope delay See Group delay Envelope detector 74345 Equilibrium states 196 198 Error signals 65051 Error vectors 642 Essential bandwidth 736 75859 Euler Leonhard 2 3 Eulers formula 56 45 252 Even component of a signal 9395 Even functions 9293 134 Everlasting exponentials continuoustime systems and 189 19395 222 discretetime systems and 29697 313 Fourier series and 637 638 641 Fourier transform and 687 Laplace transform and 36768 412 419 Everlasting signals 81 134 Exponential Fourier series 62137 661 803 periodic inputs and 63741 reasons for using 640 symmetry effect on 63032 11LathiIndex 2017925 1929 page 979 5 Index 979 Exponential Fourier spectra 62432 664 667 668 Exponential functions 8991 134 Exponential input 193 296 Exponentials computation of matrix 922913 discretetime 24749 discretetime complex 252 everlasting See Everlasting exponentials matrix 96869 monotonic 2022 90 91 134 sinusoid varying 2223 90 134 sinusoids expressed in 20 Exposition du système du monde Laplace 346 External description of a system 11920 135 External input continuoustime system response to 16896 discretetime system response to 28098 External stability See Boundedinputboundedoutput stability Fast convolution 821 886 Fast Fourier transform FFT 659 811 821 82427 835 computations reduced by 824 discretetime Fourier series and 847 discretetime Fourier transform and 88586 898 Feedback systems Laplace transform and 38688 39295 399 40412 ztransform and 521 Feedforward connections 39294 403 Filtering discrete Fourier transform and 82123 MATLAB on 30810 selective 74849 time constant and 2078 Filters analog 261 antialiasing 537 791 834 bandpass 44143 bandstop 44142 445 54546 Butterworth See Butterworth Filters cascaded RC 46162 Chebyshev 440 46366 continuoustime 45563 custom function 31011 digital 108 238 26162 finite impulse response 524 89297 firstorder hold 785 frequency response of 41218 highpass 443 445 542 73031 88283 Ideal See Ideal filters impulse invariance criterion of 548 infinite impulse response 524 56574 lowpass 43941 lowpass See Lowpass filters notch 44143 540 54546 poles and zeros of Hs and 43645 practical 44445 88283 sharp cutoff 748 windows in design of 755 zeroorder hold 785 Final value theorem 35961 508 Finite impulse response FIR filters 524 89297 Finiteduration signals 333 Finitememory systems 104 Firstorder factors method of 497 Firstorder hold filters 785 Folding frequency 78991 793 795 817 Forloops 21618 Forced response difference equations and 298 differential equations and 198 Forward amplifiers 4056 Fourier integral 722 aperiodic signal and 68089 762 discretetime 85567 Fourier series 593679 compact form of 59798 599 600 6047 computing the coefficients of 59598 discrete time See Discretetime Fourier series existence of 61213 exponential See Exponential Fourier series generalized 64159 668 Legendre 65657 limitations of analysis method 641 trigonometric See Trigonometric Fourier series waveshaping in 61517 Fourier spectrum 598607 777 exponential 62432 664 667 668 nature of 85859 of a periodic signal 84855 Fourier transform 680755 778 8023 continuoustime 867 88386 discrete See Discrete Fourier transform discretetime See Discretetime Fourier transform direct 683 7023 762 existence of 68586 fast See fast Fourier transform interpolation and 785 inverse 683 69395 699 762 78687 physical appreciation of 68789 properties of 70121 useful functions of 689701 Fourier transform pairs 683 700 Fourier Baron JeanBaptisteJoseph 61012 Fractions 12 clearing 2627 3234 34243 partial See Partial fractions Frequency apparent 53436 79394 complex 8991 11LathiIndex 2017925 1929 page 980 6 980 Index Frequency continued corner 424 cutoff 208 209 folding 78991 793 795 817 fundamental 594 60910 846 negative 62628 neper 91 radian 16 91 594 reduction in range 535 of sinusoids 16 time delay variation with 72425 Frequency convolution of the bilateral Laplace transform 452 of the discretetime Fourier transform 87576 of the Fourier transform 71416 of the Laplace transform 357 Frequency differentiation 869 Frequency domain analysis 368 72223 848 of electrical networks 37478 of the Fourier series 598 601 twodimensional view and 73233 See also Laplace transform Frequency inversion 706 Frequency resolution 807 81012 815 817 Frequency response 724 Bode plots and 41922 of continuoustime systems 41218 73233 of discretetime systems 52638 MATLAB on 45657 53132 periodic nature of 53236 from polezero location 53847 polezero plots and 56668 poles and zeros of Hs and 43639 transfer function from 435 Frequency reversal 86869 Frequency shifting of the bilateral Laplace transform 451 of the discrete Fourier transform 819 of the discretetime Fourier transform 87174 of the Fourier transform 71113 of the Laplace transform 35354 Frequency spectra 598 601 Frequencydivision multiplexing FDM 714 74950 Function Mfiles 21415 Functions characteristic 193 continuous 858 even 9293 134 exponential 8991 134 improper 2526 34 interpolation 690 MATLAB on 12633 odd 9295 134 proper 2527 rational 2529 338 singularity 89 Fundamental band 533 534 537 793 Fundamental frequency 594 60910 846 Fundamental period 79 133 23940 593 595 846 Gain enhancement by poles 43738 Gauss Karl Friedrich 34 Generalized Fourier series 64159 668 Generalized linear phase GLP 72627 Gibbs phenomenon 61921 66163 Gibbs Josiah Willard 62021 Graphical interpretation of convolution integral 17890 21720 of convolution sum 28893 Greatest common factor of frequencies 60910 Group delay 72528 881 Hs filter design and 43645 realization of 54849 See also Transfer functions Halfwave symmetry 608 Hamming window 75455 761 Hanning window 75455 761 Hardware realization 64 95 133 Harmonic distortion 634 Harmonically related frequencies 609 Heaviside coverup method 2730 3335 341 34243 497 Heaviside Oliver 34748 612 Highpass filters 443 445 542 745 747 88283 Homogeneity 9798 Ideal delay 369 416 Ideal differentiators 36971 373 41617 Ideal filters 73033 763 785 791 834 88283 Ideal integrators 369 370 373 400 41618 Ideal interpolation 78687 Ideal linear phase ILP 725 727 Ideal masses 114 Identity matrices 37 Identity systems 109 192 263 Imaginary numbers 15 Impedance 37477 379 380 382 384 387 399 Improper functions 2526 34 Impulse invariance criterion of filter design 548 Impulse matching 16466 Impulse response matrix 938 Indefinite integrals 57 Indicator function See Relational operators Inertia moment of 11618 Infinite impulse response IIR filters 524 56574 Information transmission rate 20910 11LathiIndex 2017925 1929 page 981 7 Index 981 Initial conditions 97100 102 122 134 335 at 0 and 0 36364 continuoustime systems and 15861 generators of 37683 Initial value theorem 35961 508 Input 64 complex 177 297 exponential 193 296 external See External input in linear systems 97 multiple 178 28788 ramp 41011 sinusoidal See Sinusoidal input step 40710 Inputoutput description 11119 Instantaneous systems 1034 134 263 Integrals convolution See Convolutional integral discretetime Fourier 85567 Fourier See Fourier integral indefinite 57 of matrices 90910 Integrators digital 25859 ideal 369 370 373 400 41618 system realization and 400 Integrodifferential equations 36073 466 488 Interconnected systems continuoustime 19093 discretetime 29497 Internal conditions continuoustime system response to 15163 discretetime system response to 27076 Internal description of a system 11921 135 908 See also Statespace description of a system Internal stability 110 135 263 BIBO relationship to 199203 3014 of continuoustime systems 196203 22223 of discretetime systems 298302 305 314 526 527 of the Laplace transform 372 of the ztransform 518 Interpolation 78588 of discretetime signals 24344 ideal 78687 simple 78586 spectral 804 Interpolation formula 779 787 Interpolation function 690 Intuitive insights into continuoustime systems 18990 20312 into discretetime systems 3056 into the Laplace transform 36768 Inverse continuoustime systems 19293 Inverse discrete Fourier transform IDFT 808 827 857 Inverse discretetime Fourier transform IDTFT 886 of rectangular spectrum 86566 Inverse discretetime systems 29495 Inverse Fourier transform 683 69395 699 762 78687 Inverse Laplace transform 333 335 445 549 finding 33846 Inverse ztransform 48889 491 499 500 501 510 554 555 559 finding 495 Inversion frequency 706 matrix 4042 Invertible systems 10910 135 263 Irrational numbers 12 Kaiser window 755 76062 Kelvin Lord 348 KennellyHeaviside atmosphere layer 348 Kirchhoffs laws 95 current KCL 111 213 374 voltage KVL 111 374 Kronecker delta functions 245 bandlimited interpolation of 78788 Lary digital signals 799 LHôpitals rule 58 211 690 Lagrange Louis de 347 612 613 Laplace transform 167 330487 721 bilateral See Bilateral Laplace transform differential equation solutions and 34648 36073 electrical network analysis and 37385 467 existence of 33637 Fourier transform connection with 699701 866 intuitive interpretation of 36769 inverse 549 93839 properties of 34962 stability of 37174 state equation solutions by 92733 system realization and 388404 unilateral 33336 337 338 345 360 445 467 ztransform connection with 488 489 491 56365 Laplace transform pairs 333 Laplace Marquis PierreSimon de 34647 611 612 613 Leakage 751 75355 763 8056 Left half plane LHP 91 19899 202 211 223 435 Left shift 71 73 130 134 503 509 510 512 Leftsided sequences 55556 Legendre Fourier series 65657 Leibniz Gottfried Wilhelm 801 Linear convolution 821 Linear dashpots 115 Linear phase distortionless transmission and 725 881 generalized 72627 ideal 725 727 11LathiIndex 2017925 1929 page 982 8 982 Index Linear phase continued physical description of 7079 physical explanation of 87071 Linear springs 114 Linear systems 97101 134 heuristic understanding of 72223 response of 98100 Linear timeinvariant continuoustime LTIC systems See Continuoustime systems Linear timeinvariant discretetime LTID systems See Discretetime systems Linear timeinvariant LTI systems 103 19495 Linear timeinvariant discretetime LTID systems 87980 Linear timevarying systems 103 Linear transformation of vectors 36 93947 961 Linearity of the bilateral Laplace transform 451 of the bilateral ztransform 559 concept of 9798 of the discrete Fourier transform 818 824 of the discretetime Fourier transform 867 of discretetime systems 262 of the Fourier transform 68687 824 of the Laplace transform 33132 of the ztransform 489 Log magnitude 27 42224 Loop currents continuoustime systems and 15963 175 Laplace transform and 375 Lower sideband LSB 73839 747 Lowpass filters 43941 54042 ideal 730 78485 78889 88283 poles and zeros of Hs and 43645 Mfiles 21220 function 21415 script 21314 218 Maclaurin series 6 55 Magnitude response See Amplitude response Marginally stable systems continuoustime 198200 203 211 22224 discretetime 3012 304 314 Laplace transform 373 signal transmission and 721 ztransform 519 Mathematical models of systems 9596 125 MATLAB on Butterworth filters 45963 calculator operations in 4345 on continuoustime filters 45563 on discrete Fourier transform 82734 on discretetime Fourier series and transform 88997 on discretetime systemssignals 30612 elementary operations in 4253 on filtering 30810 Fourier series applications in 66167 Fourier transform topics in 75562 frequency response plots 53132 on functions 12633 impulse invariance 553 impulse response and 167 on infiniteimpulse response filters 56574 Mfiles in 21220 matrix operations in 4953 multiple magnitude response curves 544 partial fraction expansion in 53 periodic functions 66163 phase spectrum 66467 polynomial roots and 157 simple plotting in 4648 statespace analysis in 96169 vector operations in 4546 zeroinput response and 15758 Matrices 3642 algebra of 3842 characteristic equation of 90910 933 characteristic roots of 93233 computing exponential of 91213 definitions and properties of 3738 derivatives of 90910 diagonal 37 diagonalization of 94344 equal 37 functions of 91112 identity 37 impulse response 938 integrals of 90910 inversion of 4042 MATLAB operations 4953 nonsingular 41 square 36 37 41 state transition 936 symmetric 37 transpose of 3738 zero 37 Matrix exponentials 96869 Matrix exponentiation 96869 Mechanical systems 11418 Memory systems and 104 263 Memoryless systems See Instantaneous systems Method of residues 27 Michelson Albert 62021 Minimum phase systems 435 436 Modified partial fractions 35 496 Modulation 71314 73649 amplitude 71113 736 74246 762 angle 736 763 of the discretetime Fourier transform 872 11LathiIndex 2017925 1929 page 983 9 Index 983 doublesideband suppressedcarrier 73741 742 74649 pulseamplitude 796 pulsecode 796 799 pulseposition 796 pulsewidth 796 singlesideband 74649 Moment of inertia 11618 Monotonic exponentials 2022 90 91 134 Multiple inputs 178 28788 Multipleinput multipleoutput MIMO systems 98 125 908 Multiplication bilateral ztransform and 560 of complex numbers 1214 discretetime Fourier transform and 869 of a function by an impulse 87 matrix 3840 scalar 38 4001 505 ztransform and 5067 Natural binary code NBC 799 Natural modes See Characteristic modes Natural numbers 1 Natural response difference equations and 298 differential equations and 196 Negative feedback 406 Negative frequency 62628 Negative numbers 13 45 Neper frequency 91 Neutral equilibrium 197 198 Newton Sir Isaac 2 34647 Noise 66 151 371 417 791 79799 Nonanticipative systems See Causal systems Nonbandlimited signals 792 Noncausal signals 81 Noncausal systems 1047 135 263 properties of 1046 reasons for studying 1067 Noninvertible systems 10910 135 263 Noninverting amplifiers 382 Nonlinear systems 97101 134 Nonsingular matrices 41 Nonuniqueness 533 Normalform equations 915 Norton theorem 375 Notch filters 44143 540 54546 See also Bandstop filters Numerical integration 13133 Nyquist interval 778 779 Nyquist rate 77881 78889 792 795 821 Nyquist samples 778 781 782 788 792 Observability See controllabilityobservability Octave 422 Odd component of a signals 9395 Odd functions 9295 134 Operational amplifiers 38283 399 467 Ordinary numbers 15 Orthogonal signal space 64950 Orthogonal signals 668 energy of the sum of 647 signal representation by set 64759 Orthogonal vector space 64748 Orthogonality 622 Orthonormal sets 649 Oscillators 203 Output 64 97 Output equations 122 124 908 930 941 Overdamped systems 40910 PaleyWiener criterion 444 73132 788 Parallel realization 39394 52526 921 92425 Parallel systems 190 387 Parsevals theorem 632 65152 73435 755 75859 87678 Partial fractions expansion of 2535 53 inverse transform by partial fraction expansion and tables 49598 Laplace transform and 33839 341 344 362 394 395 419 454 modified 35 ztransform 499 Passbands 441 44445 748 755 Peak time 40910 Percent overshoot PO 40910 Periodic circular convolution 81920 of the discretetime Fourier transform 875 Periodic extension of the Fourier spectrum 84855 properties of 8081 Periodic functions Fourier spectra as 858 MATLAB on 66163 Periodic gate function 85355 Periodic signals 133 63740 discretetime Fourier series and 84647 Fourier spectra of 84855 Fourier transform of 69596 properties of 7882 and trigonometric Fourier series 593612 661 Periods fundamental 79 133 23940 593 595 846 sinusoid 16 Phase response 41325 42735 439 467 Phase spectrum 598 607 61718 707 848 MATLAB on 66467 using principal values 70910 11LathiIndex 2017925 1929 page 984 10 984 Index Phaseplane analysis 125 909 Phasors 1820 Physical systems See Causal systems Picket fence effect 807 Pickoff nodes 190 25455 396 Pingala 801 Pointwise convergent series 613 Polar coordinates 56 Polar form 815 arithmetical operations in 1215 sinusoids and 18 Polezero location 53847 Polezero plots 56668 Poles complex 395 432 497 542 controlling gain by 540 firstorder 42427 gain enhancement by 43738 Hs filter design and 43645 at the origin 42223 repeated 395 525 926 in the right half plane 371 43536 secondorder 42635 wall of 43941 542 Polynomial expansion 45859 Polynomial roots 157 572 Positive feedback 406 Power series 55 Power signals 67 82 134 23940 See also Signal power Power determining 6869 matrix 91213 Powers of complex numbers 1316 Practical filters 73033 88283 Preece Sir William 349 Prewarping 57071 Principal values of the angle 9 phase spectrum using 70910 Proper functions 2527 Pulseamplitude modulation PAM 796 Pulsecode modulation PCM 796 799 Pulse dispersion 209 Pulseposition modulation PPM 796 Pulsewidth modulation PWM 796 Pupin M 348 Pythagoras 2 Quadratic equations 58 Quadratic factors 2930 for the Laplace transform 34142 for the ztransform 497 Quantization 799 83134 Quantized levels 799 Radian frequency 16 91 594 Random signals 82 134 Rational functions 2529 338 Real numbers 27 43 Real time 1056 Rectangular pulses 86365 Rectangular spectrum 86566 Rectangular windows 751 75355 763 Reflection property 86869 Region of convergence ROC for continuoustime systems 193 for finiteduration signals 333 for the Laplace transform 33133 337 347 448 449 45455 467 for the ztransform 48991 55558 561 Relational operators 12829 Repeated factors of Qx 3132 Repeated poles 395 525 926 Repeated roots of continuoustime systems 15456 195 198 202 223 of discretetime systems 270 27374 297 301 31314 Resonance phenomenon 163 204 205 21012 305 Right half plane RHP 91 198 2003 223 371 43536 Right shift 7172 131 134 5014 509 510 Rightsided sequences 55556 Rise time 2067 405 40910 411 RLC networks 914 91618 RMS value 6869 70 Rolloff rate 753 754 Roots complex 15456 27476 of complex numbers 1115 polynomial 157 572 repeated See Repeated roots unrepeated 198 202 223 301 314 Rotational systems 11619 Rotational mass See Moment of inertia Row vectors 36 45 4850 Sales estimate example 25556 SallenKey circuit 383 384 46162 463 466 Sampled continuoustime sinusoids 52731 Sampling 776844 practical 78184 properties of 8788 134 signal reconstruction and 78599 spectral 75960 8024 See also Discrete Fourier transform FastFourier transform Sampling interval 55054 Sampling rate 24344 53637 Sampling theorem 537 77684 83435 applications of 79699 spectral 802 Savings account example 25355 11LathiIndex 2017925 1929 page 985 11 Index 985 Scalar multiplication 38 4001 505 509 520 Scaling 9798 130 of the Fourier transform 7056 755 757 762 of the Laplace transform 357 See also Time scaling Script Mfiles 21314 216 218 Selectivefiltering method 74849 Sharp cutoff filters 748 Shifting of the bilateral ztransform 559 of the convolution integral 17172 of the convolution sum 283 of discretetime signals 240 See also Frequency shifting Time shifting Sideband 74649 sifting See Sampling Signal distortion 72325 Signal energy 6566 70 13133 73336 757 87778 See also Energy signals Signal power 6567 133 See also Power signals Signal reconstruction 78599 See also Interpolation Signaltonoise power ratio 66 Signal transmission 72129 Signals 6491 13334 analog See Analog signals anticausal 81 aperiodic See Aperiodic signals audio 71314 725 746 bandlimited 533 788 792 802 baseband 73740 74647 749 basis 651 655 668 causal 81 83 134 classification of 7882 13334 comparison and components of 64345 complex 9495 continuous time See continuoustime signals defined 65 deterministic 83 134 digital See Digital signals discrete time See Discretetime signals energy 82 134 23940 error 65051 even components of 9395 everlasting 81 134 finiteduration 333 modulating 711 73739 nonbandlimited 792 noncausal 81 odd components of 9395 orthogonal See Orthogonal signals periodic See Periodic signals phantoms of 189 power 82 134 23940 random 82 134 size of 6470 133 sketching 2023 time reversal of 77 time limited 802 805 807 twodimensional view of 73233 useful models 8291 useful operations 7178 as vectors 64159 video 725 749 Sinc function 757 Singleinput singleoutput SISO systems 98 125 908 Singlesideband SSB modulation 74649 Singularity functions 89 Sinusoidal input causal See Causal sinusoidal input continuoustime systems and 208 discretetime systems and 309 frequency response and 41317 steadystate response to causal sinusoidal input 41819 Sinusoids 1620 8991 134 addition of 1820 apparent frequency of sampled 79596 compression and expansion 76 continuoustime 25152 53337 discretetime 251 527 528 53337 discretetime Fourier series of 84952 in exponential terms 20 exponentially varying 2223 80 134 general condition for aliasing in 79396 power of a sum of two equalfrequency 70 sampled continuoustime 52731 verification of aliasing in 79293 Sketching signals 2023 Slidingtape method 29093 Software realization 64 95 133 Spectral density 688 Spectral folding See Aliasing Spectral interpolation 804 Spectral resolution 807 Spectral sampling 75960 802 Spectral sampling theorem 802 Spectral spreading 75153 755 763 807 Springs linear 114 torsional 11617 Square matrices 36 37 41 Square roots of negative numbers 24 Stability BIBO See Boundedinputboundedoutput stability of continuoustime systems 196203 22223 of discretetime systems 263 298305 314 of the Laplace transform 37174 Internal See Internal stability of the ztransform 51819 marginal See marginally stable systems 11LathiIndex 2017925 1929 page 986 12 986 Index Stable equilibrium 19697 Stable systems 110 263 State equations 12225 135 9089 969 alternative procedure to determine 91819 diagonal form of 94447 solution of 92639 for the state vector 94142 systematic procedure for determining 91326 timedomain method to solve 93637 State transition matrix STM 936 State variables 12125 135 908 969 State vectors 92730 961 linear transformation of 94142 Statespace analysis 90873 controllabilityobservability in 94753 961 of discretetime systems 95364 in MATLAB 96169 transfer function and 92024 transfer function matrix 93132 Statespace description of a system 12125 Steadystate error 40911 Steadystate response in continuoustime systems 41819 in discretetime systems 527 Stem plots 3068 Step input 40710 Stiffness of linear springs 114 of torsional springs 11617 Stopbands 441 444 445 456 457 459 460 463 755 Subcarriers 749 Subtraction of complex numbers 1112 Superposition 98 99 100 123 134 continuoustime systems and 168 170 178 discretetime systems and 287 Symmetric matrices 37 Symmetry conjugate See Conjugate symmetry exponential Fourier series and 63032 trigonometric Fourier series and 6078 Synchronous demodulation 74344 747 System realization 388404 51925 567 cascade 394 52526 91920 923 of complex conjugate poles 395 direct See Direct form I realization Direct form II realization differences in performance 52526 hardware 64 95 133 parallel See Parallel realization software 64 95 129 Systems 95133 13435 accumulator 259 295 519 analog 109 135 261 backward difference 258 295 519 56869 BIBO stability assessing 110 cascade 190 192 372 373 causal See causal systems causality assessing 105 classification of 97110 13435 continuous time See Continuoustime systems control See control systems critically damped 409 410 data for computing response 9697 defined 64 digital 78 135 261 discrete time See discrete time systems dynamic 1034 13435 263 electrical 9596 11114 electrical See Electrical systems electromechanical 11819 feedback See feedback systems finitememory 104 identity 109 192 263 inputoutput description 11119 instantaneous 1034 263 interconnected See interconnected systems invertible 10910 135 263 linear See Linear systems mathematical models of 9596 125 mechanical 11418 memory and 104 263 minimum phase 435 436 multipleinput multipleoutput 98 125 908 noncausal 1047 263 noninvertible 10910 135 nonlinear 97101 134 overdamped 40910 parallel 190 387 phantoms of 189 properties of 26465 rotational 11619 singleinput singleoutput 98 125 908 stable 110 263 translational 11416 time invariant See Timeinvariant systems time varying See Timevarying systems twodimensional view of 73233 underdamped 409 unstable 110 263 Tacoma Narrows Bridge failure 212 Tapered windows 75354 763 807 Taylor series 55 Théorie analytique de la chaleur Fourier 612 Thévenins theorem 375 378 379 Time constant of continuoustime systems 20510 223 of the exponential 2122 filtering and 2079 information transmission rate and 20910 11LathiIndex 2017925 1929 page 987 13 Index 987 pulse dispersion and 209 rise time and 2067 Time convolution of the bilateral Laplace transform 452 of the discretetime Fourier transform 87576 of the Fourier transform 71416 of the Laplace transform 357 of the ztransform 5078 Time delay variation with frequency 72425 Time differentiation of the bilateral Laplace transform 451 of the Fourier transform 71618 of the Laplace transform 35456 Time integration of the bilateral Laplace transform 451 of the Fourier transform 71618 of the Laplace transform 35657 Time inversion 706 Time reversal 134 of the bilateral Laplace transform 452 of the bilateral ztransform 560 of the convolution integral 178 181 described 7677 of the discretetime Fourier transform 86869 of discretetime signals 242 of the ztransform 5067 Time scaling 77 of the bilateral Laplace transform 452 described 7374 Time shifting 77 79 of the bilateral Laplace transform 451 of the convolution integral 178 described 7173 of the discrete Fourier transform 819 of the discretetime Fourier transform 870 of the Fourier transform 707 of the Laplace transform 34951 of the ztransform 5015 510 Timedivision multiplexing TDM 749 797 Timedomain analysis 723 of continuoustime systems 150236 of discretetime systems 237329 of the Fourier series 598 601 of interpolation 78588 state equation solution in 93339 twodimensional view and 73233 Timefrequency duality 7023 723 753 Time invariant systems 134 discretetime 262 linear See Linear timeinvariant systems properties of 1023 Timevarying systems 134 discretetime 262 linear 103 properties of 1023 Timelimited signals 802 805 807 Torque 11618 Torsional dashpots 116 Torsional springs 116 117 Total response of continuoustime systems 19596 of discretetime systems 29798 Traité de mécanique céleste Laplace 346 Transfer functions 522 analog filter realization with 54849 block diagrams and 38688 of continuoustime systems 19394 222 of discretetime systems 29697 314 51415 56768 from the frequency response 435 inadequacy for system description 953 realization of 38999 401 52425 state equations from 916 91926 from statespace representations 96465 Translational systems 11416 Transpose of a matrix 3738 Transposed direct form II TDFII realization 398 96769 state equations and 92024 ztransform and 52022 52526 Triangular windows 751 Trigonometric Fourier series 640 652 65758 667 668 exponential 62137 661 periodic signals and 593612 661 sampling and 777 782 symmetry effect on 6078 Trigonometric identities 5556 Tukey J W 824 Underdamped systems 409 Uniformly convergent series 613 Unilateral Laplace transform 33336 337 338 345 360 445 467 Unilateral ztransform 489 491 492 495 55455 559 Uniqueness 335 Unit delay 517 520 521 Unitgate function 689 Unitimpulse function 133 of discretetime systems 24647 280 313 as a generalized function 8889 properties of 8689 Unitimpulse response of continuoustime systems 16368 170 18993 22021 222 731 convolution with 171 determining 221 of discretetime systems 27780 286 295 313 Unit matrices 37 Unitstep function 8486 8889 of discretetime systems 24647 relational operators and 12830 11LathiIndex 2017925 1929 page 988 14 988 Index Unittriangle function 68990 Unrepeated roots 198 202 223 301 314 Unstable equilibrium 19697 Unstable systems 110 263 Upper sideband USB 73739 74648 Upsampling 24344 Vectors 3637 64159 basis 648 characteristic 910 column 36 components of 64243 error 642 MATLAB operations 4546 matrix multiplication by 40 orthogonal space 64748 row 36 45 4850 signals as 64159 state 92730 961 Vestigial sideband VSB 749 Video signals 725 749 Waveshaping 61517 WeberFechner law 421 Width of the convolution integral 172 187 of the convolution sum 283 Window functions 74955 76062 ztransform 488592 bilateral See Bilateral ztransform difference equation solutions of 488 51019 574 direct 488592 discretetime Fourier transform and 86667 88688 898 existence of 49195 inverse See inverse ztransform properties of 5019 stability of 51819 statespace analysis and 956 95965 system realization and 51925 567 timereversal property 5067 timeshifting properties 5015 unilateral 489 491 492 495 55455 559 zdomain differentiation property 506 zdomain scaling property 505 Zero matrices 37 Zero padding 81011 82930 Zeroinput response 119 123 of continuoustime systems 15163 19596 203 22022 described 98100 of discretetime systems 27076 297301 30911 insights into behavior of 16163 of the Laplace transform 363 368 in oscillators 203 of the ztransform 51213 zerostate response independence from 161 Zeroorder hold ZOH filters 785 Zerostate response 119 123 alternate interpretation 51518 causality and 17273 of continuoustime systems 151 161 16896 22122 51216 described 98101 of discretetime systems 28098 3089 311 312 313 of the Laplace transform 358 363 36667 369 370 zeroinput response independence from 161 Zeros controlling gain by 540 filter design 43645 firstorder 42427 gain suppression by 43940 at the origin 42223 secondorder 42635 11LathiIndex 2017925 1929 page 989 15 11LathiIndex 2017925 1929 page 990 16
Envie sua pergunta para a IA e receba a resposta na hora
Recomendado para você
592
Sinais e Sistemas - 2ª Edição
Sinais e Sistemas
CEFET/RJ
30
Princípios de Telecomunicações: Aula 5 - Escala Logarítmica e Decibéis
Sinais e Sistemas
CEFET/RJ
1
Cálculo da Energia dos Sinais
Sinais e Sistemas
CEFET/RJ
1
Referências Bibliográficas
Sinais e Sistemas
CEFET/RJ
55
Princípios de Telecomunicações - Aulas 2 e 3
Sinais e Sistemas
CEFET/RJ
526
Schaum's Outline of Signals and Systems
Sinais e Sistemas
CEFET/RJ
794
Signals and Systems Analysis Using Transform Methods and MATLAB
Sinais e Sistemas
CEFET/RJ
1
Referências de Exercícios e Questões
Sinais e Sistemas
CEFET/RJ
31
Princípios de Telecomunicações - Aula 7: Transformada de Fourier
Sinais e Sistemas
CEFET/RJ
853
Sinais e Sistemas Lineares - B.P. Lathi
Sinais e Sistemas
CEFET/RJ
Texto de pré-visualização
THIRD EDITION LINEAR SYSTEMS AND SIGNALS BP Lathi ROGER GREEN OXFORD UNIVERSITY PRESS 00LathiPrelims 2017928 943 page i 1 LINEAR SYSTEMS AND SIGNALS 00LathiPrelims 2017928 943 page ii 2 T H E O X F O R D S E R I E S I N E L E C T R I C A L AND COMPUTER ENGINEERING Adel S Sedra Series Editor Allen and Holberg CMOS Analog Circuit Design 3rd edition Boncelet Probability Statistics and Random Signals Bobrow Elementary Linear Circuit Analysis 2nd edition Bobrow Fundamentals of Electrical Engineering 2nd edition Campbell Fabrication Engineering at the Micro and Nanoscale 4th edition Chen Digital Signal Processing Chen Linear System Theory and Design 4th edition Chen Signals and Systems 3rd edition Comer Digital Logic and State Machine Design 3rd edition Comer MicroprocessorBased System Design Cooper and McGillem Probabilistic Methods of Signal and System Analysis 3rd edition Dimitrijev Principles of Semiconductor Device 2nd edition Dimitrijev Understanding Semiconductor Devices Fortney Principles of Electronics Analog Digital Franco Electric Circuits Fundamentals Ghausi Electronic Devices and Circuits Discrete and Integrated Guru and Hiziroglu Electric Machinery and Transformers 3rd edition Houts Signal Analysis in Linear Systems Jones Introduction to Optical Fiber Communication Systems Krein Elements of Power Electronics 2nd Edition Kuo Digital Control Systems 3rd edition Lathi and Green Linear Systems and Signals 3rd edition Lathi and Ding Modern Digital and Analog Communication Systems 5th edition Lathi Signal Processing and Linear Systems Martin Digital Integrated Circuit Design Miner Lines and Electromagnetic Fields for Engineers Mitra Signals and Systems Parhami Computer Architecture Parhami Computer Arithmetic 2nd edition Roberts and Sedra SPICE 2nd edition Roberts Taenzler and Burns An Introduction to MixedSignal IC Test and Measurement 2nd edition Roulston An Introduction to the Physics of Semiconductor Devices Sadiku Elements of Electromagnetics 7th edition Santina Stubberud and Hostetter Digital Control System Design 2nd edition Sarma Introduction to Electrical Engineering Schaumann Xiao and Van Valkenburg Design of Analog Filters 3rd edition Schwarz and Oldham Electrical Engineering An Introduction 2nd edition Sedra and Smith Microelectronic Circuits 7th edition Stefani Shahian Savant and Hostetter Design of Feedback Control Systems 4th edition Tsividis Operation and Modeling of the MOS Transistor 3rd edition Van Valkenburg Analog Filter Design Warner and Grung Semiconductor Device Electronics Wolovich Automatic Control Systems Yariv and Yeh Photonics Optical Electronics in Modern Communications 6th edition Zak Systems and Control 00LathiPrelims 2017928 943 page iii 3 LINEAR SYSTEMS AND SIGNALS THIRD EDITION B P Lathi and R A Green New York Oxford OXFORD UNIVERSITY PRESS 2018 00LathiPrelims 2017928 943 page iv 4 Oxford University Press is a department of the University of Oxford It furthers the Universitys objective of excellence in research scholarship and education by publishing worldwide Oxford New York Auckland Cape Town Dar es Salaam Hong Kong Karachi Kuala Lumpur Madrid Melbourne Mexico City Nairobi New Delhi Shanghai Taipei Toronto With offices in Argentina Austria Brazil Chile Czech Republic France Greece Guatemala Hungary Italy Japan Poland Portugal Singapore South Korea Switzerland Thailand Turkey Ukraine Vietnam Copyright c 2018 by Oxford University Press For titles covered by Section 112 of the US Higher Education Opportunity Act please visit wwwoupcomushe for the latest information about pricing and alternate formats Published by Oxford University Press 198 Madison Avenue New York NY 10016 httpwwwoupcom Oxford is a registered trademark of Oxford University Press All rights reserved No part of this publication may be reproduced stored in a retrieval system or transmitted in any form or by any means electronic mechanical photocopying recording or otherwise without the prior permission of Oxford University Press Library of Congress CataloginginPublication Data Names Lathi B P Bhagwandas Pannalal author Green R A Roger A author Title Linear systems and signals BP Lathi and RA Green Description Third Edition New York Oxford University Press 2018 Series The Oxford Series in Electrical and Computer Engineering Identifiers LCCN 2017034962 ISBN 9780190200176 hardcover acidfree paper Subjects LCSH Signal processingMathematics System analysis Linear time invariant systems Digital filters Mathematics Classification LCC TK51025 L298 2017 DDC 6213822dc23 LC record available at httpslccnlocgov2017034962 ISBN 9780190200176 Printing number 9 8 7 6 5 4 3 2 1 Printed by RR Donnelly in the United States of America 00LathiPrelims 2017928 943 page v 5 CONTENTS PREFACE xv B BACKGROUND B1 Complex Numbers 1 B11 A Historical Note 1 B12 Algebra of Complex Numbers 5 B2 Sinusoids 16 B21 Addition of Sinusoids 18 B22 Sinusoids in Terms of Exponentials 20 B3 Sketching Signals 20 B31 Monotonic Exponentials 20 B32 The Exponentially Varying Sinusoid 22 B4 Cramers Rule 23 B5 Partial Fraction Expansion 25 B51 Method of Clearing Fractions 26 B52 The Heaviside CoverUp Method 27 B53 Repeated Factors of Qx 31 B54 A Combination of Heaviside CoverUp and Clearing Fractions 32 B55 Improper Fx with m n 34 B56 Modified Partial Fractions 35 B6 Vectors and Matrices 36 B61 Some Definitions and Properties 37 B62 Matrix Algebra 38 B7 MATLAB Elementary Operations 42 B71 MATLAB Overview 42 B72 Calculator Operations 43 B73 Vector Operations 45 B74 Simple Plotting 46 B75 ElementbyElement Operations 48 B76 Matrix Operations 49 B77 Partial Fraction Expansions 53 B8 Appendix Useful Mathematical Formulas 54 B81 Some Useful Constants 54 v 00LathiPrelims 2017928 943 page vi 6 vi Contents B82 Complex Numbers 54 B83 Sums 54 B84 Taylor and Maclaurin Series 55 B85 Power Series 55 B86 Trigonometric Identities 55 B87 Common Derivative Formulas 56 B88 Indefinite Integrals 57 B89 LHôpitals Rule 58 B810 Solution of Quadratic and Cubic Equations 58 References 58 Problems 59 1 SIGNALS AND SYSTEMS 11 Size of a Signal 64 111 Signal Energy 65 112 Signal Power 65 12 Some Useful Signal Operations 71 121 Time Shifting 71 122 Time Scaling 73 123 Time Reversal 76 124 Combined Operations 77 13 Classification of Signals 78 131 ContinuousTime and DiscreteTime Signals 78 132 Analog and Digital Signals 78 133 Periodic and Aperiodic Signals 79 134 Energy and Power Signals 82 135 Deterministic and Random Signals 82 14 Some Useful Signal Models 82 141 The Unit Step Function ut 83 142 The Unit Impulse Function δt 86 143 The Exponential Function est 89 15 Even and Odd Functions 92 151 Some Properties of Even and Odd Functions 92 152 Even and Odd Components of a Signal 93 16 Systems 95 17 Classification of Systems 97 171 Linear and Nonlinear Systems 97 172 TimeInvariant and TimeVarying Systems 102 173 Instantaneous and Dynamic Systems 103 174 Causal and Noncausal Systems 104 175 ContinuousTime and DiscreteTime Systems 107 176 Analog and Digital Systems 109 177 Invertible and Noninvertible Systems 109 178 Stable and Unstable Systems 110 00LathiPrelims 2017928 943 page vii 7 Contents vii 18 System Model InputOutput Description 111 181 Electrical Systems 111 182 Mechanical Systems 114 183 Electromechanical Systems 118 19 Internal and External Descriptions of a System 119 110 Internal Description The StateSpace Description 121 111 MATLAB Working with Functions 126 1111 Anonymous Functions 126 1112 Relational Operators and the Unit Step Function 128 1113 Visualizing Operations on the Independent Variable 130 1114 Numerical Integration and Estimating Signal Energy 131 112 Summary 133 References 135 Problems 136 2 TIMEDOMAIN ANALYSIS OF CONTINUOUSTIME SYSTEMS 21 Introduction 150 22 System Response to Internal Conditions The ZeroInput Response 151 221 Some Insights into the ZeroInput Behavior of a System 161 23 The Unit Impulse Response ht 163 24 System Response to External Input The ZeroState Response 168 241 The Convolution Integral 170 242 Graphical Understanding of Convolution Operation 178 243 Interconnected Systems 190 244 A Very Special Function for LTIC Systems The Everlasting Exponential est 193 245 Total Response 195 25 System Stability 196 251 External BIBO Stability 196 252 Internal Asymptotic Stability 198 253 Relationship Between BIBO and Asymptotic Stability 199 26 Intuitive Insights into System Behavior 203 261 Dependence of System Behavior on Characteristic Modes 203 262 Response Time of a System The System Time Constant 205 263 Time Constant and Rise Time of a System 206 264 Time Constant and Filtering 207 265 Time Constant and Pulse Dispersion Spreading 209 266 Time Constant and Rate of Information Transmission 209 267 The Resonance Phenomenon 210 27 MATLAB MFiles 212 271 Script MFiles 213 272 Function MFiles 214 3 TIMEDOMAIN ANALYSIS OF DISCRETETIME SYSTEMS 237 31 Introduction 237 311 Size of a DiscreteTime Signal 238 32 Useful Signal Operations 240 33 Some Useful DiscreteTime Signal Models 245 331 DiscreteTime Impulse Function δn 245 332 DiscreteTime Unit Step Function un 246 333 DiscreteTime Exponential γn 247 334 DiscreteTime Sinusoid cosΩn θ 251 335 DiscreteTime Complex Exponential eγn 252 34 Examples of DiscreteTime Systems 253 341 Classification of DiscreteTime Systems 262 35 DiscreteTime System Equations 265 351 Recursive Iterative Solution of Difference Equation 266 36 System Response to Internal Conditions The ZeroInput Response 270 37 The Unit Impulse Response hn 277 371 The ClosedForm Solution of hn 278 38 System Response to External Input The ZeroState Response 280 381 Graphical Procedure for the Convolution Sum 288 382 Interconnected Systems 294 383 Total Response 297 39 System Stability 298 391 External BIBO Stability 298 392 Internal Asymptotic Stability 299 393 Relationship Between BIBO and Asymptotic Stability 301 310 Intuitive Insights into System Behavior 305 311 MATLAB DiscreteTime Signals and Systems 306 3111 DiscreteTime Functions and Stem Plots 306 3112 System Responses Through Filtering 308 3113 A Custom Filter Function 310 3114 DiscreteTime Convolution 311 312 Appendix Impulse Response for a Special Case 313 313 Summary 313 Problems 314 00LathiPrelims 2017928 943 page ix 9 Contents ix 4 CONTINUOUSTIME SYSTEM ANALYSIS USING THE LAPLACE TRANSFORM 41 The Laplace Transform 330 411 Finding the Inverse Transform 338 42 Some Properties of the Laplace Transform 349 421 Time Shifting 349 422 Frequency Shifting 353 423 The TimeDifferentiation Property 354 424 The TimeIntegration Property 356 425 The Scaling Property 357 426 Time Convolution and Frequency Convolution 357 43 Solution of Differential and IntegroDifferential Equations 360 431 Comments on Initial Conditions at 0 and at 0 363 432 ZeroState Response 366 433 Stability 371 434 Inverse Systems 373 44 Analysis of Electrical Networks The Transformed Network 373 441 Analysis of Active Circuits 382 45 Block Diagrams 386 46 System Realization 388 461 Direct Form I Realization 389 462 Direct Form II Realization 390 463 Cascade and Parallel Realizations 393 464 Transposed Realization 396 465 Using Operational Amplifiers for System Realization 399 47 Application to Feedback and Controls 404 471 Analysis of a Simple Control System 406 48 Frequency Response of an LTIC System 412 481 SteadyState Response to Causal Sinusoidal Inputs 418 49 Bode Plots 419 491 Constant Ka1a2b1b3 422 492 Pole or Zero at the Origin 422 493 FirstOrder Pole or Zero 424 494 SecondOrder Pole or Zero 426 495 The Transfer Function from the Frequency Response 435 410 Filter Design by Placement of Poles and Zeros of Hs 436 4101 Dependence of Frequency Response on Poles and Zeros of Hs 436 4102 Lowpass Filters 439 4103 Bandpass Filters 441 4104 Notch Bandstop Filters 441 4105 Practical Filters and Their Specifications 444 411 The Bilateral Laplace Transform 445 00LathiPrelims 2017928 943 page x 10 x Contents 4111 Properties of the Bilateral Laplace Transform 451 4112 Using the Bilateral Transform for Linear System Analysis 452 412 MATLAB ContinuousTime Filters 455 4121 Frequency Response and Polynomial Evaluation 456 4122 Butterworth Filters and the Find Command 459 4123 Using Cascaded SecondOrder Sections for Butterworth Filter Realization 461 4124 Chebyshev Filters 463 413 Summary 466 References 468 Problems 468 5 DISCRETETIME SYSTEM ANALYSIS USING THE zTRANSFORM 51 The zTransform 488 511 Inverse Transform by Partial Fraction Expansion and Tables 495 512 Inverse zTransform by Power Series Expansion 499 52 Some Properties of the zTransform 501 521 TimeShifting Properties 501 522 zDomain Scaling Property Multiplication by γ n 505 523 zDomain Differentiation Property Multiplication by n 506 524 TimeReversal Property 506 525 Convolution Property 507 53 zTransform Solution of Linear Difference Equations 510 531 ZeroState Response of LTID Systems The Transfer Function 514 532 Stability 518 533 Inverse Systems 519 54 System Realization 519 55 Frequency Response of DiscreteTime Systems 526 551 The Periodic Nature of Frequency Response 532 552 Aliasing and Sampling Rate 536 56 Frequency Response from PoleZero Locations 538 57 Digital Processing of Analog Signals 547 58 The Bilateral zTransform 554 581 Properties of the Bilateral zTransform 559 582 Using the Bilateral zTransform for Analysis of LTID Systems 560 59 Connecting the Laplace and zTransforms 563 510 MATLAB DiscreteTime IIR Filters 565 5101 Frequency Response and PoleZero Plots 566 5102 Transformation Basics 567 5103 Transformation by FirstOrder Backward Difference 568 5104 Bilinear Transformation 569 5105 Bilinear Transformation with Prewarping 570 5106 Example Butterworth Filter Transformation 571 00LathiPrelims 2017928 943 page xi 11 Contents xi 5107 Problems Finding Polynomial Roots 572 5108 Using Cascaded SecondOrder Sections to Improve Design 572 511 Summary 574 References 575 Problems 575 6 CONTINUOUSTIME SIGNAL ANALYSIS THE FOURIER SERIES 61 Periodic Signal Representation by Trigonometric Fourier Series 593 611 The Fourier Spectrum 598 612 The Effect of Symmetry 607 613 Determining the Fundamental Frequency and Period 609 62 Existence and Convergence of the Fourier Series 612 621 Convergence of a Series 613 622 The Role of Amplitude and Phase Spectra in Waveshaping 615 63 Exponential Fourier Series 621 631 Exponential Fourier Spectra 624 632 Parsevals Theorem 632 633 Properties of the Fourier Series 635 64 LTIC System Response to Periodic Inputs 637 65 Generalized Fourier Series Signals as Vectors 641 651 Component of a Vector 642 652 Signal Comparison and Component of a Signal 643 653 Extension to Complex Signals 645 654 Signal Representation by an Orthogonal Signal Set 647 66 Numerical Computation of Dn 659 67 MATLAB Fourier Series Applications 661 671 Periodic Functions and the Gibbs Phenomenon 661 672 Optimization and Phase Spectra 664 68 Summary 667 References 668 Problems 669 7 CONTINUOUSTIME SIGNAL ANALYSIS THE FOURIER TRANSFORM 71 Aperiodic Signal Representation by the Fourier Integral 680 711 Physical Appreciation of the Fourier Transform 687 72 Transforms of Some Useful Functions 689 721 Connection Between the Fourier and Laplace Transforms 700 73 Some Properties of the Fourier Transform 701 74 Signal Transmission Through LTIC Systems 721 741 Signal Distortion During Transmission 723 742 Bandpass Systems and Group Delay 726 00LathiPrelims 2017928 943 page xii 12 xii Contents 75 Ideal and Practical Filters 730 76 Signal Energy 733 77 Application to Communications Amplitude Modulation 736 771 DoubleSideband SuppressedCarrier DSBSC Modulation 737 772 Amplitude Modulation AM 742 773 SingleSideband Modulation SSB 746 774 FrequencyDivision Multiplexing 749 78 Data Truncation Window Functions 749 781 Using Windows in Filter Design 755 79 MATLAB Fourier Transform Topics 755 791 The Sinc Function and the Scaling Property 757 792 Parsevals Theorem and Essential Bandwidth 758 793 Spectral Sampling 759 794 Kaiser Window Functions 760 710 Summary 762 References 763 Problems 764 8 SAMPLING THE BRIDGE FROM CONTINUOUS TO DISCRETE 81 The Sampling Theorem 776 811 Practical Sampling 781 82 Signal Reconstruction 785 821 Practical Difficulties in Signal Reconstruction 788 822 Some Applications of the Sampling Theorem 796 83 AnalogtoDigital AD Conversion 799 84 Dual of Time Sampling Spectral Sampling 802 85 Numerical Computation of the Fourier Transform The Discrete Fourier Transform 805 851 Some Properties of the DFT 818 852 Some Applications of the DFT 820 86 The Fast Fourier Transform FFT 824 87 MATLAB The Discrete Fourier Transform 827 871 Computing the Discrete Fourier Transform 827 872 Improving the Picture with Zero Padding 829 873 Quantization 831 88 Summary 834 References 835 Problems 835 00LathiPrelims 2017928 943 page xiii 13 Contents xiii 9 FOURIER ANALYSIS OF DISCRETETIME SIGNALS 91 DiscreteTime Fourier Series DTFS 845 911 Periodic Signal Representation by DiscreteTime Fourier Series 846 912 Fourier Spectra of a Periodic Signal xn 848 92 Aperiodic Signal Representation by Fourier Integral 855 921 Nature of Fourier Spectra 858 922 Connection Between the DTFT and the zTransform 866 93 Properties of the DTFT 867 94 LTI DiscreteTime System Analysis by DTFT 878 941 Distortionless Transmission 880 942 Ideal and Practical Filters 882 95 DTFT Connection with the CTFT 883 951 Use of DFT and FFT for Numerical Computation of the DTFT 885 96 Generalization of the DTFT to the zTransform 886 97 MATLAB Working with the DTFS and the DTFT 889 971 Computing the DiscreteTime Fourier Series 889 972 Measuring Code Performance 891 973 FIR Filter Design by Frequency Sampling 892 98 Summary 898 Reference 898 Problems 899 10 STATESPACE ANALYSIS 101 Mathematical Preliminaries 909 1011 Derivatives and Integrals of a Matrix 909 1012 The Characteristic Equation of a Matrix The CayleyHamilton Theorem 910 1013 Computation of an Exponential and a Power of a Matrix 912 102 Introduction to State Space 913 103 A Systematic Procedure to Determine State Equations 916 1031 Electrical Circuits 916 1032 State Equations from a Transfer Function 919 104 Solution of State Equations 926 1041 Laplace Transform Solution of State Equations 927 1042 TimeDomain Solution of State Equations 933 105 Linear Transformation of a State Vector 939 1051 Diagonalization of Matrix A 943 106 Controllability and Observability 947 1061 Inadequacy of the Transfer Function Description of a System 953 00LathiPrelims 2017928 943 page xiv 14 xiv Contents 107 StateSpace Analysis of DiscreteTime Systems 953 1071 Solution in State Space 955 1072 The zTransform Solution 959 108 MATLAB Toolboxes and StateSpace Analysis 961 1081 zTransform Solutions to DiscreteTime StateSpace Systems 961 1082 Transfer Functions from StateSpace Representations 964 1083 Controllability and Observability of DiscreteTime Systems 965 1084 Matrix Exponentiation and the Matrix Exponential 968 109 Summary 969 References 970 Problems 970 INDEX 975 00LathiPrelims 2017928 943 page xv 15 PREFACE This book Linear Systems and Signals presents a comprehensive treatment of signals and linear systems at an introductory level Following our preferred style it emphasizes a physical appreciation of concepts through heuristic reasoning and the use of metaphors analogies and creative explanations Such an approach is much different from a purely deductive technique that uses mere mathematical manipulation of symbols There is a temptation to treat engineering subjects as a branch of applied mathematics Such an approach is a perfect match to the public image of engineering as a dry and dull discipline It ignores the physical meaning behind various derivations and deprives students of intuitive grasp and the enjoyable experience of logical uncovering of the subject matter In this book we use mathematics not so much to prove axiomatic theory as to support and enhance physical and intuitive understanding Wherever possible theoretical results are interpreted heuristically and are enhanced by carefully chosen examples and analogies This third edition which closely follows the organization of the second edition has been refined in many ways Discussions are streamlined adding or trimming material as needed Equation example and section labeling is simplified and improved Computer examples are fully updated to reflect the most current version of MATLAB Hundreds of added problems provide new opportunities to learn and understand topics We have taken special care to improve the text without the topic creep and bloat that commonly occurs with each new edition of a text NOTABLE FEATURES The notable features of this book include the following 1 Intuitive and heuristic understanding of the concepts and physical meaning of mathematical results are emphasized throughout Such an approach not only leads to deeper appreciation and easier comprehension of the concepts but also makes learning enjoyable for students 2 Often students lack an adequate background in basic material such as complex numbers sinusoids handsketching of functions Cramers rule partial fraction expansion and matrix algebra We include a background chapter that addresses these basic and pervasive topics in electrical engineering Response by students has been unanimously enthusiastic 3 There are hundreds of worked examples in addition to drills usually with answers for students to test their understanding Additionally there are over 900 endofchapter problems of varying difficulty 4 Modern electrical engineering practice requires the use of computer calculation and simulation most often using the software package MATLAB Thus we integrate xv 00LathiPrelims 2017928 943 page xvi 16 xvi Preface MATLAB into many of the worked examples throughout the book Additionally each chapter concludes with a section devoted to learning and using MATLAB in the context and support of book topics Problem sets also contain numerous computer problems 5 The discretetime and continuoustime systems may be treated in sequence or they may be integrated by using a parallel approach 6 The summary at the end of each chapter proves helpful to students in summing up essential developments in the chapter 7 There are several historical notes to enhance students interest in the subject This information introduces students to the historical background that influenced the development of electrical engineering ORGANIZATION The book may be conceived as divided into five parts 1 Introduction Chs B and 1 2 Timedomain analysis of linear timeinvariant LTI systems Chs 2 and 3 3 Frequencydomain transform analysis of LTI systems Chs 4 and 5 4 Signal analysis Chs 6 7 8 and 9 5 Statespace analysis of LTI systems Ch 10 The organization of the book permits much flexibility in teaching the continuoustime and discretetime concepts The natural sequence of chapters is meant to integrate continuoustime and discretetime analysis It is also possible to use a sequential approach in which all the continuoustime analysis is covered first Chs 1 2 4 6 7 and 8 followed by discretetime analysis Chs 3 5 and 9 SUGGESTIONS FOR USING THIS BOOK The book can be readily tailored for a variety of courses spanning 30 to 45 lecture hours Most of the material in the first eight chapters can be covered at a brisk pace in about 45 hours The book can also be used for a 30lecturehour course by covering only analog material Chs 1 2 4 6 7 and possibly selected topics in Ch 8 Alternately one can also select Chs 1 to 5 for courses purely devoted to systems analysis or transform techniques To treat continuous and discretetime systems by using an integrated or parallel approach the appropriate sequence of chapters is 1 2 3 4 5 6 7 and 8 For a sequential approach where the continuoustime analysis is followed by discretetime analysis the proper chapter sequence is 1 2 4 6 7 8 3 5 and possibly 9 depending on the time available MATLAB MATLAB is a sophisticated language that serves as a powerful tool to better understand engineering topics including control theory filter design and of course linear systems and signals MATLABs flexible programming structure promotes rapid development and analysis Outstanding visualization capabilities provide unique insight into system behavior and signal character 00LathiPrelims 2017928 943 page xvii 17 Preface xvii As with any language learning MATLAB is incremental and requires practice This book provides two levels of exposure to MATLAB First MATLAB is integrated into many examples throughout the text to reinforce concepts and perform various computations These examples utilize standard MATLAB functions as well as functions from the control system signalprocessing and symbolic math toolboxes MATLAB has many more toolboxes available but these three are commonly available in most engineering departments A second and deeper level of exposure to MATLAB is achieved by concluding each chapter with a separate MATLAB section Taken together these eleven sections provide a selfcontained introduction to the MATLAB environment that allows even novice users to quickly gain MATLAB proficiency and competence These sessions provide detailed instruction on how to use MATLAB to solve problems in linear systems and signals Except for the very last chapter special care has been taken to avoid the use of toolbox functions in the MATLAB sessions Rather readers are shown the process of developing their own code In this way those readers without toolbox access are not at a disadvantage All of this books MATLAB code is available for download at the OUP companion website wwwoupcomuslathi CREDITS AND ACKNOWLEDGMENTS The portraits of Gauss Laplace Heaviside Fourier and Michelson have been reprinted courtesy of the Smithsonian Institution Libraries The likenesses of Cardano and Gibbs have been reprinted courtesy of the Library of Congress The engraving of Napoleon has been reprinted courtesy of BettmannCorbis The many fine cartoons throughout the text are the work of Joseph Coniglio a former student of Dr Lathi Many individuals have helped us in the preparation of this book as well as its earlier editions We are grateful to each and every one for helpful suggestions and comments Book writing is an obsessively timeconsuming activity which causes much hardship for an authors family We both are grateful to our families for their enormous but invisible sacrifices B P Lathi R A Green 00LathiPrelims 2017928 943 page xviii 18 LathiBackground 2017925 1553 page 1 1 C H A P T E R BACKGROUND B The topics discussed in this chapter are not entirely new to students taking this course You have already studied many of these topics in earlier courses or are expected to know them from your previous training Even so this background material deserves a review because it is so pervasive in the area of signals and systems Investing a little time in such a review will pay big dividends later Furthermore this material is useful not only for this course but also for several courses that follow It will also be helpful later as reference material in your professional career B1 COMPLEX NUMBERS Complex numbers are an extension of ordinary numbers and are an integral part of the modern number system Complex numbers particularly imaginary numbers sometimes seem mysterious and unreal This feeling of unreality derives from their unfamiliarity and novelty rather than their supposed nonexistence Mathematicians blundered in calling these numbers imaginary for the term immediately prejudices perception Had these numbers been called by some other name they would have become demystified long ago just as irrational numbers or negative numbers were Many futile attempts have been made to ascribe some physical meaning to imaginary numbers However this effort is needless In mathematics we assign symbols and operations any meaning we wish as long as internal consistency is maintained The history of mathematics is full of entities that were unfamiliar and held in abhorrence until familiarity made them acceptable This fact will become clear from the following historical note B11 A Historical Note Among early people the number system consisted only of natural numbers positive integers needed to express the number of children cattle and quivers of arrows These people had no need for fractions Whoever heard of two and onehalf children or three and onefourth cows However with the advent of agriculture people needed to measure continuously varying quantities such as the length of a field and the weight of a quantity of butter The number system therefore was extended to include fractions The ancient Egyptians and Babylonians knew how 1 LathiBackground 2017925 1553 page 2 2 2 CHAPTER B BACKGROUND to handle fractions but Pythagoras discovered that some numbers like the diagonal of a unit square could not be expressed as a whole number or a fraction Pythagoras a number mystic who regarded numbers as the essence and principle of all things in the universe was so appalled at his discovery that he swore his followers to secrecy and imposed a death penalty for divulging this secret 1 These numbers however were included in the number system by the time of Descartes and they are now known as irrational numbers Until recently negative numbers were not a part of the number system The concept of negative numbers must have appeared absurd to early man However the medieval Hindus had a clear understanding of the significance of positive and negative numbers 2 3 They were also the first to recognize the existence of absolute negative quantities 4 The works of Bhaskar 11141185 on arithmetic Lilavati and algebra Bijaganit not only use the decimal system but also give rules for dealing with negative quantities Bhaskar recognized that positive numbers have two square roots 5 Much later in Europe the men who developed the banking system that arose in Florence and Venice during the late Renaissance fifteenth century are credited with introducing a crude form of negative numbers The seemingly absurd subtraction of 7 from 5 seemed reasonable when bankers began to allow their clients to draw seven gold ducats while their deposit stood at five All that was necessary for this purpose was to write the difference 2 on the debit side of a ledger 6 Thus the number system was once again broadened generalized to include negative numbers The acceptance of negative numbers made it possible to solve equations such as x5 0 which had no solution before Yet for equations such as x2 1 0 leading to x2 1 the solution could not be found in the real number system It was therefore necessary to define a completely new kind of number with its square equal to 1 During the time of Descartes and Newton imaginary or complex numbers came to be accepted as part of the number system but they were still regarded as algebraic fiction The Swiss mathematician Leonhard Euler introduced the notation i for imaginary around 1777 to represent 1 Electrical engineers use the notation j instead of i to avoid confusion with the notation i often used for electrical current Thus j2 1 and 1 j This notation allows us to determine the square root of any negative number For example 4 4 1 2j When imaginary numbers are included in the number system the resulting numbers are called complex numbers ORIGINS OF COMPLEX NUMBERS Ironically and contrary to popular belief it was not the solution of a quadratic equation such as x2 1 0 but a cubic equation with real roots that made imaginary numbers plausible and acceptable to early mathematicians They could dismiss 1 as pure nonsense when it appeared as a solution to x2 1 0 because this equation has no real solution But in 1545 Gerolamo Cardano of Milan published Ars Magna The Great Art the most important algebraic work of the Renaissance In this book he gave a method of solving a general cubic equation in which a root of a negative number appeared in an intermediate step According to his method the solution to a thirdorder equation x³ ax b 0 is given by x b2 b²4 a³2713 b2 b²4 a³2713 For example to find a solution of x³ 6x 20 0 we substitute a 6 b 20 in the foregoing equation to obtain x 10 108 10 108 20392 0392 2 2 We can readily verify that 2 is indeed a solution of x³ 6x 20 0 But when Cardano tried to solve the equation x³ 15x 4 0 by this formula his solution was x 2 121 2 121 Therefore Cardanos formula gives x 2 j 2 j 4 We can readily verify that x 4 is indeed a solution of x³ 15x 4 0 Cardano tried to explain halfheartedly the presence of 121 but ultimately dismissed the whole enterprise as being as subtle as it is useless A generation later however Raphael Bombelli 15251573 after examining Cardanos results proposed acceptance of imaginary numbers as a necessary vehicle that would transport the mathematician from the real cubic equation to its real solution In other words although we begin and end with real numbers we seem compelled to move into an unfamiliar world of imaginaries to complete our journey To mathematicians of the day this proposal seemed incredibly strange 7 Yet they could not dismiss the idea of imaginary numbers so easily because this concept yielded the real solution of an equation It took two more centuries for the full importance of complex numbers to become evident in the works of Euler Gauss and Cauchy Still Bombelli deserves credit for recognizing that such numbers have a role to play in algebra 7 LathiBackground 2017925 1553 page 4 4 4 CHAPTER B BACKGROUND In 1799 the German mathematician Karl Friedrich Gauss at the ripe age of 22 proved the fundamental theorem of algebra namely that every algebraic equation in one unknown has a root in the form of a complex number He showed that every equation of the nth order has exactly n solutions roots no more and no less Gauss was also one of the first to give a coherent account of complex numbers and to interpret them as points in a complex plane It is he who introduced the term complex numbers and paved the way for their general and systematic use The number system was once again broadened or generalized to include imaginary numbers Ordinary or real numbers became a special case of generalized or complex numbers The utility of complex numbers can be understood readily by an analogy with two neighboring countries X and Y as illustrated in Fig B1 If we want to travel from City a to City b both in Gerolamo Cardano Karl Friedrich Gauss Country X Country Y a b A l t e r n a t e r o u t e Direct route Figure B1 Use of complex numbers can reduce the work B12 Algebra of Complex Numbers A complex number a b or a jb can be represented graphically by a point whose Cartesian coordinates are a b in a complex plane Fig B2 Let us denote this complex number by z so that z a jb B1 This representation is the Cartesian or rectangular form of complex number z The numbers a and b the abscissa and the ordinate of z are the real part and the imaginary part respectively of z They are also expressed as Re z a and Im z b Note that in this plane all real numbers lie on the horizontal axis and all imaginary numbers lie on the vertical axis Complex numbers may also be expressed in terms of polar coordinates If r θ are the polar coordinates of a point z a jb see Fig 2 then a r cos θ and b r sin θ Consequently z a jb r cos θ j r sin θ rcos θ jsin θ B2 Eulers formula states that eiθ cos θ jsin θ B3 To prove Eulers formula we use a Maclaurin series to expand eiθ cos θ and sin θ eiθ 1 jθ jθ² 2 jθ³ 3 jθ⁴ 4 jθ⁵ 5 jθ⁶ 6 1 jθ θ² 2 θ⁴ 4 θ⁶ 6 cos θ 1 θ² 2 θ⁴ 4 θ⁶ 6 sin θ θ θ³ 3 θ⁵ 5 Clearly it follows that eiθ cos θ jsin θ Using Eq B3 in Eq B2 yields z reiθ B4 This representation is the polar form of complex number z Summarizing a complex number can be expressed in rectangular form a jb or polar form reiθ with a rcos θ and b rsin θ θ tan1ba B5 Observe that r is the distance of the point z from the origin For this reason r is also called the magnitude or absolute value of z and is denoted by z Similarly θ is called the angle of z and is denoted by Lz Therefore we can also write polar form of Eq B4 as z zeiLz where z r and Lz θ Using polar form we see that the reciprocal of a complex number is given by 1 z 1 reiθ 1 r eiθ 1 z eiLz CONJUGATE OF A COMPLEX NUMBER We define z a jb reiθ zeiLz B6 The graphical representations of z a jb and its conjugate z are depicted in Fig B2 Observe that z is a mirror image of z about the horizontal axis To find the conjugate of any number we need only replace j with j in that number which is the same as changing the sign of its angle The sum of a complex number and its conjugate is a real number equal to twice the real part of the number z z a jb a jb 2a 2Re z Thus we see that the real part of complex number z can be computed as Re z z z 2 B7 Similarly the imaginary part of complex number z can be computed as Imz z z 2j The number 1 on the other hand is also at a unit distance from the origin but has an angle 0 more generally 0 plus any integer multiple of 2π For this reason it is advisable to draw the point in the complex plane and determine the quadrant in which the point lies This issue will be clarified by the following examples LathiBackground 2017925 1553 page 10 10 10 CHAPTER B BACKGROUND We can easily verify these results using the MATLAB abs and angle commands To obtain units of degrees we must multiply the radian result of the angle command by 180 π Furthermore the angle command correctly computes angles for all four quadrants of the complex plane To provide an example let us use MATLAB to verify that 2 j1 5ej1534 22361ej1534 abs21j ans 22361 angle21j180pi ans 1534349 One can also use the cart2pol command to convert Cartesian to polar coordinates Readers particularly those who are unfamiliar with MATLAB will benefit by reading the overview in Sec B7 EXAMPLE B2 Polar to Cartesian Form Represent the following numbers in the complex plane and express them in Cartesian form a 2ejπ3 b 4ej3π4 c 2ejπ2 d 3ej3π e 2ej4π and f 2ej4π a 2ejπ3 2cos π3 jsin π3 1 j 3 see Fig B5a b 4ej3π4 4cos 3π4 jsin 3π4 2 2 j2 2 see Fig B5b c 2ejπ2 2cos π2 jsin π2 20 j1 j2 see Fig B5c d 3ej3π 3cos 3π jsin 3π 31 j0 3 see Fig B5d e 2ej4π 2cos 4π jsin 4π 21 j0 2 see Fig B5e f 2ej4π 2cos 4π jsin 4π 21 j0 2 see Fig B5f We can readily verify these results using MATLAB First we use the exp function to represent a number in polar form Next we use the real and imag commands to determine the real and imaginary components of that number To provide an example let us use MATLAB to verify the result of part a 2ejπ3 1 j 3 1 j17321 real2exp1jpi3 ans 10000 imag2exp1jpi3 ans 17321 Since MATLAB defaults to Cartesian form we could have verified the entire result in one step 2exp1jpi3 ans 10000 17321i One can also use the pol2cart command to convert polar to Cartesian coordinates B1 Complex Numbers ARITHMETICAL OPERATIONS POWERS AND ROOTS OF COMPLEX NUMBERS To conveniently perform addition and subtraction complex numbers should be expressed in Cartesian form Thus if z₁ 3 j4 5e531 and z₂ 2 j3 13e563 then z₁ z₂ 3 j4 2 j3 5 j7 Division Cartesian Form z₁ 3 j4 z₂ 2 j3 To eliminate the complex number in the denominator we multiply both the numerator and the denominator of the righthand side by 2 j3 the denominators conjugate This yields z₁z₂ 3 j42 j32 j32 j3 18 j122 32 18 j113 1813 j113 Therefore 2z1 z2 22 j2 4 j3 22 4 j22 43 117 j41 b Xω 2 jω 3 j4ω 4 ω² etan¹ω2 9 16ω² etan¹ω3 4 ω² 9 16ω² etan¹ω2 tan¹ω3 LathiBackground 2017925 1553 page 16 16 16 CHAPTER B BACKGROUND B2 SINUSOIDS Consider the sinusoid xt Ccos2πf0t θ B13 We know that cos ϕ cosϕ 2nπ n 0123 Therefore cos ϕ repeats itself for every change of 2π in the angle ϕ For the sinusoid in Eq B13 the angle 2πf0tθ changes by 2π when t changes by 1f0 Clearly this sinusoid repeats every 1f0 seconds As a result there are f0 repetitions per second This is the frequency of the sinusoid and the repetition interval T0 given by T0 1 f0 B14 is the period For the sinusoid in Eq B13 C is the amplitude f0 is the frequency in hertz and θ is the phase Let us consider two special cases of this sinusoid when θ 0 and θ π2 as follows xt Ccos 2πf0t θ 0 and xt Ccos2πf0t π2 Csin 2πf0t θ π2 The angle or phase can be expressed in units of degrees or radians Although the radian is the proper unit in this book we shall often use the degree unit because students generally have a better feel for the relative magnitudes of angles expressed in degrees rather than in radians For example we relate better to the angle 24 than to 0419 radian Remember however when in doubt use the radian unit and above all be consistent In other words in a given problem or an expression do not mix the two units It is convenient to use the variable ω0 radian frequency to express 2πf0 ω0 2πf0 B15 With this notation the sinusoid in Eq B13 can be expressed as xt Ccosω0t θ in which the period T0 and frequency ω0 are given by see Eqs B14 and B15 T0 1 ω02π 2π ω0 and ω0 2π T0 Although we shall often refer to ω0 as the frequency of the signal cosω0tθ it should be clearly understood that ω0 is the radian frequency the hertzian frequency of this sinusoid is f0 ω02π The signals Ccos ω0t and Csin ω0t are illustrated in Figs B6a and B6b respectively A general sinusoid Ccosω0tθ can be readily sketched by shifting the signal Ccos ω0t in Fig B6a by the appropriate amount Consider for example xt Ccosω0t 60 This signal can be obtained by shifting delaying the signal C cosω0t Fig B6a to the right by a phase angle of 60 We know that a sinusoid undergoes a 360 change of phase or angle in one cycle A quartercycle segment corresponds to a 90 change of angle Alternatively if we advance C sinω0t by a quartercycle we obtain C cosω0t Therefore C sinω0t π2 C cosω0t These observations mean that sinω0t lags cosω0t by 90π2 radians and that cosω0t leads sinω0t by 90 a In this case a 1 and b 3 Using Eq B17 yields C 12 32 2 and θ tan131 60 Therefore xt 2cosω0t 60 We can verify this result by drawing phasors corresponding to the two sinusoids The sinusoid cosω0t is represented by a phasor of unit length at a zero angle with the horizontal The phasor sinω0t is represented by a unit phasor at an angle of 90 with the horizontal Therefore 3sinω0t is represented by a phasor of length 3 at 90 with the horizontal as depicted in Fig B8a Observe that tan134 tan134 531 Therefore xt 5cosω0t 1269 This result is readily verified in the phasor diagram in Fig B8b Alternately a jb 3 j4 5ej1269 a fact readily confirmed using MATLAB C abs34j C 5 theta angle34j180pi theta 1268699 Hence C 5 and θ 1268699 eat ut 1e approx 037 1e2 approx 0135 LathiBackground 2017925 1553 page 22 22 22 CHAPTER B BACKGROUND manner we see that xt 1e3 at t 15 and so on A knowledge of the values of xt at t 0 05 1 and 15 allows us to sketch the desired signal as shown in Fig B10b For a monotonically growing exponential eat the waveform increases by a factor e over each interval of 1a seconds B32 The Exponentially Varying Sinusoid We now discuss sketching an exponentially varying sinusoid xt Aeat cosω0t θ Let us consider a specific example xt 4e2t cos6t 60 We shall sketch 4e2t and cos6t 60 separately and then multiply them a Sketching 4e2t This monotonically decaying exponential has a time constant of 05 second and an initial value of 4 at t 0 Therefore its values at t 05 1 15 and 2 are 4e 4e2 4e3 and 4e4 or about 147 054 02 and 007 respectively Using these values as a guide we sketch 4e2t as illustrated in Fig B11a b Sketching cos6t 60 The procedure for sketching cos6t 60 is discussed in Sec B2 Fig B6c Here the period of the sinusoid is T0 2π6 1 and there is a phase delay of 60 or twothirds of a quartercycle which is equivalent to a delay of about 603601 16 seconds see Fig B11b c Sketching 4e2t cos6t 60 We now multiply the waveforms in steps a and b This multiplication amounts to forcing the sinusoid 4 cos6t 60 to decrease exponentially with a time constant of 05 The initial amplitude at t 0 is 4 decreasing to 4e 147 at t 05 to 147e054 at t 1 and so on This is depicted in Fig B11c Note that when cos6t 60 has a value of unity peak amplitude 4e2t cos6t 60 4e2t Therefore 4e2t cos6t60 touches 4e2t at the instants at which the sinusoid cos6t 60 is at its positive peaks Clearly 4e2t is an envelope for positive amplitudes of 4e2t cos6t 60 Similar argument shows that 4e2t cos6t 60 touches 4e2t at its negative peaks Therefore 4e2t is an envelope for negative amplitudes of 4e2t cos6t 60 Thus to sketch 4e2t cos6t 60 we first draw the envelopes 4e2t and 4e2t the mirror image of 4e2t about the horizontal axis and then sketch the sinusoid cos6t 60 with these envelopes acting as constraints on the sinusoids amplitude see Fig B11c In general Keat cosω0t θ can be sketched in this manner with Keat and Keat constraining the amplitude of cosω0t θ If we wish to refine the sketch further we could consider intervals of half the time constant over which the signal decays by a factor 1e Thus at t 025 xt 1e and at t 075 xt 1ee and so on Cramers rule offers a very convenient way to solve simultaneous linear equations in n unknowns x1 x2 ldots xn A beginvmatrix 2 1 1 1 3 1 1 1 1 endvmatrix 4 x₂ frac1A beginvmatrix 2 3 1 1 7 1 1 1 1 endvmatrix frac44 1 Fx frac2x3 9x2 11x 2x2 4x 3 2x 1 fracx 1x2 4x 3 k₁ 1 k₂ 2 k₃ 2 k₄ 3 EXAMPLE B9 Heaviside CoverUp Method Expand the following rational function Fx into partial fractions Fx 2x² 9x 11 x 1x 2x 3 k1 x 1 k2 x 2 k3 x 3 To determine k1 we let x 1 in x 1Fx Note that x 1Fx is obtained from Fx by omitting the term x 1 from its denominator Therefore to compute k1 corresponding to the factor x 1 we cover up the term x 1 in the denominator of Fx and then substitute x 1 in the remaining expression Mentally conceal the term x 1 in Fx with a finger and then let x 1 in the remaining expression The steps in covering up the function Fx are as follows Step 1 Cover up conceal the factor x 1 from Fx 2x² 9x 11 x 1x 2x 3 Step 2 Substitute x 1 in the remaining expression to obtain k1 k1 21² 91 111 121 3 2 9 11 0 2 2 186 3 Similarly to compute k2 we cover up the factor x 2 in Fx and let x 2 in the remaining function as follows k2 2x² 9x 11 x 1x 2x 3 x2 8 18 11 2 122 3 15 15 1 and k3 2x² 9x 11 x 1x 2x 3 x3 18 27 11 3 13 2 20 10 2 Therefore Fx 2x² 9x 11 x 1x 2x 3 3 x 1 1 x 2 2 x 3 COMPLEX FACTORS OF Qx The procedure just given works regardless of whether the factors of Qx are real or complex Consider for example Fx 4x² 2x 18 x 1x² 4x 13 k1 x 1 k2 x² 2 j3x² 2 j3 where k1 4x² 2x 18 x 1x² 4x 13 x2j3 2 Similarly k2 4x² 2x 18 x 1x² 2 j3x² 2 j3 x2j3 1 j2 5ej3π4 k3 4x² 2x 18 x 1x² 2 j3x² 2 j3 x2j3 1 j2 5ej3π4 Therefore Fx 2x 1 5ej3π4 x² 2 j3 5ej3π4 x² 2 j3 LathiBackground 2017925 1553 page 30 30 30 CHAPTER B BACKGROUND Equating terms of similar powers yields c1 2 c2 8 and 4x2 2x 18 x 1x2 4x 13 2 x 1 2x 8 x2 4x 13 SHORTCUTS The values of c1 and c2 in Eq B26 can also be determined by using shortcuts After computing k1 2 by the Heaviside method as before we let x 0 on both sides of Eq B26 to eliminate c1 This gives us 18 13 2 c2 13 c2 8 To determine c1 we multiply both sides of Eq B26 by x and then let x Remember that when x only the terms of the highest power are significant Therefore 4 2 c1 c1 2 In the procedure discussed here we let x 0 to determine c2 and then multiply both sides by x and let x to determine c1 However nothing is sacred about these values x 0 or x We use them because they reduce the number of computations involved We could just as well use other convenient values for x such as x 1 Consider the case Fx 2x2 4x 5 xx2 2x 5 k x c1x c2 x2 2x 5 We find k 1 by the Heaviside method in the usual manner As a result 2x2 4x 5 xx2 2x 5 1 x c1x c2 x2 2x 5 B27 If we try letting x 0 to determine c1 and c2 we obtain on both sides So let us choose x 1 This yields 11 8 1 c1 c2 8 or c1 c2 3 We can now choose some other value for x such as x 2 to obtain one more relationship to use in determining c1 and c2 In this case however a simple method is to multiply both sides of Eq B27 by x and then let x This yields 2 1 c1 c1 1 Since c1 c2 3 we see that c2 2 and therefore Fx 1 x x 2 x2 2x 5 B5 Repeated Factors of Qx If a function Fx has a repeated factor in its denominator it has the form Fx Px xλrxα1xα2 xαj Its partial fraction expansion is given by Fx a0 xλ a1 xλr ar1 xλ k1 xα1 k2 xα2 kj xαj B28 The coefficients k1 k2 kj corresponding to the unrepeated factors in this equation are determined by the Heaviside method as before Eq B24 To find the coefficients a0 a1 a2 ar1 we multiply both sides of Eq B28 by xλr This gives us xλrFx a0 a1xλ a2xλ² ar1xλr1 k1xλr xα1 k2xλr xα2 kjxλr xαj B29 If we let x λ on both sides of Eq B29 we obtain λλrFλ xλ a0 Therefore a0 is obtained by concealing the factor xλr in Fx and letting xλ in the remaining expression the Heaviside coverup method If we take the derivative with respect to x of both sides of Eq B29 the righthand side is a1 terms containing a factor xλ in their numerators Letting x λ on both sides of this equation we obtain ddx xλrFx xλ a1 Thus a1 is obtained by concealing the factor xλr in Fx taking the derivative of the remaining expression and then letting x λ Continuing in this manner we find aj 1j dj dxj xλrFx xλ B30 Observe that xλrFx is obtained from Fx by omitting the factor xλr from its denominator Therefore the coefficient aj is obtained by concealing the factor xλr in Fx taking the jth derivative of the remaining expression and then letting x λ while dividing by j Expand Fx into partial fractions if Fx 3x² 9x 20 x² x 6 3x² 9x 20 x 2x 3 Here m n 2 with b₂ 3 Therefore Fx 3x² 9x 20 x 2x 3 3 k₁ x 2 k₂ x 3 4x³ 16x² 23x 13 x 1³x 2 2 x 1³ a₁ x 1² a₂ x 1 x 2 There is only one unknown a₁ which can be readily found by setting x equal to any convenient value say x 0 This yields 13 2 2 a₁ 3 1 2 a₁ 1 in which k₁ 3x² 9x 20 x 2x 3 12 18 20 2 3 10 5 2 and k₂ 3x² 9x 20 x 2x 3 27 27 20 3 2 20 5 4 Therefore Fx 3x² 9x 20 x 2x 3 3 2 x 2 4 x 3 LathiBackground 2017925 1553 page 36 36 36 CHAPTER B BACKGROUND B6 VECTORS AND MATRICES An entity specified by n numbers in a certain order ordered ntuple is an ndimensional vector Thus an ordered ntuple x1 x2 xn represents an ndimensional vector x A vector may be represented as a row row vector x x1 x2 xn or as a column column vector x x1 x2 xn Simultaneous linear equations can be viewed as the transformation of one vector into another Consider for example the m simultaneous linear equations y1 a11x1 a12x2 a1nxn y2 a21x1 a22x2 a2nxn ym am1x1 am2x2 amnxn B31 If we define two column vectors x and y as x x1 x2 xn and y y1 y2 ym then Eq B31 may be viewed as the relationship or the function that transforms vector x into vector y Such a transformation is called a linear transformation of vectors To perform a linear transformation we need to define the array of coefficients aij appearing in Eq B31 This array is called a matrix and is denoted by A for convenience A a11 a12 a1n a21 a22 a2n am1 am2 amn A matrix with m rows and n columns is called a matrix of order mn or an m n matrix For the special case of m n the matrix is called a square matrix of order n It should be stressed at this point that a matrix is not a number such as a determinant but an array of numbers arranged in a particular order It is convenient to abbreviate the representation of matrix A with the form aijmn implying a matrix of order m n with aij as its ijth element In practice when the order m n is understood or need not be specified the notation can be A square matrix whose elements are zero everywhere except on the main diagonal is a diagonal matrix An example of a diagonal matrix is I 2 0 0 0 1 0 0 0 0 5 A diagonal matrix with unity for all its diagonal elements is called an identity matrix or a unit matrix denoted by I This is a square matrix LathiBackground 2017925 1553 page 38 38 38 CHAPTER B BACKGROUND Using the abbreviated notation if A aijmn then AT ajinm Intuitively further notice that ATT A B62 Matrix Algebra We shall now define matrix operations such as addition subtraction multiplication and division of matrices The definitions should be formulated so that they are useful in the manipulation of matrices ADDITION OF MATRICES For two matrices A and B both of the same order m n A a11 a12 a1n a21 a22 a2n am1 am2 amn and B b11 b12 b1n b21 b22 b2n bm1 bm2 bmn we define the sum A B as A B a11 b11 a12 b12 a1n b1n a21 b21 a22 b22 a2n b2n am1 bm1 am2 bm2 amn bmn or A B aij bijmn Note that two matrices can be added only if they are of the same order MULTIPLICATION OF A MATRIX BY A SCALAR We multiply a matrix A by a scalar c as follows cA c a11 a12 a1n a21 a22 a2n am1 am2 amn ca11 ca12 ca1n ca21 ca22 ca2n cam1 cam2 camn Ac Thus we also observe that the scalar c and the matrix A commute cA Ac MATRIX MULTIPLICATION We define the product AB C in which cij the element of C in the ith row and jth column is found by adding the products of the elements of A in the ith row multiplied by the corresponding elements of B in the jth column cij ai1bj1 ai2bj2 cdots ainbnj sumk1n aikbkj B33 This result is expressed as follows a11 a12 cdots a1n b1j b2j cdots bmj cij LathiBackground 2017925 1553 page 40 40 40 CHAPTER B BACKGROUND In the matrix product AB matrix A is said to be postmultiplied by B or matrix B is said to be premultiplied by A We may also verify the following relationships A BC AC BC CA B CA CB We can verify that any matrix A premultiplied or postmultiplied by the identity matrix I remains unchanged AI IA A Of course we must make sure that the order of I is such that the matrices are conformable for the corresponding product We give here without proof another important property of matrices AB AB where A and B represent determinants of matrices A and B MULTIPLICATION OF A MATRIX BY A VECTOR Consider Eq B32 which represents Eq B31 The righthand side of Eq B32 is a product of the mn matrix A and a vector x If for the time being we treat the vector x as if it were an n1 matrix then the product Ax according to the matrix multiplication rule yields the righthand side of Eq B31 Thus we may multiply a matrix by a vector by treating the vector as if it were an n 1 matrix Note that the constraint of conformability still applies Thus in this case xA is not defined and is meaningless MATRIX INVERSION To define the inverse of a matrix let us consider the set of equations represented by Eq B32 when m n y1 y2 yn a11 a12 a1n a21 a22 a2n an1 an2 ann x1 x2 xn B34 We can solve this set of equations for x1 x2 xn in terms of y1 y2 yn by using Cramers rule see Eq B21 This yields x1 x2 xn D11 A D21 A Dn1 A D12 A D22 A Dn2 A D1n A D2n A Dnn A y1 y2 yn B35 in which A is the determinant of the matrix A and Dij is the cofactor of element aij in the matrix A The cofactor of element aij is given by 1ij times the determinant of the LathiBackground 2017925 1553 page 41 41 B6 Vectors and Matrices 41 n 1 n 1 matrix that is obtained when the ith row and the jth column in matrix A are deleted We can express Eq B34 in compact matrix form as y Ax B36 We now define A1 the inverse of a square matrix A with the property A1A I unit matrix Then premultiplying both sides of Eq B36 by A1 we obtain A1y A1Ax Ix x or x A1y B37 A comparison of Eq B37 with Eq B35 shows that A1 1 A D11 D21 Dn1 D12 D22 Dn2 D1n D2n Dnn One of the conditions necessary for a unique solution of Eq B34 is that the number of equations must equal the number of unknowns This implies that the matrix A must be a square matrix In addition we observe from the solution as given in Eq B35 that if the solution is to exist A 0 Therefore the inverse exists only for a square matrix and only under the condition that the determinant of the matrix be nonzero A matrix whose determinant is nonzero is a nonsingular matrix Thus an inverse exists only for a nonsingular square matrix Since A1A I AA1 we further note that the matrices A and A1 commute The operation of matrix division can be accomplished through matrix inversion EXAMPLE B12 Computing the Inverse of a Matrix Let us find A1 if A 2 1 1 1 2 3 3 2 1 These two conditions imply that the number of equations is equal to the number of unknowns and that all the equations are independent To prove AA1 I notice first that we define A1A I Thus IA AI AA1A AA1A Subtracting AA1A we see that IA AA1A 0 or I AA1A 0 This requires AA1 I LathiBackground 2017925 1553 page 42 42 42 CHAPTER B BACKGROUND Here D11 4 D12 8 D13 4 D21 1 D22 1 D23 1 D31 1 D32 5 D33 3 and A 4 Therefore A1 1 4 4 1 1 8 1 5 4 1 3 B7 MATLAB ELEMENTARY OPERATIONS B71 MATLAB Overview Although MATLABl a registered trademark of The MathWorks Inc is easy to use it can be intimidating to new users Over the years MATLAB has evolved into a sophisticated computational package with thousands of functions and thousands of pages of documentation This section provides a brief introduction to the software environment When MATLAB is first launched its command window appears When MATLAB is ready to accept an instruction or input a command prompt is displayed in the command window Nearly all MATLAB activity is initiated at the command prompt Entering instructions at the command prompt generally results in the creation of an object or objects Many classes of objects are possible including functions and strings but usually objects are just data Objects are placed in what is called the MATLAB workspace If not visible the workspace can be viewed in a separate window by typing workspace at the command prompt The workspace provides important information about each object including the objects name size and class Another way to view the workspace is the whos command When whos is typed at the command prompt a summary of the workspace is printed in the command window The who command is a short version of whos that reports only the names of workspace objects Several functions exist to remove unnecessary data and help free system resources To remove specific variables from the workspace the clear command is typed followed by the names of the variables to be removed Just typing clear removes all objects from the workspace Additionally the clc command clears the command window and the clf command clears the current figure window Often important data and objects created in one session need to be saved for future use The save command followed by the desired filename saves the entire workspace to a file which has the mat extension It is also possible to selectively save objects by typing save followed by the filename and then the names of the objects to be saved The load command followed by the filename is used to load the data and objects contained in a MATLAB data file mat file Although MATLAB does not automatically save workspace data from one session to the next lines entered at the command prompt are recorded in the command history Previous command lines can be viewed copied and executed directly from the command history window From the LathiBackground 2017925 1553 page 43 43 B7 MATLAB Elementary Operations 43 command window pressing the up or down arrow key scrolls through previous commands and redisplays them at the command prompt Typing the first few characters and then pressing the arrow keys scrolls through the previous commands that start with the same characters The arrow keys allow command sequences to be repeated without retyping Perhaps the most important and useful command for new users is help To learn more about a function simply type help followed by the function name Helpful text is then displayed in the command window The obvious shortcoming of help is that the function name must first be known This is especially limiting for MATLAB beginners Fortunately help screens often conclude by referencing related or similar functions These references are an excellent way to learn new MATLAB commands Typing help help for example displays detailed information on the help command itself and also provides reference to relevant functions such as the lookfor command The lookfor command helps locate MATLAB functions based on a keyword search Simply type lookfor followed by a single keyword and MATLAB searches for functions that contain that keyword MATLAB also has comprehensive HTMLbased help The HTML help is accessed by using MATLABs integrated help browser which also functions as a standard web browser The HTML help facility includes a function and topic index as well as full textsearching capabilities Since HTML documents can contain graphics and special characters HTML help can provide more information than the commandline help After a little practice it is easy to find information in MATLAB When MATLAB graphics are created the print command can save figures in a common file format such as postscript encapsulated postscript JPEG or TIFF The format of displayed data such as the number of digits displayed is selected by using the format command MATLAB help provides the necessary details for both these functions When a MATLAB session is complete the exit command terminates MATLAB B72 Calculator Operations MATLAB can function as a simple calculator working as easily with complex numbers as with real numbers Scalar addition subtraction multiplication division and exponentiation are accomplished using the traditional operator symbols and Since MATLAB predefines i j 1 a complex constant is readily created using Cartesian coordinates For example z 34j z 30000 40000i assigns the complex constant 3 j4 to the variable z The real and imaginary components of z are extracted by using the real and imag operators In MATLAB the input to a function is placed parenthetically following the function name zreal realz zimag imagz When a command is terminated with a semicolon the statement is evaluated but the results are not displayed to the screen This feature is useful when one is computing intermediate results and it allows multiple instructions on a single line Although not displayed the results zreal 3 and zimag 4 are calculated and available for additional operations such as computing z There are many ways to compute the modulus or magnitude of a complex quantity Trigonometry confirms that z 3 j4 which corresponds to a 345 triangle has modulus z 13 j4 32 42 5 The MATLAB sqrt command provides one way to compute the required square root zmag sqrtzreal2 zimag2 zmag 5 In MATLAB most commands including sqrt accept inputs in a variety of forms including constants variables functions expressions and combinations thereof The same result is also obtained by computing z zz In this case complex conjugation is performed by using the conj command zmag sqrtzconjz zmag 5 More simply MATLAB computes absolute values directly by using the abs command zmag absz zmag 5 In addition to magnitude polar notation requires phase information The angle command provides the angle of a complex number zrad anglez zrad 22143 MATLAB expects and returns angles in a radian measure Angles expressed in degrees require an appropriate conversion factor zdeg anglez180pi zdeg 1268699 Notice MATLAB predefines the variable pi π It is also possible to obtain the angle of z using a twoargument arctangent function atan2 zrad atan2zimagzreal zrad 22143 Unlike a singleargument arctangent function the twoargument arctangent function ensures that the angle reflects the proper quadrant MATLAB supports a full complement of trigonometric functions standard trigonometric functions cos sin tan reciprocal trigonometric functions sec csc cot inverse trigonometric functions acos asin atan asec acsc acot and hyperbolic variations cosh sinh tanh csch coth acosh asinh atanh asec acsch and acoth Of course MATLAB comfortably supports complex arguments for any trigonometric function As with the angle command MATLAB trigonometric functions utilize units of radians The results can contradict what is often taught in introductory mathematics courses For example a common claim is that cosx 1 While this is true for real x it is not necessarily true for complex x This is readily verified by example using MATLAB and the cos function cos1j ans 15431 LathiBackground 2017925 1553 page 45 45 B7 MATLAB Elementary Operations 45 Problem B119 investigates these ideas further Similarly the claim that it is impossible to take the logarithm of a negative number is false For example the principal value of ln1 is jπ a fact easily verified by means of Eulers equation In MATLAB base10 and basee logarithms are computed by using the log10 and log commands respectively log1 ans 0 31416i B73 Vector Operations The power of MATLAB becomes apparent when vector arguments replace scalar arguments Rather than computing one value at a time a single expression computes many values Typically vectors are classified as row vectors or column vectors For now we consider the creation of row vectors with evenly spaced real elements To create such a vector the notation abc is used where a is the initial value b designates the step size and c is the termination value For example 0211 creates the length6 vector of evenvalued integers ranging from 0 to 10 k 0211 k 0 2 4 6 8 10 In this case the termination value does not appear as an element of the vector Negative and noninteger step sizes are also permissible k 111030 k 110000 76667 43333 10000 If a step size is not specified a value of 1 is assumed k 011 k 0 1 2 3 4 5 6 7 8 9 10 11 Vector notation provides the basis for solving a wide variety of problems For example consider finding the three cube roots of minus one w3 1 ejπ2πk for integer k Taking the cube root of each side yields w ejπ32πk3 To find the three unique solutions use any three consecutive integer values of k and MATLABs exp function k 02 w exp1jpi3 2pik3 w 05000 08660i 10000 00000i 05000 08660i The solutions particularly w 1 are easy to verify Finding the 100 unique roots of w100 1 is just as simple k 099 w exp1jpi100 2pik100 A semicolon concludes the final instruction to suppress the inconvenient display of all 100 solutions To view a particular solution the user must use an index to specify desired elements LathiBackground 2017925 1553 page 46 46 46 CHAPTER B BACKGROUND MATLAB indices are integers that increase from a starting value of 1 For example the fifth element of w is extracted using an index of 5 w5 ans 09603 02790i Notice that this solution corresponds to k 4 The independent variable of a function in this case k rarely serves as the index Since k is also a vector it can likewise be indexed In this way we can verify that the fifth value of k is indeed 4 k5 ans 4 It is also possible to use a vector index to access multiple values For example index vector 98100 identifies the last three solutions corresponding to k 979899 w98100 ans 09877 01564i 09956 00941i 09995 00314i Vector representations provide the foundation to rapidly create and explore various signals Consider the simple 10 Hz sinusoid described by ft sin2π10t π6 Two cycles of this sinusoid are included in the interval 0 t 02 A vector t is used to uniformly represent 500 points over this interval t 0025000202500 Next the function ft is evaluated at these points f sin2pi10tpi6 The value of ft at t 0 is the first element of the vector and is thus obtained by using an index of 1 f1 ans 05000 Unfortunately MATLABs indexing syntax conflicts with standard equation notation That is the MATLAB indexing command f1 is not the same as the standard notation f1 ftt1 Care must be taken to avoid confusion remember that the index parameter rarely reflects the independent variable of a function B74 Simple Plotting MATLABs plot command provides a convenient way to visualize data such as graphing ft against the independent variable t plottf Some other programming languages such as C begin indexing at 0 Careful attention is warranted MATLAB anonymous functions considered in Sec 111 are an important and useful exception LathiBackground 2017925 1553 page 47 47 B7 MATLAB Elementary Operations 47 0 005 01 015 02 t 1 0 1 ft Figure B12 ft sin2π10t π6 Axis labels are added using the xlabel and ylabel commands where the desired string must be enclosed by single quotation marks The result is shown in Fig B12 xlabelt ylabelft The title command is used to add a title above the current axis By default MATLAB connects data points with solid lines Plotting discrete points such as the 100 unique roots of w100 1 is accommodated by supplying the plot command with an additional string argument For example the string o tells MATLAB to mark each data point with a circle rather than connecting points with lines A full description of the supported plot options is available from MATLABs help facilities plotrealwimagwo xlabelRew ylabelImw axis equal The axis equal command ensures that the scale used for the horizontal axis is equal to the scale used for the vertical axis Without axis equal the plot would appear elliptical rather than circular Figure B13 illustrates that the 100 unique roots of w100 1 lie equally spaced on the unit circle a fact not easily discerned from the raw numerical data MATLAB also includes many specialized plotting functions For example MATLAB commands semilogx semilogy and loglog operate like the plot command but use base10 logarithmic scales for the horizontal axis vertical axis and the horizontal and vertical axes 1 05 0 05 1 Rew 05 0 05 Imw Figure B13 Unique roots of w100 1 LathiBackground 2017925 1553 page 48 48 48 CHAPTER B BACKGROUND respectively Monochrome and color images can be displayed by using the image command and contour plots are easily created with the contour command Furthermore a variety of threedimensional plotting routines are available such as plot3 contour3 mesh and surf Information about these instructions including examples and related functions is available from MATLAB help B75 ElementbyElement Operations Suppose a new function ht is desired that forces an exponential envelope on the sinusoid ft ht ftgt where gt e10t First row vector gt is created g exp10t Given MATLABs vector representation of gt and ft computing ht requires some form of vector multiplication There are three standard ways to multiply vectors inner product outer product and elementbyelement product As a matrixoriented language MATLAB defines the standard multiplication operator according to the rules of matrix algebra the multiplicand must be conformable to the multiplier A 1 N row vector times an N 1 column vector results in the scalarvalued inner product An N 1 column vector times a 1 M row vector results in the outer product which is an N M matrix Matrix algebra prohibits multiplication of two row vectors or multiplication of two column vectors Thus the operator is not used to perform elementbyelement multiplication Elementbyelement operations require vectors to have the same dimensions An error occurs if elementbyelement operations are attempted between row and column vectors In such cases one vector must first be transposed to ensure both vector operands have the same dimensions In MATLAB most elementbyelement operations are preceded by a period For example elementbyelement multiplication division and exponentiation are accomplished using and respectively Vector addition and subtraction are intrinsically elementbyelement operations and require no period Intuitively we know ht should be the same size as both gt and ft Thus ht is computed using elementbyelement multiplication h fg The plot command accommodates multiple curves and also allows modification of line properties This facilitates sidebyside comparison of different functions such as ht and ft Line characteristics are specified by using options that follow each vector pair and are enclosed in single quotes plottfkthk xlabelt ylabelAmplitude legendftht Here k instructs MATLAB to plot ft using a solid black line while k instructs MATLAB to use a dotted black line to plot ht A legend and axis labels complete the plot as shown in While grossly inefficient elementbyelement multiplication can be accomplished by extracting the main diagonal from the outer product of two Nlength vectors LathiBackground 2017925 1553 page 49 49 B7 MATLAB Elementary Operations 49 0 005 01 015 02 t 1 05 0 05 1 Amplitude ft ht Figure B14 Graphical comparison of ft and ht Fig B14 It is also possible although more cumbersome to use pull down menus to modify line properties and to add labels and legends directly in the figure window B76 Matrix Operations Many applications require more than row vectors with evenly spaced elements row vectors column vectors and matrices with arbitrary elements are typically needed MATLAB provides several functions to generate common useful matrices Given integers m n and vector x the function eyem creates the mm identity matrix the function onesmn creates the m n matrix of all ones the function zerosmn creates the m n matrix of all zeros and the function diagx uses vector x to create a diagonal matrix The creation of general matrices and vectors however requires each individual element to be specified Vectors and matrices can be input spreadsheet style by using MATLABs array editor This graphical approach is rather cumbersome and is not often used A more direct method is preferable Consider a simple row vector r r 1 0 0 The MATLAB notation abc cannot create this row vector Rather square brackets are used to create r r 1 0 0 r 1 0 0 Square brackets enclose elements of the vector and spaces or commas are used to separate row elements Next consider the 3 2 matrix A A 2 3 4 5 0 6 LathiBackground 2017925 1553 page 50 50 50 CHAPTER B BACKGROUND Matrix A can be viewed as a threehigh stack of twoelement row vectors With a semicolon to separate rows square brackets are used to create the matrix A 2 34 50 6 A 2 3 4 5 0 6 Each row vector needs to have the same length to create a sensible matrix In addition to enclosing string arguments a single quote performs the complex conjugate transpose operation In this way row vectors become column vectors and vice versa For example a column vector c is easily created by transposing row vector r c r c 1 0 0 Since vector r is real the complexconjugate transpose is just the transpose Had r been complex the simple transpose could have been accomplished by either r or conjr More formally square brackets are referred to as a concatenation operator A concatenation combines or connects smaller pieces into a larger whole Concatenations can involve simple numbers such as the sixelement concatenation used to create the 32 matrix A It is also possible to concatenate larger objects such as vectors and matrices For example vector c and matrix A can be concatenated to form a 3 3 matrix B B c A B 1 2 3 0 4 5 0 0 6 Errors will occur if the component dimensions do not sensibly match a 22 matrix would not be concatenated with a 3 3 matrix for example Elements of a matrix are indexed much like vectors except two indices are typically used to specify row and column Element 1 2 of matrix B for example is 2 B12 ans 2 Indices can likewise be vectors For example vector indices allow us to extract the elements common to the first two rows and last two columns of matrix B B1223 ans 2 3 4 5 Matrix elements can also be accessed by means of a single index which enumerates along columns Formally the element from row m and column n of an M N matrix may be obtained with a single index n 1M m For example element 1 2 of matrix B is accessed by using the index 2 13 1 4 That is B4 yields 2 LathiBackground 2017925 1553 page 51 51 B7 MATLAB Elementary Operations 51 One indexing technique is particularly useful and deserves special attention A colon can be used to specify all elements along a specified dimension For example B2 selects all column elements along the second row of B B2 ans 0 4 5 Now that we understand basic vector and matrix creation we turn our attention to using these tools on real problems Consider solving a set of three linear simultaneous equations in three unknowns x1 2x2 3x3 1 3x1 x2 5x3 π 3x1 7x2 x3 e This system of equations is represented in matrix form according to Ax y where A 1 2 3 3 1 5 3 7 1 x x1 x2 x3 and y 1 π e Although Cramers rule can be used to solve Ax y it is more convenient to solve by multiplying both sides by the matrix inverse of A That is x A1Ax A1y Solving for x by hand or by calculator would be tedious at best so MATLAB is used We first create A and y A 1 2 3sqrt3 1 sqrt53 sqrt7 1 y 1piexp1 The vector solution is found by using MATLABs inv function x invAy x 19999 38998 15999 It is also possible to use MATLABs left divide operator x Ay to find the same solution The left divide is generally more computationally efficient than matrix inverses As with matrix multiplication left division requires that the two arguments be conformable Of course Cramers rule can be used to compute individual solutions such as x1 by using vector indexing concatenation and MATLABs det command to compute determinants x1 detyA23detA x1 19999 Another nice application of matrices is the simultaneous creation of a family of curves Consider hαt eαt sin2π10t π6 over 0 t 02 Figure B14 shows hαt for α 0 and α 10 Lets investigate the family of curves hαt for α 0110 An inefficient way to solve this problem is create hαt for each α of interest This requires 11 individual cases Instead a matrix approach allows all 11 curves to be computed simultaneously First a vector is created that contains the desired values of α alpha 010 By using a sampling interval of one millisecond Δt 0001 a time vector is also created t 0000102 The result is a length201 column vector By replicating the time vector for each of the 11 curves required a time matrix T is created This replication can be accomplished by using an outer product between t and a 1 x 11 vector of ones T tones111 The result is a 201 x 11 matrix that has identical columns Right multiplying T by a diagonal matrix created from α columns of T can be individually scaled and the final result is computed H expTdiagalphasin2pi10Tpi6 Here H is a 201 x 11 matrix where each column corresponds to a different value of α That is H h0h1h10 where hx are column vectors As shown in Fig B15 the 11 desired curves are simultaneously displayed by using MATLABs plot command which allows matrix arguments plottH xlabelt ylabelht This example illustrates an important technique called vectorization which increases execution efficiency for interpretive languages such as MATLAB Algorithm vectorization uses matrix and vector operations to avoid manual repetition and loop structures It takes practice and effort to become proficient at vectorization but the worthwhile result is efficient compact code B77 Partial Fraction Expansions There are a wide variety of techniques and shortcuts to compute the partial fraction expansion of rational function Fx BαAα but few are more simple than the MATLAB residue command The basic form of this command is RPK residueBA The two input vectors B and A specify the polynomial coefficients of the numerator and denominator respectively These vectors are ordered in descending powers of the independent variable Three vectors are output The vector R contains the coefficients of each partial fraction vector P contains the corresponding roots of each partial fraction For a root repeated r times the r partial fractions are ordered in ascending powers When the rational function is not proper the vector K contains the direct terms which are ordered in descending powers of the independent variable To demonstrate the direct use of the residue command consider finding the partial fraction expansion of Fx x5 πx2x23 x5 πx4 8x3 32x 4 By hand the partial fraction expansion of Fx is difficult to compute MATLAB however makes short work of the expansion RPK residue1 0 0 0 0 pi1 sqrt8 0 sqrt32 4 R P K The two outputs R and P specify the partial fraction expansion of Fx is Fx x 28284 78888x 2 59713x 22 31107x 23 01112x 2 The signalprocessing toolbox function residuez is similar to the residue command and offers more convenient expansion of certain rational functions such as those commonly encountered in the study of discretetime systems Additional information about the residue and residuez commands is available from MATLABs help facilities B8 APPENDIX USEFUL MATHEMATICAL FORMULAS We conclude this chapter with a selection of useful mathematical facts B84 Taylor and Maclaurin Series fx fa x af a x a2 f a 2 k0 x ak k fka B87 Common Derivative Formulas d dx u d du fu du dx d dx uv u dv dx v du dx udv uv vdu B89 LHôpitals Rule If lim fxgx results in the indeterministic form 00 or then lim fx gx lim fx gx LathiBackground 2017925 1553 page 59 59 Problems 59 4 Cajori Florian A History of Mathematics 4th ed Chelsea New York 1985 5 Encyclopaedia Britannica Micropaedia IV 15th ed vol 11 p 1043 Chicago 1982 6 Singh Jagjit Great Ideas of Modern Mathematics Dover New York 1959 7 Dunham William Journey Through Genius Wiley New York 1990 PROBLEMS B11 Given a complex number w x jy the com plex conjugate of w is defined in rectangular coordinates as w xjy Use this fact to derive complex conjugation in polar form B12 Express the following numbers in polar form a wa 1 j b wb 1 ej c wc 4 j3 d wd 1 j4 j3 e we ejπ4 2ejπ4 f wf 1j 2j g wg 1 j4 j3 h wh 1j sinj B13 Express the following numbers in Cartesian rectangular form a wa j ej b wb 3ejπ4 c wc 1ej d wd 1 j4 j3 e we ejπ4 2ejπ4 f wf ej 1 g wg 12j h wh jjj j raised to the j raised to the j B14 Showing all work and simplifying your answer determine the real part of the following num bers a wa 1 j j 5e23j b wb 1 jln1 j B15 Showing all work and simplifying your answer determine the imaginary part of the following numbers a wa jejπ4 b wb 1 2je24j c wc tanj B16 For complex constant w prove a Rew w w2 b Imw w w2j B17 Given w x jy determine a Reew b Imew B18 For arbitrary complex constants w1 and w2 prove or disprove the following a Rejw1 Imw1 b Imjw1 Rew1 c Rew1 Rew2 Rew1 w2 d Imw1 Imw2 Imw1 w2 e Rew1Rew2 Rew1w2 f Imw1Imw2 Imw1w2 B19 Given w1 3 j4 and w2 2ejπ4 a Express w1 in standard polar form b Express w2 in standard rectangular form c Determine w12 and w22 d Express w1 w2 in standard rectangular form e Express w1 w2 in standard polar form f Express w1w2 in standard rectangular form g Express w1w2 in standard polar form B110 Repeat Prob B19 using w1 3 j42 and w2 25jej40π B111 Repeat Prob B19 using w1 j eπ4 and w2 cosj B112 Using the complex plane a Evaluate and locate the distinct solutions to w4 1 b Evaluate and locate the distinct solutions to w 1 j25 32 21 j c Sketch the solution to w 2j 3 d Graph wt 1 tejt for 10 t 10 B113 The distinct solutions to w w1n w2 lie on a circle in the complex plane as shown in Fig PB113 One solution is located on the real axis at 3 1 2732 and one solution is located on the imaginary axis at 31 0732 Determine w1 w2 and n B114 Find the distinct solutions to each of the following Use MATLAB to graph each solution set in the complex plane a w³ 2 b w i³ 1 c w² j 0 d 16w i4 81 0 e w 2j³ 8 f j w¹² 2 j2 g w 1¹² j2 a Show that coshw coshx cosy sinhx siny b Determine a similar expression for sinhw in rectangular form that only uses functions of real arguments such as sinx cosy and so on B25 Use Eulers identity to solve or prove the following a Find real positive constants c and φ for all real t such that 25 cos3t 15 sin3t π3 cos3t φ Sketch the resulting sinusoid b Prove that cosθ φ cosθ cosφ sinθ sinφ c Given real constants a b and α complex constant w and the fact that ewx dx 1w ewx ewx evaluate the integral ex sinax dx B26 A particularly boring stretch of interstate highway has a posted speed limit of 70 mph A highway engineer wants to install rumble bars raised ridges on the side of the road so that cars traveling the speed limit will produce quartersecond bursts of 1 kHz sound every second a strategy that is particularly effective at startling sleepy drivers awake Provide design specifications for the engineer B31 By hand accurately sketch the following signals over 0 t 1 a x₁t et b x₂t sin2πt c x₃t esin2πt B32 In 1950 the human population was approximately 25 billion people Assuming a doubling time of 40 years formulate an exponential model for human population in the form pt aekt where t is measured in years Sketch pt over the interval 1950 t 2100 According to this model in what year can we expect the population to reach the estimated 15 billion carrying capacity of the earth B33 Determine an expression for an exponentially decaying sinusoid that oscillates three times per second and whose amplitude envelopes decrease by 50 every 2 seconds Use MATLAB to plot the signal over 1 t 2 B34 By hand sketch the following against independent variable t a x₃t Ree212ty b x₄t ln3 e2t c x₆t 3 1ee12t B41 Consider the following system of equations 1 2 4 x₁ x₂ 3 Expressing all answers in rational form ratio of integers use Cramers rule to determine x₁ and x₂ Perform all calculations by hand including matrix determinants B42 Consider the following system of equations 1 2 0 x₁ 0 3 4 x₂ 5 0 6 x₃ Expressing all answers in rational form ratio of integers use Cramers rule to determine x₁ x₂ and x₃ Perform all calculations by hand including matrix determinants B43 Consider the following system of equations x₁ x₂ x₃ x₄ 1 x₁ 2x₂ 3x₃ 2 x₁ x₃ 7x₄ 3 2x₂ 3x₃ 4x₄ 4 Use Cramers rule to determine x₁ x₂ and x₃ Matrix determinants can be computed by using MATLABs det command B51 Determine the constants a₀ a₁ and a₂ of the partial fraction expansion Fs ss³ 1 a₀s 1 a₁s 1² a₂s 1³ 01LathiC01 2017925 1553 page 64 1 C H A P T E R SIGNALS AND SYSTEMS 1 In this chapter we shall discuss basic aspects of signals and systems We shall also introduce fundamental concepts and qualitative explanations of the hows and whys of systems theory thus building a solid foundation for understanding the quantitative analysis in the remainder of the book For simplicity the focus of this chapter is on continuoustime signals and systems Chapter 3 presents the same ideas for discretetime signals and systems SIGNALS A signal is a set of data or information Examples include a telephone or a television signal monthly sales of a corporation or daily closing prices of a stock market eg the Dow Jones averages In all these examples the signals are functions of the independent variable time This is not always the case however When an electrical charge is distributed over a body for instance the signal is the charge density a function of space rather than time In this book we deal almost exclusively with signals that are functions of time The discussion however applies equally well to other independent variables SYSTEMS Signals may be processed further by systems which may modify them or extract additional infor mation from them For example an antiaircraft gun operator may want to know the future location of a hostile moving target that is being tracked by his radar Knowing the radar signal he knows the past location and velocity of the target By properly processing the radar signal the input he can approximately estimate the future location of the target Thus a system is an entity that processes a set of signals inputs to yield another set of signals outputs A system may be made up of physical components as in electrical mechanical or hydraulic systems hardware realization or it may be an algorithm that computes an output from an input signal software realization 11 SIZE OF A SIGNAL The size of any entity is a number that indicates the largeness or strength of that entity Generally speaking the signal amplitude varies with time How can a signal that exists over a certain time 64 B52 Compute by hand the partial fraction expansions of the following rational functions a F₁s s² 9s⁴ 4s² 3 b F₂t t 1t² 1 c F₃t t 1t² 1 d F₄s s² 2s 3s³ 2s² d e F₅t 2t² 6t 5 f F₆t 2tt² 4t 3 g F₇t 1t² 2t 2 h F₈s ss² 4s 4 i F₉s s²s³ s 1 j F₁₀s s² 2s 1 k F₁₁s 3 5ss² 1 B61 A system of equations in terms of unknowns x₁ and x₂ and arbitrary constants a b c d e and f is given by ax₁ bx₂ c dx₁ ex₂ f a Represent this system of equations in matrix form b Identify specific constants a b c d e and f such that x₁ 3 and x₂ 2 Are the constants you selected unique c Identify nonzero constants a b c d e and f such that no solutions x₁ and x₂ exist d Identify nonzero constants a b c d e and f such that an infinite number of solutions x₁ and x₂ exist interval with varying amplitude be measured by one number that will indicate the signal size or signal strength Such a measure must consider not only the signal amplitude but also its duration For instance if we are to devise a single number V as a measure of the size of a human being we must consider not only his or her width girth but also the height If we make a simplifying assumption that the shape of a person is a cylinder of variable radius r which varies with the height h then one possible measure of the size of a person of height H is the persons volume V given by V π 0 to H r²h dh 111 Signal Energy 01LathiC01 2017925 1553 page 66 3 66 CHAPTER 1 SIGNALS AND SYSTEMS b xt t xt a t Figure 11 Examples of signals a a signal with finite energy and b a signal with finite power When xt is periodic xt2 is also periodic Hence the power of xt can be computed from Eq 12 by averaging xt2 over one period Comments The signal energy as defined in Eq 11 does not indicate the actual energy in the conventional sense of the signal because the signal energy depends not only on the signal but also on the load It can however be interpreted as the energy dissipated in a normalized load of a 1 ohm resistor if a voltage xt were to be applied across the 1 ohm resistor or if a current xt were to be passed through the 1 ohm resistor The measure of energy is therefore indicative of the energy capability of the signal not the actual energy For this reason the concepts of conservation of energy should not be applied to this signal energy Parallel observation applies to signal power defined in Eq 12 These measures are but convenient indicators of the signal size which prove useful in many applications For instance if we approximate a signal xt by another signal gt the error in the approximation is et xt gt The energy or power of et is a convenient indicator of the goodness of the approximation It provides us with a quantitative measure of determining the closeness of the approximation In communication systems during transmission over a channel message signals are corrupted by unwanted signals noise The quality of the received signal is judged by the relative sizes of the desired signal and the unwanted signal noise In this case the ratio of the message signal and noise signal powers signaltonoise power ratio is a good indication of the received signal quality Units of Energy and Power Equation 11 is not correct dimensionally This is because here we are using the term energy not in its conventional sense but to indicate the signal size The same observation applies to Eq 12 for power The units of energy and power as defined here depend on the nature of the signal xt If xt is a voltage signal its energy Ex has units of volts squaredseconds V2 s and its power Px has units of volts squared If xt is a current signal these units will be amperes squaredseconds A2 s and amperes squared respectively In this manner we may consider the area under a signal xt as a possible measure of its size because it takes account not only of the amplitude but also of the duration However this will be a defective measure because even for a large signal xt its positive and negative areas could cancel each other indicating a signal of small size This difficulty can be corrected by defining the signal size as the area under xt² which is always positive We call this measure the signal energy Eₓ defined as Eₓ to xt² dt 11 This definition simplifies for a realvalued signal xt to Eₓ to x²t dt There are also other possible measures of signal size such as the area under xt The energy measure however is not only more tractable mathematically but is also more meaningful as shown later in the sense that it is indicative of the energy that can be extracted from the signal 112 Signal Power Signal energy must be finite for it to be a meaningful measure of signal size A necessary condition for the energy to be finite is that the signal amplitude 0 as t Fig 11a Otherwise the integral in Eq 11 will not converge When the amplitude of xt does not 0 as t Fig 11b the signal energy is infinite A more meaningful measure of the signal size in such a case would be the time average of the energy if it exists This measure is called the power of the signal For a signal xt we define its power Pₓ as Pₓ lim T 1T T2 to T2 xt² dt 12 The first and second integrals on the righthand side are the powers of two sinusoids which are C1²2 and C2²2 as found in part a The third term the product of two sinusoids can be expressed as a sum of two sinusoids cosω1ω2tθ1θ2 and cosω1ω2tθ1θ2 respectively Now arguing as in part a we see that the third term is zero Hence we have Px C1²2 C2²2 and the rms value is C1²C2²2 We can readily extend this result to a sum of any number of sinusoids with distinct frequencies Thus if xt n1 to Cn cosωntθn assuming that none of the two sinusoids have identical frequencies and ωn ωm then Px 12 n1 to Cn² If xt also has a dc term as xt C0 n1 to Cn cosωntθn then Px C0² 12 n1 to Cn² c In this case the signal is complex and we use Eq 12 to compute the power Px lim T 1T T2 to T2 Dejω0t² dt Recall that ejω0t 1 so that Dejω0t² D² and Px D² 14 The rms value is D Comment In part b of Ex 12 we have shown that the power of the sum of two sinusoids is equal to the sum of the powers of the sinusoids It may appear that the power of x1t x2t is Px1 Px2 Unfortunately this conclusion is not true in general It is true only under a certain condition orthogonality discussed later Sec 653 12 SOME USEFUL SIGNAL OPERATIONS We discuss here three useful signal operations shifting scaling and inversion Since the independent variable in our signal description is time these operations are discussed as time shifting time scaling and time reversal inversion However this discussion is valid for functions having independent variables other than time eg frequency or distance 121 Time Shifting Consider a signal xt Fig 14a and the same signal delayed by T seconds Fig 14b which we shall denote by φt Whatever happens in xt Fig 14a at some instant t also happens in φt Fig 14b T seconds later at the instant t T Therefore φtT xt and φt xt T Therefore to timeshift a signal by T we replace t with t T Thus xt represents xt timeshifted by T seconds If T is positive the shift is to the right delay as in Fig 14b If T is negative the shift is to the left advance as in Fig 14c Clearly xt 2 is xt delayed rightshifted by 2 seconds and xt 2 is xt advanced leftshifted by 2 seconds The function xt can be described mathematically as xt e2t t 0 0 t 0 15 by replacing t with t 1 in Eq 15 Thus Write a mathematical description of the signal x3t in Fig 13c Next delay this signal by 2 seconds Sketch the delayed signal Show that this delayed signal x4t can be described mathematically as x4t 2t 2 for 2 t 3 and equal to 0 otherwise Now repeat the procedure with the signal advanced leftshifted by 1 second Show that this advanced signal x5t can be described as x5t 2t 1 for 1 t 0 and 0 otherwise Figure 17 a Signal xt b signal x3t and c signal xt2 DRILL 15 Compression and Expansion of Sinusoids EXAMPLE 15 Time Reversal of a Signal 01LathiC01 2017925 1553 page 78 15 78 CHAPTER 1 SIGNALS AND SYSTEMS Alternately we can first timecompress xt by factor 2 to obtain x2t then delay this signal by 3 replace t with t 3 to obtain x2t 6 13 CLASSIFICATION OF SIGNALS Classification helps us better understand and utilize the items around us Cars for example are classified as sports offroad family and so forth Knowing you have a sports car is useful in deciding whether to drive on a highway or on a dirt road Knowing you want to drive up a mountain you would probably choose an offroad vehicle over a family sedan Similarly there are several classes of signals Some signal classes are more suitable for certain applications than others Further different signal classes often require different mathematical tools Here we shall consider only the following classes of signals which are suitable for the scope of this book 1 Continuoustime and discretetime signals 2 Analog and digital signals 3 Periodic and aperiodic signals 4 Energy and power signals 5 Deterministic and probabilistic signals 131 ContinuousTime and DiscreteTime Signals A signal that is specified for a continuum of values of time t Fig 110a is a continuoustime signal and a signal that is specified only at discrete values of t Fig 110b is a discretetime signal Telephone and video camera outputs are continuoustime signals whereas the quarterly gross national product GNP monthly sales of a corporation and stock market daily averages are discretetime signals 132 Analog and Digital Signals The concept of continuous time is often confused with that of analog The two are not the same The same is true of the concepts of discrete time and digital A signal whose amplitude can take on any value in a continuous range is an analog signal This means that an analog signal amplitude can take on an infinite number of values A digital signal on the other hand is one whose amplitude can take on only a finite number of values Signals associated with a digital computer are digital because they take on only two values binary signals A digital signal whose amplitudes can take on M values is an Mary signal of which binary M 2 is a special case The terms continuous time and discrete time qualify the nature of a signal along the time horizontal axis The terms analog and digital on the other hand qualify the nature of the signal amplitude vertical axis Figure 111 shows examples of signals of various types It is clear that analog is not necessarily continuoustime and digital need not be discretetime Figure 111c shows an example of an analog discretetime signal An analog signal can be converted into a digital signal analogtodigital AD conversion through quantization rounding off as explained in Sec 83 xt 01LathiC01 2017925 1553 page 80 17 80 CHAPTER 1 SIGNALS AND SYSTEMS a c b t t t t d xt xt xt xt Figure 111 Examples of signals a analog continuous time b digital continuous time c analog discrete time and d digital discrete time t T0 xt Figure 112 A periodic signal of period T0 xt Therefore a periodic signal by definition must start at t and continue forever as illustrated in Fig 112 Another important property of a periodic signal xt is that xt can be generated by periodic extension of any segment of xt of duration T0 the period As a result we can generate xt from any segment of xt having a duration of one period by placing this segment and the reproduction thereof end to end ad infinitum on either side Figure 113 shows a periodic signal xt of period T0 6 The shaded portion of Fig 113a shows a segment of xt starting at t 1 and having a duration of one period 6 seconds This segment when repeated forever in either direction results in the periodic signal xt Figure 113b shows another shaded segment of xt of duration T0 starting at t 0 Again we see that this segment when repeated forever on either side results in xt The reader can verify that this construction is possible with any segment of xt starting at any instant as long as the segment duration is one period Quarterly GNP The return of recession In percent change seasonally adjusted annual rates Source Commerce Department news reports 01LathiC01 2017925 1553 page 82 19 82 CHAPTER 1 SIGNALS AND SYSTEMS eg an impulse and an everlasting sinusoid that cannot be generated in practice do serve a very useful purpose in the study of signals and systems 134 Energy and Power Signals A signal with finite energy is an energy signal and a signal with finite and nonzero power is a power signal The signals in Figs 12a and 12b are examples of energy and power signals respectively Observe that power is the time average of energy Since the averaging is over an infinitely large interval a signal with finite energy has zero power and a signal with finite power has infinite energy Therefore a signal cannot be both an energy signal and a power signal If it is one it cannot be the other On the other hand there are signals that are neither energy nor power signals The ramp signal is one such case Comments All practical signals have finite energies and are therefore energy signals A power signal must necessarily have infinite duration otherwise its power which is its energy averaged over an infinitely large interval will not approach a nonzero limit Clearly it is impossible to generate a true power signal in practice because such a signal has infinite duration and infinite energy Also because of periodic repetition periodic signals for which the area under xt2 over one period is finite are power signals however not all power signals are periodic DRILL 16 Neither Energy nor Power Show that an everlasting exponential eat is neither an energy nor a power signal for any real value of a However if a is imaginary it is a power signal with power Px 1 regardless of the value of a 135 Deterministic and Random Signals A signal whose physical description is known completely in either a mathematical form or a graphical form is a deterministic signal A signal whose values cannot be predicted precisely but are known only in terms of probabilistic description such as mean value or meansquared value is a random signal In this book we shall exclusively deal with deterministic signals Random signals are beyond the scope of this study 14 SOME USEFUL SIGNAL MODELS In the area of signals and systems the step the impulse and the exponential functions play very important roles Not only do they serve as a basis for representing other signals but their use can simplify many aspects of the signals and systems ut 1 t 0 0 t 0 Use the unit step function to describe the signal in Fig 116a Over the interval from 15 to 0 the signal can be described by a constant 2 and over the interval from 0 to 3 it can be described by 2et2 Therefore Show that the signal shown in Fig 118 can be described as xt t 1ut 1 t 2ut 2 ut 4 In the limit as α the pulse height and its width or duration 0 Yet the area under the pulse is unity regardless of the value of α because 0 aeαt dt 1 The definition of the unit impulse function given in Eq 19 is not mathematically rigorous which leads to serious difficulties First the impulse function does not define a unique function for example it can be shown that δt δt also satisfies Eq 19 Moreover δt is not even a true function in the ordinary sense An ordinary function is specified by its values for all time t The impulse function is zero everywhere except at t 0 and at this the only interesting part of its range it is undefined These difficulties are resolved by defining the impulse as a generalized function rather than an ordinary function A generalized function is defined by its effect on other functions instead of by its value at every instant of time This result shows that the unit step function can be obtained by integrating the unit impulse function Similarly the unit ramp function xt tut can be obtained by integrating the unit step function We may continue with unit parabolic function t²2 obtained by integrating the unit ramp and so on On the other side we have derivatives of impulse function which can be defined as generalized functions see Prob 1412 All these functions derived from the unit impulse function successive derivatives and integrals are called singularity functions Therefore est e sigma j omega t esigma t ej omega t esigma t cos omega t j sin omega t 113 Since s sigma j omega the conjugate of s then est e sigma j omega t esigma t ej omega t esigma t cos omega t j sin omega t and esigma t cos omega t frac12 est est 114 A comparison of Eq 113 with Eulers formula shows that est is a generalization of the function ej omega t where the frequency variable j omega is generalized to a complex variable s sigma j omega For this reason we designate the variable s as the complex frequency In fact function est encompasses a large class of functions The following functions are either special cases of or can be expressed in terms of est 1 A constant k ke0t s 0 2 A monotonic exponential est omega 0 s sigma 3 A sinusoid cos omega t sigma 0 s j omega 4 An exponentially varying sinusoid est cos s s sigma pm j omega These functions are illustrated in Fig 121 The complex frequency s can be conveniently represented on a complex frequency plane s plane as depicted in Fig 122 The horizontal axis is the real axis sigma axis and the vertical axis is the imaginary axis omega axis The absolute value of the imaginary part of s is omega the 01LathiC01 2017925 1553 page 91 28 14 Some Useful Signal Models 91 Exponentially increasing signals Left halfplane Right halfplane Real axis Imaginary axis jv s Exponentially decreasing signals Figure 122 Complex frequency plane radian frequency which indicates the frequency of oscillation of est the real part σ the neper frequency gives information about the rate of increase or decrease of the amplitude of est For signals whose complex frequencies lie on the real axis σ axis where ω 0 the frequency of oscillation is zero Consequently these signals are monotonically increasing or decreasing exponentials Fig 121a For signals whose frequencies lie on the imaginary axis ω axis where σ 0 eσt 1 Therefore these signals are conventional sinusoids with constant amplitude Fig 121b The case s 0 σ ω 0 corresponds to a constant dc signal because e0t 1 For the signals illustrated in Figs 121c and 121d both σ and ω are nonzero the frequency s is complex and does not lie on either axis The signal in Fig 121c decays exponentially Therefore σ is negative and s lies to the left of the imaginary axis In contrast the signal in Fig 121d grows exponentially Therefore σ is positive and s lies to the right of the imaginary axis Thus the s plane Fig 121 can be separated into two parts the left halfplane LHP corresponding to exponentially decaying signals and the right halfplane RHP corresponding to exponentially growing signals The imaginary axis separates the two regions and corresponds to signals of constant amplitude An exponentially growing sinusoid e2t cos 5t for example can be expressed as a linear combination of exponentials e2j5t and e2j5t with complex frequencies 2 j5 and 2 j5 respectively which lie in the RHP An exponentially decaying sinusoid e2t cos 5t can be expressed as a linear combination of exponentials e2j5t and e2j5t with complex frequencies 2 j5 and 2 j5 respectively which lie in the LHP A constantamplitude sinusoid cos 5t can be expressed as a linear combination of exponentials ej5t and ej5t with complex frequencies j5 which lie on the imaginary axis Observe that the monotonic exponentials e2t are also generalized sinusoids with complex frequencies 2 15 EVEN AND ODD FUNCTIONS A function xet is said to be an even function of t if it is symmetrical about the vertical axis A function xot is said to be an odd function of t if it is antisymmetrical about the vertical axis Mathematically expressed these symmetry conditions require xet xet and xot xot 115 An even function has the same value at the instants t and t for all values of t On the other hand the value of an odd function at the instant t is the negative of its value at the instant t An example even signal and an example odd signal are shown in Figs 123a and 123b respectively 151 Some Properties of Even and Odd Functions Even and odd functions have the following properties even function odd function odd function odd function odd function even function even function even function even function The proofs are trivial and follow directly from the definition of odd and even functions Eq 115 AREA Because of the symmetries of even and odd functions about the vertical axis it follows from Eq 115 or Fig 123 that intaa xet dt 2 int0a xet dt and intaa xot dt 0 116 These results are valid under the assumption that there is no impulse or its derivatives at the origin The proof of these statements is obvious from the plots of even and odd functions Formal proofs left as an exercise for the reader can be accomplished by using the definitions in Eq 115 Because of their properties study of odd and even functions proves useful in many applications as will become evident in later chapters 152 Even and Odd Components of a Signal Every signal xt can be expressed as a sum of even and odd components because xt frac12 xt xt frac12 xt xt 117 From the definitions in Eq 115 we can clearly see that the first component on the righthand side is an even function while the second component is odd This is apparent from the fact that replacing t by t in the first component yields the same function The same maneuver in the second component yields the negative of that component Find the even and odd components of eit From Eq 117 eit xet xot where xet frac12eit eit cos t and xot frac12eit eit jsin t 01LathiC01 2017925 1553 page 95 32 16 Systems 95 A MODIFICATION FOR COMPLEX SIGNALS While a complex signal can be decomposed into even and odd components it is more common to decompose complex signals using conjugate symmetries A complex signal xt is said to be conjugatesymmetric if xt xt A conjugatesymmetric signal is even in the real part and odd in the imaginary part Thus a real conjugatesymmetric signal is an even signal A signal is conjugateantisymmetric if xt xt A conjugateantisymmetric signal is odd in the real part and even in the imaginary part A real conjugateantisymmetric signal is an odd signal Any signal xt can be decomposed into a conjugatesymmetric portion xcst plus a conjugateantisymmetric portion xcat That is xt xcst xcat where xcst xt xt 2 and xcat xt xt 2 The proof is similar to the one for decomposing a signal into even and odd components As we shall see in later chapters conjugate symmetries commonly occur in realworld signals and their transforms 16 SYSTEMS As mentioned in Sec 11 systems are used to process signals to allow modification or extraction of additional information from the signals A system may consist of physical components hardware realization or of an algorithm that computes the output signal from the input signal software realization Roughly speaking a physical system consists of interconnected components which are characterized by their terminal inputoutput relationships In addition a system is governed by laws of interconnection For example in electrical systems the terminal relationships are the familiar voltagecurrent relationships for the resistors capacitors inductors transformers transistors and so on as well as the laws of interconnection ie Kirchhoffs laws We use these laws to derive mathematical equations relating the outputs to the inputs These equations then represent a mathematical model of the system A system can be conveniently illustrated by a black box with one set of accessible terminals where the input variables x1t x2t xjt are applied and another set of accessible terminals where the output variables y1t y2t ykt are observed Fig 125 The study of systems consists of three major areas mathematical modeling analysis and design Although we shall be dealing with mathematical modeling our main concern is with y1t y2t ykt x1t x2t xjt Figure 125 Representation of a system 01LathiC01 2017925 1553 page 97 34 17 Classification of Systems 97 past to t0 that we need to compute yt for t t0 Therefore the response of a system at t t0 can be determined from its inputs during the interval t0 to t and from certain initial conditions at t t0 In the preceding example we needed only one initial condition However in more complex systems several initial conditions may be necessary We know for example that in passive RLC networks the initial values of all inductor currents and all capacitor voltages are needed to determine the outputs at any instant t 0 if the inputs are given over the interval 0t 17 CLASSIFICATION OF SYSTEMS Systems may be classified broadly in the following categories 1 Linear and nonlinear systems 2 Constantparameter and timevaryingparameter systems 3 Instantaneous memoryless and dynamic with memory systems 4 Causal and noncausal systems 5 Continuoustime and discretetime systems 6 Analog and digital systems 7 Invertible and noninvertible systems 8 Stable and unstable systems Other classifications such as deterministic and probabilistic systems are beyond the scope of this text and are not considered 171 Linear and Nonlinear Systems THE CONCEPT OF LINEARITY A system whose output is proportional to its input is an example of a linear system But linearity implies more than this it also implies the additivity property that is if several inputs are acting on a system then the total effect on the system due to all these inputs can be determined by considering one input at a time while assuming all the other inputs to be zero The total effect is then the sum of all the component effects This property may be expressed as follows for a linear system if an input x1 acting alone has an effect y1 and if another input x2 also acting alone has an effect y2 then with both inputs acting on the system the total effect will be y1 y2 Thus if x1 y1 and x2 y2 then for all x1 and x2 x1 x2 y1 y2 120 In addition a linear system must satisfy the homogeneity or scaling property which states that for arbitrary real or imaginary number k if an input is increased kfold the effect also increases kfold Thus if x y Strictly speaking this means independent inductor currents and capacitor voltages 01LathiC01 2017925 1553 page 98 35 98 CHAPTER 1 SIGNALS AND SYSTEMS then for all real or imaginary k kx ky 121 Thus linearity implies two properties homogeneity scaling and additivity Both these properties can be combined into one property superposition which is expressed as follows If x1 y1 and x2 y2 then for all inputs x1 and x2 and all constants k1 and k2 k1x1 k2x2 k1y1 k2y2 122 There is another useful way to view the linearity condition described in Eq 122 the response of a linear system is unchanged whether the operations of summing and scaling precede the system sum and scale act on inputs or follow the system sum and scale act on outputs Thus linearity implies commutability between a system and the operations of summing and scaling It may appear that additivity implies homogeneity Unfortunately homogeneity does not always follow from additivity Drill 111 demonstrates such a case DRILL 111 Additivity but Not Homogeneity Show that a system with the input xt and the output yt related by yt Rext satisfies the additivity property but violates the homogeneity property Hence such a system is not linear Hint Show that Eq 121 is not satisfied when k is complex RESPONSE OF A LINEAR SYSTEM For the sake of simplicity we discuss only singleinput singleoutput SISO systems But the discussion can be readily extended to multipleinput multipleoutput MIMO systems A systems output for t 0 is the result of two independent causes the initial conditions of the system or the system state at t 0 and the input xt for t 0 If a system is to be linear the output must be the sum of the two components resulting from these two causes first the zeroinput response ZIR that results only from the initial conditions at t 0 with the input xt 0 for t 0 and then the zerostate response ZSR that results only from the input xt for t 0 when the initial conditions at t 0 are assumed to be zero When all the appropriate initial conditions are zero the system is said to be in zero state The system output is zero when the input is zero only if the system is in zero state In summary a linear system response can be expressed as the sum of the zeroinput and zerostate responses total response zeroinput response zerostate response A linear system must also satisfy the additional condition of smoothness where small changes in the systems inputs must result in small changes in its outputs 3 01LathiC01 2017925 1553 page 100 37 100 CHAPTER 1 SIGNALS AND SYSTEMS show that a system described by a differential equation of the form a0 dNyt dtN a1 dN1yt dtN1 aNyt bNM dMxt dtM bN1 dxt dt bNxt 125 is a linear system The coefficients ai and bi in this equation can be constants or functions of time Although here we proved only zerostate linearity it can be shown that such systems are also zeroinput linear and have the decomposition property DRILL 112 Linearity of a Differential Equation with TimeVarying Parameters Show that the system described by the following equation is linear dyt dt t2yt 2t 3xt DRILL 113 A Nonlinear Differential Equation Show that the system described by the following equation is nonlinear ytdyt dt 3yt xt MORE COMMENTS ON LINEAR SYSTEMS Almost all systems observed in practice become nonlinear when large enough signals are applied to them However it is possible to approximate most of the nonlinear systems by linear systems for smallsignal analysis The analysis of nonlinear systems is generally difficult Nonlinearities can arise in so many ways that describing them with a common mathematical form is impossible Not only is each system a category in itself but even for a given system changes in initial conditions or input amplitudes may change the nature of the problem On the other hand the superposition property of linear systems is a powerful unifying principle that allows for a general solution The superposition property linearity greatly simplifies the analysis of linear systems Because of the decomposition property we can evaluate separately the two components of the output The zeroinput response can be computed by assuming the input to be zero and the zerostate response can be computed by assuming zero initial conditions Moreover if we express an 01LathiC01 2017925 1553 page 103 40 17 Classification of Systems 103 It is possible to verify that the system in Fig 126 is a timeinvariant system Networks composed of RLC elements and other commonly used active elements such as transistors are timeinvariant systems A system with an inputoutput relationship described by a linear differential equation of the form given in Ex 110 Eq 125 is a linear timeinvariant LTI system when the coefficients ai and bi of such equation are constants If these coefficients are functions of time then the system is a linear timevarying system The system described in Drill 112 is linear time varying Another familiar example of a timevarying system is the carbon microphone in which the resistance R is a function of the mechanical pressure generated by sound waves on the carbon granules of the microphone The output current from the microphone is thus modulated by the sound waves as desired EXAMPLE 111 Assessing System Time Invariance Determine the time invariance of the following systems a yt xtut and b yt d dtxt a In this case the output equals the input for t 0 and is otherwise zero Clearly the input is being modified by a timedependent function so the system is likely time variant We can prove that the system is not time invariant through a counterexample Letting x1t δt1 we see that y1t 0 However x2t x1t2 δt1 produces an output of y2t δt 1 which does equal y1t 2 0 as timeinvariance would require Thus yt xtut is a time variant system b Although it appears that xt is being modified by a timedependent function this is not the case The output of this system is simply the slope of the input If the input is delayed so too is the output Applying input xt to the system produces output yt d dtxt delaying this output by T produces yt T d dtTxt T d dtxt T This is just the output of the system to a delayed input xt T Since the Tdelayed output of the system to input xt equals the output of the system to the Tdelayed input xt T the system is time invariant DRILL 114 A TimeVariant System Show that a system described by the following equation is a timevaryingparameter system yt sin txt 2 Hint Show that the system fails to satisfy the timeinvariance property 173 Instantaneous and Dynamic Systems As observed earlier a systems output at any instant t generally depends on the entire past input However in a special class of systems the output at any instant t depends only on its input at that 01LathiC01 2017925 1553 page 104 41 104 CHAPTER 1 SIGNALS AND SYSTEMS instant In resistive networks for example any output of the network at some instant t depends only on the input at the instant t In these systems past history is irrelevant in determining the response Such systems are said to be instantaneous or memoryless systems More precisely a system is said to be instantaneous or memoryless if its output at any instant t depends at most on the strength of its inputs at the same instant t and not on any past or future values of the inputs Otherwise the system is said to be dynamic or a system with memory A system whose response at t is completely determined by the input signals over the past T seconds interval from tT to t is a finitememory system with a memory of T seconds Networks containing inductive and capacitive elements generally have infinite memory because the response of such networks at any instant t is determined by their inputs over the entire past t This is true for the RC circuit of Fig 126 EXAMPLE 112 Assessing System Memory Determine whether the following systems are memoryless a yt 1 2xt 1 b yt d dtxt and c yt t 1xt a In this case the output at time t 1 is just twice the input at the same time t 1 Since the output at a particular time depends only on the strength of the input at the same time the system is memoryless b Although it appears that the output yt at time t depends on the input xt at the same time t we know that the slope derivative of xt cannot be determined solely from a single point There must be some memory even if infinitesimally small involved This is confirmed by using the fundamental theorem of calculus to express the system as yt lim T0 xt xt T T Since the output at a particular time depends on more than just the input at the same time the system is not memoryless c The output yt at time t is just the input xt at the same time t multiplied by the timedependent coefficient t 1 Since the output at a particular time depends only on the strength of the input at the same time the system is memoryless 174 Causal and Noncausal Systems A causal also known as a physical or nonanticipative system is one for which the output at any instant t0 depends only on the value of the input xt for t t0 In other words the value of the output at the present instant depends only on the past and present values of the input xt not on its future values To put it simply in a causal system the output cannot start before the input is applied If the response starts before the input it means that the system knows the input in the 01LathiC01 2017925 1553 page 106 43 106 CHAPTER 1 SIGNALS AND SYSTEMS a Here the output is a reflection of the input We can easily use a counterexample to disprove the causality of this system The input xt δt 1 which is nonzero at t 1 produces an output yt δt 1 which is nonzero at t 1 a time 2 seconds earlier than the input Clearly the system is not causal b In this case the output at time t depends on the input at future time of t 1 Clearly the system is not causal c In this case the output at time t 1 depends on the input one second in the past at time t Since the output does not depend on future values of the input the system is causal WHY STUDY NONCAUSAL SYSTEMS The foregoing discussion may suggest that noncausal systems have no practical purpose This is not the case they are valuable in the study of systems for several reasons First noncausal systems are realizable when the independent variable is other than time eg space Consider for example an electric charge of density qx placed along the x axis for x 0 This charge density produces an electric field Ex that is present at every point on the x axis from x to In this case the input ie the charge density qx starts at x 0 but its output the electric field Ex begins before x 0 Clearly this spacecharge system is noncausal This discussion shows that only temporal systems systems with time as independent variable must be causal to be realizable The terms before and after have a special connection to causality only when the independent variable is time This connection is lost for variables other than time Nontemporal systems such as those occurring in optics can be noncausal and still realizable Moreover even for temporal systems such as those used for signal processing the study of noncausal systems is important In such systems we may have all input data prerecorded This often happens with speech geophysical and meteorological signals and with space probes In such cases the inputs future values are available to us For example suppose we had a set of input signal records available for the system described by Eq 126 We can then compute yt since for any t we need only refer to the records to find the inputs value 2 seconds before and 2 seconds after t Thus noncausal systems can be realized although not in real time We may therefore be able to realize a noncausal system provided we are willing to accept a time delay in the output Consider a system whose output ˆyt is the same as yt in Eq 126 delayed by 2 seconds Fig 130c so that ˆyt yt 2 xt 4 xt Here the value of the output ˆy at any instant t is the sum of the values of the input x at t and at the instant 4 seconds earlier at t 4 In this case the output at any instant t does not depend on future values of the input and the system is causal The output of this system which is ˆyt is identical to that in Eq 126 or Fig 130b except for a delay of 2 seconds Thus a noncausal system may be realized or satisfactorily approximated in real time by using a causal system with a delay A third reason for studying noncausal systems is that they provide an upper bound on the performance of causal systems For example if we wish to design a filter for separating a signal from noise then the optimum filter is invariably a noncausal system Although unrealizable this 01LathiC01 2017925 1553 page 109 46 17 Classification of Systems 109 176 Analog and Digital Systems Analog and digital signals are discussed in Sec 132 A system whose input and output signals are analog is an analog system a system whose input and output signals are digital is a digital system A digital computer is an example of a digital binary system Observe that a digital computer is a digital as well as a discretetime system 177 Invertible and Noninvertible Systems A system S performs certain operations on input signals If we can obtain the input xt back from the corresponding output yt by some operation the system S is said to be invertible When several different inputs result in the same output as in a rectifier it is impossible to obtain the input from the output and the system is noninvertible Therefore for an invertible system it is essential that every input have a unique output so that there is a onetoone mapping between an input and the corresponding output The system that achieves the inverse operation of obtaining xt from yt is the inverse system for S For instance if S is an ideal integrator then its inverse system is an ideal differentiator Consider a system S connected in tandem with its inverse Si as shown in Fig 133 The input xt to this tandem system results in signal yt at the output of S and the signal yt which now acts as an input to Si yields back the signal xt at the output of Si Thus Si undoes the operation of S on xt yielding back xt A system whose output is equal to the input for all possible inputs is an identity system Cascading a system with its inverse system as shown in Fig 133 results in an identity system In contrast a rectifier specified by an equation yt xt is noninvertible because the rectification operation cannot be undone Inverse systems are very important in signal processing In many applications the signals are distorted during the processing and it is necessary to undo the distortion For instance in transmis sion of data over a communication channel the signals are distorted owing to nonideal frequency response and finite bandwidth of a channel It is necessary to restore the signal as closely as possi ble to its original shape Such equalization is also used in audio systems and photographic systems Si S xt yt xt Figure 133 A cascade of a system with its inverse results in an identity system EXAMPLE 114 Assessing System Invertibility Determine whether the following systems are invertible a yt xt b yt txt and c yt d dtxt a Here the output is a reflection of the input which does not cause any loss to the input The input can in fact be exactly recovered by simply reflecting the output xt yt which is to say that a reflecting system is its own inverse Thus yt xt is an invertible system 01LathiC01 2017925 1553 page 110 47 110 CHAPTER 1 SIGNALS AND SYSTEMS b In this case one might be tempted to recover the input from the output as xt 1 t yt This approach works almost everywhere except at t 0 where the input value x0 cannot be recovered Due to this single lost point the system yt txt is not invertible c Differentiation eliminates any dc component For example the inputs x1t 1 and x2t 2 both produce the same output yt 0 Given only yt 0 it is impossible to know if the original input was x1t 1 x2t 2 or something else entirely Since unique inputs do produce unique outputs we know that yt d dtxt is not an invertible system 178 Stable and Unstable Systems Systems can also be classified as stable or unstable systems Stability can be internal or external If every bounded input applied at the input terminal results in a bounded output the system is said to be stable externally External stability can be ascertained by measurements at the external terminals input and output of the system This type of stability is also known as the stability in the BIBO boundedinputboundedoutput sense The concept of internal stability is postponed to Ch 2 because it requires some understanding of internal system behavior introduced in that chapter EXAMPLE 115 Assessing System BIBO Stability Determine whether the following systems are BIBOstable a yt x2t b yt txt and c yt d dtxt a This system squares an input to produce the output If the input is bounded which is to say that xt Mx for all t then we see that yt x2t xt2 M2 x Since the output amplitude is guaranteed to be bounded for any boundedamplitude input the system yt x2t is BIBOstable b We can prove that yt txt is not BIBOstable with a simple example The boundedamplitude input xt ut produces the output yt tut whose amplitude grows to infinity as t Thus yt txt is a BIBOunstable system c We can prove that yt d dtxt is not BIBOstable with an example The boundedamplitude input xt ut produces the output yt δt whose amplitude is infinite at t 0 Thus yt d dtxt is a BIBOunstable system DRILL 116 A Noninvertible BIBOStable System Show that a system described by the equation yt x2t is noninvertible but BIBOstable 01LathiC01 2017925 1553 page 114 51 114 CHAPTER 1 SIGNALS AND SYSTEMS b Multiplying both sides of Eq 130 by D ie differentiating the equation we obtain 15D 5it Dxt Using the fact that it C dyt dt 1 5Dyt simple substitution yields 3D 1yt xt 131 DRILL 117 InputOutput Equation of a Series RLC Circuit with Inductor Voltage as Output If the inductor voltage vLt is taken as the output show that the RLC circuit in Fig 134 has an inputoutput equation of D2 3D 2vLt D2xt DRILL 118 InputOutput Equation of a Series RC Circuit with Capacitor Voltage as Output If the capacitor voltage vCt is taken as the output show that the RLC circuit in Fig 134 has an inputoutput equation of D2 3D 2vCt 2xt 182 Mechanical Systems Planar motion can be resolved into translational rectilinear motion and rotational torsional motion Translational motion will be considered first We shall restrict ourselves to motions in one dimension TRANSLATIONAL SYSTEMS The basic elements used in modeling translational systems are ideal masses linear springs and dashpots providing viscous damping The laws of various mechanical elements are now discussed For a mass M Fig 136a a force xt causes a motion yt and acceleration yt From Newtons law of motion xt Myt M d2yt dt2 MD2yt The force xt required to stretch or compress a linear spring Fig 136b by an amount yt is given by xt Kyt where K is the stiffness of the spring 01LathiC01 2017925 1553 page 115 52 18 System Model InputOutput Description 115 M a b c K B xt xt xt yt yt yt Figure 136 Some elements in translational mechanical systems For a linear dashpot Fig 136c which operates by virtue of viscous friction the force moving the dashpot is proportional to the relative velocity yt of one surface with respect to the other Thus xt Byt Bdyt dt BDyt where B is the damping coefficient of the dashpot or the viscous friction EXAMPLE 118 InputOutput Equation for a Translational Mechanical System Find the inputoutput relationship for the translational mechanical system shown in Fig 137a or its equivalent in Fig 137b The input is the force xt and the output is the mass position yt M K B Frictionless K B b a M M Byt Kyt xt yt xt yt xt yt c Figure 137 Mechanical system for Ex 118 01LathiC01 2017925 1553 page 116 53 116 CHAPTER 1 SIGNALS AND SYSTEMS In mechanical systems it is helpful to draw a freebody diagram of each junction which is a point at which two or more elements are connected In Fig 137 the point representing the mass is a junction The displacement of the mass is denoted by yt The spring is also stretched by the amount yt and therefore it exerts a force Kyt on the mass The dashpot exerts a force Byt on the mass as shown in the freebody diagram Fig 137c By Newtons second law the net force must be Myt Therefore Myt Byt Kyt xt or MD2 BD Kyt xt ROTATIONAL SYSTEMS In rotational systems the motion of a body may be defined as its motion about a certain axis The variables used to describe rotational motion are torque in place of force angular position in place of linear position angular velocity in place of linear velocity and angular acceleration in place of linear acceleration The system elements are rotational mass or moment of inertia in place of mass and torsional springs and torsional dashpots in place of linear springs and dashpots The terminal equations for these elements are analogous to the corresponding equations for translational elements If J is the moment of inertia or rotational mass of a rotating body about a certain axis then the external torque required for this motion is equal to J rotational mass times the angular acceleration If θt is the angular position of the body θt is its angular acceleration and torque J θt J d2θt dt2 JD2θt Similarly if K is the stiffness of a torsional spring per unit angular twist and θ is the angular displacement of one terminal of the spring with respect to the other then torque Kθt Finally the torque due to viscous damping of a torsional dashpot with damping coefficient B is torque B θt BDθt EXAMPLE 119 InputOutput Equation for Aircraft Roll Angle The attitude of an aircraft can be controlled by three sets of surfaces shown shaded in Fig 138 elevators rudder and ailerons By manipulating these surfaces one can set the aircraft on a desired flight path The roll angle ϕt can be controlled by deflecting in the opposite direction the two aileron surfaces as shown in Fig 138 Assuming only rolling motion find the equation relating the roll angle ϕt to the input deflection θt 01LathiC01 2017925 1553 page 117 54 18 System Model InputOutput Description 117 u u Elevator Elevator Rudder Aileron Aileron x w Figure 138 Attitude control of an airplane The aileron surfaces generate a torque about the roll axis proportional to the aileron deflection angle θt Let this torque be cθt where c is the constant of proportionality Air friction dissipates the torque B ϕt The torque available for rolling motion is then cθt B ϕt If J is the moment of inertia of the plane about the x axis roll axis then net torque J ϕt cθt B ϕt and J d2ϕt dt2 Bdϕt dt cθt or JD2 BDϕt cθt This is the desired equation relating the output roll angle ϕt to the input aileron angle θt The roll velocity ωt is ϕt If the desired output is the roll velocity ωt rather than the roll angle ϕt then the inputoutput equation would be J dωt dt Bωt cθt or JD Bωt cθt DRILL 119 InputOutput Equation of a Rotational Mechanical System Torque T t is applied to the rotational mechanical system shown in Fig 139a The torsional spring stiffness is K the rotational mass the cylinders moment of inertia about the shaft is J the viscous damping coefficient between the cylinder and the ground is B Find the equation 01LathiC01 2017925 1553 page 118 55 118 CHAPTER 1 SIGNALS AND SYSTEMS relating the output angle θt to the input torque T t Hint A freebody diagram is shown in Fig 139b ANSWER J d2θt dt2 Bdθt dt Kθt T t or JD2 BD Kθt T t J K B b a Bu t ut Kut J J Figure 139 Rotational system for Drill 119 183 Electromechanical Systems A wide variety of electromechanical systems is used to convert electrical signals into mechanical motion mechanical energy and vice versa Here we consider a rather simple example of an armaturecontrolled dc motor driven by a current source xt as shown in Fig 140a The torque T t generated in the motor is proportional to the armature current xt Therefore T t KTxt where KT is a constant of the motor This torque drives a mechanical load whose freebody diagram is shown in Fig 140b The viscous damping with coefficient B dissipates a torque B θt If J is the moment of inertia of the load including the rotor of the motor then the net torque T tB θt must be equal to J θt J θt T t B θt Thus JD2 BDθt T t KTxt which in conventional form can be expressed as J d2θt dt2 Bdθt dt KT xt 132 01LathiC01 2017925 1553 page 126 63 126 CHAPTER 1 SIGNALS AND SYSTEMS 111 MATLAB WORKING WITH FUNCTIONS Working with functions is fundamental to signals and systems applications MATLAB provides several methods of defining and evaluating functions An understanding and proficient use of these methods are therefore necessary and beneficial 1111 Anonymous Functions Many simple functions are most conveniently represented by using MATLAB anonymous functions An anonymous function provides a symbolic representation of a function defined in terms of MATLAB operators functions or other anonymous functions For example consider defining the exponentially damped sinusoid ft et cos2πt f t exptcos2pit In this context the symbol identifies the expression as an anonymous function which is assigned a name of f Parentheses following the symbol are used to identify the functions independent variables input arguments which in this case is the single time variable t Input arguments such as t are local to the anonymous function and are not related to any workspace variables with the same names Once defined ft can be evaluated simply by passing the input values of interest For example t 0 ft ans 1 evaluates ft at t 0 confirming the expected result of unity The same result is obtained by passing t 0 directly f0 ans 1 Vector inputs allow the evaluation of multiple values simultaneously Consider the task of plotting ft over the interval 2 t 2 Gross function behavior is clear ft should oscillate four times with a decaying envelope Since accurate hand sketches are cumbersome MATLABgenerated plots are an attractive alternative As the following example illustrates care must be taken to ensure reliable results Suppose vector t is chosen to include only the integers contained in 2 t 2 namely 21012 t 22 This vector input is evaluated to form a vector output ft ans 73891 27183 10000 03679 01353 01LathiC01 2017925 1553 page 127 64 111 MATLAB Working with Functions 127 The plot command graphs the result which is shown in Fig 146 plottft xlabelt ylabelft grid Grid lines added by using the grid command aid feature identification Unfortunately the plot does not illustrate the expected oscillatory behavior More points are required to adequately represent ft The question then is how many points is enough If too few points are chosen information is lost If too many points are chosen memory and time are wasted A balance is needed For oscillatory functions plotting 20 to 200 points per oscillation is normally adequate For the present case t is chosen to give 100 points per oscillation t 20012 Again the function is evaluated and plotted 2 15 1 05 0 05 1 15 2 t 0 2 4 6 8 ft Figure 146 ft et cos2πt for t 22 2 15 1 05 0 05 1 15 2 t 5 0 5 10 ft Figure 147 ft et cos2πt for t 20012 Sampling theory presented later formally addresses important aspects of this question 01LathiC01 2017925 1553 page 128 65 128 CHAPTER 1 SIGNALS AND SYSTEMS plottft xlabelt ylabelft grid The result shown in Fig 147 is an accurate depiction of ft 1112 Relational Operators and the Unit Step Function The unit step function ut arises naturally in many practical situations For example a unit step can model the act of turning on a system With the help of relational operators anonymous functions can represent the unit step function In MATLAB a relational operator compares two items If the comparison is true a logical true 1 is returned If the comparison is false a logical false 0 is returned Sometimes called indicator functions relational operators indicates whether a condition is true Six relational operators are available and The unit step function is readily defined using the relational operator u t 10t0 Any function with a jump discontinuity such as the unit step is difficult to plot Consider plotting ut by using t 22 t 22 plottut xlabelt ylabelut Two significant problems are apparent in the resulting plot shown in Fig 148 First MATLAB automatically scales plot axes to tightly bound the data In this case this normally desirable feature obscures most of the plot Second MATLAB connects plot data with lines making a true jump discontinuity difficult to achieve The coarse resolution of vector t emphasizes the effect by showing an erroneous sloping line between t 1 and t 0 The first problem is corrected by vertically enlarging the bounding box with the axis command The second problem is reduced but not eliminated by adding points to vector t 2 15 1 05 0 05 1 15 2 t 0 05 1 ut Figure 148 ut for t 22 01LathiC01 2017925 1553 page 129 66 111 MATLAB Working with Functions 129 2 15 1 05 0 05 1 15 2 t 0 05 1 ut Figure 149 ut for t 20012 with axis modification t 20012 plottut xlabelt ylabelut axis2 2 01 11 The fourelement vector argument of axis specifies x axis minimum x axis maximum y axis minimum and y axis maximum respectively The improved results are shown in Fig 149 Relational operators can be combined using logical AND logical OR and logical negation and respectively For example t0t1 and t0t1 both test if 0 t 1 To demonstrate consider defining and plotting the unit pulse pt ut ut 1 as shown in Fig 150 p t 10t0t1 t 10012 plottpt xlabelt ylabelpt utut1 axis1 2 1 11 Since anonymous functions can be constructed using other anonymous functions we could have used our previously defined unit step anonymous function to define pt as p t utut1 1 05 0 05 1 15 2 t 0 05 1 pt utut1 Figure 150 pt ut ut 1 over 1 t 2 01LathiC01 2017925 1553 page 130 67 130 CHAPTER 1 SIGNALS AND SYSTEMS For scalar operands MATLAB also supports two shortcircuit logical constructs A shortcircuit logical AND is performed by using and a shortcircuit logical OR is performed by using Shortcircuit logical operators are often more efficient than traditional logical operators because they test the second portion of the expression only when necessary That is when scalar expression A is found false in AB scalar expression B is not evaluated since a false result is already guaranteed Similarly scalar expression B is not evaluated when scalar expression A is found true in AB since a true result is already guaranteed 1113 Visualizing Operations on the Independent Variable Two operations on a functions independent variable are commonly encountered shifting and scaling Anonymous functions are well suited to investigate both operations Consider gt ftut et cos2πtut a causal version of ft MATLAB easily multiplies anonymous functions Thus we create gt by multiplying our anonymous functions for ft and ut g t ftut A combined shifting and scaling operation is represented by gat b where a and b are arbitrary real constants As an example consider plotting g2t 1 over 2 t 2 With a 2 the function is compressed by a factor of 2 resulting in twice the oscillations per unit t Adding the condition b 0 shifts the waveform to the left Given anonymous function g an accurate plot is nearly trivial to obtain t 20012 plottg2t1 xlabelt ylabelg2t1 grid Figure 151 confirms the expected waveform compression and left shift As a final check realize that function g turns on when the input argument is zero Therefore g2t 1 should turn on when 2t 1 0 or at t 05 a fact again confirmed by Fig 151 2 15 1 05 0 05 1 15 2 t 1 05 0 05 1 g2t1 Figure 151 g2t 1 over 2 t 2 Although we define g in terms of f and u the function g will not change if we later change either f or u unless we subsequently redefine g as well 01LathiC01 2017925 1553 page 131 68 111 MATLAB Working with Functions 131 2 15 1 05 0 05 1 15 2 t 1 05 0 05 1 gt1 Figure 152 gt 1 over 2 t 2 2 15 1 05 0 05 1 15 2 t 1 05 0 05 1 15 ht Figure 153 ht g2t 1 gt 1 over 2 t 2 Next consider plotting gt 1 over 2 t 2 Since a 0 the waveform will be reflected Adding the condition b 0 shifts the final waveform to the right plottgt1 xlabelt ylabelgt1 grid Figure 152 confirms both the reflection and the right shift Up to this point Figs 151 and 152 could be reasonably sketched by hand Consider plotting the more complicated function ht g2t 1 gt 1 over 2 t 2 Fig 153 an accurate hand sketch would be quite difficult With MATLAB the work is much less burdensome plottg2t1gt1 xlabelt ylabelht grid 1114 Numerical Integration and Estimating Signal Energy Interesting signals often have nontrivial mathematical representations Computing signal energy which involves integrating the square of these expressions can be a daunting task Fortunately many difficult integrals can be accurately estimated by means of numerical integration techniques 01LathiC01 2017925 1553 page 134 71 134 CHAPTER 1 SIGNALS AND SYSTEMS 4 An everlasting signal starts at t and continues forever to t Hence periodic signals are everlasting signals A causal signal is a signal that is zero for t 0 5 A signal with finite energy is an energy signal Similarly a signal with a finite and nonzero power meansquare value is a power signal A signal can be either an energy signal or a power signal but not both However there are signals that are neither energy nor power signals 6 A signal whose physical description is known completely in a mathematical or graphical form is a deterministic signal A random signal is known only in terms of its probabilistic description such as mean value or meansquare value rather than by its mathematical or graphical form A signal xt delayed by T seconds rightshifted can be expressed as xt T on the other hand xt advanced by T leftshifted is xt T A signal xt timecompressed by a factor aa 1 is expressed as xat on the other hand the same signal timeexpanded by factor aa 1 is xta The signal xt when timereversed can be expressed as xt The unit step function ut is very useful in representing causal signals and signals with different mathematical descriptions over different intervals In the classical Dirac definition the unit impulse function δt is characterized by unit area and is concentrated at a single instant t 0 The impulse function has a sampling or sifting property which states that the area under the product of a function with a unit impulse is equal to the value of that function at the instant at which the impulse is located assuming the function to be continuous at the impulse location In the modern approach the impulse function is viewed as a generalized function and is defined by the sampling property The exponential function est where s is complex encompasses a large class of signals that includes a constant a monotonic exponential a sinusoid and an exponentially varying sinusoid A real signal that is symmetrical about the vertical axis t 0 is an even function of time and a real signal that is antisymmetrical about the vertical axis is an odd function of time The product of an even function and an odd function is an odd function However the product of an even function and an even function or an odd function and an odd function is an even function The area under an odd function from t a to a is always zero regardless of the value of a On the other hand the area under an even function from t a to a is two times the area under the same function from t 0 to a or from t a to 0 Every signal can be expressed as a sum of odd and even functions of time A system processes input signals to produce output signals response The input is the cause and the output is its effect In general the output is affected by two causes the internal conditions of the system such as the initial conditions and the external input Systems can be classified in several ways 1 Linear systems are characterized by the linearity property which implies superposition if several causes such as various inputs and initial conditions are acting on a linear system the total output response is the sum of the responses from each cause assuming that all the remaining causes are absent A system is nonlinear if superposition does not hold 2 In timeinvariant systems system parameters do not change with time The parameters of timevaryingparameter systems change with time 3 For memoryless or instantaneous systems the system response at any instant t depends only on the value of the input at t For systems with memory also known as dynamic 01LathiC01 2017925 1553 page 135 72 112 Summary 135 systems the system response at any instant t depends not only on the present value of the input but also on the past values of the input values before t 4 In contrast if a system response at t also depends on the future values of the input values of input beyond t the system is noncausal In causal systems the response does not depend on the future values of the input Because of the dependence of the response on the future values of input the effect response of noncausal systems occurs before the cause When the independent variable is time temporal systems the noncausal systems are prophetic systems and therefore unrealizable although close approximation is possible with some time delay in the response Noncausal systems with independent variables other than time eg space are realizable 5 Systems whose inputs and outputs are continuoustime signals are continuoustime systems systems whose inputs and outputs are discretetime signals are discretetime systems If a continuoustime signal is sampled the resulting signal is a discretetime signal We can process a continuoustime signal by processing the samples of the signal with a discretetime system 6 Systems whose inputs and outputs are analog signals are analog systems those whose inputs and outputs are digital signals are digital systems 7 If we can obtain the input xt back from the output yt of a system S by some operation the system S is said to be invertible Otherwise the system is noninvertible 8 A system is stable if bounded input produces bounded output This defines external stability because it can be ascertained from measurements at the external terminals of the system External stability is also known as the stability in the BIBO boundedinputboundedoutput sense Internal stability discussed later in Ch 2 is measured in terms of the internal behavior of the system The system model derived from a knowledge of the internal structure of the system is its internal description In contrast an external description is a representation of a system as seen from its input and output terminals it can be obtained by applying a known input and measuring the resulting output In the majority of practical systems an external description of a system so obtained is equivalent to its internal description At times however the external description fails to describe the system adequately Such is the case with the socalled uncontrollable or unobservable systems A system may also be described in terms of certain set of key variables called state variables In this description an Nthorder system can be characterized by a set of N simultaneous firstorder differential equations in N state variables State equations of a system represent an internal description of that system REFERENCES 1 Papoulis A The Fourier Integral and Its Applications McGrawHill New York 1962 2 Mason S J Electronic Circuits Signals and Systems Wiley New York 1960 3 Kailath T Linear Systems PrenticeHall Englewood Cliffs NJ 1980 4 Lathi B P Signals and Systems BerkeleyCambridge Press Carmichael CA 1987 02LathiC02 2017925 1554 page 150 1 C H A P T E R TIMEDOMAIN ANALYSIS OF CONTINUOUSTIME SYSTEMS 2 In this book we consider two methods of analysis of linear timeinvariant LTI systems the timedomain method and the frequencydomain method In this chapter we discuss the timedomain analysis of linear timeinvariant continuoustime LTIC systems 21 INTRODUCTION For the purpose of analysis we shall consider linear differential systems This is the class of LTIC systems introduced in Ch 1 for which the input xt and the output yt are related by linear differential equations of the form dNyt dtN a1 dN1yt dtN1 aN1 dyt dt aNyt bNM dMxt dtM bNM1 dM1xt dtM1 bN1 dxt dt bNxt 21 where all the coefficients ai and bi are constants Using operator notation D to represent ddt we can express this equation as DN a1DN1 aN1D aNyt bNMDM bNM1DM1 bN1D bNxt or QDyt PDxt 22 where the polynomials QD and PD are QD DN a1DN1 aN1D aN PD bNMDM bNM1DM1 bN1D bN Theoretically the powers M and N in the foregoing equations can take on any value However practical considerations make M N undesirable for two reasons In Sec 433 we shall show that 150 02LathiC02 2017925 1554 page 151 2 22 System Response to Internal Conditions The ZeroInput Response 151 an LTIC system specified by Eq 21 acts as an M Nthorder differentiator A differentiator represents an unstable system because a bounded input like the step input results in an unbounded output δt Second noise is enhanced by a differentiator Noise is a wideband signal containing components of all frequencies from 0 to a very high frequency approaching Hence noise contains a significant amount of rapidly varying components We know that the derivative of any rapidly varying signal is high Therefore any system specified by Eq 21 in which M N will magnify the highfrequency components of noise through differentiation It is entirely possible for noise to be magnified so much that it swamps the desired system output even if the noise signal at the systems input is tolerably small Hence practical systems generally use M N For the rest of this text we assume implicitly that M N For the sake of generality we shall assume M N in Eq 21 In Ch 1 we demonstrated that a system described by Eq 22 is linear Therefore its response can be expressed as the sum of two components the zeroinput response and the zerostate response decomposition property Therefore total response zeroinput response zerostate response The zeroinput response is the system output when the input xt 0 and thus it is the result of internal system conditions such as energy storages initial conditions alone It is independent of the external input xt In contrast the zerostate response is the system output to the external input xt when the system is in zero state meaning the absence of all internal energy storages that is all initial conditions are zero 22 SYSTEM RESPONSE TO INTERNAL CONDITIONS THE ZEROINPUT RESPONSE The zeroinput response y0t is the solution of Eq 22 when the input xt 0 so that QDy0t 0 Noise is any undesirable signal natural or manufactured that interferes with the desired signals in the system Some of the sources of noise are the electromagnetic radiation from stars the random motion of electrons in system components interference from nearby radio and television stations transients produced by automobile ignition systems and fluorescent lighting We can verify readily that the system described by Eq 22 has the decomposition property If y0t is the zeroinput response then by definition QDy0t 0 If yt is the zerostate response then yt is the solution of QDyt PDxt subject to zero initial conditions zerostate Adding these two equations we have QDy0t yt PDxt Clearly y0t yt is the general solution of Eq 22 02LathiC02 2017925 1554 page 152 3 152 CHAPTER 2 TIMEDOMAIN ANALYSIS OF CONTINUOUSTIME SYSTEMS or DN a1DN1 aN1D aNy0t 0 23 A solution to this equation can be obtained systematically 1 However we will take a shortcut by using heuristic reasoning Equation 23 shows that a linear combination of y0t and its N successive derivatives is zero not at some values of t but for all t Such a result is possible if and only if y0t and all its N successive derivatives are of the same form Otherwise their sum can never add to zero for all values of t We know that only an exponential function eλt has this property So let us assume that y0t ceλt is a solution to Eq 23 Then Dy0t dy0t dt cλeλt D2y0t d2y0t dt2 cλ2eλt DNy0t dNy0t dtN cλNeλt Substituting these results in Eq 23 we obtain cλN a1λN1 aN1λ aNeλt 0 For a nontrivial solution of this equation λN a1λN1 aN1λ aN 0 24 This result means that ceλt is indeed a solution of Eq 23 provided λ satisfies Eq 24 Note that the polynomial in Eq 24 is identical to the polynomial QD in Eq 23 with λ replacing D Therefore Eq 24 can be expressed as Qλ 0 Expressing Qλ in factorized form we obtain Qλ λ λ1λ λ2 λ λN 0 25 Clearly λ has N solutions λ1 λ2 λN assuming that all λi are distinct Consequently Eq 23 has N possible solutions c1eλ1t c2eλ2t cNeλNt with c1 c2 cN as arbitrary constants We 02LathiC02 2017925 1554 page 153 4 22 System Response to Internal Conditions The ZeroInput Response 153 can readily show that a general solution is given by the sum of these N solutions so that y0t c1eλ1t c2eλ2t cNeλNt 26 where c1 c2 cN are arbitrary constants determined by N constraints the auxiliary conditions on the solution Observe that the polynomial Qλ which is characteristic of the system has nothing to do with the input For this reason the polynomial Qλ is called the characteristic polynomial of the system The equation Qλ 0 is called the characteristic equation of the system Equation 25 clearly indicates that λ1 λ2 λN are the roots of the characteristic equation consequently they are called the characteristic roots of the system The terms characteristic values eigenvalues and natural frequencies are also used for characteristic roots The exponentials eλit i 12 n in the zeroinput response are the characteristic modes also known as natural modes or simply as modes of the system There is a characteristic mode for each characteristic root of the system and the zeroinput response is a linear combination of the characteristic modes of the system An LTIC systems characteristic modes comprise its single most important attribute Characteristic modes not only determine the zeroinput response but also play an important role in determining the zerostate response In other words the entire behavior of a system is dictated primarily by its characteristic modes In the rest of this chapter we shall see the pervasive presence of characteristic modes in every aspect of system behavior REPEATED ROOTS The solution of Eq 23 as given in Eq 26 assumes that the N characteristic roots λ1 λ2 λN are distinct If there are repeated roots same root occurring more than once the form of the solution is modified slightly By direct substitution we can show that the solution of the equation D λ2y0t 0 is given by y0t c1 c2teλt To prove this assertion assume that y1t y2t yNt are all solutions of Eq 23 Then QDy1t 0 QDy2t 0 QDyNt 0 Multiplying these equations by c1 c2 cN respectively and adding them together yield QDc1y1t c2y2t cNynt 0 This result shows that c1y1t c2y2t cNynt is also a solution of the homogeneous equation Eq 23 Eigenvalue is German for characteristic value 02LathiC02 2017925 1554 page 155 6 22 System Response to Internal Conditions The ZeroInput Response 155 EXAMPLE 21 Finding the ZeroInput Response Find y0t the zeroinput response of the response for an LTIC system described by a the simpleroot system D2 3D 2yt Dxt with initial conditions y00 0 and y00 5 b the repeatedroot system D2 6D 9yt 3D 5xt with initial conditions y00 3 and y00 7 c the complexroot system D2 4D 40yt D 2xt with initial conditions y00 2 and y00 1678 a Note that y0t being the zeroinput response xt 0 is the solution of D2 3D 2y0t 0 The characteristic polynomial of the system is λ2 3λ 2 The characteristic equation of the system is therefore λ2 3λ 2 λ 1λ 2 0 The characteristic roots of the system are λ1 1 and λ2 2 and the characteristic modes of the system are et and e2t Consequently the zeroinput response is y0t c1et c2e2t Differentiating this expression we obtain y0t c1et 2c2e2t To determine the constants c1 and c2 we set t 0 in the equations for y0t and y0t and substitute the initial conditions y00 0 and y00 5 yielding 0 c1 c2 5 c1 2c2 Solving these two simultaneous equations in two unknowns for c1 and c2 yields c1 5 and c2 5 Therefore y0t 5et 5e2t 29 This is the zeroinput response of yt Because y0t is present at t 0 we are justified in assuming that it exists for t 0 b The characteristic polynomial is λ2 6λ 9 λ 32 and its characteristic roots are λ1 3 λ2 3 repeated roots Consequently the characteristic modes of the system are e3t and te3t The zeroinput response being a linear combination of the characteristic modes is given by y0t c1 c2te3t y0t may be present even before t 0 However we can be sure of its presence only from t 0 onward 02LathiC02 2017925 1554 page 157 8 22 System Response to Internal Conditions The ZeroInput Response 157 EXAMPLE 22 Using MATLAB to Find Polynomial Roots Find the roots λ1 and λ2 of the polynomial λ2 4λ k for three values of k a k 3 b k 4 and c k 40 a r roots1 4 3 r 3 1 For k 3 the polynomial roots are therefore λ1 3 and λ2 1 b r roots1 4 4 r 2 2 For k 4 the polynomial roots are therefore λ1 λ2 2 c r roots1 4 40 r 200600i 200600i For k 40 the polynomial roots are therefore λ1 2 j6 and λ2 2 j6 EXAMPLE 23 Using MATLAB to Find the ZeroInput Response Consider an LTIC system specified by the differential equation D2 4D kyt 3D 5xt Using initial conditions y00 3 and y00 7 apply MATLABs dsolve command to determine the zeroinput response when a k 3 b k 4 and c k 40 a y0 dsolveD2y4Dy3y0y03Dy07t y0 1expt 2exp3t For k 3 the zeroinput response is therefore y0t et 2e3t b y0 dsolveD2y4Dy4y0y03Dy07t y0 3exp2t texp2t 02LathiC02 2017925 1554 page 158 9 158 CHAPTER 2 TIMEDOMAIN ANALYSIS OF CONTINUOUSTIME SYSTEMS For k 4 the zeroinput response is therefore y0t 3e2t te2t c y0 dsolveD2y4Dy40y0y03Dy07t y0 3cos6texp2t sin6t6exp2t For k 40 the zeroinput response is therefore y0t 3e2t cos6t 1 6e2t sin6t DRILL 21 Finding the ZeroInput Response of a FirstOrder System Find the zeroinput response of an LTIC system described by D 5yt xt if the initial condition is y0 5 ANSWER y0t 5e5t t 0 DRILL 22 Finding the ZeroInput Response of a SecondOrder System Letting y00 1 and y00 4 solve D2 2Dy0t 0 ANSWER y0t 3 2e2t t 0 PRACTICAL INITIAL CONDITIONS AND THE MEANING OF 0 AND 0 In Ex 21 the initial conditions y00 and y00 were supplied In practical problems we must derive such conditions from the physical situation For instance in an RLC circuit we may be given the conditions initial capacitor voltages initial inductor currents etc From this information we need to derive y00 y00 for the desired variable as demonstrated in the next example In much of our discussion the input is assumed to start at t 0 unless otherwise mentioned Hence t 0 is the reference point The conditions immediately before t 0 just before the input is applied are the conditions at t 0 and those immediately after t 0 just after the input is applied are the conditions at t 0 compare this with the historical time frames BCE and CE In 02LathiC02 2017925 1554 page 159 10 22 System Response to Internal Conditions The ZeroInput Response 159 practice we are likely to know the initial conditions at t 0 rather than at t 0 The two sets of conditions are generally different although in some cases they may be identical The total response yt consists of two components the zeroinput response y0t response due to the initial conditions alone with xt 0 and the zerostate response resulting from the input alone with all initial conditions zero At t 0 the total response yt consists solely of the zeroinput response y0t because the input has not started yet Hence the initial conditions on yt are identical to those of y0t Thus y0 y00 y0 y00 and so on Moreover y0t is the response due to initial conditions alone and does not depend on the input xt Hence application of the input at t 0 does not affect y0t This means the initial conditions on y0t at t 0 and 0 are identical that is y00 y00 are identical to y00 y00 respectively It is clear that for y0t there is no distinction between the initial conditions at t 0 0 and 0 They are all the same But this is not the case with the total response yt which consists of both the zeroinput and zerostate responses Thus in general y0 y0 y0 y0 and so on EXAMPLE 24 Consideration of Initial Conditions A voltage xt 10e3tut is applied at the input of the RLC circuit illustrated in Fig 22a Find the loop current yt for t 0 if the initial inductor current is zero y0 0 and the initial capacitor voltage is 5 volts vC0 5 The differential loop equation relating yt to xt was derived in Eq 129 as D2 3D 2yt Dxt The zerostate component of yt resulting from the input xt assuming that all initial conditions are zero that is y0 vC0 0 will be obtained later in Ex 29 In this example we shall find the zeroinput reponse y0t For this purpose we need two initial conditions y00 and y00 These conditions can be derived from the given initial conditions y0 0 and vC0 5 as follows Recall that y0t is the loop current when the input terminals are shorted so that the input xt 0 zeroinput as depicted in Fig 22b We now compute y00 and y00 the values of the loop current and its derivative at t 0 from the initial values of the inductor current and the capacitor voltage Remember that the inductor current cannot change instantaneously in the absence of an impulsive voltage Similarly the capacitor voltage cannot change instantaneously in the absence of an impulsive current Therefore when the input terminals are shorted at t 0 the inductor current is still zero and the capacitor voltage is still 5 volts Thus y00 0 02LathiC02 2017925 1554 page 161 12 22 System Response to Internal Conditions The ZeroInput Response 161 The loop current y0 y0 0 because it cannot change instantaneously in the absence of impulsive voltage The same is true of the capacitor voltage Hence vC0 vC0 5 Substituting these values in the foregoing equations we obtain y0 5 and y0 5 Thus y0 0 y0 5 and y0 0 y0 5 210 DRILL 23 ZeroInput Response of an RC Circuit In the circuit in Fig 22a the inductance L 0 and the initial capacitor voltage vC0 30 volts Show that the zeroinput component of the loop current is given by y0t 10e2t3 for t 0 INDEPENDENCE OF THE ZEROINPUT AND ZEROSTATE RESPONSES In Ex 24 we computed the zeroinput component without using the input xt The zerostate response can be computed from the knowledge of the input xt alone the initial conditions are assumed to be zero system in zero state The two components of the system response the zeroinput and zerostate responses are independent of each other The two worlds of zeroinput response and zerostate response coexist side by side neither one knowing or caring what the other is doing For each component the other is totally irrelevant ROLE OF AUXILIARY CONDITIONS IN SOLUTION OF DIFFERENTIAL EQUATIONS The solution of a differential equation requires additional pieces of information the auxiliary conditions Why We now show heuristically why a differential equation does not in general have a unique solution unless some additional constraints or conditions on the solution are known Differentiation operation is not invertible unless one piece of information about yt is given To get back yt from dydt we must know one piece of information such as y0 Thus differentiation is an irreversible noninvertible operation during which certain information is lost To invert this operation one piece of information about yt must be provided to restore the original yt Using a similar argument we can show that given d2ydt2 we can determine yt uniquely only if two additional pieces of information constraints about yt are given In general to determine yt uniquely from its Nth derivative we need N additional pieces of information constraints about yt These constraints are also called auxiliary conditions When these conditions are given at t 0 they are called initial conditions 221 Some Insights into the ZeroInput Behavior of a System By definition the zeroinput response is the system response to its internal conditions assuming that its input is zero Understanding this phenomenon provides interesting insight into system behavior If a system is disturbed momentarily from its rest position and if the disturbance is then 02LathiC02 2017925 1554 page 163 14 23 The Unit Impulse Response ht 163 Clearly the loop current yt ce2t is sustained by the RL circuit on its own without the necessity of an external input THE RESONANCE PHENOMENON We have seen that any signal consisting of a systems characteristic mode is sustained by the system on its own the system offers no obstacle to such signals Imagine what would happen if we were to drive the system with an external input that is one of its characteristic modes This would be like pouring gasoline on a fire in a dry forest or hiring a child to eat ice cream A child would gladly do the job without pay Think what would happen if he were paid by the amount of ice cream he ate He would work overtime He would work day and night until he became sick The same thing happens with a system driven by an input of the form of characteristic mode The system response grows without limit until it burns out We call this behavior the resonance phenomenon An intelligent discussion of this important phenomenon requires an understanding of the zerostate response for this reason we postpone this topic until Sec 267 23 THE UNIT IMPULSE RESPONSE ht In Ch 1 we explained how a system response to an input xt may be found by breaking this input into narrow rectangular pulses as illustrated earlier in Fig 127a and then summing the system response to all the components The rectangular pulses become impulses in the limit as their widths approach zero Therefore the system response is the sum of its responses to various impulse components This discussion shows that if we know the system response to an impulse input we can determine the system response to an arbitrary input xt We now discuss a method of determining ht the unit impulse response of an LTIC system described by the Nthorder differential equation Eq 21 dNyt dtN a1 dN1yt dtN1 aN1 dyt dt aNyt bNM dMxt dtM bNM1 dM1xt dtM1 bN1 dxt dt bNxt Recall that noise considerations restrict practical systems to M N Under this constraint the most general case is M N Therefore Eq 21 can be expressed as DN a1DN1 aN1D aNyt b0DN b1DN1 bN1D bNxt 211 Before deriving the general expression for the unit impulse response ht it is illuminating to understand qualitatively the nature of ht The impulse response ht is the system response to an impulse input δt applied at t 0 with all the initial conditions zero at t 0 An impulse input δt is like lightning which strikes instantaneously and then vanishes But in its wake in that single moment objects that have been struck are rearranged Similarly an impulse input δt appears momentarily at t 0 and then it is gone forever But in that moment it generates energy storages that is it creates nonzero initial conditions instantaneously within the system at In practice the system in resonance is more likely to go in saturation because of high amplitude levels 02LathiC02 2017925 1554 page 164 15 164 CHAPTER 2 TIMEDOMAIN ANALYSIS OF CONTINUOUSTIME SYSTEMS t 0 Although the impulse input δt vanishes for t 0 so that the system has no input after the impulse has been applied the system will still have a response generated by these newly created initial conditions The impulse response ht therefore must consist of the systems characteristic modes for t 0 As a result ht characteristic mode terms t 0 This response is valid for t 0 But what happens at t 0 At a single moment t 0 there can at most be an impulse so the form of the complete response ht is ht A0δt characteristic mode terms t 0 212 because ht is the unit impulse response Setting xt δt and yt ht in Eq 211 yields DN a1DN1 aN1D aNht b0DN b1DN1 bN1D bNδt In this equation we substitute ht from Eq 212 and compare the coefficients of similar impulsive terms on both sides The highest order of the derivative of impulse on both sides is N with its coefficient value as A0 on the lefthand side and b0 on the righthand side The two values must be matched Therefore A0 b0 and ht b0δt characteristic modes 213 In Eq 211 if M N b0 0 Hence the impulse term b0δt exists only if M N The unknown coefficients of the N characteristic modes in ht in Eq 213 can be determined by using the technique of impulse matching as explained in the following example EXAMPLE 25 Impulse Response via Impulse Matching Find the impulse response ht for a system specified by D2 5D 6yt D 1xt 214 In this case b0 0 Hence ht consists of only the characteristic modes The characteristic polynomial is λ2 5λ 6 λ 2λ 3 The roots are 2 and 3 Hence the impulse It might be possible for the derivatives of δt to appear at the origin However if M N it is impossible for ht to have any derivatives of δt This conclusion follows from Eq 211 with xt δt and yt ht The coefficients of the impulse and all its derivatives must be matched on both sides of this equation If ht contains δ1t the first derivative of δt the lefthand side of Eq 211 will contain a term δN1t But the highestorder derivative term on the righthand side is δNt Therefore the two sides cannot match Similar arguments can be made against the presence of the impulses higherorder derivatives in ht 02LathiC02 2017925 1554 page 167 18 23 The Unit Impulse Response ht 167 Comment In the above discussion we have assumed M N as specified by Eq 211 Section 28 shows that the expression for ht applicable to all possible values of M and N is given by ht PDyntut where ynt is a linear combination of the characteristic modes of the system subject to initial conditions Eq 218 This expression reduces to Eq 217 when M N Determination of the impulse response ht using the procedures in this section is relatively simple However in Ch 4 we shall discuss another even simpler method using the Laplace transform As the next example demonstrates it is also possible to find ht using functions from MATLABs symbolic math toolbox EXAMPLE 27 Using MATLAB to Find the Impulse Response Determine the impulse response ht for an LTIC system specified by the differential equation D2 3D 2yt Dxt This is a secondorder system with b0 0 First we find the zeroinput component for initial conditions y0 0 and y0 1 Since PD D the zeroinput response is differentiated and the impulse response immediately follows as ht 0δt Dyntut yn dsolveD2y3Dy2y0y00Dy01t h diffyn h 2exp2t 1expt Therefore ht 2e2t etut DRILL 24 Finding the Impulse Response Determine the unit impulse response of LTIC systems described by the following equations a D 2yt 3D 5xt b DD 2yt D 4xt c D2 2D 1yt Dxt ANSWERS a 3δt e2tut b 2 e2tut c 1 tetut 02LathiC02 2017925 1554 page 175 26 24 System Response to External Input The ZeroState Response 175 DRILL 26 ZeroState Response with Resonance Repeat Drill 25 for the input xt etut ANSWER 6tetut THE CONVOLUTION TABLE The task of convolution is considerably simplified by a readymade convolution table Table 21 This table which lists several pairs of signals and their convolution can conveniently determine yt a system response to an input xt without performing the tedious job of integration For instance we could have readily found the convolution in Ex 28 by using pair 4 with λ1 1 and λ2 2 to be et e2tut The following example demonstrates the utility of this table EXAMPLE 29 Convolution by Tables Use Table 21 to compute the loop current yt of the RLC circuit in Ex 24 for the input xt 10e3tut when all the initial conditions are zero The loop equation for this circuit see Ex 116 or Eq 129 is D2 3D 2yt Dxt The impulse response ht for this system as obtained in Ex 26 is ht 2e2t etut The input is xt 10e3tut and the response yt is yt xt ht 10e3tut 2e2t etut Using the distributive property of the convolution Eq 226 we obtain yt 10e3tut 2e2tut 10e3tut etut 20e3tut e2tut 10e3tut etut Now the use of pair 4 in Table 21 yields yt 20 3 2e3t e2tut 10 3 1e3t etut 20e3t e2tut 5e3t etut 5et 20e2t 15e3tut 02LathiC02 2017925 1554 page 180 31 180 CHAPTER 2 TIMEDOMAIN ANALYSIS OF CONTINUOUSTIME SYSTEMS A similar procedure is followed in computing the value of ct at t t2 where t2 is negative Fig 27g In this case the function gτ is shifted by a negative amount that is leftshifted to obtain gt2τ Multiplication of this function with xτ yields the product xτgt2τ The area under this product is ct2 A2 giving us another point on the curve ct at t t2 Fig 27i This procedure can be repeated for all values of t from to The result will be a curve describing ct for all time t Note that when t 3xτ and gtτ do not overlap see Fig 27h therefore ct 0 for t 3 SUMMARY OF THE GRAPHICAL PROCEDURE The procedure for graphical convolution can be summarized as follows 1 Keep the function xτ fixed 2 Visualize the function gτ as a rigid wire frame and rotate or invert this frame about the vertical axis τ 0 to obtain gτ 3 Shift the inverted frame along the τ axis by t0 seconds The shifted frame now represents gt0 τ 4 The area under the product of xτ and gt0 τ the shifted frame is ct0 the value of the convolution at t t0 5 Repeat this procedure shifting the frame by different values positive and negative to obtain ct for all values of t The graphical procedure discussed here appears very complicated and discouraging at first reading Indeed some people claim that convolution has driven many electrical engineering undergraduates to contemplate theology either for salvation or as an alternative career IEEE Spectrum March 1991 p 60 Actually the bark of convolution is worse than its bite In graphical convolution we need to determine the area under the product xτgt τ for all values of t from to However a mathematical description of xτgt τ is generally valid over a range Convolution Its bark is worse than its bite 02LathiC02 2017925 1554 page 187 38 24 System Response to External Input The ZeroState Response 187 Both Eqs 233 and 234 apply at the transition point t 2 We can readily verify that c2 43 when either of these expressions is used For t 4 xt τ has been shifted so far to the right that it no longer overlaps with gτ as depicted in Fig 210g Consequently ct 0 t 4 We now turn our attention to negative values of t We have already determined ct up to t 1 For t 1 there is no overlap between the two functions as illustrated in Fig 210h so that ct 0 t 1 Combining our results we see that ct 1 6t 12 1 t 1 2 3t 1 t 2 1 6t2 2t 8 2 t 4 0 otherwise Figure 210i plots ct according to this expression THE WIDTH OF CONVOLVED FUNCTIONS The widths durations of xt gt and ct in Ex 212 Fig 210 are 2 3 and 5 respectively Note that the width of ct in this case is the sum of the widths of xt and gt This observation is not a coincidence Using the concept of graphical convolution we can readily see that if xt and gt have the finite widths of T1 and T2 respectively then the width of ct is equal to T1 T2 The reason is that the time it takes for a signal of width duration T1 to completely pass another signal of width duration T2 so that they become nonoverlapping is T1T2 When the two signals become nonoverlapping the convolution goes to zero DRILL 210 Interchanging Convolution Order Rework Ex 211 by evaluating gt xt DRILL 211 Showing Commutability Using Two Causal Signals Use graphical convolution to show that xt gt gt xt ct in Fig 211 02LathiC02 2017925 1554 page 189 40 24 System Response to External Input The ZeroState Response 189 THE PHANTOM OF THE SIGNALS AND SYSTEMS OPERA In the study of signals and systems we often come across some signals such as an impulse which cannot be generated in practice and have never been sighted by anyone One wonders why we even consider such idealized signals The answer should be clear from our discussion so far in this chapter Even if the impulse function has no physical existence we can compute the system response ht to this phantom input according to the procedure in Sec 23 and knowing ht we can compute the system response to any arbitrary input The concept of impulse response therefore provides an effective intermediary for computing system response to an arbitrary input In addition the impulse response ht itself provides a great deal of information and insight about the system behavior In Sec 26 we show that the knowledge of impulse response provides much valuable information such as the response time pulse dispersion and filtering properties of the system Many other useful insights about the system behavior can be obtained by inspection of ht Similarly in frequencydomain analysis discussed in later chapters we use an everlasting exponential or sinusoid to determine system response An everlasting exponential or sinusoid too is a phantom which nobody has ever seen and which has no physical existence But it provides another effective intermediary for computing the system response to an arbitrary input Moreover the system response to everlasting exponential or sinusoid provides valuable information and insight regarding the systems behavior Clearly idealized impulses and everlasting sinusoids are friendly and helpful spirits Interestingly the unit impulse and the everlasting exponential or sinusoid are the dual of each other in the timefrequency duality to be studied in Ch 7 Actually the timedomain and the frequencydomain methods of analysis are the dual of each other WHY CONVOLUTION AN INTUITIVE EXPLANATION OF SYSTEM RESPONSE On the surface it appears rather strange that the response of linear systems those gentlest of the gentle systems should be given by such a tortuous operation of convolution where one signal is fixed and the other is inverted and shifted To understand this odd behavior consider a hypothetical impulse response ht that decays linearly with time Fig 214a This response is strongest at t 0 the moment the impulse is applied and it decays linearly at future instants so that one second later at t 1 and beyond it ceases to exist This means that the closer the impulse input is to an instant t the stronger is its response at t Now consider the input xt shown in Fig 214b To compute the system response we break the input into rectangular pulses and approximate these pulses with impulses Generally the response of a causal system at some instant t will be determined by all the impulse components of the input before t Each of these impulse components will have different weight in determining the response at the instant t depending on its proximity to t As seen earlier the closer the impulse is to t the stronger is its influence at t The impulse at t has the greatest weight unity in determining The late Prof S J Mason the inventor of signal flow graph techniques used to tell a story of a student frustrated with the impulse function The student said The unit impulse is a thing that is so small you cant see it except at one place the origin where it is so big you cant see it In other words you cant see it at all at least I cant 2 02LathiC02 2017925 1554 page 194 45 194 CHAPTER 2 TIMEDOMAIN ANALYSIS OF CONTINUOUSTIME SYSTEMS For a system specified by Eq 22 the transfer function is given by Hs Ps Qs 241 This follows readily by considering an everlasting input xt est According to Eq 238 the output is yt Hsest Substitution of this xt and yt in Eq 22 yields HsQDest PDest Moreover Drest drest dtr srest Hence PDest Psest and QDest Qsest Consequently Hs Ps Qs DRILL 214 Ideal Integrator and Differentiator Transfer Functions Show that the transfer function of an ideal integrator is Hs 1s and that of an ideal differentiator is Hs s Find the answer in two ways using Eq 239 and using Eq 241 Hint Find ht for the ideal integrator and differentiator You also may need to use the result in Prob 1412 A FUNDAMENTAL PROPERTY OF LTI SYSTEMS We can show that Eq 238 is a fundamental property of LTI systems and it follows directly as a consequence of linearity and time invariance To show this let us assume that the response of an LTI system to an everlasting exponential est is yst If we define Hst yst est then yst Hstest Because of the timeinvariance property the system response to input estT is Hst TestT that is yst T Hst TestT 242 The delayed input estT represents the input est multiplied by a constant esT Hence according to the linearity property the system response to estT must be ystesT Hence yst T ystesT HstestT 02LathiC02 2017925 1554 page 199 50 25 System Stability 199 a b c d e f g h Characteristic root location Characteristic root location Zeroinput response Zeroinput response t t t t t t t t 0 0 0 0 0 0 0 0 0 0 0 0 0 0 0 0 Figure 217 Location of characteristic roots and the corresponding characteristic modes 253 Relationship Between BIBO and Asymptotic Stability External stability is determined by applying an external input with zero initial conditions while internal stability is determined by applying the nonzero initial conditions and no external input This is why these stabilities are also called the zerostate stability and the zeroinput stability respectively Recall that ht the impulse response of an LTIC system is a linear combination of the system characteristic modes For an LTIC system specified by Eq 21 we can readily show that when a characteristic root λk is in the LHP the corresponding mode eλkt is absolutely integrable In 02LathiC02 2017925 1554 page 202 53 202 CHAPTER 2 TIMEDOMAIN ANALYSIS OF CONTINUOUSTIME SYSTEMS The characteristic polynomials of these systems are a λ 1λ2 4λ 8 λ 1λ 2 j2λ 2 j2 b λ 1λ2 4λ 8 λ 1λ 2 j2λ 2 j2 c λ 2λ2 4 λ 2λ j2λ j2 d λ 1λ2 42 λ 2λ j22λ j22 Consequently the characteristic roots of the systems are see Fig 220 a 1 2 j2 b 1 2 j2 c 2 j2 d 1 j2 j2 System a is asymptotically stable all roots in LHP system b is unstable one root in RHP system c is marginally stable unrepeated roots on imaginary axis and no roots in RHP and system d is unstable repeated roots on the imaginary axis BIBO stability is readily determined from the asymptotic stability System a is BIBOstable system b is BIBOunstable system c is BIBOunstable and system d is BIBOunstable We have assumed that these systems are controllable and observable d a 0 c 0 0 b 0 Figure 220 Characteristic root locations for the systems of Ex 214 DRILL 215 Assessing Stability by Characteristic Roots For each case plot the characteristic roots and determine asymptotic and BIBO stabilities Assume the equations reflect internal descriptions a DD 2yt 3xt b D2D 3yt D 5xt c D 1D 2yt 2D 3xt d D2 1D2 9yt D2 2D 4xt e D 1D2 4D 9yt D 7xt 02LathiC02 2017925 1554 page 204 55 204 CHAPTER 2 TIMEDOMAIN ANALYSIS OF CONTINUOUSTIME SYSTEMS ground however the plant that springs from it is totally determined by the seed The imprint of the seed exists on every cell of the plant To understand this interesting phenomenon recall that the characteristic modes of a system are very special to that system because it can sustain these signals without the application of an external input In other words the system offers a free ride and ready access to these signals Now imagine what would happen if we actually drove the system with an input having the form of a characteristic mode We would expect the system to respond strongly this is in fact the resonance phenomenon discussed later in this section If the input is not exactly a characteristic mode but is close to such a mode we would still expect the system response to be strong However if the input is very different from any of the characteristic modes we would expect the system to respond poorly We shall now show that these intuitive deductions are indeed true Intuition can cut the math jungle instantly We have devised a measure of similarity of signals later see in Ch 6 Here we shall take a simpler approach Let us restrict the systems inputs to exponentials of the form eζt where ζ is generally a complex number The similarity of two exponential signals eζt and eλt will then be measured by the closeness of ζ and λ If the difference ζ λ is small the signals are similar if ζ λ is large the signals are dissimilar Now consider a firstorder system with a single characteristic mode eλt and the input eζt The impulse response of this system is then given by Aeλt where the exact value of A is not important for this qualitative discussion The system response yt is given by yt ht xt Aeλtut eζtut From the convolution table Table 21 we obtain yt A ζ λeζt eλtut 246 02LathiC02 2017925 1554 page 208 59 208 CHAPTER 2 TIMEDOMAIN ANALYSIS OF CONTINUOUSTIME SYSTEMS with a time constant Th acts as a lowpass filter having a cutoff frequency of fc 1Th hertz so that sinusoids with frequencies below fc Hz are transmitted reasonably well while those with frequencies above fc Hz are suppressed To demonstrate this fact let us determine the system response to a sinusoidal input xt by convolving this input with the effective impulse response ht in Fig 223a From Figs 223b and 223c we see the process of convolution of ht with the sinusoidal inputs of two different frequencies The sinusoid in Fig 223b has a relatively high frequency while the frequency of the sinusoid in Fig 223c is low Recall that the convolution of xt and ht is equal to the area under the product xτht τ This area is shown shaded in Figs 223b and 223c for the two cases For the highfrequency sinusoid it is clear from Fig 223b that the area under xτht τ is very small because its positive and negative areas nearly cancel each other out In this case the output yt remains periodic but has a rather small amplitude This happens when the period of the sinusoid is much smaller than the system time constant Th In contrast for the lowfrequency sinusoid the period of the sinusoid is larger than Th rendering the partial cancellation of area under xτht τ less effective Consequently the output yt is much larger as depicted in Fig 223c Between these two possible extremes in system behavior a transition point occurs when the period of the sinusoid is equal to the system time constant Th The frequency at which this transition occurs is known as the cutoff frequency fc of the system Because Th is the period of cutoff frequency fc fc 1 Th The frequency fc is also known as the bandwidth of the system because the system transmits or passes sinusoidal components with frequencies below fc while attenuating components with frequencies above fc Of course the transition in system behavior is gradual There is no dramatic change in system behavior at fc 1Th Moreover these results are based on an idealized rectangular pulse impulse response in practice these results will vary somewhat depending on the exact shape of ht Remember that the feel of general system behavior is more important than exact system response for this qualitative discussion Since the system time constant is equal to its rise time we have Tr 1 fc or fc 1 Tr 248 Thus a systems bandwidth is inversely proportional to its rise time Although Eq 248 was derived for an idealized rectangular impulse response its implications are valid for lowpass LTIC systems in general For a general case we can show that 1 fc k Tr where the exact value of k depends on the nature of ht An experienced engineer often can estimate quickly the bandwidth of an unknown system by simply observing the system response to a step input on an oscilloscope 02LathiC02 2017925 1554 page 209 60 26 Intuitive Insights into System Behavior 209 265 Time Constant and Pulse Dispersion Spreading In general the transmission of a pulse through a system causes pulse dispersion or spreading Therefore the output pulse is generally wider than the input pulse This system behavior can have serious consequences in communication systems in which information is transmitted by pulse amplitudes Dispersion or spreading causes interference or overlap with neighboring pulses thereby distorting pulse amplitudes and introducing errors in the received information Earlier we saw that if an input xt is a pulse of width Tx then Ty the width of the output yt is Ty Tx Th This result shows that an input pulse spreads out disperses as it passes through a system Since Th is also the systems time constant or rise time the amount of spread in the pulse is equal to the time constant or rise time of the system 266 Time Constant and Rate of Information Transmission In pulse communications systems which convey information through pulse amplitudes the rate of information transmission is proportional to the rate of pulse transmission We shall demonstrate that to avoid the destruction of information caused by dispersion of pulses during their transmission through the channel transmission medium the rate of information transmission should not exceed the bandwidth of the communications channel Since an input pulse spreads out by Th seconds the consecutive pulses should be spaced Th seconds apart to avoid interference between pulses Thus the rate of pulse transmission should not exceed 1Th pulsessecond But 1Th fc the channels bandwidth so that we can transmit pulses through a communications channel at a rate of fc pulses per second and still avoid significant interference between the pulses The rate of information transmission is therefore proportional to the channels bandwidth or to the reciprocal of its time constant The discussion of Secs 262 263 264 265 and 266 shows that the system time constant determines much of a systems behaviorits filtering characteristics rise time pulse dispersion and so on In turn the time constant is determined by the systems characteristic roots Clearly the characteristic roots and their relative amounts in the impulse response ht determine the behavior of a system EXAMPLE 215 Intuitive Insights into Lowpass System Behavior Find the time constant Th rise time Tr and cutoff frequency fc for a lowpass system that has impulse response ht tetut Determine the maximum rate that pulses of 1 second Theoretically a channel of bandwidth fc can transmit correctly up to 2fc pulse amplitudes per second 4 Our derivation here being very simple and qualitative yields only half the theoretical limit In practice it is not easy to attain the upper theoretical limit 02LathiC02 2017925 1554 page 212 63 212 CHAPTER 2 TIMEDOMAIN ANALYSIS OF CONTINUOUSTIME SYSTEMS is a characteristic mode But even in an asymptotically stable system we see a manifestation of resonance if its characteristic roots are close to the imaginary axis so that Re λ is a small negative value We can show that when the characteristic roots of a system are σ jω0 then the system response to the input ejω0t or the sinusoid cosω0t is very large for small σ The system response drops off rapidly as the input signal frequency moves away from ω0 This frequencyselective behavior can be studied more profitably after an understanding of frequencydomain analysis has been acquired For this reason we postpone full discussion of this subject until Ch 4 IMPORTANCE OF THE RESONANCE PHENOMENON The resonance phenomenon is very important because it allows us to design frequencyselective systems by choosing their characteristic roots properly Lowpass bandpass highpass and bandstop filters are all examples of frequencyselective networks In mechanical systems the inadvertent presence of resonance can cause signals of such tremendous magnitude that the system may fall apart A musical note periodic vibrations of proper frequency can shatter glass if the frequency is matched to the characteristic root of the glass which acts as a mechanical system Similarly a company of soldiers marching in step across a bridge amounts to applying a periodic force to the bridge If the frequency of this input force happens to be nearer to a characteristic root of the bridge the bridge may respond vibrate violently and collapse even though it would have been strong enough to carry many soldiers marching out of step A case in point is the Tacoma Narrows Bridge failure of 1940 This bridge was opened to traffic in July 1940 Within four months of opening on November 7 1940 it collapsed in a mild gale not because of the winds brute force but because the frequencies of windgenerated vortices which matched the natural frequencies characteristic roots of the bridge caused resonance Because of the great damage that may occur mechanical resonance is generally to be avoided especially in structures or vibrating mechanisms If an engine with periodic force such as piston motion is mounted on a platform the platform with its mass and springs should be designed so that their characteristic roots are not close to the engines frequency of vibration Proper design of this platform can not only avoid resonance but also attenuate vibrations if the system roots are placed far away from the frequency of vibration 27 MATLAB MFILES Mfiles are stored sequences of MATLAB commands and help simplify complicated tasks There are two types of Mfile script and function Both types are simple text files and require a m filename extension Although Mfiles can be created by using any text editor MATLABs builtin editor is the preferable choice because of its special features As with any program comments improve the readability of an Mfile Comments begin with the character and continue through the end of the line An Mfile is executed by simply typing the filename without the m extension To execute Mfiles need to be located in the current directory or any other directory in the MATLAB path New directories are easily added to the MATLAB path by using the addpath command This follows directly from Eq 249 with λ σ jω0 and ϵ σ 02LathiC02 2017925 1554 page 214 65 214 CHAPTER 2 TIMEDOMAIN ANALYSIS OF CONTINUOUSTIME SYSTEMS CH2MP1m Chapter 2 MATLAB Program 1 Script Mfile determines characteristic roots of opamp circuit Set component values R 1e4 1e4 1e4 C 1e6 1e6 Determine coefficients for characteristic equation A 1 1R11R21R3C2 1R1R2C1C2 Determine characteristic roots lambda rootsA A script file is created by placing these commands in a text file which in this case is named CH2MP1m While comment lines improve program clarity their removal does not affect program functionality The program is executed by typing CH2MP1 After execution all the resulting variables are available in the workspace For example to view the characteristic roots type lambda lambda 2618034 381966 Thus the characteristic modes are simple decaying exponentials e2618034t and e381966t Script files permit simple or incremental changes thereby saving significant effort Consider what happens when capacitor C1 is changed from 10 µF to 10 nF Changing CH2MP1m so that C 1e9 1e6 allows computation of the new characteristic roots CH2MP1 lambda lambda 10e003 01500 31587i 01500 31587i Perhaps surprisingly the characteristic modes are now complex exponentials capable of supporting oscillations The imaginary portion of λ dictates an oscillation rate of 31587 rads or about 503 Hz The real portion dictates the rate of decay The time expected to reduce the amplitude to 25 is approximately t ln025Reλ 001 second 272 Function MFiles It is inconvenient to modify and save a script file each time a change of parameters is desired Function Mfiles provide a sensible alternative Unlike script Mfiles function Mfiles can accept input arguments as well as return outputs Functions truly extend the MATLAB language in ways that script files cannot 02LathiC02 2017925 1554 page 217 68 27 MATLAB MFiles 217 240 220 200 180 160 140 120 100 Real 5000 0 5000 Imaginary Char Roots Min Val Roots Max Val Roots Figure 226 Effect of component values on characteristic root locations The command lambda zeros2243 preallocates a 2243 array to store the computed roots When necessary MATLAB performs dynamic memory allocation so this command is not strictly necessary However preallocation significantly improves script execution speed Notice also that it would be nearly useless to call script CH2MP1 from within the nested loop script file parameters cannot be changed during execution The plot instruction is quite long Long commands can be broken across several lines by terminating intermediate lines with three dots The three dots tell MATLAB to continue the present command to the next line Black xs locate roots of each permutation The command lambda vectorizes the 2 243 matrix lambda into a 486 1 vector This is necessary in this case to ensure that a proper legend is generated Because of loop order permutation p 1 corresponds to the case of all components at the smallest values and permutation p 243 corresponds to the case of all components at the largest values This information is used to separately highlight the minimum and maximum cases using downtriangles and uptriangles respectively In addition to terminating each for loop end is used to indicate the final index along a particular dimension which eliminates the need to remember the particular size of a variable An overloaded function such as end serves multiple uses and is typically interpreted based on context The graphical results provided by CH2MP3 are shown in Fig 226 Between extremes root oscillations vary from 365 to 745 Hz and decay times to 25 amplitude vary from 62 to 127 ms Clearly this circuits behavior is quite sensitive to ordinary component variations 274 Graphical Understanding of Convolution MATLAB graphics effectively illustrate the convolution process Consider the case of yt xt ht where xt 15sinπtut ut 1 and ht 15ut ut 15 ut 2 ut 25 Program CH2MP4 steps through the convolution over the time interval 025 t 375 02LathiC02 2017925 1554 page 218 69 218 CHAPTER 2 TIMEDOMAIN ANALYSIS OF CONTINUOUSTIME SYSTEMS CH2MP4m Chapter 2 MATLAB Program 4 Script Mfile graphically demonstrates the convolution process figure1 Create figure window and make visible on screen u t 10t0 x t 15sinpitutut1 h t 15utut15ut2ut25 dtau 0005 tau 1dtau4 ti 0 tvec 251375 y NaNzeros1lengthtvec Preallocate memory for t tvec ti ti1 Time index xh xttauhtau lxh lengthxh yti sumxhdtau Trapezoidal approximation of convolution integral subplot211plottauhtauktauxttaukt0ok axistau1 tauend 20 25 patchtau1end1tau1end1tau2endtau2end zeros1lxh1xh1end1xh2endzeros1lxh1 8 8 8edgecolornone xlabel au titleh au solid xt au dashed h auxt au gray c getgcachildren setgcachildrenc2c3c4c1 subplot212plottvecyktvectiytiok xlabelt ylabelyt int h auxt au d au axistau1 tauend 10 20 grid drawnow end At each step the program plots hτ xt τ and shades the area hτxt τ gray This gray area which reflects the integral of hτxt τ is also the desired result yt Figures 227 228 and 229 display the convolution process at times t of 075 225 and 285 seconds respectively These figures help illustrate how the regions of integration change with time Figure 227 has limits of integration from 0 to t 075 Figure 228 has two regions of integration with limits t 1 125 to 15 and 20 to t 225 The last plot Fig 229 has limits from 20 to 25 Several comments regarding CH2MP4 are in order The command figure1 opens the first figure window and more important makes sure it is visible Anonymous functions are used to represent the functions ut xt and ht NaN standing for notanumber usually results from operations such as 00 or MATLAB refuses to plot NaN values so preallocating yt with NaNs ensures that MATLAB displays only values of yt that have been computed As its name suggests length returns the length of the input vector The subplotabc command partitions the current figure window into an abyb matrix of axes and selects axes c for use Subplots facilitate graphical comparison by allowing multiple axes in a single figure window The patch command is used to create the grayshaded area for hτxt τ In CH2MP4 the get and set commands are used to reorder plot objects so that the gray area does not obscure other lines Details of the patch get and set commands as used in CH2MP4 are somewhat advanced and are not pursued here MATLAB also prints most Greek letters if the Greek name is preceded by a backslash character For example au in the xlabel command produces the symbol τ in the plots axis label Similarly an integral sign is produced by int Finally the drawnow Interested students should consult the MATLAB help facilities for further information Actually the get and set commands are extremely powerful and can help modify plots in almost any conceivable way 02LathiC02 2017925 1554 page 219 70 28 MATLAB MFiles 219 1 05 0 05 1 15 2 25 3 35 4 2 0 2 hτ solid xtτ dashed hτtτ gray 1 05 0 05 1 15 2 25 3 35 4 t τ 1 0 1 2 yt hτtτ dτ Figure 227 Graphical convolution at step t 075 second 2 0 2 1 05 0 05 1 15 2 25 3 35 4 t 1 05 0 05 1 15 2 25 3 35 4 τ 1 0 1 2 hτ solid xtτ dashed hτtτ gray yt hτtτ dτ Figure 228 Graphical convolution at step t 225 seconds command forces MATLAB to update the graphics window for each loop iteration Although slow this creates an animationlike effect Replacing drawnow with the pause command allows users to manually step through the convolution process The pause command still forces the graphics window to update but the program will not continue until a key is pressed 02LathiC02 2017925 1554 page 220 71 220 CHAPTER 2 TIMEDOMAIN ANALYSIS OF CONTINUOUSTIME SYSTEMS 1 05 0 05 1 15 2 25 3 35 4 2 0 2 1 05 0 05 1 15 2 25 3 35 4 t τ 1 0 1 2 hτ solid xtτ dashed hτtτ gray yt hτtτ dτ Figure 229 Graphical convolution at step t 285 seconds 28 APPENDIX DETERMINING THE IMPULSE RESPONSE In Eq 213 we showed that for an LTIC system S specified by Eq 211 the unit impulse response ht can be expressed as ht b0δt characteristic modes 251 To determine the characteristic mode terms in Eq 251 let us consider a system S0 whose input xt and the corresponding output wt are related by QDwt xt 252 Observe that both the systems S and S0 have the same characteristic polynomial namely Qλ and consequently the same characteristic modes Moreover S0 is the same as S with PD 1 that is b0 0 Therefore according to Eq 251 the impulse response of S0 consists of characteristic mode terms only without an impulse at t 0 Let us denote this impulse response of S0 by ynt Observe that ynt consists of characteristic modes of S and therefore may be viewed as a zeroinput response of S Now ynt is the response of S0 to input δt Therefore according to Eq 252 QDynt δt or DN a1DN1 aN1D aNynt δt or yN n t a1yN1 n t aN1y1 n t aNynt δt 02LathiC02 2017925 1554 page 221 72 29 Summary 221 where yk n t represents the kth derivative of ynt The righthand side contains a single impulse term δt This is possible only if yN1 n t has a unit jump discontinuity at t 0 so that yN n t δt Moreover the lowerorder terms cannot have any jump discontinuity because this would mean the presence of the derivatives of δt Therefore yn0 y1 n 0 yN2 n 0 0 no discontinuity at t 0 and the N initial conditions on ynt are yn0 y1 n 0 yN2 n 0 0 and yN1 n 0 1 253 This discussion means that ynt is the zeroinput response of the system S subject to initial conditions Eq 253 We now show that for the same input xt to both systems S and S0 their respective outputs yt and wt are related by yt PDwt 254 To prove this result we operate on both sides of Eq 252 by PD to obtain QDPDwt PDxt Comparison of this equation with Eq 22 leads immediately to Eq 254 Now if the input xt δt the output of S0 is ynt and the output of S according to Eq 254 is PDynt This output is ht the unit impulse response of S Note however that because it is an impulse response of a causal system S0 the function ynt is causal To incorporate this fact we must represent this function as yntut Now it follows that ht the unit impulse response of the system S is given by ht PDyntut 255 where ynt is a linear combination of the characteristic modes of the system subject to initial conditions 253 The righthand side of Eq 255 is a linear combination of the derivatives of yntut Evaluating these derivatives is clumsy and inconvenient because of the presence of ut The derivatives will generate an impulse and its derivatives at the origin Fortunately when M N Eq 211 we can avoid this difficulty by using the observation in Eq 251 which asserts that at t 0 the origin ht b0δt Therefore we need not bother to find ht at the origin This simplification means that instead of deriving PDyntut we can derive PDynt and add to it the term b0δt so that ht b0δt PDynt t 0 b0δt PDyntut This expression is valid when M N the form given in Eq 211 When M N Eq 255 should be used 29 SUMMARY This chapter discusses timedomain analysis of LTIC systems The total response of a linear system is a sum of the zeroinput response and zerostate response The zeroinput response is the system 02LathiC02 2017925 1554 page 222 73 222 CHAPTER 2 TIMEDOMAIN ANALYSIS OF CONTINUOUSTIME SYSTEMS response generated only by the internal conditions initial conditions of the system assuming that the external input is zero hence the adjective zeroinput The zerostate response is the system response generated by the external input assuming that all initial conditions are zero that is when the system is in zero state Every system can sustain certain forms of response on its own with no external input zero input These forms are intrinsic characteristics of the system that is they do not depend on any external input For this reason they are called characteristic modes of the system Needless to say the zeroinput response is made up of characteristic modes chosen in a combination required to satisfy the initial conditions of the system For an Nthorder system there are N distinct modes The unit impulse function is an idealized mathematical model of a signal that cannot be generated in practice Nevertheless introduction of such a signal as an intermediary is very helpful in analysis of signals and systems The unit impulse response of a system is a combination of the characteristic modes of the system because the impulse δt 0 for t 0 Therefore the system response for t 0 must necessarily be a zeroinput response which as seen earlier is a combination of characteristic modes The zerostate response response due to external input of a linear system can be obtained by breaking the input into simpler components and then adding the responses to all the components In this chapter we represent an arbitrary input xt as a sum of narrow rectangular pulses staircase approximation of xt In the limit as the pulse width 0 the rectangular pulse components approach impulses Knowing the impulse response of the system we can find the system response to all the impulse components and add them to yield the system response to the input xt The sum of the responses to the impulse components is in the form of an integral known as the convolution integral The system response is obtained as the convolution of the input xt with the systems impulse response ht Therefore the knowledge of the systems impulse response allows us to determine the system response to any arbitrary input LTIC systems have a very special relationship to the everlasting exponential signal est because the response of an LTIC system to such an input signal is the same signal within a multiplicative constant The response of an LTIC system to the everlasting exponential input est is Hsest where Hs is the transfer function of the system If every bounded input results in a bounded output the system is stable in the boundedinputboundedoutput BIBO sense An LTIC system is BIBOstable if and only if its impulse response is absolutely integrable Otherwise it is BIBOunstable BIBO stability is a stability seen from external terminals of the system Hence it is also called external stability or zerostate stability In contrast internal stability or the zeroinput stability examines the system stability from inside When some initial conditions are applied to a system in zero state then if the system eventually returns to zero state the system is said to be stable in the asymptotic or Lyapunov sense If the systems response increases without bound it is unstable If the system does not go to zero state and the response does not increase indefinitely the system is marginally stable The internal stability criterion in terms of the location of a systems characteristic roots can be summarized as follows However it can be closely approximated by a narrow pulse of unit area and having a width that is much smaller than the time constant of an LTIC system in which it is used There is the possibility of an impulse in addition to the characteristic modes 02LathiC02 2017925 1554 page 223 74 Problems 223 1 An LTIC system is asymptotically stable if and only if all the characteristic roots are in the LHP The roots may be repeated or unrepeated 2 An LTIC system is unstable if and only if either one or both of the following conditions exist i at least one root is in the RHP ii there are repeated roots on the imaginary axis 3 An LTIC system is marginally stable if and only if there are no roots in the RHP and there are some unrepeated roots on the imaginary axis It is possible for a system to be externally BIBO stable but internally unstable When a system is controllable and observable its external and internal descriptions are equivalent Hence external BIBO and internal asymptotic stabilities are equivalent and provide the same information Such a BIBOstable system is also asymptotically stable and vice versa Similarly a BIBOunstable system is either marginally stable or asymptotically unstable system The characteristic behavior of a system is extremely important because it determines not only the system response to internal conditions zeroinput behavior but also the system response to external inputs zerostate behavior and the system stability The system response to external inputs is determined by the impulse response which itself is made up of characteristic modes The width of the impulse response is called the time constant of the system which indicates how fast the system can respond to an input The time constant plays an important role in determining such diverse system behaviors as the response time and filtering properties of the system dispersion of pulses and the rate of pulse transmission through the system REFERENCES 1 Lathi B P Signals and Systems BerkeleyCambridge Press Carmichael CA 1987 2 Mason S J Electronic Circuits Signals and Systems Wiley New York 1960 3 Kailath T Linear System PrenticeHall Englewood Cliffs NJ 1980 4 Lathi B P Modern Digital and Analog Communication Systems 3rd ed Oxford University Press New York 1998 PROBLEMS 221 Determine the constants c1 c2 λ1 and λ2 for each of the following secondorder systems which have zeroinput responses of the form yzirt c1eλ1t c2eλ2t a yt 2yt 5yt xt 5xt with yzir0 2 and yzir0 0 b yt 2yt 5yt xt 5xt with yzir0 4 and yzir0 1 c d2 dt2 yt 2 d dtyt xt with yzir0 1 and yzir0 2 d D2 2D10yt D5 Dxt with yzir0 yzir0 1 e D2 7 2D 3 2yt D 2xt with yzir0 3 and yzir0 8 Caution The second IC is given in terms of the second derivative not the first derivative f 13yt 4 d dtyt d2 dt2 yt 2xt 4 d dtxt with yzir0 3 and yzir0 15 Caution The second IC is given in terms of the second derivative not the first derivative 222 Consider a linear timeinvariant system with input xt and output yt that is described by the differential equation D 1D2 1yt D5 1xt Furthermore assume y0 y0 y0 1 02LathiC02 2017925 1554 page 227 78 Problems 227 2410 If xt gt ct then show that xat gat 1acat This timescaling property of convolution states that if both xt and gt are timescaled by a their convolution is also timescaled by a and multiplied by 1a ht t 0 2 1 1 2 3 4 5 1 Figure P248 2411 Show that the convolution of an odd and an even function is an odd function and the convolution of two odd or two even functions is an even function Hint Use the timescaling property of convolution in Prob 2410 2412 Suppose an LTIC system has impulse response ht 1 tut ut 1 and input xt ut 1ut 1 Use the graphical convolu tion procedure to determine yzsrt xt ht Accurately sketch yzsrt When solving for yzsrt flip and shift ht explicitly show all integration steps and simplify your answer 2413 Using direct integration find eatut ebtut 2414 Using direct integration find ut ut eatut eatut and tut ut 2415 Using direct integration find sin tut ut and cos tut ut 2416 The unit impulse response of an LTIC system is ht etut Find this systems zerostate response yt if the input xt is a ut b etut c e2tut d sin3tut Use the convolution table Table 21 to find your answers 2417 Repeat Prob 2416 for ht 2e3t e2tut and if the input xt is a ut b etut c e2tut 2418 Repeat Prob 2416 for ht 1 2te2tut and input xt ut 2419 Repeat Prob 2416 for ht 4e2t cos3tut and each of the following inputs xt a ut b etut 2420 Repeat Prob 2416 for ht etut and each of the following inputs xt a e2tut b e2t3ut c e2tut 3 d The gate pulse depicted in Fig P2420and provide a sketch of yt xt 0 t 1 1 Figure P2420 2421 A firstorder allpass filter impulse response is given by ht δt 2etut a Find the zerostate response of this filter for the input etut b Sketch the input and the corresponding zerostate response 2422 Figure P2422 shows the input xt and the impulse response ht for an LTIC system Let the output be yt a By inspection of xt and ht find y1y0y1y2y3y4y5 and 02LathiC02 2017925 1554 page 230 81 230 CHAPTER 2 TIMEDOMAIN ANALYSIS OF CONTINUOUSTIME SYSTEMS ht t 1 1 1 t 1 1 1 2 xt Figure P2430 t 1 1 1 2 ht xt yt ht ht Figure P2431 xt yt C L Figure P2432 a b h1 h2 xt ypt xt h1 h2 yst Figure P2433 2433 Two LTIC systems have impulse response functions given by h1t 1tutut1 and h2t tut 2 ut 2 a Carefully sketch the functions h1t and h2t b Assume that the two systems are connected in parallel as shown in Fig P2433a Carefully plot the equivalent impulse response function hpt c Assume that the two systems are connected in cascade as shown in Fig P2433b Carefully plot the equivalent impulse response function hst 2434 Consider the circuit shown in Fig P2434 a Find the output yt given an initial capacitor voltage of y0 2 volts and an input xt ut b Given an input xt ut 1 determine the initial capacitor voltage y0 so that the output yt is 05 volt at t 2 seconds xt yt C R Figure P2434 03LathiC03 2017925 1554 page 237 1 C H A P T E R TIMEDOMAIN ANALYSIS OF DISCRETETIME SYSTEMS 3 In this chapter we introduce the basic concepts of discretetime signals and systems Furthermore we explore the timedomain analysis of linear timeinvariant discretetime LTID systems We show how to compute the zeroinput response determine the unit impulse response and use convolution to evaluate the zerostate response 31 INTRODUCTION A discretetime signal is basically a sequence of numbers Such signals arise naturally in inherently discretetime situations such as population studies amortization problems national income models and radar tracking They may also arise as a result of sampling continuoustime signals in sampled data systems and digital filtering Such signals can be denoted by xn yn and so on where the variable n takes integer values and xn denotes the nth number in the sequence labeled x In this notation the discretetime variable n is enclosed in square brackets instead of parentheses which we have reserved for enclosing continuoustime variables such as t Systems whose inputs and outputs are discretetime signals are called discretetime systems A digital computer is a familiar example of this type of system A discretetime signal is a sequence of numbers and a discretetime system processes a sequence of numbers xn to yield another sequence yn as the output A discretetime signal when obtained by uniform sampling of a continuoustime signal xt can also be expressed as xnT where T is the sampling interval and n the discrete variable taking on integer values Thus xnT denotes the value of the signal xt at t nT The signal xnT is a sequence of numbers sample values and hence by definition is a discretetime signal Such a signal can also be denoted by the customary discretetime notation xn where xn xnT A typical discretetime signal is depicted in Fig 31 which shows both forms of notation By way of an example a continuoustime exponential xt et when sampled every T 01 seconds results in a discretetime signal xnT given by xnT enT e01n There may be more than one input and more than one output 237 03LathiC03 2017925 1554 page 242 6 242 CHAPTER 3 TIMEDOMAIN ANALYSIS OF DISCRETETIME SYSTEMS DRILL 33 RightShift Operation Show that xk n can be obtained from xn by first rightshifting xn by k units and then timereversing this shifted signal TIME REVERSAL To timereverse xn in Fig 34a we rotate xn about the vertical axis to obtain the timereversed signal xrn shown in Fig 34c Using the argument employed for a similar operation in continuoustime signals Sec 12 we obtain xrn xn Therefore to timereverse a signal we replace n with n so that xn is the timereversed xn For example if xn 09n for 3 n 10 then xrn 09n for 3 n 10 that is 3 n 10 as shown in Fig 34c The origin n 0 is the anchor point which remains unchanged under timereversal operation because at n 0 xn xn x0 Note that while the reversal of xn about the vertical axis is xn the reversal of xn about the horizontal axis is xn EXAMPLE 32 Time Reversal and Shifting In the convolution operation discussed later we need to find the function xk n from xn This can be done in two steps i timereverse the signal xn to obtain xn ii now rightshift xn by k Recall that rightshifting is accomplished by replacing n with n k Hence rightshifting xn by k units is xn k xk n Figure 34d shows x5 n obtained this way We first timereverse xn to obtain xn in Fig 34c Next we shift xn by k 5 to obtain xk n x5 n as shown in Fig 34d In this particular example the order of the two operations employed is interchangeable We can first leftshift xk to obtain xn 5 Next we timereverse xn 5 to obtain xn 5 x5 n The reader is encouraged to verify that this procedure yields the same result as in Fig 34d DRILL 34 Time Reversal Sketch the signal xn e05n for 3 n 2 and zero otherwise Sketch the corresponding timereversed signal and show that it can be expressed as xrn e05n for 2 n 3 03LathiC03 2017925 1554 page 250 14 250 CHAPTER 3 TIMEDOMAIN ANALYSIS OF DISCRETETIME SYSTEMS Accurately handsketching DT signals can be tedious and difficult As the next example shows MATLAB is particularly well suited to plot DT signals including exponentials EXAMPLE 34 Plotting DT Exponentials with MATLAB Use MATLAB to plot the following discretetime signals over 0 n 8 a xan 08n b xbn 08n c xcn 05n and d xdn 11n To begin we use anonymous functions to represent each of the four signals Next we plot these functions over the desired range of n The results shown in Fig 310 match the earlier Fig 39 plots of the same signals n 08 xa n 08n xb n 08n xc n 05n xd n 11n subplot221 stemnxank ylabelxan xlabeln subplot222 stemnxbnk ylabelxbn xlabeln subplot223 stemnxcnk ylabelxcn xlabeln subplot224 stemnxdnk ylabelxdn xlabeln 0 05 1 xan 1 0 1 xbn 0 2 4 6 8 0 2 4 6 8 n 0 05 1 xcn n 0 2 4 6 8 0 2 4 6 8 n n 0 1 2 3 xdn Figure 310 DT plots for Ex 34 03LathiC03 2017925 1554 page 253 17 34 Examples of DiscreteTime Systems 253 30 20 10 0 10 20 30 n 1 0 1 xn Figure 312 Sinusoid plot for Ex 35 34 EXAMPLES OF DISCRETETIME SYSTEMS We shall give here four examples of discretetime systems In the first two examples the signals are inherently of the discretetime variety In the third and fourth examples a continuoustime signal is processed by a discretetime system as illustrated in Fig 32 by discretizing the signal through sampling EXAMPLE 36 Savings Account A person makes a deposit the input in a bank regularly at an interval of T say 1 month The bank pays a certain interest on the account balance during the period T and mails out a periodic statement of the account balance the output to the depositor Find the equation relating the output yn the balance to the input xn the deposit In this case the signals are inherently discrete time Let xn deposit made at the nth discrete instant yn account balance at the nth instant computed immediately after receipt of the nth deposit xn r interest per dollar per period T The balance yn is the sum of i the previous balance yn 1 ii the interest on yn 1 during the period T and iii the deposit xn yn yn 1 ryn 1 xn 1 ryn 1 xn or yn ayn 1 xn a 1 r 33 In this example the deposit xn is the input cause and the balance yn is the output effect 03LathiC03 2017925 1554 page 260 24 260 CHAPTER 3 TIMEDOMAIN ANALYSIS OF DISCRETETIME SYSTEMS KINSHIP OF DIFFERENCE EQUATIONS TO DIFFERENTIAL EQUATIONS We now show that a digitized version of a differential equation results in a difference equation Let us consider a simple firstorder differential equation dyt dt cyt xt 312 Consider uniform samples of xt at intervals of T seconds As usual we use the notation xn to denote xnT the nth sample of xt Similarly yn denotes ynT the nth sample of yt From the basic definition of a derivative we can express Eq 312 at t nT as lim T0 yn yn 1 T cyn xn Clearing the fractions and rearranging the terms yield assuming nonzero but very small T yn αyn 1 βxn 313 where α 1 1 cT and β T 1 cT We can also express Eq 313 in advance form as yn 1 αyn βxn 1 It is clear that a differential equation can be approximated by a difference equation of the same order In this way we can approximate an nthorder differential equation by a difference equation of nth order Indeed a digital computer solves differential equations by using an equivalent difference equation which can be solved by means of simple operations of addition multiplication and shifting Recall that a computer can perform only these simple operations It must necessarily approximate complex operation like differentiation and integration in terms of such simple operations The approximation can be made as close to the exact answer as possible by choosing sufficiently small value for T At this stage we have not developed tools required to choose a suitable value of the sampling interval T This subject is discussed in Ch 5 and also in Ch 8 In Sec 57 we shall discuss a systematic procedure impulse invariance method for finding a discretetime system with which to realize an Nthorder LTIC system ORDER OF A DIFFERENCE EQUATION Equations 33 35 39 311 and 313 are examples of difference equations The highestorder difference of the output signal or the input signal whichever is higher represents the order of the difference equation Hence Eqs 33 39 311 and 313 are firstorder difference equations whereas Eq 35 is of the second order 03LathiC03 2017925 1554 page 261 25 34 Examples of DiscreteTime Systems 261 DRILL 38 Digital Integrator Design Design a digital integrator in Ex 39 using the fact that for an integrator the output yt and the input xt are related by dytdt xt Approximation similar to that in Ex 38 of this equation at t nT yields the recursive form in Eq 311 ANALOG DIGITAL CONTINUOUSTIME AND DISCRETETIME SYSTEMS The basic difference between continuoustime systems and analog systems as also between discretetime and digital systems is fully explained in Secs 175 and 176 Historically discretetime systems have been realized with digital computers where continuoustime signals are processed through digitized samples rather than unquantized samples Therefore the terms digital filters and discretetime systems are used synonymously in the literature This distinction is irrelevant in the analysis of discretetime systems For this reason we follow this loose convention in this book where the term digital filter implies a discretetime system and analog filter means continuoustime system Moreover the terms CD continuoustodiscretetime and DC will occasionally be used interchangeably with terms AD analogtodigital and DA respectively ADVANTAGES OF DIGITAL SIGNAL PROCESSING 1 Digital systems operation can tolerate considerable variation in signal values and hence are less sensitive to changes in the component parameter values due to temperature variation aging and other factors This results in greater degree of precision and stability Since digital systems are binary circuits their accuracy can be increased by using more complex circuitry to increase word length subject to cost limitations 2 Digital systems do not require any factory adjustment and can be easily duplicated in volume without having to worry about precise component values They can be fully integrated and even highly complex systems can be placed on a single chip by using VLSI verylargescale integrated circuits 3 Digital filters are more flexible Their characteristics can be easily altered simply by changing the program Digital hardware implementation permits the use of microprocessors miniprocessors digital switching and largescale integrated circuits 4 A greater variety of filters can be realized by digital systems 5 Digital signals can be stored easily and inexpensively on various media eg magnetic optical and solid state without deterioration of signal quality It is also possible and increasingly popular to search and select information from distant electronic storehouses such as the cloud 6 Digital signals can be coded to yield extremely low error rates and high fidelity as well as privacy Also more sophisticated signalprocessing algorithms can be used to process digital signals The terms discretetime and continuoustime qualify the nature of a signal along the time axis horizontal axis The terms analog and digital in contrast qualify the nature of the signal amplitude vertical axis 03LathiC03 2017925 1554 page 262 26 262 CHAPTER 3 TIMEDOMAIN ANALYSIS OF DISCRETETIME SYSTEMS 7 Digital filters can be easily timeshared and therefore can serve a number of inputs simultaneously Moreover it is easier and more efficient to multiplex several digital signals on the same channel 8 Reproduction with digital messages is extremely reliable without deterioration Analog messages such as photocopies and films for example lose quality at each successive stage of reproduction and have to be transported physically from one distant place to another often at relatively high cost One must weigh these advantages against such disadvantages as increased system complexity due to use of AD and DA interfaces limited range of frequencies available in practice affordable rates are gigahertz or less and use of more power than is needed for the passive analog circuits Digital systems use powerconsuming active devices 341 Classification of DiscreteTime Systems Before examining the nature of discretetime system equations let us consider the concepts of linearity time invariance or shift invariance and causality which apply to discretetime systems also LINEARITY AND TIME INVARIANCE For discretetime systems the definition of linearity is identical to that for continuoustime systems as given in Eq 122 We can show that the systems in Exs 36 37 38 and 39 are all linear Time invariance or shift invariance for discretetime systems is also defined in a way similar to that for continuoustime systems Systems whose parameters do not change with time with n are timeinvariant or shiftinvariant also constantparameter systems For such a system if the input is delayed by k units or samples the output is the same as before but delayed by k samples assuming the initial conditions also are delayed by k The systems in Exs 36 37 38 and 39 are timeinvariant because the coefficients in the system equations are constants independent of n If these coefficients were functions of n time then the systems would be linear timevarying systems Consider for example a system described by yn enxn For this system let a signal x1n yield the output y1n and another input x2n yield the output y2n Then y1n enx1n and y2n enx2n If we let x2n x1n N0 then y2n enx2n enx1n N0 y1n N0 Clearly this is a timevarying parameter system 03LathiC03 2017925 1554 page 263 27 34 Examples of DiscreteTime Systems 263 CAUSAL AND NONCAUSAL SYSTEMS A causal also known as a physical or nonanticipative system is one for which the output at any instant n k depends only on the value of the input xn for n k In other words the value of the output at the present instant depends only on the past and present values of the input xn not on its future values As we shall see the systems in Exs 36 37 38 and 39 are all causal INVERTIBLE AND NONINVERTIBLE SYSTEMS A discretetime system S is invertible if an inverse system Si exists such that the cascade of S and Si results in an identity system An identity system is defined as one whose output is identical to the input In other words for an invertible system the input can be uniquely determined from the corresponding output For every input there is a unique output When a signal is processed through such a system its input can be reconstructed from the corresponding output There is no loss of information when a signal is processed through an invertible system A cascade of a unit delay with a unit advance results in an identity system because the output of such a cascaded system is identical to the input Clearly the inverse of an ideal unit delay is ideal unit advance which is a noncausal and unrealizable system In contrast a compressor yn xMn is not invertible because this operation loses all but every Mth sample of the input and generally the input cannot be reconstructed Similarly operations such as yn cosxn or yn xn are not invertible DRILL 39 Invertibility Show that a system specified by equation yn axn b is invertible but that the system yn xn2 is noninvertible STABLE AND UNSTABLE SYSTEMS The concept of stability is similar to that in continuoustime systems Stability can be internal or external If every bounded input applied at the input terminal results in a bounded output the system is said to be stable externally External stability can be ascertained by measurements at the external terminals of the system This type of stability is also known as the stability in the BIBO boundedinputboundedoutput sense Both internal and external stability are discussed in greater detail in Sec 39 MEMORYLESS SYSTEMS AND SYSTEMS WITH MEMORY The concepts of memoryless or instantaneous systems and those with memory or dynamic are identical to the corresponding concepts of the continuoustime case A system is memoryless if its response at any instant n depends at most on the input at the same instant n The output at any instant of a system with memory generally depends on the past present and future values of the input For example yn sinxn is an example of instantaneous system and ynyn1 xn is an example of a dynamic system or a system with memory 03LathiC03 2017925 1554 page 265 29 35 DiscreteTime System Equations 265 Since any bounded input is guaranteed to produce a bounded output it follows that the system is BIBOstable f To be memoryless a systems output can only depend on the strength of the current input Since the output y at time n depends on the input x not only at present time n but also on past time n 1 we see that the system is not memoryless 35 DISCRETETIME SYSTEM EQUATIONS In this section we discuss timedomain analysis of LTID linear timeinvariant discretetime systems With minor differences the procedure is parallel to that for continuoustime systems DIFFERENCE EQUATIONS Equations 33 35 38 and 313 are examples of difference equations Equations 33 38 and 313 are firstorder difference equations and Eq 35 is a secondorder difference equation All these equations are linear with constant not timevarying coefficients Before giving a general form of an Nthorder linear difference equation we recall that a difference equation can be written in two forms the first form uses delay terms such as yn 1 yn 2 xn 1 xn 2 and so on and the alternate form uses advance terms such as yn 1 yn 2 and so on Although the delay form is more natural we shall often prefer the advance form not just for the general notational convenience but also for resulting notational uniformity with the operator form for differential equations This facilitates the commonality of the solutions and concepts for continuoustime and discretetime systems We start here with a general difference equation written in advance form as yn N a1yn N 1 aN1yn 1 aNyn bNMxn M bNM1xn M 1 bN1xn 1 bNxn 314 This is a linear difference equation whose order is maxNM We have assumed the coefficient of yn N to be unity a0 1 without loss of generality If a0 1 we can divide the equation throughout by a0 to normalize the equation to have a0 1 CAUSALITY CONDITION For a causal system the output cannot depend on future input values This means that when the system equation is in the advance form of Eq 314 causality requires M N If M were to be greater than N then ynN the output at nN would depend on xnM which is the input at the later instant n M For a general causal case M N and Eq 314 can be expressed as yn N a1yn N 1 aN1yn 1 aNyn b0xn N b1 xn N 1 bN1xn 1 bNxn 315 Equations such as 33 35 38 and 313 are considered to be linear according to the classical definition of linearity Some authors label such equations as incrementally linear We prefer the classical definition It is just a matter of individual choice and makes no difference in the final results 03LathiC03 2017925 1554 page 266 30 266 CHAPTER 3 TIMEDOMAIN ANALYSIS OF DISCRETETIME SYSTEMS where some of the coefficients on either side can be zero In this Nthorder equation a0 the coefficient of ynN is normalized to unity Equation 315 is valid for all values of n Therefore it is still valid if we replace n by n N throughout the equation see Eqs 33 and 34 Such replacement yields a delayform alternative yn a1yn 1 aN1yn N 1 aNyn N b0xn b1xn 1 bN1xn N 1 bNxn N 316 351 Recursive Iterative Solution of Difference Equation Equation 316 can be expressed as yn a1yn 1 a2yn 2 aNyn N b0xn b1xn 1 bNxn N 317 In Eq 317 yn is computed from 2N 1 pieces of information the preceding N values of the output yn 1 yn 2 yn N and the preceding N values of the input xn 1 xn 2 xn N and the present value of the input xn Initially to compute y0 the N initial conditions y1 y2 yN serve as the preceding N output values Hence knowing the N initial conditions and the input we can determine recursively the entire output y0 y1 y2 y3 one value at a time For instance to find y0 we set n 0 in Eq 317 The lefthand side is y0 and the righthand side is expressed in terms of N initial conditions y1 y2 yN and the input x0 if xn is causal because of causality other input terms xn 0 Similarly knowing y0 and the input we can compute y1 by setting n 1 in Eq 317 Knowing y0 and y1 we find y2 and so on Thus we can use this recursive procedure to find the complete response y0 y1 y2 For this reason this equation is classed as a recursive form This method basically reflects the manner in which a computer would solve a recursive difference equation given the input and initial conditions Equation 317 or Eq 316 is nonrecursive if all the N 1 coefficients ai 0 i 12 N 1 In this case it can be seen that yn is computed only from the input values and without using any previous outputs Generally speaking the recursive procedure applies only to equations in the recursive form The recursive iterative procedure is demonstrated by the following examples EXAMPLE 311 Iterative Solution to a FirstOrder Difference Equation Solve iteratively yn 05yn 1 xn with initial condition y1 16 and causal input xn n2un This equation can be expressed as yn 05yn 1 xn 318 03LathiC03 2017925 1554 page 267 31 35 DiscreteTime System Equations 267 If we set n 0 in Eq 318 we obtain y0 05y1 x0 0516 0 8 Now setting n 1 in Eq 318 and using the value y0 8 computed in the first step and x1 12 1 we obtain y1 058 12 5 Next setting n 2 in Eq 318 and using the value y1 5 computed in the previous step and x2 22 we obtain y2 055 22 65 Continuing in this way iteratively we obtain y3 0565 32 1225 y4 051225 42 22125 The output yn is depicted in Fig 317 yn 8 5 65 1225 0 1 2 3 4 5 n Figure 317 Iterative solution of a difference equation We now present one more example of iterative solutionthis time for a secondorder equation The iterative method can be applied to a difference equation in delay form or advance form In Ex 311 we considered the former Let us now apply the iterative method to the advance form EXAMPLE 312 Iterative Solution to a SecondOrder Difference Equation Solve iteratively yn 2 yn 1 024yn xn 2 2xn 1 03LathiC03 2017925 1554 page 268 32 268 CHAPTER 3 TIMEDOMAIN ANALYSIS OF DISCRETETIME SYSTEMS with initial conditions y1 2 y2 1 and a causal input xn nun The system equation can be expressed as yn 2 yn 1 024yn xn 2 2xn 1 319 Setting n 2 in Eq 319 and then substituting y1 2 y2 1 x0 x1 0 we obtain y0 2 0241 0 0 176 Setting n 1 in Eq 319 and then substituting y0 176 y1 2 x1 1 x0 0 we obtain y1 176 0242 1 0 228 Setting n 0 in Eq 319 and then substituting y0 176 y1 228 x2 2 and x1 1 yield y2 228 024176 2 21 18576 and so on With MATLAB we can readily verify and extend these recursive calculations n 25 y 12zeros1lengthn2 x 00n3end for k 1lengthn2 yk2 yk1024ykxk22xk1 end ny n 2 1 0 1 2 3 4 5 y 10000 20000 17600 22800 18576 03104 21354 52099 Note carefully the recursive nature of the computations From the N initial conditions and the input we obtained y0 first Then using this value of y0 and the preceding N 1 initial conditions along with the input we find y1 Next using y0 y1 along with the past N 2 initial conditions and input we obtained y2 and so on This method is general and can be applied to a recursive difference equation of any order It is interesting that the hardware realization of Eq 318 depicted in Fig 314 with a 05 generates the solution precisely in this iterative fashion DRILL 310 Iterative Solution to a Difference Equation Using the iterative method find the first three terms of yn for yn 1 2yn xn The initial condition is y1 10 and the input xn 2 starting at n 0 ANSWER y0 20 y1 42 and y2 86 03LathiC03 2017925 1554 page 270 34 270 CHAPTER 3 TIMEDOMAIN ANALYSIS OF DISCRETETIME SYSTEMS RESPONSE OF LINEAR DISCRETETIME SYSTEMS Following the procedure used for continuoustime systems we can show that Eq 320 is a linear equation with constant coefficients A system described by such an equation is a linear timeinvariant discretetime LTID system We can verify as in the case of LTIC systems see the footnote on page 151 that the general solution of Eq 320 consists of zeroinput and zerostate components 36 SYSTEM RESPONSE TO INTERNAL CONDITIONS THE ZEROINPUT RESPONSE The zeroinput response y0n is the solution of Eq 320 with xn 0 that is QEy0n 0 or EN a1EN1 aN1E aNy0n 0 321 Although we can solve this equation systematically even a cursory examination points to the solution This equation states that a linear combination of y0n and advanced y0n is zero not for some values of n but for all n Such a situation is possible if and only if y0n and advanced y0n have the same form Only an exponential function γ n has this property as the following equation indicates Ekγ n γ nk γ kγ n This expression shows that γ n advanced by k units is a constant γ k times γ n Therefore the solution of Eq 321 must be of the form y0n cγ n 322 To determine c and γ we substitute this solution in Eq 321 Since Eky0n y0nk cγ nk this produces cγ N a1γ N1 aN1γ aNγ n 0 For a nontrivial solution of this equation γ N a1γ n1 aN1γ aN 0 323 or Qγ 0 Our solution cγ n Eq 322 is correct provided γ satisfies Eq 323 Now Qγ is an Nthorder polynomial and can be expressed in the factored form assuming all distinct roots γ γ1γ γ2 γ γN 0 Clearly γ has N solutions γ1 γ2 γN and therefore Eq 321 also has N solutions c1γ n 1 c2γ n 2 cnγ n N In such a case we have shown that the general solution is a linear combination A signal of the form nmγ n also satisfies this requirement under certain conditions repeated roots discussed later 03LathiC03 2017925 1554 page 272 36 272 CHAPTER 3 TIMEDOMAIN ANALYSIS OF DISCRETETIME SYSTEMS Therefore y0n 1 502n 4 508n n 0 The reader can verify this solution by computing the first few terms using the iterative method see Exs 311 and 312 DRILL 311 ZeroInput Response of FirstOrder Systems Find and sketch the zeroinput response for the systems described by the following equations a yn 1 08yn 3xn 1 b yn 1 08yn 3xn 1 In each case the initial condition is y1 10 Verify the solutions by computing the first three terms using the iterative method ANSWERS a 808n b 808n DRILL 312 ZeroInput Response of a SecondOrder System with Real Roots Find the zeroinput response of a system described by the equation yn 03yn 1 01yn 2 xn 2xn 1 The initial conditions are y01 1 and y02 33 Verify the solution by computing the first three terms iteratively ANSWER y0n 02n 205n Section 351 introduced the method of recursion to solve difference equations As the next example illustrates the zeroinput response can likewise be found through recursion Since it does 03LathiC03 2017925 1554 page 273 37 36 System Response to Internal Conditions The ZeroInput Response 273 not provide a closedform solution recursion is generally not the preferred method of solving difference equations EXAMPLE 314 Iterative Solution to ZeroInput Response Using the initial conditions y1 2 and y2 1 use MATLAB to iteratively compute and then plot the zeroinput response for the system described by E2 156E 081yn E 3xn n 220 y 12zeroslengthn21 for k 1lengthn2 yk2 156yk1081yk end clf stemnyk xlabeln ylabelyn axis2 20 15 25 0 5 10 15 20 n 1 0 1 2 yn Figure 318 Zeroinput response for Ex 314 REPEATED ROOTS So far we have assumed the system to have N distinct characteristic roots γ1 γ2 γN with corresponding characteristic modes γ n 1 γ n 2 γ n N If two or more roots coincide repeated roots the form of characteristic modes is modified Direct substitution shows that if a root γ repeats r times root of multiplicity r the corresponding characteristic modes for this root are γ n nγ n n2γ n nr1γ n Thus if the characteristic equation of a system is Qγ γ γ1rγ γr1γ γr2 γ γN then the zeroinput response of the system is y0n c1 c2n c3n2 crnr1γ n 1 cr1γ n r1 cr2γ n r2 cnγ n N 03LathiC03 2017925 1554 page 274 38 274 CHAPTER 3 TIMEDOMAIN ANALYSIS OF DISCRETETIME SYSTEMS EXAMPLE 315 ZeroInput Response of a SecondOrder System with Repeated Roots Consider a secondorder difference equation with repeated roots E2 6E 9yn 2E2 6Exn Determine the zeroinput response y0n if the initial conditions are y01 13 and y02 29 The characteristic polynomial is γ 2 6γ 9 γ 32 and we have a repeated characteristic root at γ 3 The characteristic modes are 3n and n3n Hence the zeroinput response is y0n c1 c2n3n Although we can determine the constants c1 and c2 from the initial conditions following a procedure similar to Ex 313 we instead use MATLAB to perform the needed calculations c inv31 13132 2321329 c 4 3 Thus the zeroinput response is y0n 4 3n3n n 0 COMPLEX ROOTS As in the case of continuoustime systems the complex roots of a discretetime system will occur in pairs of conjugates if the system equation coefficients are real Complex roots can be treated exactly as we would treat real roots However just as in the case of continuoustime systems we can also use the real form of solution as an alternative First we express the complex conjugate roots γ and γ in polar form If γ is the magnitude and β is the angle of γ then γ γ ejβ and γ γ ejβ The zeroinput response is given by y0n c1γ n c2γ n c1γ nejβn c2γ nejβn For a real system c1 and c2 must be conjugates so that y0n is a real function of n Let c1 c 2ejθ and c2 c 2ejθ 03LathiC03 2017925 1554 page 280 44 280 CHAPTER 3 TIMEDOMAIN ANALYSIS OF DISCRETETIME SYSTEMS EXAMPLE 319 Filtering Perspective of the Unit Impulse Response Use the MATLAB filter command to solve Ex 318 There are several ways to find the impulse response using MATLAB In this method we first specify the unit impulse function which will serve as our input Vectors a and b are created to specify the system The filter command is then used to determine the impulse response In fact this method can be used to determine the zerostate response for any input n 019 delta n 10n0 a 1 06 016 b 5 0 0 h filterbadeltan clf stemnhk xlabeln ylabelhn 0 5 10 15 20 n 0 2 4 6 hn Figure 319 Impulse response for Ex 319 Comment Although it is relatively simple to determine the impulse response hn by using the procedure in this section in Ch 5 we shall discuss the much simpler method of the ztransform 38 SYSTEM RESPONSE TO EXTERNAL INPUT THE ZEROSTATE RESPONSE The zerostate response yn is the system response to an input xn when the system is in the zero state In this section we shall assume that systems are in the zero state unless mentioned otherwise so that the zerostate response will be the total response of the system Here we follow the procedure parallel to that used in the continuoustime case by expressing an arbitrary input xn as a sum of impulse components A signal xn in Fig 320a can be expressed as a sum of impulse components such as those depicted in Figs 320b320f The component of xn at n m is xmδn m and xn is the sum of all these components summed from m to 03LathiC03 2017925 1554 page 291 55 38 System Response to External Input The ZeroState Response 291 EXAMPLE 324 SlidingTape Method for the Convolution Sum Use the slidingtape method to convolve the two sequences xn and gn depicted in Figs 323a and 323b respectively In this procedure we write the sequences xnandgn in the slots of two tapes x tape and g tape Fig 323c Now leave the x tape stationary to correspond to xm The gm tape is obtained by inverting the gm tape about the origin m 0 so that the slots corresponding to x0 and g0 remain aligned Fig 323d We now shift the inverted tape by n slots multiply values on two tapes in adjacent slots and add all the products to find cn Figures 323d323i show the cases for n 05 Figures 323j 323k and 323l show the cases for n 12 and 3 respectively For the case of n 0 for example Fig 323d c0 2 1 1 1 0 1 3 For n 1 Fig 323e c1 2 1 1 1 0 1 1 1 2 Similarly c2 2 1 1 1 0 1 1 1 2 1 0 c3 2 1 1 1 0 1 1 1 2 1 3 1 3 c4 2 1 1 1 0 1 1 1 2 1 3 1 4 1 7 c5 2 1 1 1 0 1 1 1 2 1 3 1 4 1 7 Figure 323i shows that cn 7 for n 4 Similarly we compute cn for negative n by sliding the tape backward one slot at a time as shown in the plots corresponding to n 1 2 and 3 respectively Figs 323j 323k and 323l c1 2 1 1 1 3 c2 2 1 2 c3 0 Figure 323l shows that cn 0 for n 3 Figure 323m shows the plot of cn 03LathiC03 2017925 1554 page 293 57 38 System Response to External Input The ZeroState Response 293 DRILL 319 SlidingTape Method for the Convolution Sum Use the graphical procedure of Ex 324 slidingtape technique to show that xngn cn in Fig 324 Verify the width property of convolution a xn 1 n 2 3 1 2 3 4 5 6 0 1 2 3 4 5 gn b 1 1 2 3 4 5 6 7 8 9 1011 cn c 1 n n 3 0 6 9 8 Figure 324 Signals for Drill 319 EXAMPLE 325 Convolution of Two FiniteDuration Signals Using MATLAB For the signals xn and gn depicted in Fig 324 use MATLAB to compute and plot cn xn gn x 0 1 2 3 2 1 g 1 1 1 1 1 1 n 01lengthxlengthg2 c convxg clf stemnck xlabeln ylabelcn axis05 105 0 10 0 2 4 6 8 10 n 0 5 10 cn Figure 325 Convolution result for Ex 325 03LathiC03 2017925 1554 page 300 64 300 CHAPTER 3 TIMEDOMAIN ANALYSIS OF DISCRETETIME SYSTEMS n n n n n n n n a b d c n n n n Complex plane Figure 327 Characteristic roots locations and the corresponding characteristic modes 03LathiC03 2017925 1554 page 305 69 310 Intuitive Insights into System Behavior 305 DRILL 321 Assessing Stability by Characteristic Roots Using the complex plane locate the characteristic roots of the following systems and use the characteristic root locations to determine external and internal stability of each system a E 1E2 6E 25yn 3Exn b E 12E 05yn E2 2E 3xn ANSWERS Both systems are BIBOand asymptotically unstable 310 INTUITIVE INSIGHTS INTO SYSTEM BEHAVIOR The intuitive insights into the behavior of continuoustime systems and their qualitative proofs discussed in Sec 26 also apply to discretetime systems For this reason we shall merely mention here without discussion some of the insights presented in Sec 26 The systems entire zeroinput and zerostate behavior is strongly influenced by the characteristic roots or modes of the system The system responds strongly to input signals similar to its characteristic modes and poorly to inputs very different from its characteristic modes In fact when the input is a characteristic mode of the system the response goes to infinity provided the mode is a nondecaying signal This is the resonance phenomenon The width of an impulse response hn indicates the response time time required to respond fully to an input of the system It is the time constant of the system Discretetime pulses are generally dispersed when passed through a discretetime system The amount of dispersion or spreading out is equal to the system time constant or width of hn The system time constant also determines the rate at which the system can transmit information A smaller time constant corresponds to a higher rate of information transmission and vice versa We keep in mind that concepts such as time constant and pulse dispersion only coarsely illustrate system behavior Let us illustrate these ideas with an example EXAMPLE 328 Intuitive Insights into Lowpass DT System Behavior Determine the time constant rise time pulse dispersion and filter characteristics of a lowpass DT system with impulse response hn 206nun This part of the discussion applies to systems with impulse response hn that is a mostly positive or mostly negative pulse 03LathiC03 2017925 1554 page 307 71 311 MATLAB DiscreteTime Signals and Systems 307 A true discretetime function is undefined or zero for noninteger n Although anonymous function f is intended as a discretetime function its present construction does not restrict n to be integer and it can therefore be misused For example MATLAB dutifully returns 08606 to f05 when a NaN notanumber or zero is more appropriate The user is responsible for appropriate function use Next consider plotting the discretetime function fn over 10 n 10 The stem command simplifies this task n 1010 stemnfnk xlabeln ylabelfn Here stem operates much like the plot command dependent variable fn is plotted against independent variable n with black lines The stem command emphasizes the discretetime nature of the data as Fig 331 illustrates For discretetime functions the operations of shifting inversion and scaling can have surprising results Compare f2n with f2n 1 Contrary to the continuous case the second is not a shifted version of the first We can use separate subplots each over 10 n 10 to help illustrate this fact Notice that unlike the plot command the stem command cannot simultaneously plot multiple functions on a single axis overlapping stem lines would make such plots difficult to read anyway subplot211 stemnf2nk ylabelf2n subplot212 stemnf2n1k ylabelf2n1 xlabeln The results are shown in Fig 332 Interestingly the original function fn can be recovered by interleaving samples of f2n and f2n 1 and then timereflecting the result Care must always be taken to ensure that MATLAB performs the desired computations Our anonymous function f is a case in point although it correctly downsamples it does not properly upsample see Prob 3112 MATLAB does what it is told but it is not always told how to do everything correctly 10 5 0 5 10 n 05 0 05 1 fn Figure 331 fn over 10 n 10 03LathiC03 2017925 1554 page 311 75 311 MATLAB DiscreteTime Signals and Systems 311 function y CH3MP1baxyi CH3MP1m Chapter 3 MATLAB Program 1 Function Mfile filters data x to create y INPUTS b vector of feedforward coefficients a vector of feedback coefficients x input data vector yi vector of initial conditions y1 y2 OUTPUTS y vector of filtered output data yi flipudyi Properly format ICs y yizeroslengthx1 Preinitialize y beginning with ICs x zeroslengthyi1x Append x with zeros to match size of y b ba1a aa1 Normalize coefficients for n lengthyi1lengthy for nb 0lengthb1 yn yn bnb1xnnb Feedforward terms end for na 1lengtha1 yn yn ana1ynna Feedback terms end end y ylengthyi1end Strip off ICs for final output Most instructions in CH3MP1 have been discussed now we turn to the flipud instruction The flip updown command flipud reverses the order of elements in a column vector Although not used here the flip leftright command fliplr reverses the order of elements in a row vector Note that typing help filename displays the first contiguous set of comment lines in an Mfile Thus it is good programming practice to document Mfiles as in CH3MP1 with an initial block of clear comment lines As an exercise the reader should verify that CH3MP1 correctly computes the impulse response hn the zerostate response yn the zeroinput response y0n and the total response yny0n 3114 DiscreteTime Convolution Convolution of two finiteduration discretetime signals is accomplished by using the conv command For example the discretetime convolution of two length4 rectangular pulses gn unun4unun4 is a length441 7 triangle Representing unun4 by the vector 1111 the convolution is computed by conv1 1 1 11 1 1 1 ans 1 2 3 4 3 2 1 Notice that un4ununun4 is also computed by conv1 1 1 11 1 1 1 and obviously yields the same result The difference between these two cases is the regions of support 0 n 6 for the first and 4 n 2 for the second Although the conv command 03LathiC03 2017925 1554 page 313 77 313 Summary 313 312 APPENDIX IMPULSE RESPONSE FOR A SPECIAL CASE When aN 0 A0 bNaN becomes indeterminate and the procedure needs to be modified slightly When aN 0 QE can be expressed as E ˆQE and Eq 326 can be expressed as E ˆQEhn PEδn PEEδn 1 EPEδn 1 Hence ˆQEhn PEδn 1 In this case the input vanishes not for n 1 but for n 2 Therefore the response consists not only of the zeroinput term and an impulse A0δn at n 0 but also of an impulse A1δn1 at n 1 Therefore hn A0δn A1δn 1 ycnun We can determine the unknowns A0 A1 and the N 1 coefficients in ycn from the N 1 number of initial values h0 h1 hN determined as usual from the iterative solution of the equation QEhn PEδn Similarly if aN aN1 0 we need to use the form hn A0δnA1δn1A2δn2ycnun The N 1 unknown constants are determined from the N 1 values h0 h1 hN determined iteratively and so on 313 SUMMARY This chapter discusses timedomain analysis of LTID linear timeinvariant discretetime systems The analysis is parallel to that of LTIC systems with some minor differences Discretetime systems are described by difference equations For an Nthorder system N auxiliary conditions must be specified for a unique solution Characteristic modes are discretetime exponentials of the form γ n corresponding to an unrepeated root γ and the modes are of the form niγ n corresponding to a repeated root γ The unit impulse function δn is a sequence of a single number of unit value at n 0 The unit impulse response hn of a discretetime system is a linear combination of its characteristic modes The zerostate response response due to external input of a linear system is obtained by breaking the input into impulse components and then adding the system responses to all the impulse components The sum of the system responses to the impulse components is in the form of a sum known as the convolution sum whose structure and properties are similar to the convolution integral The system response is obtained as the convolution sum of the input xn with the systems impulse response hn Therefore the knowledge of the systems impulse response allows us to determine the system response to any arbitrary input LTID systems have a very special relationship to the everlasting exponential signal zn because the response of an LTID system to such an input signal is the same signal within a multiplicative ˆQγ is now an N 1order polynomial Hence there are only N 1 unknowns in ycn There is a possibility of an impulse δn in addition to characteristic modes 03LathiC03 2017925 1554 page 320 84 320 CHAPTER 3 TIMEDOMAIN ANALYSIS OF DISCRETETIME SYSTEMS 355 Solve the following equation recursively first three terms only yn 2 3yn 1 2yn xn 2 3xn 1 3xn with xn 3nun y1 3 and y2 2 356 Repeat Prob 355 for yn 2yn 1 yn 2 2xn xn 1 withxn 3nuny1 2andy2 3 361 Given y01 3 and y02 1 determine the closedform expression of the zeroinput response y0n of an LTID system described by the equation yn 1 6yn1 1 6yn2 1 3xn 2 3xn 2 362 Solve yn 2 3yn 1 2yn 0 if y1 0 and y2 1 363 Solve yn 2 2yn 1 yn 0 if y1 1 and y2 1 364 Solve yn 2 2yn 1 2yn 0 if y1 1 and y2 0 365 For the general Nthorder difference Eq 316 letting a1 a2 aN1 0 results in a general causal Nthorder LTI nonre cursive difference equation yn b0xn b1xn 1 bNxn N Show that the characteristic roots for this system are zerohence that the zeroinput response is zero Consequently the total response consists of the zerostate component only 366 Leonardo Pisano Fibonacci a famous thirteenth century mathematician generated the sequence of integers 0112358132134 while addressing oddly enough a problem involving rabbit reproduction An element of the Fibonacci sequence is the sum of the previous two a Find the constantcoefficient difference equation whose zeroinput response fn with auxiliary conditions f1 0 and f2 1 is a Fibonacci sequence Given fn is the system output what is the system input b What are the characteristic roots of this system Is the system stable c Designating 0 and 1 as the first and second Fibonacci numbers determine the fiftieth Fibonacci number Determine the one thou sandth Fibonacci number 367 Find vn the voltage at the nth node of the resistive ladder depicted in Fig P348 if V 100 volts and a 2 Hint 1 Consider the node equation at the nth node with voltage vn Hint 2 See Prob 348 for the equation for vn The auxiliary conditions are v0 100 and vN 0 368 Consider the discretetime system yn yn 1 025yn 2 3xn 8 Find the zero input response y0n if y01 1 and y01 1 369 Provide a standardform polynomial QX such that QEyn xn corresponds to a marginally stable thirdorder LTID system and QDyt xt corresponds to a stable thirdorder LTIC system 371 Find the unit impulse response hn of systems specified by the following equations a yn 1 2yn xn b yn 2yn 1 xn 372 Determine the unit impulse response hn of the following systems In each case use recursion to verify the n 3 value of the closedform expression of hn a E2 1yn E 05xn b yn yn 1 025yn 2 xn c yn 1 6yn 1 1 6yn 2 1 3xn 2 03LathiC03 2017925 1554 page 326 90 326 CHAPTER 3 TIMEDOMAIN ANALYSIS OF DISCRETETIME SYSTEMS continues sometimes for many many cups of coffee Joe has noted that his coffee tends to taste sweeter with the number of refills Let independent variable n designate the coffee refill number In this way n 0 indicates the first cup of coffee n 1 is the first refill and so forth Let xn represent the sugar measured in teaspoons added into the system a coffee mug on refill n Let yn designate the amount of sugar again teaspoons contained in the mug on refill n a The sugar teaspoons in Joes coffee can be represented using a standard secondorder constant coefficient difference equation yn a1yn 1 a2yn 2 b0xn b1xn 1 b2xn 2 Determine the constants a1 a2 b0 b1 and b2 b Determine xn the driving function to this system c Solve the difference equation for yn This requires finding the total solution Joe always starts with a clean mug from the dishwasher so y1 the sugar content before the first cup is zero d Determine the steadystate value of yn That is what is yn as n If possible suggest a way of modifying xn so that the sugar content of Joes coffee remains a constant for all nonnegative n 3837 A system is called complex if a realvalued input can produce a complexvalued output Con sider a causal complex system described by a firstorder constant coefficient linear difference equation jE 05yn 5Exn a Determine the impulse response function hn for this system b Given input xn un 5 and initial con dition y01 j determine the systems total output yn for n 0 3838 A discretetime LTI system has impulse response function hn nun 2 un 2 a Carefully sketch the function hn over 5 n 5 b Determine the difference equation represen tation of this system using yn to designate the output and xn to designate the input 3839 Consider three discretetime signals xn yn and zn Denoting convolution as iden tify the expressions that isare equivalent to xnyn zn a xn ynzn b xnyn xnzn c xnyn zn d none of the above Justify your answer 3840 A causal system with input xn and output yn is described by yn nyn 1 xn a By recursion determine the first six nonzero values of hn the response to xn δn Do you think this system is BIBOstable Why b Compute yR4 recursively from yRn nyRn1 xn assuming all initial condi tions are zero and xn un The subscript R is only used to emphasize a recursive solution c Define yCn xnhn Using xn un and hn from part a compute yC4 The subscript C is only used to emphasize a convolution solution d In this chapter both recursion and convo lution are presented as potential methods to compute the zerostate response ZSR of a discretetime system Comparing parts b and c we see that yR4 yC4 Why are the two results not the same Which method if any yields the correct ZSR value 391 In Sec 391 we showed that for BIBO stability in an LTID system it is sufficient for its impulse response hn to satisfy Eq 343 Show that this is also a necessary condition for the system to be BIBOstable In other words show that if Eq 343 is not satisfied there exists a bounded input that produces unbounded output Hint Assume that a system exists for which hn violates Eq 343 yet its output is bounded for every bounded input Establish the contradiction in this statement by considering an input xn defined by xn1 m 1 when hm 0 and xn1m 1 when hm 0 where n1 is some fixed integer 04LathiC04 2017925 1946 page 344 15 344 CHAPTER 4 CONTINUOUSTIME SYSTEM ANALYSIS EXAMPLE 44 Inverse Laplace Transform with MATLAB Using the MATLAB residue command determine the inverse Laplace transform of each of the following functions a Xas 2s2 5 s2 3s 2 b Xbs 2s2 7s 4 s 1s 22 c Xcs 8s2 21s 19 s 2s2 s 7 In each case we use the MATLAB residue command to perform the necessary partial fraction expansions The inverse Laplace transform follows using Table 41 a num 2 0 5 den 1 3 2 r p k residuenumden r 13 7 p 2 1 k 2 Therefore Xas 13s 2 7s 1 2 and xat 13e2t 7etut 2δt b num 2 7 4 den conv1 1conv1 21 2 r p k residuenumden r 3 2 1 p 2 2 1 k Therefore Xbs 3s 2 2s 22 1s 1 and xbt 3e2t 2te2t etut c In this case a few calculations are needed beyond the results of the residue command so that pair 10b of Table 41 can be utilized num 8 21 19 den conv1 21 1 7 r p k residuenumden 04LathiC04 2017925 1946 page 345 16 41 The Laplace Transform 345 r 35000048113i 35000048113i 10000 p 0500025981i 0500025981i 20000 k ang angler mag absr ang 013661 013661 0 mag 35329 35329 10000 Thus Xcs 1 s 2 35329ej013661 s 05 j25981 35329ej013661 s 05 j25981 and xct e2t 17665e05t cos25981t 01366ut EXAMPLE 45 Symbolic Laplace and Inverse Laplace Transforms with MATLAB Using MATLABs symbolic math toolbox determine the following a the direct unilateral Laplace transform of xat sinat cosbt b the inverse unilateral Laplace transform of Xbs as2s2 b2 a Here we use the sym command to symbolically define our variables and expression for xat and then we use the laplace command to compute the unilateral Laplace transform syms a b t xa sinatcosbt Xa laplacexa Xa aa2 s2 sb2 s2 Therefore Xas a s2a2 s s2b2 It is also easy to use MATLAB to determine Xas in standard rational form Xa collectXa Xa a2s ab2 as2 s3s4 a2 b2s2 a2b2 Thus we also see that Xas s3as2a2sab2 s4a2b2s2a2b2 b A similar approach is taken for the inverse Laplace transform except that the ilaplace command is used rather than the laplace command 04LathiC04 2017925 1946 page 347 18 41 The Laplace Transform 347 PierreSimon de Laplace and Oliver Heaviside been unable to explain the irregularities of some heavenly bodies in desperation he concluded that God himself must intervene now and then to prevent such catastrophes as Jupiter eventually falling into the sun and the moon into the earth as predicted by Newtons calculations Laplace proposed to show that these irregularities would correct themselves periodically and that a little patiencein Jupiters case 929 yearswould see everything returning automatically to order thus there was no reason why the solar and the stellar systems could not continue to operate by the laws of Newton and Laplace to the end of time 4 Laplace presented a copy of Mécanique céleste to Napoleon who after reading the book took Laplace to task for not including God in his scheme You have written this huge book on the system of the world without once mentioning the author of the universe Sire Laplace retorted I had no need of that hypothesis Napoleon was not amused and when he reported this reply to another great mathematicianastronomer Louis de Lagrange the latter remarked Ah but that is a fine hypothesis It explains so many things 5 Napoleon following his policy of honoring and promoting scientists made Laplace the minister of the interior To Napoleons dismay however the new appointee attempted to bring the spirit of infinitesimals into administration and so Laplace was transferred hastily to the Senate OLIVER HEAVISIDE 18501925 Although Laplace published his transform method to solve differential equations in 1779 the method did not catch on until a century later It was rediscovered independently in a rather awkward form by an eccentric British engineer Oliver Heaviside 18501925 one of the tragic figures in the history of science and engineering Despite his prolific contributions to electrical engineering he was severely criticized during his lifetime and was neglected later to the point that 04LathiC04 2017925 1946 page 348 19 348 CHAPTER 4 CONTINUOUSTIME SYSTEM ANALYSIS hardly a textbook today mentions his name or credits him with contributions Nevertheless his studies had a major impact on many aspects of modern electrical engineering It was Heaviside who made transatlantic communication possible by inventing cable loading but few mention him as a pioneer or an innovator in telephony It was Heaviside who suggested the use of inductive cable loading but the credit is given to M Pupin who was not even responsible for building the first loading coil In addition Heaviside was 6 The first to find a solution to the distortionless transmission line The innovator of lowpass filters The first to write Maxwells equations in modern form The codiscoverer of rate energy transfer by an electromagnetic field An early champion of the nowcommon phasor analysis An important contributor to the development of vector analysis In fact he essentially created the subject independently of Gibbs 7 An originator of the use of operational mathematics used to solve linear integrodifferential equations which eventually led to rediscovery of the ignored Laplace transform The first to theorize along with Kennelly of Harvard that a conducting layer the KennellyHeaviside layer of atmosphere exists which allows radio waves to follow earths curvature instead of traveling off into space in a straight line The first to posit that an electrical charge would increase in mass as its velocity increases an anticipation of an aspect of Einsteins special theory of relativity 8 He also forecast the possibility of superconductivity Heaviside was a selfmade selfeducated man Although his formal education ended with elementary school he eventually became a pragmatically successful mathematical physicist He began his career as a telegrapher but increasing deafness forced him to retire at the age of 24 He then devoted himself to the study of electricity His creative work was disdained by many professional mathematicians because of his lack of formal education and his unorthodox methods Heaviside had the misfortune to be criticized both by mathematicians who faulted him for lack of rigor and by men of practice who faulted him for using too much mathematics and thereby confusing students Many mathematicians trying to find solutions to the distortionless transmission line failed because no rigorous tools were available at the time Heaviside succeeded because he used mathematics not with rigor but with insight and intuition Using his much maligned operational method Heaviside successfully attacked problems that the rigid mathematicians could not solve problems such as the flowofheat in a body of spatially varying conductivity Heaviside brilliantly used this method in 1895 to demonstrate a fatal flaw in Lord Kelvins determination of the geological age of the earth by secular cooling he used the same flowofheat theory as for his cable analysis Yet the mathematicians of the Royal Society remained unmoved and were not the least impressed by the fact that Heaviside had found the answer to problems no one else could solve Many mathematicians who examined his work dismissed it Heaviside developed the theory for cable loading George Campbell built the first loading coil and the telephone circuits using Campbells coils were in operation before Pupin published his paper In the legal fight over the patent however Pupin won the battle he was a shrewd selfpromoter and Campbell had poor legal support 04LathiC04 2017925 1946 page 349 20 42 Some Properties of the Laplace Transform 349 with contempt asserting that his methods were either complete nonsense or a rehash of known ideas 6 Sir William Preece the chief engineer of the British Post Office a savage critic of Heaviside ridiculed Heavisides work as too theoretical and therefore leading to faulty conclusions Heavisides work on transmission lines and loading was dismissed by the British Post Office and might have remained hidden had not Lord Kelvin himself publicly expressed admiration for it 6 Heavisides operational calculus may be formally inaccurate but in fact it anticipated the operational methods developed in more recent years 9 Although his method was not fully understood it provided correct results When Heaviside was attacked for the vague meaning of his operational calculus his pragmatic reply was Shall I refuse my dinner because I do not fully understand the process of digestion Heaviside lived as a bachelor hermit often in nearsqualid conditions and died largely unnoticed in poverty His life demonstrates the persistent arrogance and snobbishness of the intellectual establishment which does not respect creativity unless it is presented in the strict language of the establishment 42 SOME PROPERTIES OF THE LAPLACE TRANSFORM Properties of the Laplace transform are useful not only in the derivation of the Laplace transform of functions but also in the solutions of linear integrodifferential equations A glance at Eqs 42 and 41 shows that there is a certain measure of symmetry in going from xt to Xs and vice versa This symmetry or duality is also carried over to the properties of the Laplace transform This fact will be evident in the following development We are already familiar with two properties linearity Eq 43 and the uniqueness property of the Laplace transform discussed earlier 421 Time Shifting The timeshifting property states that if xt Xs then for t0 0 xt t0 Xsest0 412 Observe that xt starts at t 0 and therefore xt t0 starts at t t0 This fact is implicit but is not explicitly indicated in Eq 412 This often leads to inadvertent errors To avoid such a pitfall we should restate the property as follows If xtut Xs then xt t0ut t0 Xsest0 t0 0 04LathiC04 2017925 1946 page 364 35 364 CHAPTER 4 CONTINUOUSTIME SYSTEM ANALYSIS of linear systems because the response obtained cannot be separated into zeroinput and zerostate components As we know the zerostate component represents the system response as an explicit function of the input and without knowing this component it is not possible to assess the effect of the input on the system response in a general way The L version can separate the response in terms of the natural and the forced components which are not as interesting as the zeroinput and the zerostate components Note that we can always determine the natural and the forced components from the zeroinput and the zerostate components eg Eq 244 from Eq 243 but the converse is not true Because of these and some other problems electrical engineers wisely started discarding the L version in the early 1960s It is interesting to note the timedomain duals of these two Laplace versions The classical method is the dual of the L method and the convolution zeroinputzerostate method is the dual of the L method The first pair uses the initial conditions at 0 and the second pair uses those at t 0 The first pair the classical method and the L version is awkward in the theoretical study of linear system analysis It was no coincidence that the L version was adopted immediately after the introduction to the electrical engineering community of statespace analysis which uses zeroinputzerostate separation of the output DRILL 47 Laplace Transform to Solve a SecondOrder Linear Differential Equation Solve d2yt dt2 4dyt dt 3yt 2dxt dt xt for the input xt ut The initial conditions are y0 1 and y0 2 ANSWER yt 1 31 9et 7e3tut EXAMPLE 413 Laplace Transform to Solve an Electric Circuit In the circuit of Fig 47a the switch is in the closed position for a long time before t 0 when it is opened instantaneously Find the inductor current yt for t 0 When the switch is in the closed position for a long time the inductor current is 2 amperes and the capacitor voltage is 10 volts When the switch is opened the circuit is equivalent to that depicted in Fig 47b with the initial inductor current y0 2 and the initial capacitor voltage vC0 10 The input voltage is 10 volts starting at t 0 and therefore can be represented by 10ut 04LathiC04 2017925 1946 page 371 42 43 Solution of Differential and IntegroDifferential Equations 371 433 Stability Equation 427 shows that the denominator of Hs is Qs which is apparently identical to the characteristic polynomial Qλ defined in Ch 2 Does this mean that the denominator of Hs is the characteristic polynomial of the system This may or may not be the case since if Ps and Qs in Eq 427 have any common factors they cancel out and the effective denominator of Hs is not necessarily equal to Qs Recall also that the system transfer function Hs like ht is defined in terms of measurements at the external terminals Consequently Hs and ht are both external descriptions of the system In contrast the characteristic polynomial Qs is an internal description Clearly we can determine only external stability that is BIBO stability from Hs If all the poles of Hs are in LHP all the terms in ht are decaying exponentials and ht is absolutely integrable see Eq 245 Consequently the system is BIBOstable Otherwise the system is BIBOunstable Beware of right halfplane poles So far we have assumed that Hs is a proper function that is M N We now show that if Hs is improper that is if M N the system is BIBOunstable In such a case using long division we obtain Hs Rs Hs where Rs is an M Nthorder polynomial and Hs is a proper transfer function For example Hs s3 4s2 4s 5 s2 3s 2 s s2 2s 5 s2 3s 2 As shown in Eq 431 the term s is the transfer function of an ideal differentiator If we apply step function bounded input to this system the output will contain an impulse unbounded output Clearly the system is BIBOunstable Moreover such a system greatly amplifies noise because differentiation enhances higher frequencies which generally predominate in a noise signal These Values of s for which Hs is are the poles of Hs Thus poles of Hs are the values of s for which the denominator of Hs is zero 04LathiC04 2017925 1946 page 373 44 44 Analysis of Electrical Networks The Transformed Network 373 black box with only the input and the output terminals accessible any measurement from these external terminals would show that the transfer function of the system is 1s1 without any hint of the fact that the system is housing an unstable system Fig 49b The impulse response of the cascade system is ht etut which is absolutely integrable Consequently the system is BIBOstable To determine the asymptotic stability we note that S1 has one characteristic root at 1 and S2 also has one root at 1 Recall that the two systems are independent one does not load the other and the characteristic modes generated in each subsystem are independent of the other Clearly the mode et will not be eliminated by the presence of S2 Hence the composite system has two characteristic roots located at 1 and the system is asymptotically unstable though BIBOstable Interchanging the positions of S1 and S2 makes no difference in this conclusion This example shows that BIBO stability can be misleading If a system is asymptotically unstable it will destroy itself or more likely lead to saturation condition because of unchecked growth of the response due to intended or unintended stray initial conditions BIBO stability is not going to save the system Control systems are often compensated to realize certain desirable characteristics One should never try to stabilize an unstable system by canceling its RHP poles with RHP zeros Such a misguided attempt will fail not because of the practical impossibility of exact cancellation but for the more fundamental reason as just explained DRILL 49 BIBO and Asymptotic Stability Show that an ideal integrator is marginally stable but BIBOunstable 434 Inverse Systems If Hs is the transfer function of a system S then Si its inverse system has a transfer function His given by His 1 Hs This follows from the fact the cascade of S with its inverse system Si is an identity system with impulse response δt implying HsHis 1 For example an ideal integrator and its inverse an ideal differentiator have transfer functions 1s and s respectively leading to HsHis 1 44 ANALYSIS OF ELECTRICAL NETWORKS THE TRANSFORMED NETWORK Example 412 shows how electrical networks may be analyzed by writing the integrodifferential equations of the system and then solving these equations by the Laplace transform We now show that it is also possible to analyze electrical networks directly without having to write the 04LathiC04 2017925 1946 page 384 55 384 CHAPTER 4 CONTINUOUSTIME SYSTEM ANALYSIS Figure 417 a SallenKey circuit and b its equivalent We are required to find Hs Vos Vis assuming all initial conditions to be zero Figure 417b shows the transformed version of the circuit in Fig 417a The noninverting amplifier is replaced by its equivalent circuit All the voltages are replaced by their Laplace transforms and all the circuit elements are shown by their impedances All the initial conditions are assumed to be zero as required for determining Hs We shall use node analysis to derive the result There are two unknown node voltages Vas and Vbs requiring two node equations At node a IR1s the current in R1 leaving the node a is Vas VisR1 Similarly IR2s the current in R2 leaving the node a is Vas VbsR2 and IC1s the current in capacitor C1 leaving the node a is Vas VosC1s Vas KVbsC1s 04LathiC04 2017925 1946 page 387 58 45 Block Diagrams 387 We can extend this result to any number of transfer functions in cascade It follows from this discussion that the subsystems in cascade can be interchanged without affecting the overall transfer function This commutation property of LTI systems follows directly from the commutative and associative property of convolution We have already proved this property in Sec 243 Every possible ordering of the subsystems yields the same overall transfer function However there may be practical consequences such as sensitivity to parameter variation affecting the behavior of different ordering Similarly when two transfer functions H1s and H2s appear in parallel as illustrated in Fig 418c the overall transfer function is given by H1s H2s the sum of the two transfer functions The proof is trivial This result can be extended to any number of systems in parallel When the output is fed back to the input as shown in Fig 418d the overall transfer function YsXs can be computed as follows The inputs to the adder are Xs and HsYs Therefore Es the output of the adder is Es Xs HsYs But Ys GsEs GsXs HsYs Therefore Ys1 GsHs GsXs so that Ys Xs Gs 1 GsHs 435 Therefore the feedback loop can be replaced by a single block with the transfer function shown in Eq 435 see Fig 418d In deriving these equations we implicitly assume that when the output of one subsystem is connected to the input of another subsystem the latter does not load the former For example the transfer function H1s in Fig 418b is computed by assuming that the second subsystem H2s was not connected This is the same as assuming that H2s does not load H1s In other words the inputoutput relationship of H1s will remain unchanged regardless of whether H2s is connected Many modern circuits use op amps with high input impedances so this assumption is justified When such an assumption is not valid H1s must be computed under operating conditions ie with H2s connected EXAMPLE 421 Transfer Functions of Feedback Systems Using MATLAB Consider the feedback system of Fig 418d with Gs Kss 8 and Hs 1 Use MATLAB to determine the transfer function for each of the following cases a K 7 b K 16 and c K 80 We solve these cases using the control system toolbox function feedback 04LathiC04 2017925 1946 page 388 59 388 CHAPTER 4 CONTINUOUSTIME SYSTEM ANALYSIS a H tf11 K 7 G tf0 0 K1 8 0 TFa feedbackGH Ha 7 s2 8 s 7 Thus Has 7s2 8s 7 b H tf11 K 16 G tf0 0 K1 8 0 TFb feedbackGH Hb 16 s2 8 s 16 Thus Hbs 16s2 8s 16 c H tf11 K 80 G tf0 0 K1 8 0 TFc feedbackGH Hc 80 s2 8 s 80 Thus Hcs 80s2 8s 80 46 SYSTEM REALIZATION We now develop a systematic method for realization or implementation of an arbitrary Nthorder transfer function The most general transfer function with M N is given by Hs b0sN b1sN1 bN1s bN sN a1sN1 aN1s aN 436 Since realization is basically a synthesis problem there is no unique way of realizing a system A given transfer function can be realized in many different ways A transfer function Hs can be realized by using integrators or differentiators along with adders and multipliers We avoid use of differentiators for practical reasons discussed in Secs 21 and 433 Hence in our implementation we shall use integrators along with scalar multipliers and adders We are already familiar with representation of all these elements except the integrator The integrator can be represented by a box with integral sign timedomain representation Fig 419a or by a box with transfer function 1s frequencydomain representation Fig 419b 04LathiC04 2017925 1946 page 392 63 392 CHAPTER 4 CONTINUOUSTIME SYSTEM ANALYSIS EXAMPLE 422 Canonic Direct Form Realizations Find the canonic direct form realization of the following transfer functions a 5 s 7 b s s 7 c s 5 s 7 d 4s 28 s2 6s 5 All four of these transfer functions are special cases of Hs in Eq 436 a The transfer function 5s 7 is of the first order N 1 therefore we need only one integrator for its realization The feedback and feedforward coefficients are a1 7 and b0 0 b1 5 The realization is depicted in Fig 423a Because N 1 there is a single feedback connection from the output of the integrator to the input adder with coefficient a1 7 For N 1 generally there are N 1 2 feedforward connections However in this case b0 0 and there is only one feedforward connection with coefficient b1 5 from the output of the integrator to the output adder Because there is only one input signal to the output adder we can do away with the adder as shown in Fig 423a b Hs s s 7 In this firstorder transfer function b1 0 The realization is shown in Fig 423b Because there is only one signal to be added at the output adder we can discard the adder c Hs s 5 s 7 The realization appears in Fig 423c Here Hs is a firstorder transfer function with a1 7 and b0 1 b1 5 There is a single feedback connection with coefficient 7 from the integrator output to the input adder There are two feedforward connections Fig 423c When M N as in this case Hs can also be realized in another way by recognizing that Hs 1 2 s 7 We now realize Hs as a parallel combination of two transfer functions as indicated by this equation 04LathiC04 2017925 1946 page 406 77 406 CHAPTER 4 CONTINUOUSTIME SYSTEM ANALYSIS gain which is reduced from 10000 to 99 There is no dearth of forward gain obtained by cascading stages But low sensitivity is extremely precious in precision systems Now consider what happens when we add instead of subtract the signal fed back to the input Such addition means the sign on the feedback connection is instead of which is same as changing the sign of H in Fig 434 Consequently T G 1 GH If we let G 10000 as before and H 09 104 then T 10000 1 09104104 100000 Suppose that because of aging or replacement of some transistors the gain of the forward amplifier changes to 11000 The new gain of the feedback amplifier is T 11000 1 0911000104 1100000 Observe that in this case a mere 10 increase in the forward gain G caused 1000 increase in the gain T from 100000 to 1100000 Clearly the amplifier is very sensitive to parameter variations This behavior is exactly opposite of what was observed earlier when the signal fed back was subtracted from the input What is the difference between the two situations Crudely speaking the former case is called the negative feedback and the latter is the positive feedback The positive feedback increases system gain but tends to make the system more sensitive to parameter variations It can also lead to instability In our example if G were to be 111111 then GH 1 T and the system would become unstable because the signal fed back was exactly equal to the input signal itself since GH 1 Hence once a signal has been applied no matter how small and how short in duration it comes back to reinforce the input undiminished which further passes to the output and is fed back again and again and again In essence the signal perpetuates itself forever This perpetuation even when the input ceases to exist is precisely the symptom of instability Generally speaking a feedback system cannot be described in black and white terms such as positive or negative Usually H is a frequencydependent component more accurately represented by Hs hence it varies with frequency Consequently what was negative feedback at lower frequencies can turn into positive feedback at higher frequencies and may give rise to instability This is one of the serious aspects of feedback systems which warrants a designers careful attention 471 Analysis of a Simple Control System Figure 435a represents an automatic position control system which can be used to control the angular position of a heavy object eg a tracking antenna an antiaircraft gun mount or the position of a ship The input θi is the desired angular position of the object which can be set at any given value The actual angular position θo of the object the output is measured by a potentiometer whose wiper is mounted on the output shaft The difference between the input θi 04LathiC04 2017925 1946 page 411 82 47 Application to Feedback and Controls 411 6 4 2 0 Time seconds 05 1 Amplitude Step Response K7 K16 K80 Figure 436 Step responses for Ex 426 d The unit ramp response is equivalent to the integral of the unit step response We can obtain the ramp response by taking the step response of the system in cascade with an integrator To help highlight waveform detail we compute the ramp response over the short time interval of 0 t 15 t 000115 Hd seriesHctf11 0 stepHdkt titleUnit Ramp Response 05 0 15 1 Time seconds 05 1 15 Amplitude Unit Ramp Response Figure 437 Ramp response for Ex 426 with K 80 DESIGN SPECIFICATIONS Now the reader has some idea of the various specifications a control system might require Generally a control system is designed to meet given transient specifications steadystate error specifications and sensitivity specifications Transient specifications include overshoot rise time and settling time of the response to step input The steadystate error is the difference between 04LathiC04 2017925 1946 page 413 84 48 Frequency Response of an LTIC System 413 ROC for Hs does not include the ω axis where s jω see Eq 410 This means that Hs when s jω is meaningless for BIBOunstable systems Equation 443 shows that for a sinusoidal input of radian frequency ω the system response is also a sinusoid of the same frequency ω The amplitude of the output sinusoid is Hjω times the input amplitude and the phase of the output sinusoid is shifted by Hjω with respect to the input phase see later Fig 438 in Ex 427 For instance a certain system with Hj10 3 and Hj10 30 amplifies a sinusoid of frequency ω 10 by a factor of 3 and delays its phase by 30 The system response to an input 5cos10t 50 is 3 5cos10t 50 30 15cos10t 20 Clearly Hjω is the amplitude gain of the system and a plot of Hjω versus ω shows the amplitude gain as a function of frequency ω We shall call Hjω the amplitude response It also goes under the name magnitude response Similarly Hjω is the phase response and a plot of Hjω versus ω shows how the system modifies or changes the phase of the input sinusoid Plots of the magnitude response Hjω and phase response Hjω show at a glance how a system responds to sinusoids of various frequencies Observe that Hjω has the information of Hjω and Hjω and is therefore termed the frequency response of the system Clearly the frequency response of a system represents its filtering characteristics EXAMPLE 427 Frequency Response Find the frequency response amplitude and phase responses of a system whose transfer function is Hs s 01 s 5 Also find the system response yt if the input xt is a cos 2t b cos10t 50 In this case Hjω jω 01 jω 5 This may also be argued as follows For BIBOunstable systems the zeroinput response contains nondecaying natural mode terms of the form cosω0t or eat cosω0t a 0 Hence the response of such a system to a sinusoid cosωt will contain not just the sinusoid of frequency ω but also nondecaying natural modes rendering the concept of frequency response meaningless Strictly speaking Hω is magnitude response There is a fine distinction between amplitude and magnitude Amplitude A can be positive and negative In contrast the magnitude A is always nonnegative We refrain from relying on this useful distinction between amplitude and magnitude in the interest of avoiding proliferation of essentially similar entities This is also why we shall use the amplitude instead of magnitude spectrum for Hω 04LathiC04 2017925 1946 page 415 86 48 Frequency Response of an LTIC System 415 We also could have read these values directly from the frequency response plots in Fig 438a corresponding to ω 2 This result means that for a sinusoidal input with frequency ω 2 the amplitude gain of the system is 0372 and the phase shift is 653 In other words the output amplitude is 0372 times the input amplitude and the phase of the output is shifted with respect to that of the input by 653 Therefore the system response to the input cos 2t is yt 0372cos2t 653 The input cos 2t and the corresponding system response 0372cos2t 653 are illustrated in Fig 438b b For the input cos10t 50 instead of computing the values Hjω and Hjω as in part a we shall read them directly from the frequency response plots in Fig 438a corresponding to ω 10 These are Hj10 0894 and Hj10 26 Therefore for a sinusoidal input of frequency ω 10 the output sinusoid amplitude is 0894 times the input amplitude and the output sinusoid is shifted with respect to the input sinusoid by 26 Therefore the system response yt to an input cos10t 50 is yt 0894cos10t 50 26 0894cos10t 24 If the input were sin10t 50 the response would be 0894sin10t 50 26 0894 sin10t 24 The frequency response plots in Fig 438a show that the system has highpass filtering characteristics it responds well to sinusoids of higher frequencies ω well above 5 and suppresses sinusoids of lower frequencies ω well below 5 PLOTTING FREQUENCY RESPONSE WITH MATLAB It is simple to use MATLAB to create magnitude and phase response plots Here we consider two methods In the first method we use an anonymous function to define the transfer function Hs and then obtain the frequency response plots by substituting jω for s H s s01s5 omega 00120 subplot121 plotomegaabsH1jomegak subplot122 plotomegaangleH1jomega180pik In the second method we define vectors that contain the numerator and denominator coefficients of Hs and then use the freqs command to compute frequency response B 1 01 A 1 5 H freqsBAomega omega 00120 subplot121 plotomegaabsHk subplot122 plotomegaangleH180pik Both approaches generate plots that match Fig 438a 04LathiC04 2017925 1946 page 416 87 416 CHAPTER 4 CONTINUOUSTIME SYSTEM ANALYSIS EXAMPLE 428 Frequency Responses of Delay Differentiator and Integrator Systems Find and sketch the frequency responses magnitude and phase for a an ideal delay of T seconds b an ideal differentiator and c an ideal integrator a Ideal delay of T seconds The transfer function of an ideal delay is see Eq 430 Hs esT Therefore Hjω ejωT Consequently Hjω 1 and Hjω ωT These amplitude and phase responses are shown in Fig 439a The amplitude response is constant unity for all frequencies The phase shift increases linearly with frequency with a slope of T This result can be explained physically by recognizing that if a sinusoid cosωt is passed through an ideal delay of T seconds the output is cosωt T The output sinusoid amplitude is the same as that of the input for all values of ω Therefore the amplitude response gain is unity for all frequencies Moreover the output cosωt T cosωt ωT has a phase shift ωT with respect to the input cosωt Therefore the phase response is linearly proportional to the frequency ω with a slope T b An ideal differentiator The transfer function of an ideal differentiator is see Eq 431 Hs s Therefore Hjω jω ωejπ2 Consequently Hjω ω and Hjω π 2 These amplitude and phase responses are depicted in Fig 439b The amplitude response increases linearly with frequency and phase response is constant π2 for all frequencies This result can be explained physically by recognizing that if a sinusoid cosωt is passed through an ideal differentiator the output is ωsin ωt ωcosωt π2 Therefore the output sinusoid amplitude is ω times the input amplitude that is the amplitude response gain increases linearly with frequency ω Moreover the output sinusoid undergoes a phase shift π2 with respect to the input cosωt Therefore the phase response is constant π2 with frequency 04LathiC04 2017925 1946 page 418 89 418 CHAPTER 4 CONTINUOUSTIME SYSTEM ANALYSIS with frequency Because its gain is 1ω the ideal integrator suppresses higherfrequency components but enhances lowerfrequency components with ω 1 Consequently noise signals if they do not contain an appreciable amount of verylowfrequency components are suppressed smoothed out by an integrator DRILL 415 Sinusoidal Response of an LTIC System Find the response of an LTIC system specified by d2yt dt2 3dyt dt 2yt dxt dt 5xt if the input is a sinusoid 20sin3t 35 ANSWER 1023sin3t 6191 481 SteadyState Response to Causal Sinusoidal Inputs So far we have discussed the LTIC system response to everlasting sinusoidal inputs starting at t In practice we are more interested in causal sinusoidal inputs sinusoids starting at t 0 Consider the input ejωtut which starts at t 0 rather than at t In this case Xs 1s jω Moreover according to Eq 427 Hs PsQs where Qs is the A puzzling aspect of this result is that in deriving the transfer function of the integrator in Eq 432 we have assumed that the input starts at t 0 In contrast in deriving its frequency response we assume that the everlasting exponential input ejωt starts at t There appears to be a fundamental contradiction between the everlasting input which starts at t and the integrator which opens its gates only at t 0 Of what use is everlasting input since the integrator starts integrating at t 0 The answer is that the integrator gates are always open and integration begins whenever the input starts We restricted the input to start at t 0 in deriving Eq 432 because we were finding the transfer function using the unilateral transform where the inputs begin at t 0 So the integrator starting to integrate at t 0 is restricted because of the limitations of the unilateral transform method not because of the limitations of the integrator itself If we were to find the integrator transfer function using Eq 240 where there is no such restriction on the input we would still find the transfer function of an integrator as 1s Similarly even if we were to use the bilateral Laplace transform where t starts at we would find the transfer function of an integrator to be 1s The transfer function of a system is the property of the system and does not depend on the method used to find it 04LathiC04 2017925 1946 page 422 93 422 CHAPTER 4 CONTINUOUSTIME SYSTEM ANALYSIS We can sketch these four basic terms as functions of ω and use them to construct the logamplitude plot of any desired transfer function Let us discuss each of the terms 491 Constant Ka1a2b1b3 The log amplitude of the constant Ka1a2b1b2 term is also a constant 20logKa1a2b1b3 The phase contribution from this term is zero for positive value and π for negative value of the constant complex constants can have different phases 492 Pole or Zero at the Origin LOG MAGNITUDE A pole at the origin gives rise to the term 20logjω which can be expressed as 20logjω 20logω This function can be plotted as a function of ω However we can effect further simplification by using the logarithmic scale for the variable ω itself Let us define a new variable u such that u logω Hence 20logω 20u The logamplitude function 20u is plotted as a function of u in Fig 440a This is a straight line with a slope of 20 It crosses the u axis at u 0 The ωscale u logω also appears in Fig 440a Semilog graphs can be conveniently used for plotting and we can directly plot ω on semilog paper A ratio of 10 is a decade and a ratio of 2 is known as an octave Furthermore a decade along the ω scale is equivalent to 1 unit along the u scale We can also show that a ratio of 2 an octave along the ω scale equals to 03010 which is log10 2 along the u scale This point can be shown as follows Let ω1 and ω2 along the ω scale correspond to u1 and u2 along the u scale so that logω1 u1 and logω2 u2 Then u2 u1 log10 ω2 log10 ω1 log10ω2ω1 Thus if ω2ω1 10 which is a decade then u2 u1 log10 10 1 and if ω2ω1 2 which is an octave then u2 u1 log10 2 03010 04LathiC04 2017925 1946 page 432 103 432 CHAPTER 4 CONTINUOUSTIME SYSTEM ANALYSIS d The correction at ω 100 because of the corner frequency at ω 100 is 3 dB and the corrections because of the other corner frequencies may be ignored e In addition to the corrections at corner frequencies we may consider corrections at intermediate points for more accurate plots For instance the corrections at ω 4 because of corner frequencies at ω 2 and 10 are 1 and about 065 totaling 165 dB In the same way the corrections at ω 5 because of corner frequencies at ω 2 and 10 are 065 and 1 totaling 165 dB With these corrections the resulting amplitude plot is illustrated in Fig 445a PHASE PLOT We draw the asymptotes corresponding to each of the four factors a The zero at the origin causes a 90 phase shift b The pole at s 2 has an asymptote with a zero value for ω 02 and a slope of 45decade beginning at ω 02 and going up to ω 20 The asymptotic value for ω 20 is 90 c The pole at s 10 has an asymptote with a zero value for ω 1 and a slope of 45decade beginning at ω 1 and going up to ω 100 The asymptotic value for ω 100 is 90 d The zero at s 100 has an asymptote with a zero value for ω 10 and a slope of 45decade beginning at ω 10 and going up to ω 1000 The asymptotic value for ω 1000 is 90 All the asymptotes are added as shown in Fig 445b The appropriate corrections are applied from Fig 442b and the exact phase plot is depicted in Fig 445b EXAMPLE 430 Bode Plots for SecondOrder Transfer Function with Complex Poles Sketch the amplitude and phase response Bode plots for the transfer function Hs 10s 100 s2 2s 100 10 1 s 100 1 s 50 s2 100 MAGNITUDE PLOT Here the constant term is 10 that is 20 dB20 log10 20 To add this term we simply label the horizontal axis from which the asymptotes begin as the 20 dB line as before see Fig 446a 04LathiC04 2017925 1946 page 434 105 434 CHAPTER 4 CONTINUOUSTIME SYSTEM ANALYSIS we have ωn 10 and ζ 01 Step 1 Draw an asymptote of 40 dBdecade 12 dBoctave starting at ω 10 for the complex conjugate poles and draw another asymptote of 20 dBdecade starting at ω 100 for the real zero Step 2 Add both asymptotes Step 3 Apply the correction at ω 100 where the correction because of the corner frequency ω 100 is 3 dB The correction because of the corner frequency ω 10 as seen from Fig 444a for ζ 01 can be safely ignored Next the correction at ω 10 because of the corner frequency ω 10 is 1390 dB see Fig 444a for ζ 01 The correction because of the real zero at 100 can be safely ignored at ω 10 We may find corrections at a few more points The resulting plot is illustrated in Fig 446a PHASE PLOT The asymptote for the complex conjugate poles is a step function with a jump of 180 at ω 10 The asymptote for the zero at s 100 is zero for ω 10 and is a straight line with a slope of 45decade starting at ω 10 and going to ω 1000 For ω 1000 the asymptote is 90 The two asymptotes add to give the sawtooth shown in Fig 446b We now apply the corrections from Figs 442b and 444b to obtain the exact plot 60 40 20 0 20 40 Magnitude dB Bode Diagram 10 0 10 1 10 2 10 3 10 4 Frequency rads 180 135 90 45 0 Phase deg Figure 447 MATLABgenerated Bode plots for Ex 430 04LathiC04 2017925 1946 page 436 107 436 CHAPTER 4 CONTINUOUSTIME SYSTEM ANALYSIS system from the systems response to sinusoids This application has significant practical utility If we are given a system in a black box with only the input and output terminals available the transfer function has to be determined by experimental measurements at the input and output terminals The frequency response to sinusoidal inputs is one of the possibilities that is very attractive because the measurements involved are so simple One needs only to apply a sinusoidal signal at the input and observe the output We find the amplitude gain Hjω and the output phase shift Hjω with respect to the input sinusoid for various values of ω over the entire range from 0 to This information yields the frequency response plots Bode plots when plotted against log ω From these plots we determine the appropriate asymptotes by taking advantage of the fact that the slopes of all asymptotes must be multiples of 20 dBdecade if the transfer function is a rational function function that is a ratio of two polynomials in s From the asymptotes the corner frequencies are obtained Corner frequencies determine the poles and zeros of the transfer function Because of the ambiguity about the location of zeros since LHP and RHP zeros zeros at s a have identical magnitudes this procedure works only for minimum phase systems 410 FILTER DESIGN BY PLACEMENT OF POLES AND ZEROS OF Hs In this section we explore the strong dependence of frequency response on the location of poles and zeros of Hs This dependence points to a simple intuitive procedure to filter design 4101 Dependence of Frequency Response on Poles and Zeros of Hs Frequency response of a system is basically the information about the filtering capability of the system A system transfer function can be expressed as Hs Ps Qs b0 s z1s z2 s zN s λ1s λ2 s λN where z1 z2 zN are λ1 λ2 λN are the poles of Hs Now the value of the transfer function Hs at some frequency s p is Hssp b0 p z1p z2 p zN p λ1p λ2 p λN 453 This equation consists of factors of the form pzi and pλi The factor pzi is a complex number represented by a vector drawn from point z to the point p in the complex plane as illustrated in Fig 448a The length of this line segment is p zi the magnitude of p zi The angle of this directed line segment with the horizontal axis is p zi To compute Hs at s p we draw line segments from all poles and zeros of Hs to the point p as shown in Fig 448b The vector connecting a zero zi to the point p is p zi Let the length of this vector be ri and let its angle with the horizontal axis be φi Then pzi riejφi Similarly the vector connecting a pole λi to the point p is p λi diejθi where di and θi are the length and the angle with the horizontal axis 04LathiC04 2017925 1946 page 439 110 410 Filter Design by Placement of Poles and Zeros of Hs 439 behavior in the vicinity of ω0 This is because the gain in this case is Kdd where d is the distance of a point jω from the conjugate pole α jω0 Because the conjugate pole is far from jω0 there is no dramatic change in the length d as ω varies in the vicinity of ω0 There is a gradual increase in the value of d as ω increases which leaves the frequencyselective behavior as it was originally with only minor changes GAIN SUPPRESSION BY A ZERO Using the same argument we observe that zeros at α jω0 Fig 449d will have exactly the opposite effect of suppressing the gain in the vicinity of ω0 as shown in Fig 449e A zero on the imaginary axis at jω0 will totally suppress the gain zero gain at frequency ω0 Repeated zeros will further enhance the effect Also a closely placed pair of a pole and a zero dipole tend to cancel out each others influence on the frequency response Clearly a proper placement of poles and zeros can yield a variety of frequencyselective behavior We can use these observations to design lowpass highpass bandpass and bandstop or notch filters Phase response can also be computed graphically In Fig 449a angles formed by the complex conjugate poles αjω0 at ω 0 the origin are equal and opposite As ω increases from 0 up the angle θ1 due to the pole α jω0 which has a negative value at ω 0 is reduced in magnitude the angle θ2 because of the pole α jω0 which has a positive value at ω 0 increases in magnitude As a result θ1 θ2 the sum of the two angles increases continuously approaching a value π as ω The resulting phase response Hjω θ1 θ2 is illustrated in Fig 449c Similar arguments apply to zeros at α jω0 The resulting phase response Hjω φ1 φ2 is depicted in Fig 449f We now focus on simple filters using the intuitive insights gained in this discussion The discussion is essentially qualitative 4102 Lowpass Filters A typical lowpass filter has a maximum gain at ω 0 Because a pole enhances the gain at frequencies in its vicinity we need to place a pole or poles on the real axis opposite the origin jω 0 as shown in Fig 450a The transfer function of this system is Hs ωc s ωc We have chosen the numerator of Hs to be ωc to normalize the dc gain H0 to unity If d is the distance from the pole ωc to a point jω Fig 450a then Hjω ωc d with H0 1 As ω increases d increases and Hjω decreases monotonically with ω as illustrated in Fig 450d with label N 1 This is clearly a lowpass filter with gain enhanced in the vicinity of ω 0 WALL OF POLES An ideal lowpass filter characteristic shaded in Fig 450d has a constant gain of unity up to frequency ωc Then the gain drops suddenly to 0 for ω ωc To achieve the ideal lowpass 04LathiC04 2017925 1946 page 448 119 448 CHAPTER 4 CONTINUOUSTIME SYSTEM ANALYSIS Figure 457 Regions of convergence for causal anticausal and combined signals rightsided the poles of Xs lie to the left of the ROC and if xt is anticausal or leftsided the poles of Xs lie to the right of the ROC To prove this generalization we observe that a rightsided signal can be expressed as xt xf t where xt is a causal signal and xf t is some finiteduration signal The ROC of any finiteduration signal is the entire splane no finite poles Hence the ROC of the rightsided signal xt xf t is the region common to the ROCs of xt and xf t which is same as the ROC for xt This proves the generalization for rightsided signals We can use a similar argument to generalize the result for leftsided signals Let us find the bilateral Laplace transform of xt ebtut eatut 458 We already know the Laplace transform of the causal component eatut 1 s a Res a 459 For the anticausal component x2t ebtut we have x2t ebtut 1 s b Res b so that X2s 1 s b 1 s b Res b Therefore ebtut 1 s b Res b 460 04LathiC04 2017925 1946 page 454 125 454 CHAPTER 4 CONTINUOUSTIME SYSTEM ANALYSIS s 2 lies to the right of the ROC and thus represents an anticausal signal Hence yt 1 6etut 1 2etut 2 3e2tut Figure 460c shows yt Note that in this example if xt e4tut e2tut then the ROC of Xs is 4 Res 2 Here no region of convergence exists for XsHs Hence the response yt goes to infinity EXAMPLE 434 Response of a Noncausal System Find the response yt of a noncausal system with the transfer function Hs 1 s 1 Res 1 to the input xt e2tut We have Xs 1 s 2 Res 2 and Ys XsHs 1 s 1s 2 The ROC of XsHs is the region 2 Res 1 By partial fraction expansion Ys 13 s 1 13 s 2 2 Res 1 and yt 1 3etut e2tut Note that the pole of Hs lies in the RHP at 1 Yet the system is not unstable The poles in the RHP may indicate instability or noncausality depending on its location with respect to the region of convergence of Hs For example if Hs 1s1 with Res 1 the system is causal and unstable with ht etut In contrast if Hs 1s 1 with Res 1 the system is noncausal and stable with ht etut 04LathiC04 2017925 1946 page 458 129 458 CHAPTER 4 CONTINUOUSTIME SYSTEM ANALYSIS 0 02 04 06 08 1 12 14 16 18 2 f Hz 104 0 02 04 06 08 1 Hj2 π f Ideal Firstorder RC Figure 462 Magnitude response HRCj2πf of a firstorder RC filter xt R yt R C C Figure 463 A cascaded RC filter A CASCADED RC FILTER AND POLYNOMIAL EXPANSION A firstorder RC filter is destined for poor performance one pole is simply insufficient to obtain good results A cascade of RC circuits increases the number of poles and improves the filter response To simplify the analysis and prevent loading between stages we employ opamp followers to buffer the output of each stage as shown in Fig 463 A cascade of N stages results in an Nthorder filter with transfer function given by Hcascades HRCsN RCs 1N Upon choosing a cascade of 10 stages and C 1 nF a 3 kHz cutoff frequency is obtained by setting R 2110 1Cωc 2110 16π106 R sqrt21101Comegac R 14213e004 This cascaded filter has a 10thorder pole at λ 1RC and no finite zeros To compute the magnitude response polynomial coefficient vectors A and B are needed Setting B 1 ensures there are no finite zeros or equivalently that all zeros are at infinity The poly command which expands a vector of roots into a corresponding vector of polynomial coefficients is used to obtain A 04LathiC04 2017925 1946 page 459 130 412 MATLAB ContinuousTime Filters 459 B 1 A poly1RCones101A AAend Hmagcascade absCH4MP1BAf2pi plotfabsf2piomegackfHmagcascadek axis0 20000 005 105 xlabelf Hz ylabelHj2pi f legendIdealTenthorder RC cascadelocationbest Notice that scaling a polynomial by a constant does not change its roots Conversely the roots of a polynomial specify a polynomial within a scale factor The command A AAend properly scales the denominator polynomial to ensure unity gain at ω 0 The magnitude response plot of the tenthorder RC cascade is shown in Fig 464 Compared with the simple RC response of Fig 462 the passband remains relatively unchanged but stopband attenuation is greatly improved to over 60 dB at 20 kHz 0 02 04 06 08 1 12 14 16 18 2 f Hz 10 4 0 02 04 06 08 1 Hj2 π f Ideal Tenthorder RC cascade Figure 464 Magnitude response Hcascadej2πf of a tenthorder RC cascade 4122 Butterworth Filters and the Find Command The pole location of a firstorder lowpass filter is necessarily fixed by the cutoff frequency There is little reason however to place all the poles of a 10thorder filter at one location Better pole placement will improve our filters magnitude response One strategy discussed in Sec 410 is to place a wall of poles opposite the passband frequencies A semicircular wall of poles leads to the Butterworth family of filters and a semielliptical shape leads to the Chebyshev family of filters Butterworth filters are considered first To begin notice that a transfer function Hs with real coefficients has a squared magnitude response given by Hjω2 HjωHjω HjωHjω HsHssjω Thus half the poles of Hjω2 correspond to the filter Hs and the other half correspond to Hs Filters that are both stable and causal require Hs to include only lefthalfplane poles The squared magnitude response of a Butterworth filter is HBWjω2 1 1 jωjωc2N This function has the same appealing characteristics as the firstorder RC filter a gain that is unity at ω 0 and monotonically decreases to zero as ω By construction the halfpower gain 04LathiC04 2017925 1946 page 460 131 460 CHAPTER 4 CONTINUOUSTIME SYSTEM ANALYSIS 2 15 1 05 0 05 1 15 2 2 15 1 05 0 05 1 15 2 Imaginary 3 104 Real 3 104 Figure 465 Roots of HBWjω2 for N 10 and ωc 30002π occurs at ωc Perhaps most importantly however the first 2N 1 derivatives of HBWjω with respect to ω are zero at ω 0 Put another way the passband is constrained to be very flat for low frequencies For this reason Butterworth filters are sometimes called maximally flat filters As discussed in Sec B7 the roots of minus 1 must lie equally spaced on a circle centered at the origin Thus the 2N poles of HBWjω2 naturally lie equally spaced on a circle of radius ωc centered at the origin Figure 465 displays the 20 poles corresponding to the case N 10 and ωc 30002π rads An Nthorder Butterworth filter that is both causal and stable uses the N lefthalfplane poles of HBWjω2 To design a 10thorder Butterworth filter we first compute the 20 poles of HBWjω2 N10 poles roots1jomegac2Nzeros12N11 The find command is a powerful and useful function that returns the indices of a vectors nonzero elements Combined with relational operators the find command allows us to extract the 10 lefthalfplane roots that correspond to the poles of our Butterworth filter BWpoles polesfindrealpoles0 To compute the magnitude response these roots are converted to coefficient vector A A polyBWpoles A AAend HmagBW absCH4MP1BAf2pi plotfabsf2piomegackfHmagBWk axis0 20000 005 105 xlabelf Hz ylabelHj2pi f legendIdealTenthorder Butterworthlocationbest The magnitude response plot of the Butterworth filter is shown in Fig 466 The Butterworth response closely approximates the brickwall function and provides excellent filter characteristics flat passband rapid transition to the stopband and excellent stopband attenuation 40 dB at 5 kHz 04LathiC04 2017925 1946 page 462 133 462 CHAPTER 4 CONTINUOUSTIME SYSTEM ANALYSIS xt yt R2 C1 R1 C2 Figure 467 SallenKey filter stage provides a measure of the peakedness of the response HighQ filters have poles close to the ω axis which boost the magnitude response near those frequencies Although many ways exist to determine suitable component values a simple method is to assign R1 a realistic value and then let R2 R1 C1 2Qω0R1 and C2 12Qω0R2 Butterworth poles are a distance ωc from the origin so ω0 ωc For our 10thorder Butterworth filter the angles ψ are regularly spaced at 9 27 45 63 and 81 degrees MATLAB program CH4MP2 automates the task of computing component values and magnitude responses for each stage CH4MP2m Chapter 4 MATLAB Program 2 Script Mfile computes SallenKey component values and magnitude responses for each of the five cascaded secondorder filter sections omega0 30002pi Filter cutoff frequency psi 9 27 45 63 81pi180 Butterworth pole angles f linspace06000200 Frequency range for magnitude response calculations HmagSK zeros5200 Preallocate array for magnitude responses for stage 15 Q 12cospsistage Compute Q for current stage Compute and display filter components to the screen dispStage num2strstage Q num2strQ R1 R2 num2str56000 C1 num2str2Qomega056000 C2 num2str12Qomega056000 B omega02 A 1 omega0Q omega02 Compute filter coefficients HmagSKstage absCH4MP1BA2pif Compute magnitude response end plotfHmagSKkfprodHmagSKk xlabelf Hz ylabelMagnitude Response The disp command displays a character string to the screen Character strings must be enclosed in single quotation marks The num2str command converts numbers to character strings and facilitates the formatted display of information The prod command multiplies along the columns of a matrix it computes the total magnitude response as the product of the magnitude responses of the five stages Executing the program produces the following output CH4MP2 Stage 1 Q 050623 R1 R2 56000 C1 95916e10 C2 93569e10 Stage 2 Q 056116 R1 R2 56000 C1 10632e09 C2 8441e10 Stage 3 Q 070711 R1 R2 56000 C1 13398e09 C2 66988e10 Stage 4 Q 11013 R1 R2 56000 C1 20867e09 C2 43009e10 Stage 5 Q 31962 R1 R2 56000 C1 60559e09 C2 1482e10 04LathiC04 2017925 1946 page 463 134 412 MATLAB ContinuousTime Filters 463 0 1000 2000 3000 4000 5000 6000 f Hz 0 05 1 15 2 25 3 35 Magnitude Response Figure 468 Magnitude responses for SallenKey filter stages Since all the component values are practical this filter is possible to implement Figure 468 displays the magnitude responses for all five stages solid lines The total response dotted line confirms a 10thorder Butterworth response Stage 5 which has the largest Q and implements the pair of conjugate poles nearest the ω axis is the most peaked response Stage 1 which has the smallest Q and implements the pair of conjugate poles furthest from the ω axis is the least peaked response In practice it is best to order highQ stages last this reduces the risk that the high gains will saturate the filter hardware 4124 Chebyshev Filters Like an orderN Butterworth lowpass filter LPF an orderN Chebyshev LPF is an allpole filter that possesses many desirable characteristics Compared with an equalorder Butterworth filter the Chebyshev filter achieves better stopband attenuation and reduced transition bandwidth by allowing an adjustable amount of ripple within the passband The squared magnitude response of a Chebyshev filter is HCjω2 1 1 ϵ2C2 Nωωc where ϵ controls the passband ripple CNωωc is a degreeN Chebyshev polynomial and ωc is the radian cutoff frequency Several characteristics of Chebyshev LPFs are noteworthy An orderN Chebyshev LPF is equiripple in the passband ω ωc has a total of N maxima and minima over 0 ω ωc and is monotonic decreasing in the stopband ω ωc 04LathiC04 2017925 1946 page 464 135 464 CHAPTER 4 CONTINUOUSTIME SYSTEM ANALYSIS In the passband the maximum gain is 1 and the minimum gain is 1 1 ϵ2 For oddvalued N Hj0 1 For evenvalued N HCj0 1 1 ϵ2 Ripple is controlled by setting ϵ 10R10 1 where R is the allowable passband ripple expressed in decibels Reducing ϵ adversely affects filter performance see Prob 41210 Unlike Butterworth filters the cutoff frequency ωc rarely specifies the 3 dB point For ϵ 1 HCjωc2 11ϵ2 05 The cutoff frequency ωc simply indicates the frequency after which HCjω 1 1 ϵ2 The Chebyshev polynomial CNx is defined as CNx cosN cos1x coshN cosh1x In this form it is difficult to verify that CNx is a degreeN polynomial in x A recursive form of CNx makes this fact more clear see Prob 41213 CNx 2xCN1x CN2x With C0x 1 and C1x x the recursive form shows that any CN is a linear combination of degreeN polynomials and is therefore a degreeN polynomial itself For N 2 MATLAB program CH4MP3 generates the N 1 coefficients of Chebyshev polynomial CNx function CN CH4MP3N CH4MP3m Chapter 4 MATLAB Program 3 Function Mfile computes Chebyshev polynomial coefficients using the recursion relation CNx 2xCN1x CN2x INPUTS N degree of Chebyshev polynomial OUTPUTS CN vector of Chebyshev polynomial coefficients CNm2 1 CNm1 1 0 Initial polynomial coefficients for t 2N CN 2conv1 0CNm1zeros1lengthCNm1lengthCNm21CNm2 CNm2 CNm1 CNm1 CN end As examples consider C2x 2xC1x C0x 2xx 1 2x2 1 and C3x 2xC2x C1x 2x2x2 1 x 4x3 3x CH4MP3 easily confirms these cases CH4MP32 ans 2 0 1 CH4MP33 ans 4 0 3 0 Since CNωωc is a degreeN polynomial HCjω2 is an allpole rational function with 2N finite poles Similar to the Butterworth case the N poles specifying a causal and stable Chebyshev filter can be found by selecting the N lefthalfplane roots of 1 ϵ2C2 Nsjωc Root locations and dc gain are sufficient to specify a Chebyshev filter for a given N and ϵ To demonstrate consider the design of an order8 Chebyshev filter with cutoff frequency fc 1 kHz and allowable passband ripple R 1 dB First filter parameters are specified 04LathiC04 2017925 1946 page 465 136 412 MATLAB ContinuousTime Filters 465 omegac 2pi1000 R 1 N 8 epsilon sqrt10R101 The coefficients of CNsjωc are obtained with the help of CH4MP3 and then the coefficients of 1 ϵ2C2 Nsjωc are computed by using convolution to perform polynomial multiplication CN CH4MP3N11jomegacN10 CP epsilon2convCNCN CPend CPend1 Next the polynomial roots are found and the lefthalfplane poles are retained and plotted poles rootsCP i findrealpoles0 Cpoles polesi plotrealCpolesimagCpoleskx axis equal axisomegac11 11 11 11 xlabelReal ylabelImaginary As shown in Fig 469 the roots of a Chebyshev filter lie on an ellipse see Prob 41214 5000 0 5000 Real 6000 4000 2000 0 2000 4000 6000 Imaginary Figure 469 Polezero plot for an order8 Chebyshev LPF with fc 1 kHz and R 1 dB To compute the filters magnitude response the poles are expanded into a polynomial the dc gain is set based on the even value of N and CH4MP1 is used A polyCpoles B Aendsqrt1epsilon2 omega linspace02pi20002001 HC CH4MP1BAomega plotomega2piabsHCk axis0 2000 0 11 xlabelf Hz ylabelHCj2pi f E A Guillemin demonstrates a wonderful relationship between the Chebyshev ellipse and the Butterworth circle in his book Synthesis of Passive Networks Wiley New York 1957 04LathiC04 2017925 1946 page 467 138 413 Summary 467 equations Therefore solving these integrodifferential equations reduces to solving algebraic equations The Laplace transform method cannot be used for timevaryingparameter systems or for nonlinear systems in general The transfer function Hs of an LTIC system is the Laplace transform of its impulse response It may also be defined as a ratio of the Laplace transform of the output to the Laplace transform of the input when all initial conditions are zero system in zero state If Xs is the Laplace transform of the input xt and Ys is the Laplace transform of the corresponding output yt when all initial conditions are zero then Ys XsHs For an LTIC system described by an Nthorder differential equation QDyt PDxt the transfer function Hs PsQs Like the impulse response ht the transfer function Hs is also an external description of the system Electrical circuit analysis can also be carried out by using a transformed circuit method in which all signals voltages and currents are represented by their Laplace transforms all elements by their impedances or admittances and initial conditions by their equivalent sources initial condition generators In this method a network can be analyzed as if it were a resistive circuit Large systems can be depicted by suitably interconnected subsystems represented by blocks Each subsystem being a smaller system can be readily analyzed and represented by its inputoutput relationship such as its transfer function Analysis of large systems can be carried out with the knowledge of inputoutput relationships of its subsystems and the nature of interconnection of various subsystems LTIC systems can be realized by scalar multipliers adders and integrators A given transfer function can be synthesized in many different ways such as canonic cascade and parallel Moreover every realization has a transpose which also has the same transfer function In practice all the building blocks scalar multipliers adders and integrators can be obtained from operational amplifiers The system response to an everlasting exponential est is also an everlasting exponential Hsest Consequently the system response to an everlasting exponential ejωt is Hjωejωt Hence Hjω is the frequency response of the system For a sinusoidal input of unit amplitude and having frequency ω the system response is also a sinusoid of the same frequency ω with amplitude Hjω and its phase is shifted by Hjω with respect to the input sinusoid For this reason Hjω is called the amplitude response gain and Hjω is called the phase response of the system Amplitude and phase response of a system indicate the filtering characteristics of the system The general nature of the filtering characteristics of a system can be quickly determined from a knowledge of the location of poles and zeros of the system transfer function Most of the input signals and practical systems are causal Consequently we are required most of the time to deal with causal signals When all signals must be causal the Laplace transform analysis is greatly simplified the region of convergence of a signal becomes irrelevant to the analysis process This special case of the Laplace transform which is restricted to causal signals is called the unilateral Laplace transform Much of the chapter deals with this variety of Laplace transform Section 411 discusses the general Laplace transform the bilateral Laplace transform which can handle causal and noncausal signals and systems In the bilateral transform the inverse transform of Xs is not unique but depends on the region of convergence of Xs Thus the region of convergence plays a very crucial role in the bilateral Laplace transform 04LathiC04 2017925 1946 page 470 141 470 CHAPTER 4 CONTINUOUSTIME SYSTEM ANALYSIS t t t xt a gt b T0 2T0 3T0 pt c 1 2 8 10 16 18 24 Figure P429 4211 a Find the Laplace transform of the pulses in Fig 42 by using only the timedifferent iation property the timeshifting property and the fact that δt 1 b In Ex 49 the Laplace transform of xt is found by finding the Laplace transform of d2xdt2 Find the Laplace transform of xt in that example by finding the Laplace transform of dxdt and using Table 41 if necessary 4212 Determine the inverse unilateral Laplace trans form of Xs 1 es3 s2 s 1s 2 4213 Since 13 is such a lucky number determine the inverse Laplace transform of Xs 1s 113 given region of convergence σ 1 Hint What is the nth derivative of 1s a 4214 It is difficult to compute the Laplace transform Xs of signal xt 1 t ut by using direct integration Instead properties provide a simpler method a Use Laplace transform properties to express the Laplace transform of txt in terms of the unknown quantity Xs b Use the definition to determine the Laplace transform of yt txt c Solve for Xs by using the two pieces from a and b Simplify your answer 431 Use the Laplace transform to solve the following differential equations a D2 3D 2yt Dxt if y0 y0 0 and xt ut b D2 4D 4yt D 1xt if y0 2 y0 1 and xt etut c D2 6D25yt D2xt if y0 y0 1 and xt 25ut 432 Solve the differential equations in Prob 431 using the Laplace transform In each case deter mine the zeroinput and zerostate components of the solution 433 Consider a causal LTIC system described by the differential equation 2yt 6yt xt 4xt a Using transformdomain techniques deter mine the ZIR yzirt if y0 3 b Using transformdomain techniques deter mine the ZSR yzsrt to the input xt eδt π 434 Consider a causal LTIC system described by the differential equation yt 3yt 2yt 2xt xt 04LathiC04 2017925 1946 page 478 149 478 CHAPTER 4 CONTINUOUSTIME SYSTEM ANALYSIS Fig P454b consider three cases a K 10 b K 50 and c K 48 461 Realize Hs ss 2 s 1s 3s 4 by canonic direct series and parallel forms 462 Realize the transfer function in Prob 461 by using the transposed form of the realizations found in Prob 461 463 Repeat Prob 461 for a Hs 3ss 2 s 1s2 2s 2 b Hs 2s 4 s 2s2 4 464 Realize the transfer functions in Prob 463 by using the transposed form of the realizations found in Prob 463 465 Repeat Prob 461 for Hs 2s 3 5ss 22s 3 466 Realize the transfer function in Prob 465 by using the transposed form of the realizations found in Prob 465 467 Repeat Prob 461 for Hs ss 1s 2 s 5s 6s 8 468 Realize the transfer function in Prob 467 by using the transposed form of the realizations found in Prob 467 469 Repeat Prob 461 for Hs s3 s 12s 2s 3 4610 Realize the transfer function in Prob 469 by using the transposed form of the realizations found in Prob 469 4611 Repeat Prob 461 for Hs s3 s 1s2 4s 13 4612 Realize the transfer function in Prob 4611 by using the transposed form of the realizations found in Prob 4611 4613 Draw a TDFII block realization of a causal LTIC system with transfer function Hs s2js2j sjsjs2 Give two reasons why TDFII tends to be a good structure 4614 Consider a causal LTIC system with transfer function Hs s2js2js3js3j 9s1s2s1js1j a Realize Hs using a single fourthorder real TDFII structure Is this block realization unique Explain b Realize Hs using a cascade of second order real DFII structures Is this block realization unique Explain c Realize Hs using a parallel connection of secondorder real DFI structures Is this block realization unique Explain 4615 In this problem we show how a pair of complex conjugate poles may be realized by using a cascade of two firstorder transfer functions and feedback Show that the transfer functions of the block diagrams in Figs P4615a and P4615b are a Has 1 s a2 b2 1 s2 2as a2 b2 b Hbs s a s a2 b2 s a s2 2as a2 b2 Hence show that the transfer function of the block diagram in Fig P4615c is c Hcs As B s a2 b2 As B s2 2as a2 b2 4616 Show opamp realizations of the following transfer functions a 10 s 5 b 10 s 5 c s 2 s 5 04LathiC04 2017925 1946 page 480 151 480 CHAPTER 4 CONTINUOUSTIME SYSTEM ANALYSIS c To decrease the bandwidth of this system we use positive feedback with Hs 09 as illustrated in Fig P471c Show that the 3 dB bandwidth of this system is ωc10 What is the dc gain d The system gain at dc times its 3 dB bandwidth is the gainbandwidth product of a system Show that this product is the same for all the three systems in Fig P471 This result shows that if we increase the bandwidth the gain decreases and vice versa 481 Suppose an engineer builds a controllable observable LTIC system with transfer function Hs s24 2s24s4 a By direct calculation compute the magni tude response at frequencies ω 0 1 2 3 5 10 and Use these calculations to roughly sketch the magnitude response over 0 ω 10 b To test the system the engineer connects a signal generator to the system in hopes to measure the magnitude response using a standard oscilloscope What type of signal should the engineer input into the system to make the measurements How should the engineer make the measurements Pro vide sufficient detail to fully justify your answers c Suppose the engineer accidentally con structs the system H1s 1 Hs 2s24s4 s24 What impact will this mistake have on his tests 482 For an LTIC system described by the transfer function Hs s 2 s2 5s 4 find the response to the following everlasting sinusoidal inputs a 5cos2t 30 b 10sin2t 45 c 10cos3t 40 Observe that these are everlasting sinusoids 483 For an LTIC system described by the transfer function Hs s 3 s 22 find the steadystate system response to the following inputs a 10ut b cos2t 60ut c sin3t 45ut d ej3tut 484 For an allpass filter specified by the transfer function Hs s 10 s 10 find the system response to the following ever lasting inputs a ejωt b cosωt θ c cos t d sin 2t e cos 10t f cos 100t Comment on the filter response 485 The polezero plot of a secondorder system Hs is shown in Fig P485 The dc response of this system is minus 1 Hj0 1 a Letting Hs ks2 b1s b2s2 a1s a2 determine the constants k b1 b2 a1 and a2 b What is the output yt of this system in response to the input xt 4 cost2 π3 2 15 1 05 0 s v 05 1 15 2 2 15 1 05 0 05 1 15 2 Figure P485 486 Consider a CT system described by D1D 2yt xt 1 Notice that this differential equation is in terms of xt 1 not xt a Determine the output yt given input xt 1 04LathiC04 2017925 1946 page 481 152 Problems 481 b Determine the output yt given input xt cost 487 An LTIC system has transfer function Hs 4s s22s37 4s s16js16j Determine the steadystate output in response to input xt 1 3ej6tπ3u6t π3 491 Suppose a real firstorder lowpass system Hs has unity gain in the passband one finite pole at s 2 and one finite zero at an unspecified location a Determine the location of the system zero so that the filter achieves 40 dB of stop band attenuation Sketch the corresponding straightline Bode approximation of the system magnitude response b Determine the location of the system zero so that the filter achieves 30 dB of stop band attenuation Sketch the corresponding straightline Bode approximation of the system magnitude response 492 Repeat Prob 491 for a highpass rather than a lowpass system 493 Repeat Prob 491 for a secondorder system that has a pair of repeated poles and a pair of repeated zeros 494 Sketch Bode plots for the following transfer functions a ss 100 s 2s 20 b s 10s 20 s2s 100 c s 10s 200 s 202s 1000 495 Repeat Prob 494 for a s2 s 1s2 4s 16 b s s 1s2 1414s 100 c s 10 ss2 1414s 100 496 Using the lowest order possible determine a system function Hs with realvalued roots that matches the frequency response in Fig P496 Verify your answer with MATLAB 497 A graduate student recently implemented an analog phase lock loop PLL as part of his the sis His PLL consists of four basic components a phasefrequency detector a charge pump a loop filter and a voltagecontrolled oscillator This problem considers only the loop filter which is shown in Fig P497a The loop filter input is the current xt and the output is the voltage yt a Derive the loop filters transfer function Hs Express Hs in standard form b Figure P497b provides four possible fre quency response plots labeled A through D Each loglog plot is drawn to the same scale and line slopes are either 20 dBdecade 0 dBdecade or 20 dBdecade Clearly identify which plots if any could repre sent the loop filter c Holding the other components constant what is the general effect of increasing the resistance R on the magnitude response for lowfrequency inputs 101 100 101 102 103 104 10 5 0 5 10 15 20 25 30 35 40 v rads Hjv dB Bode approximation True H jv Figure P496 04LathiC04 2017925 1946 page 482 153 482 CHAPTER 4 CONTINUOUSTIME SYSTEM ANALYSIS d Holding the other components constant what is the general effect of increasing the resistance R on the magnitude response for highfrequency inputs A B C D b xt yt a R C1 C2 Figure P497 4101 A causal LTIC system Hs 2s4js4j s12js12j has input xt 1 2cos2t 3sin4t π3 4cos10t Below perform accurate cal culations at ω 0 2 4 and 10 a Using the graphical method of Sec 4101 accurately sketch the magnitude response Hjω over 10 ω 10 b Using the graphical method of Sec 4101 accurately sketch the phase response Hjω over 10 ω 10 c Approximate the system output yt in response to the input xt 4102 The polezero plot of a secondorder system Hs is shown in Fig P4102 The dc response of this system is minus 2 Hj0 2 a Letting Hs k s2b1sb2 s2a1sa2 determine the constants k b1 b2 a1 and a2 b Using the graphical method of Sec 4101 handsketch the magnitude response Hjω over 10 ω 10 Verify your sketch with MATLAB c Using the graphical method of Sec 4101 handsketch the phase response Hjω over 10 ω 10 Verify your sketch with MATLAB d What is the output yt in response to input xt 3 cos3tπ3 sin4tπ8 Re Im 1 4 3 3 4 Figure P4102 4103 Using the graphical method of Sec 4101 draw a rough sketch of the amplitude and phase responses of an LTIC system described by the transfer function Hs s2 2s 50 s2 2s 50 s 1 j7s 1 j7 s 1 j7s 1 j7 What kind of filter is this 4104 Using the graphical method of Sec 4101 draw a rough sketch of the amplitude and phase responses of LTIC systems whose polezero plots are shown in Fig P4104 04LathiC04 2017925 1946 page 486 157 486 CHAPTER 4 CONTINUOUSTIME SYSTEM ANALYSIS xt R C R R10 C R R yt R2 R26 Figure P4123 xt yt R2 C1 R1 C2 Figure P4124 4124 Design an order12 Butterworth lowpass filter with a cutoff frequency of ωc 2π5000 by completing the following a Locate and plot the filters poles and zeros in the complex plane Plot the correspond ing magnitude response HLPjω to verify proper design b Setting all resistor values to 100000 deter mine the capacitor values to implement the filter using a cascade of six secondorder SallenKey circuit sections The form of a SallenKey stage is shown in Fig P4124 On a single plot plot the magnitude response of each section as well as the over all magnitude response Identify the poles that correspond to each sections magnitude response curve Are the capacitor values realistic 4125 Rather than a Butterworth filter repeat Prob 4124 for a Chebyshev LPF with R 3 dB of passband ripple Since each SallenKey stage is constrained to have unity gain at dc an overall gain error of 1 1 ϵ2 is acceptable 4126 An analog lowpass filter with cutoff frequency ωc can be transformed into a highpass filter with cutoff frequency ωc by using an RCCR transformation rule each resistor Ri is replaced by a capacitor C i 1Riωc and each capacitor Ci is replaced by a resistor R i 1Ciωc Use this rule to design an order8 Butterworth highpass filter with ωc 2π4000 by completing the following a Design an order8 Butterworth lowpass filter with ωc 2π4000 by using four secondorder SallenKey circuit stages the form of which is shown in Fig P4124 Give resistor and capacitor values for each stage Choose the resistors so that the RCCR transformation will result in 1 nF capacitors At this point are the component values realistic b Draw an RCCR transformed SallenKey circuit stage Determine the transfer func tion Hs of the transformed stage in terms of the variables R 1 R 2 C 1 and C 2 c Transform the LPF designed in part a by using an RCCR transformation Give the resistor and capacitor values for each stage Are the component values realistic Using Hs derived in part b plot the magnitude response of each section as well 04LathiC04 2017925 1946 page 487 158 Problems 487 as the overall magnitude response Does the overall response look like a highpass Butterworth filter Plot the HPF system poles and zeros in the complex s plane How do these locations compare with those of the Butterworth LPF 4127 Repeat Prob 4126 using ωc 2π1500 and an order16 filter That is eight secondorder stages need to be designed 4128 Rather than a Butterworth filter repeat Prob 4126 for a Chebyshev LPF with R 3 dB of passband ripple Since each transformed SallenKey stage is constrained to have unity gain at ω an overall gain error of 1 1 ϵ2 is acceptable 4129 The MATLAB signalprocessing toolbox func tion butter helps design analog Butterworth filters Use MATLAB help to learn how butter works For each of the following cases design the filter plot the filters poles and zeros in the complex s plane and plot the decibel magnitude response 20log10 Hjω a Design a sixthorder analog lowpass filter with ωc 2π3500 b Design a sixthorder analog highpass filter with ωc 2π3500 c Design a sixthorder analog bandpass filter with a passband between 2 and 4 kHz d Design a sixthorder analog bandstop filter with a stopband between 2 and 4 kHz 41210 The MATLAB signalprocessing toolbox func tion cheby1 helps design analog Chebyshev type I filters A Chebyshev type I filter has a passband ripple and a smooth stopband Set ting the passband ripple to Rp 3 dB repeat Prob 4129 using the cheby1 command With all other parameters held constant what is the general effect of reducing Rp the allowable passband ripple 41211 The MATLAB signalprocessing toolbox func tion cheby2 helps design analog Chebyshev type II filters A Chebyshev type II filter has a smooth passband and ripple in the stopband Setting the stopband ripple Rs 20 dB down repeat Prob 4129 using the cheby2 command With all other parameters held constant what is the general effect of increasing Rs the minimum stopband attenuation 41212 The MATLAB signalprocessing toolbox func tion ellip helps design analog elliptic filters An elliptic filter has ripple in both the passband and the stopband Setting the passband ripple to Rp 3 dB and the stopband ripple Rs 20 dB down repeat Prob 4129 using the ellip command 41213 Using the definition CNx coshN cosh1x prove the recursive relation CNx 2xCN1x CN2x 41214 Prove that the poles of a Chebyshev filter which are located at pk ωc sinhξsinφk jωc coshξcosφk lie on an ellipse Hint The equation of an ellipse in the xy plane is xa2 yb2 1 where constants a and b define the major and minor axes of the ellipse 05LathiC05 2017925 1554 page 495 8 51 The zTransform 495 ANSWERS a Xz z5 z4 z3 z2 z 1 z9 or z z 1z4 z10 b z32z 172 z2 2z 2 n 1 4 5 6 7 8 9 0 xn Figure 53 Signal for Drill 51a 511 Inverse Transform by Partial Fraction Expansion and Tables As in the Laplace transform we shall avoid the integration in the complex plane required to find the inverse ztransform Eq 52 by using the unilateral transform table Table 51 Many of the transforms Xz of practical interest are rational functions ratio of polynomials in z which can be expressed as a sum of partial fractions whose inverse transforms can be readily found in a table of transform The partial fraction method works because for every transformable xn defined for n 0 there is a corresponding unique Xz defined for z r0 where r0 is some constant and vice versa EXAMPLE 53 Inverse zTransform by Partial Fraction Expansion Find the inverse ztransforms of a 8z 19 z 2z 3 b z2z2 11z 12 z 1z 23 c 2z3z 17 z 1z2 6z 25 a Expanding Xz into partial fractions yields Xz 8z 19 z 2z 3 3 z 2 5 z 3 05LathiC05 2017925 1554 page 510 23 510 CHAPTER 5 DISCRETETIME SYSTEM ANALYSIS USING THE ZTRANSFORM 53 zTRANSFORM SOLUTION OF LINEAR DIFFERENCE EQUATIONS The timeshifting leftshift or rightshift property has set the stage for solving linear difference equations with constant coefficients As in the case of the Laplace transform with differential equations the ztransform converts difference equations into algebraic equations that are readily solved to find the solution in the z domain Taking the inverse ztransform of the zdomain solution yields the desired timedomain solution The following examples demonstrate the procedure EXAMPLE 55 zTransform Solution of a Linear Difference Equation Solve yn 2 5yn 1 6yn 3xn 1 5xn if the initial conditions are y1 116 y2 3736 and the input xn 2nun As we shall see difference equations can be solved by using the rightshift or the left shift property Because the difference equation here is in advance form the use of the leftshift property in Eq 516 may seem appropriate for its solution Unfortunately this leftshift property requires a knowledge of auxiliary conditions y0 y1 yN 1 rather than of the initial conditions y1 y2 yn which are generally given This difficulty can be overcome by expressing the difference equation in delay form obtained by replacing n with n 2 and then using the rightshift property The resulting delayform difference equation is yn 5yn 1 6yn 2 3xn 1 5xn 2 521 We now use the rightshift property to take the ztransform of this equation But before proceeding we must be clear about the meaning of a term like yn 1 here Does it mean yn 1un 1 or yn 1un In any equation we must have some time reference n 0 and every term is referenced from this instant Hence ynk means ynkun Remember also that although we are considering the situation for n 0 yn is present even before n 0 in the form of initial conditions Now ynun Yz yn 1un 1 z Yz y1 1 z Yz 11 6 yn 2un 1 z2 Yz 1 z y1 y2 1 z2 Yz 11 6z 37 36 Noting that for causal input xn x1 x2 xn 0 Another approach is to find y0 y1 y2 yN 1 from y1 y2 yn iteratively as in Sec 351 and then apply the leftshift property to the advanceform difference equation 05LathiC05 2017925 1554 page 516 29 516 CHAPTER 5 DISCRETETIME SYSTEM ANALYSIS USING THE ZTRANSFORM Figure 56 The transformed representation of an LTID system representing all signals by their ztransforms and all system components or elements by their transfer functions as shown in Fig 56b The result Yz HzXz greatly facilitates derivation of the system response to a given input We shall demonstrate this assertion by an example EXAMPLE 56 Transfer Function to Find the ZeroState Response Find the response yn of an LTID system described by the difference equation yn 2 yn 1 016yn xn 1 032xn or E2 E 016yn E 032xn for the input xn 2nun and with all the initial conditions zero system in the zero state From the difference equation we find Hz Pz Qz z 032 z2 z 016 For the input xn 2nun 21nun 05nun Xz z z 05 and Yz XzHz zz 032 z2 z 016z 05 05LathiC05 2017925 1554 page 519 32 54 System Realization 519 3 An LTID system is marginally stable if and only if there are no poles of Hz outside the unit circle and there are some simple poles on the unit circle DRILL 514 Transfer Function to Determine Stability Show that an accumulator whose impulse response is hn un is marginally stable but BIBOunstable 533 Inverse Systems If Hz is the transfer function of a system S then Si its inverse system has a transfer function Hiz given by Hiz 1 Hz This follows from the fact the inverse system Si undoes the operation of S Hence if Hz is placed in cascade with Hiz the transfer function of the composite system identity system is unity For example an accumulator whose transfer function is Hz zz 1 and a backward difference system whose transfer function is Hiz z 1z are inverse of each other Similarly if Hz z 04 z 07 its inverse system transfer function is Hiz z 07 z 04 as required by the property HzHiz 1 Hence it follows that hn hin δn DRILL 515 Inverse Systems Find the impulse responses of an accumulator and a firstorder backward difference system Show that the convolution of the two impulse responses yields δn 54 SYSTEM REALIZATION Because of the similarity between LTIC and LTID systems conventions for block diagrams and rules of interconnection for LTID are identical to those for continuoustime LTIC systems It is not necessary to rederive these relationships We shall merely restate them to refresh the readers memory 05LathiC05 2017925 1554 page 521 34 54 System Realization 521 system is H1z H2z For a feedback system as in Fig 418d the transfer function is Gz1 GzHz We now consider a systematic method for realization or simulation of an arbitrary Nthorder LTID transfer function Since realization is basically a synthesis problem there is no unique way of realizing a system A given transfer function can be realized in many different ways We present here the two forms of direct realization Each of these forms can be executed in several other ways such as cascade and parallel Furthermore a system can be realized by the transposed version of any known realization of that system This artifice doubles the number of system realizations A transfer function Hz can be realized by using time delays along with adders and multipliers We shall consider a realization of a general Nthorder causal LTID system whose transfer function is given by Hz b0zN b1zN1 bN1z bN zN a1zN1 aN1z aN 529 This equation is identical to the transfer function of a general Nthorder proper LTIC system given in Eq 436 The only difference is that the variable z in the former is replaced by the variable s in the latter Hence the procedure for realizing an LTID transfer function is identical to that for the LTIC transfer function with the basic element 1s integrator replaced by the element 1z unit delay The reader is encouraged to follow the steps in Sec 46 and rederive the results for the LTID transfer function in Eq 529 Here we shall merely reproduce the realizations from Sec 46 with integrators 1s replaced by unit delays 1z The direct form I DFI is shown in Fig 58a the canonic direct form DFII is shown in Fig 58b and the transpose of canonic direct is shown in Fig 58c The DFII and its transpose are canonic because they require N delays which is the minimum number needed to implement the Nthorder LTID transfer function in Eq 529 In contrast the form DFI is a noncanonic because it generally requires 2N delays The DFII realization in Fig 58b is also called a canonic direct form EXAMPLE 58 Canonical Realizations of Transfer Functions Find the canonic direct and the transposed canonic direct realizations of the following transfer functions a 2 z 5 b 4z 28 z 1 c z z 7 and d 4z 28 z2 6z 5 All four of these transfer functions are special cases of Hz in Eq 529 a Hz 2 z 5 For this case the transfer function is of the first order N 1 therefore we need only one delay for its realization The feedback and feedforward coefficients are a1 5 and b0 0 b1 2 05LathiC05 2017925 1554 page 547 60 57 Digital Processing of Analog Signals 547 DRILL 521 Highpass Filter by PoleZero Placement Use the graphical argument to show that a filter with transfer function Hz z 09 z acts like a highpass filter Make a rough sketch of the amplitude response 57 DIGITAL PROCESSING OF ANALOG SIGNALS An analog meaning continuoustime signal can be processed digitally by sampling the analog signal and processing the samples by a digital meaning discretetime processor The output of the processor is then converted back to analog signal as shown in Fig 524a We saw some simple cases of such processing in Exs 38 39 514 and 515 In this section we shall derive a criterion for designing such a digital processor for a general LTIC system Suppose that we wish to realize an equivalent of an analog system with transfer function Has shown in Fig 524b Let the digital processor transfer function in Fig 524a that realizes this desired Has be Hz In other words we wish to make the two systems in Fig 524 equivalent at least approximately By equivalence we mean that for a given input xt the systems in Fig 524 yield the same output yt Therefore ynT the samples of the output in Fig 524b are identical to yn the output of Hz in Fig 524a xn xt yn yt xt yt Continuous to discrete CD Discretetime system Hz Discrete to continuous DC a a b Has b Has Figure 524 Analog filter realization with a digital filter 05LathiC05 2017925 1554 page 573 86 510 MATLAB DiscreteTime IIR Filters 573 1 05 0 05 1 Real 1 05 0 05 1 Imag Figure 532 Polezero plot computed by using roots CH5MP5m Chapter 5 MATLAB Program 5 Script Mfile designs a 180thorder Butterworth lowpass discretetime filter with cutoff Omegac 06pi using 90 cascaded secondorder filter sections omega0 1 Use normalized cutoff frequency for analog prototype psi 05190pi180 Butterworth pole angles Omegac 06pi Discretetime cutoff frequency Omega linspace0pi1000 Frequency range for magnitude response Hmag zeros901000 p zeros1180 z zeros1180 Preallocation for stage 190 Q 12cospsistage Compute Q for stage B omega02 A 1 omega0Q omega02 Compute stage coefficients B1A1 CH5MP4BA2omega0tan06pi2 Transform stage to DT pstage21stage2 rootsA1 Compute zdomain poles for stage zstage21stage2 rootsB1 Compute zdomain zeros for stage Hmagstage absCH5MP1B1A1Omega Compute stage mag response end ucirc expjlinspace02pi200 Compute unit circle for polezero plot figure plotrealpimagpkxrealzimagzokrealucircimagucirck axis equal xlabelReal ylabelImag figure plotOmegaprodHmagk axis0 pi 005 105 xlabelOmega rad ylabelMagnitude Response The figure command preceding each plot command opens a separate window for each plot The filters polezero plot is shown in Fig 533 along with the unit circle for reference All 180 zeros of the cascaded design are properly located at minus 1 The wall of poles provides an amazing approximation to the desired brickwall response as shown by the magnitude response in Fig 534 It is virtually impossible to realize such highorder designs with continuoustime filters which adds another reason for the popularity of discretetime filters Still the design is not 05LathiC05 2017925 1554 page 574 87 574 CHAPTER 5 DISCRETETIME SYSTEM ANALYSIS USING THE ZTRANSFORM 1 05 0 05 1 Real 08 06 04 02 0 02 04 06 08 Imag Figure 533 Polezero plot for 180thorder discretetime Butterworth filter 0 05 1 15 2 25 3 Ω rad 0 02 04 06 08 1 Magnitude Response Figure 534 Magnitude response for a 180thorder discretetime Butterworth filter trivial even functions from the MATLAB signalprocessing toolbox fail to properly design such a highorder discretetime Butterworth filter 511 SUMMARY In this chapter we discussed the analysis of linear timeinvariant discretetime LTID systems by means of the ztransform The ztransform changes the difference equations of LTID systems into algebraic equations Therefore solving these difference equations reduces to solving algebraic equations The transfer function Hz of an LTID system is equal to the ratio of the ztransform of the output to the ztransform of the input when all initial conditions are zero Therefore if Xz is the ztransform of the input xn and Yz is the ztransform of the corresponding output yn 05LathiC05 2017925 1554 page 575 88 Problems 575 when all initial conditions are zero then Yz HzXz For an LTID system specified by the difference equation QEyn PExn the transfer function Hz PzQz Moreover Hz is the ztransform of the system impulse response hn We showed in Ch 3 that the system response to an everlasting exponential zn is Hzzn We may also view the ztransform as a tool that expresses a signal xn as a sum of exponentials of the form zn over a continuum of the values of z Using the fact that an LTID system response to zn is Hzzn we find the system response to xn as a sum of the systems responses to all the components of the form zn over the continuum of values of z LTID systems can be realized by scalar multipliers adders and time delays A given transfer function can be synthesized in many different ways We discussed canonical transposed canonical cascade and parallel forms of realization The realization procedure is identical to that for continuoustime systems with 1s integrator replaced by 1z unit delay The majority of the input signals and practical systems are causal Consequently we are required to deal with causal signals most of the time Restricting all signals to the causal type greatly simplifies ztransform analysis the ROC of a signal becomes irrelevant to the analysis process This special case of ztransform which is restricted to causal signals is called the unilateral ztransform Much of the chapter deals with this transform Section 58 discusses the general variety of the ztransform bilateral ztransform which can handle causal and noncausal signals and systems In the bilateral transform the inverse transform of Xz is not unique but depends on the ROC of Xz Thus the ROC plays a crucial role in the bilateral ztransform In Sec 59 we showed that discretetime systems can be analyzed by the Laplace transform as if they were continuoustime systems In fact we showed that the ztransform is the Laplace transform with a change in variable REFERENCES 1 Lyons R G Understanding Digital Signal Processing AddisonWesley Reading MA 1997 2 Oppenheim A V and R W Schafer DiscreteTime Signal Processing 2nd ed PrenticeHall Upper Saddle River NJ 1999 3 Mitra S K Digital Signal Processing 2nd ed McGrawHill New York 2001 PROBLEMS 511 Using the definition compute the ztransform of xn 1nun un 8 Sketch the poles and zeros of Xz in the z plane No calculator is needed to do this problem 512 Determine the unilateral ztransform Xz of the signal xn shown in Fig P512 As the picture suggests xn 3 for all n 9 and xn 0 for all n 3 513 a A causal signal has ztransform given by Xz z2 z31 Determine the timedomain signal xn and sketch xn over 4 n 11 Hint No complex arithmetic is needed to solve this problem yn n 3 1 1 3 15 10 5 5 Figure P512 05LathiC05 2017925 1554 page 579 92 Problems 579 a Use transformdomain techniques to determine the zerostate response yzsrn to input xn 3un 5 b Use transformdomain techniques to determine the zeroinput response yzirn given yzir2 yzir1 1 537 a Find the output yn of an LTID system specified by the equation 2yn 2 3yn 1 yn 4xn 2 3xn 1 for input xn 4nun and initial conditions y1 0 and y2 1 b Find the zeroinput and the zerostate components of the response c Find the transient and the steadystate components of the response 538 Solve Prob 537 if initial conditions y1 and y2 are instead replaced with auxiliary conditions y0 32 and y1 354 539 a Solve 4yn 2 4yn 1 yn xn 1 with y1 0 y2 1 and xn un b Find the zeroinput and the zerostate components of the response c Find the transient and the steadystate components of the response 5310 Solve yn 2 3yn 1 2yn xn 1 if y1 2 y2 3 and xn 3nun 5311 Solve yn 2 2yn 1 2yn xn with y1 1 y2 0 and xn un 5312 Consider a causal LTID system described as Hz 21z21 16z2 1 4 z 3 8 a Determine the standard delayform differ ence equation description of this system b Using transformdomain techniques deter mine the system impulse response hn c Using transformdomain techniques determine yzirn given y1 16 and y2 8 5313 Consider a causal LTID system described as yn 5 6yn 1 1 6yn 2 3 2xn 1 3 2xn 2 a Determine the standardform system transfer function Hz and sketch the system polezero plot b Using transformdomain techniques determine yzirn given y1 2 and y2 2 5314 Solve yn2yn12yn2 xn12xn2 with y0 0 y1 1 and xn enun 5315 A system with impulse response hn 213nun 1 produces an output yn 2nun 1 Determine the corresponding input xn 5316 A professor recently received an unexpected 10 a futile bribe attached to a test Being the savvy investor that she is the professor decides to invest the 10 into a savings account that earns 05 interest compounded monthly 617 APY Furthermore she decides to supplement this initial investment with an additional 5 deposit made every month beginning the month immediately following her initial investment a Model the professors savings account as a constant coefficient linear difference equation Designate yn as the account balance at month n where n 0 corresponds to the first month that interest is awarded and that her 5 deposits begin b Determine a closedform solution for yn That is you should express yn as a function only of n c If we consider the professors bank account as a system what is the system impulse response hn What is the system transfer function Hz d Explain this fact if the input to the professors bank account is the everlasting exponential xn 1n 1 then the output is not yn 1nH1 H1 5317 Sally deposits 100 into her savings account on the first day of every month except for each December when she uses her money to buy 05LathiC05 2017925 1554 page 592 105 592 CHAPTER 5 DISCRETETIME SYSTEM ANALYSIS USING THE ZTRANSFORM type I filters A Chebyshev type I filter has passband ripple and smooth stopband Setting the passband ripple to Rp 3 dB repeat Prob 51012 using the cheby1 command With all other parameters held constant what is the general effect of reducing Rp the allowable passband ripple 51014 The MATLAB signalprocessing toolbox function cheby2 helps design digital Chebyshev type II filters A Chebyshev type II filter has smooth passband and ripple in the stopband Setting the stopband ripple Rs 20 dB down repeat Prob 51012 using the cheby2 command With all other parameters held constant what is the general effect of increasing Rs the minimum stopband attenuation 51015 The MATLAB signalprocessing toolbox function ellip helps design digital elliptic filters An elliptic filter has ripple in both the passband and the stopband Setting the passband ripple to Rp 3 dB and the stopband ripple Rs 20 dB down repeat Prob 51012 using the ellip command 06LathiC06 2017925 1554 page 593 1 C H A P T E R CONTINUOUSTIME SIGNAL ANALYSIS THE FOURIER SERIES 6 Electrical engineers instinctively think of signals in terms of their frequency spectra and think of systems in terms of their frequency response Most teenagers know about the audible portion of audio signals having a bandwidth of about 20 kHz and the need for goodquality speakers to respond up to 20 kHz This is basically thinking in the frequency domain In Chs 4 and 5 we discussed extensively the frequencydomain representation of systems and their spectral response system response to signals of various frequencies In Chs 6 through 9 we discuss spectral representation of signals where signals are expressed as a sum of sinusoids or exponentials Actually we touched on this topic in Chs 4 and 5 Recall that the Laplace transform of a continuoustime signal is its spectral representation in terms of exponentials or sinusoids of complex frequencies Similarly the ztransform of a discretetime signal is its spectral representation in terms of discretetime exponentials However in the earlier chapters we were concerned mainly with system representation the spectral representation of signals was incidental to the system analysis Spectral analysis of signals is an important topic in its own right and now we turn to this subject In this chapter we show that a periodic signal can be represented as a sum of sinusoids or exponentials of various frequencies These results are extended to aperiodic signals in Ch 7 and to discretetime signals in Ch 9 The fascinating subject of sampling of continuoustime signals is discussed in Ch 8 leading to AD analogtodigital and DA conversion Chapter 8 forms the bridge between the continuoustime and the discretetime worlds 61 PERIODIC SIGNAL REPRESENTATION BY TRIGONOMETRIC FOURIER SERIES As seen in Sec 133 Eq 17 a periodic signal xt with period T0 Fig 61 has the property xt xt T0 for all t The smallest value of T0 that satisfies this periodicity condition is the fundamental period of xt As argued in Sec 133 this equation implies that xt starts at and continues to Moreover the area under a periodic signal xt over any interval of duration T0 is the same that is for any 593 06LathiC06 2017925 1554 page 601 9 61 Periodic Signal Representation by Trigonometric Fourier Series 601 PLOTTING FOURIER SERIES SPECTRA USING MATLAB MATLAB is well suited to compute and plot Fourier series spectra The results in Fig 63 which plot Cn and θn as functions of n match Figs 62b and 62c which plot Cn and θn as functions of ω nω0 2n Plots of an and bn are similarly simple to generate n 010 thetan atan4n Cnn0 0504 Cnn0 05042sqrt116nn02 subplot121 stemnCnk axis5 105 0 6 xlabeln ylabelCn subplot122 stemnthetank axis5 105 16 0 xlabeln ylabel hetan 10 n 0 02 04 06 Cn 0 2 4 6 8 0 2 4 6 8 10 n 15 1 05 0 θn Figure 63 Fourier Series spectra for Ex 61 using MATLAB The amplitude and phase spectra for xt in Figs 62b and 62c tell us at a glance the frequency composition of xt that is the amplitudes and phases of various sinusoidal components of xt Knowing the frequency spectra we can reconstruct xt as shown on the righthand side of Eq 611 Therefore the frequency spectra Figs 62b 62c provide an alternative descriptionthe frequencydomain description of xt The timedomain description of xt is shown in Fig 62a A signal therefore has a dual identity the timedomain identity xt and the frequencydomain identity Fourier spectra The two identities complement each other taken together they provide a better understanding of a signal An interesting aspect of Fourier series is that whenever there is a jump discontinuity in xt the series at the point of discontinuity converges to an average of the lefthand and righthand limits of xt at the instant of discontinuity In the present example for instance xt is discontinuous at t 0 with x0 1 and x0 xπ eπ2 0208 The corresponding Fourier series converges to a value 1 02082 0604 at t 0 This is easily verified from Eq 611 by setting t 0 This behavior of the Fourier series is dictated by its convergence in the mean discussed later in Secs 62 and 65 06LathiC06 2017925 1554 page 611 19 61 Periodic Signal Representation by Trigonometric Fourier Series 611 JeanBaptisteJoseph Fourier and Napoleon Napoleon was the first modern ruler with a scientific education and he was one of the rare persons who are equally comfortable with soldiers and scientists The age of Napoleon was one of the most fruitful in the history of science Napoleon liked to sign himself as member of Institut de France a fraternity of scientists and he once expressed to Laplace his regret that force of circumstances has led me so far from the career of a scientist 2 Many great figures in science and mathematics including Fourier and Laplace were honored and promoted by Napoleon In 1798 he took a group of scientists artists and scholarsFourier among themon his Egyptian expedition with the promise of an exciting and historic union of adventure and research Fourier proved to be a capable administrator of the newly formed Institut dÉgypte which incidentally was responsible for the discovery of the Rosetta Stone The inscription on this stone in two languages and three scripts hieroglyphic demotic and Greek enabled Thomas Young and JeanFrançois Champollion a protégé of Fourier to invent a method of translating hieroglyphic writings of ancient Egyptthe only significant result of Napoleons Egyptian expedition Back in France in 1801 Fourier briefly served in his former position as professor of mathematics at the École Polytechnique in Paris In 1802 Napoleon appointed him the prefect of Isère with its headquarters in Grenoble a position in which Fourier served with distinction Fourier was named Baron of the Empire by Napoleon in 1809 Later when Napoleon was exiled to Elba his route was to take him through Grenoble Fourier had the route changed to avoid meeting Napoleon which would have displeased Fouriers new master King Louis XVIII Within a year Napoleon escaped from Elba and returned to France At Grenoble Fourier was brought before him in chains Napoleon scolded Fourier for his ungrateful behavior but reappointed him the prefect of Rhône at Lyons Within four months Napoleon was defeated at Waterloo and was exiled to St Helena where he died in 1821 Fourier once again was in disgrace as a Bonapartist and had 06LathiC06 2017925 1554 page 617 25 62 Existence and Convergence of the Fourier Series 617 EXAMPLE 65 SquareWave Synthesis by Truncated Fourier Series Using MATLAB Use MATLAB to synthesize and plot the square wave of Fig 68a using a Fourier series that is truncated to the 19th harmonic The result should match Fig 68e To synthesize the waveform we use the Fourier series of Eq 613 x t 10modtpi22pipi t linspace2pi2pi10001 x19 05onessizet for n119 x19 x192pinsinpin2cosnt end plottx19k axis2pi 2pi 02 12 xlabelt ylabelx19t As expected the result of Fig 69 matches Fig 68e 6 4 2 0 2 4 6 t 0 05 1 x19t Figure 69 Using MATLAB to synthesize a square wave via truncated Fourier series PHASE SPECTRUM THE WOMAN BEHIND A SUCCESSFUL MAN The role of the amplitude spectrum in shaping the waveform xt is quite clear However the role of the phase spectrum in shaping this waveform is less obvious Yet the phase spectrum like the woman behind a successful man plays an equally important role in waveshaping We can explain this role by considering a signal xt that has rapid changes such as jump discontinuities To synthesize an instantaneous change at a jump discontinuity the phases of the various sinusoidal components in its spectrum must be such that all or most of the harmonic components will have Or to keep up with the times the man behind a successful woman 06LathiC06 2017925 1554 page 619 27 62 Existence and Convergence of the Fourier Series 619 FOURIER SYNTHESIS OF DISCONTINUOUS FUNCTIONS THE GIBBS PHENOMENON Figure 68 showed the square function xt and its approximation by a truncated trigonometric Fourier series that includes only the first N harmonics for N 1 3 5 and 19 The plot of the truncated series approximates closely the function xt as N increases and we expect that the series will converge exactly to xt as N Yet the curious fact as seen from Fig 68 is that even for large N the truncated series exhibits an oscillatory behavior and an overshoot approaching a value of about 9 in the vicinity of the discontinuity at the nearest peak of oscillation Regardless of the value of N the overshoot remains at about 9 Such strange behavior certainly would undermine anyones faith in the Fourier series In fact this behavior puzzled many scholars at the turn of the century Josiah Willard Gibbs an eminent mathematical physicist who was the inventor of vector analysis gave a mathematical explanation of this behavior now called the Gibbs phenomenon We can reconcile the apparent aberration in the behavior of the Fourier series by observing from Fig 68 that the frequency of oscillation of the synthesized signal is Nf0 so the width of the spike with 9 overshoot is approximately 12Nf0 As we increase N the frequency of oscillation increases and the spike width 12Nf0 diminishes As N the error power 0 because the error consists mostly of the spikes whose widths 0 Therefore as N the corresponding Fourier series differs from xt by about 9 at the immediate left and right of the points of discontinuity and yet the error power 0 The reason for all this confusion is that in this case the Fourier series converges in the mean When this happens all we promise is that the error energy over one period 0 as N Thus the series may differ from xt at some points and yet have the error signal power zero as verified earlier Note that the series in this case also converges pointwise at all points except the points of discontinuity It is precisely at the discontinuities that the series differs from xt by 9 When we use only the first N terms in the Fourier series to synthesize a signal we are abruptly terminating the series giving a unit weight to the first N harmonics and zero weight to all the remaining harmonics beyond N This abrupt termination of the series causes the Gibbs phenomenon in synthesis of discontinuous functions Section 78 offers more discussion on the Gibbs phenomenon its ramifications and cure The Gibbs phenomenon is present only when there is a jump discontinuity in xt When a continuous function xt is synthesized by using the first N terms of the Fourier series the synthesized function approaches xt for all t as N No Gibbs phenomenon appears This can be seen in Fig 611 which shows one cycle of a continuous periodic signal being synthesized from the first 19 harmonics Compare the similar situation for a discontinuous signal in Fig 68 DRILL 63 Rate of Spectral Decay By inspection of signals in Figs 62a 67a and 67b determine the asymptotic rate of decay of their amplitude spectra There is also an undershoot of 9 at the other side at t π2 of the discontinuity Actually at discontinuities the series converges to a value midway between the values on either side of the discontinuity The 9 overshoot occurs at t π2 and 9 undershoot occurs at t π2 06LathiC06 2017925 1554 page 620 28 620 CHAPTER 6 CONTINUOUSTIME SIGNAL ANALYSIS THE FOURIER SERIES 0 t 1 Figure 611 Fourier synthesis of a continuous signal using first 19 harmonics ANSWERS 1n1n2 and 1n respectively A HISTORICAL NOTE ON THE GIBBS PHENOMENON Normally speaking troublesome functions with strange behavior are invented by mathematicians we rarely see such oddities in practice In the case of the Gibbs phenomenon however the tables were turned A rather puzzling behavior was observed in a mundane object a mechanical wave synthesizer and then wellknown mathematicians of the day were dispatched on the scent of it to discover its hideout Albert Michelson of MichelsonMorley fame was an intense practical man who developed ingenious physical instruments of extraordinary precision mostly in the field of optics His harmonic analyzer developed in 1898 could compute the first 80 coefficients of the Fourier series of a signal xt specified by any graphical description The instrument could also be used as a harmonic synthesizer which could plot a function xt generated by summing the first 80 harmonics Fourier components of arbitrary amplitudes and phases This analyzer therefore had the ability of selfchecking its operation by analyzing a signal xt and then adding the resulting 80 components to see whether the sum yielded a close approximation of xt Michelson found that the instrument checked very well with most of signals analyzed However when he tried a discontinuous function such as a square wave a curious behavior was observed The sum of 80 components showed oscillatory behavior ringing with an overshoot of 9 in the vicinity of the points of discontinuity Moreover this behavior was a constant feature regardless of the number of terms added A larger number of terms made the oscillations proportionately faster but regardless of the number of terms added the overshoot remained 9 This puzzling behavior caused Michelson to suspect some mechanical defect in his synthesizer He wrote about his observation in a letter to Nature December 1898 Josiah Willard Gibbs who was a professor at Yale investigated and clarified this behavior for a sawtooth periodic signal in a letter to Nature 7 Later in 1906 Bôcher generalized the result for any function with discontinuity 8 Actually it was a periodic sawtooth signal 06LathiC06 2017925 1554 page 626 34 626 CHAPTER 6 CONTINUOUSTIME SIGNAL ANALYSIS THE FOURIER SERIES 5 n 0 02 04 06 Dn 5 0 5 0 5 n 2 0 2 Dn rad Figure 613 Exponential Fourier series spectra for Ex 67 WHAT IS A NEGATIVE FREQUENCY The existence of the spectrum at negative frequencies is somewhat disturbing because by definition the frequency number of repetitions per second is a positive quantity How do we interpret a negative frequency We can use a trigonometric identity to express a sinusoid of a negative frequency ω0 as cosω0t θ cosω0t θ This equation clearly shows that the frequency of a sinusoid cosω0t θ is ω0 which is a positive quantity The same conclusion is reached by observing that ejω0t cos ω0t jsin ω0t Thus the frequency of exponentials ejω0t is indeed ω0 How do we then interpret the spectral plots for negative values of ω A more satisfying way of looking at the situation is to say that exponential spectra are a graphical representation of coefficients Dn as a function of ω Existence of the spectrum at ω nω0 is merely an indication that an exponential component ejnω0t exists in the series We know that a sinusoid of frequency nω0 can be expressed in terms of a pair of exponentials ejnω0t and ejnω0t We see a close connection between the exponential spectra in Fig 612 and the spectra of the corresponding trigonometric Fourier series for xt Figs 62b 62c Equation 622 explains the reason for the close connection for real xt between the trigonometric spectra Cn and θn with exponential spectra Dn and Dn The dc components D0 and C0 are identical in both spectra Moreover the exponential amplitude spectrum Dn is half the trigonometric amplitude spectrum Cn for n 1 The exponential angle spectrum Dn is identical to the trigonometric phase spectrum θn for n 0 We can therefore produce the exponential spectra merely by inspection of trigonometric spectra and vice versa The following example demonstrates this feature 06LathiC06 2017925 1554 page 635 43 63 Exponential Fourier Series 635 633 Properties of the Fourier Series As with the Laplace and ztransforms the Fourier series has a variety of properties that can simplify work and help provide a more intuitive understanding of signals Table 62 provides the most important properties of the Fourier series for a periodic signal xt and its spectrum Dn Properties that involve two signals require that the two signals have a common fundamental frequency ω0 While not given here the proofs of these properties are straightforward and parallel the proofs of the Fourier transform properties given in Ch 7 To demonstrate the utility of Fourier series properties let us consider an example where we use a selection of properties to simplify the work of finding a piecewise polynomial signals spectrum TABLE 62 Selected Fourier Series Properties Operation xt Dn Scalar multiplication kxt kDn Addition x1t x2t D1n D2n x1t x2t require same ω0 Conjugation xt D n Reversal xt Dn Time shifting xt t0 Dnejnω0t0 Frequency shifting xtejn0ω0t Dnn0 Frequency convolution x1tx2t D1n D2n x1t x2t require same ω0 Time differentiation dkxt dtk jnω0kDn EXAMPLE 611 Using Fourier Series Properties Use properties rather than integration to compute the exponential Fourier series coefficients Dn of the triangular signal xt shown in Fig 64 Verify the correctness of Dn for A 1 by synthesizing xt with a suitable truncation of Eq 619 From Fig 64 we see that xt is a piecewise linear function that is T0 2 periodic To compute Dn directly using Eq 619 would therefore require tedious integration by parts Fortunately we can compute Dn without integration by instead using Fourier series properties First however we must compute the dc component D0 separately from other Dn By simple inspection of Fig 64 we see that xt has no dc component so D0 0 To determine the remaining Dn we begin by noting that xt has a constant slope of either 2A or 2A Thus differentiating xt once yields a square wave with amplitudes 2A Here differentiation reduces xt from a piecewise linear to a piecewise constant function 06LathiC06 2017925 1554 page 641 49 65 Generalized Fourier Series Signals as Vectors 641 DUAL PERSONALITY OF A SIGNAL The discussion so far shows that a periodic signal has a dual personalitythe time domain and the frequency domain It can be described by its waveform or by its Fourier spectra The time and frequencydomain descriptions provide complementary insights into a signal For indepth perspective we need to understand both these identities It is important to learn to think of a signal from both perspectives In the next chapter we shall see that aperiodic signals also have this dual personality Moreover we shall show that even LTI systems have this dual personality which offers complementary insights into the system behavior LIMITATIONS OF THE FOURIER SERIES METHOD OF ANALYSIS We have developed here a method of representing a periodic signal as a weighted sum of everlasting exponentials whose frequencies lie along the ω axis in the s plane This representation Fourier series is valuable in many applications However as a tool for analyzing linear systems it has serious limitations and consequently has limited utility for the following reasons 1 The Fourier series can be used only for periodic inputs All practical inputs are aperiodic remember that a periodic signal starts at t 2 The Fourier methods can be applied readily to BIBOstable or asymptotically stable systems It cannot handle unstable or even marginally stable systems The first limitation can be overcome by representing aperiodic signals in terms of everlasting exponentials This representation can be achieved through the Fourier integral which may be considered to be an extension of the Fourier series We shall therefore use the Fourier series as a steppingstone to the Fourier integral developed in the next chapter The second limitation can be overcome by using exponentials est where s is not restricted to the imaginary axis but is free to take on complex values This generalization leads to the Laplace integral discussed in Ch 4 the Laplace transform 65 GENERALIZED FOURIER SERIES SIGNALS AS VECTORS We now consider a very general approach to signal representation with farreaching consequences There is a perfect analogy between signals and vectors the analogy is so strong that the term analogy understates the reality Signals are not just like vectors Signals are vectors A vector can be represented as a sum of its components in a variety of ways depending on the choice of coordinate system A signal can also be represented as a sum of its components in a variety of ways Let us begin with some basic vector concepts and then apply these concepts to signals This section closely follows the material from the authors earlier book 10 Omission of this section will not cause any discontinuity in understanding the rest of the book Derivation of Fourier series through the signalvector analogy provides an interesting insight into signal representation and other topics such as signal correlation data truncation and signal detection 06LathiC06 2017925 1554 page 642 50 642 CHAPTER 6 CONTINUOUSTIME SIGNAL ANALYSIS THE FOURIER SERIES 651 Component of a Vector A vector is specified by its magnitude and its direction We shall denote all vectors by boldface For example x is a certain vector with magnitude or length x For the two vectors x and y shown in Fig 621 we define their dot inner or scalar product as x y xycos θ where θ is the angle between these vectors Using this definition we can express x the length of a vector x as x2 x x Let the component of x along y be cy as depicted in Fig 621 Geometrically the component of x along y is the projection of x on y and is obtained by drawing a perpendicular from the tip of x on the vector y as illustrated in Fig 621 What is the mathematical significance of a component of a vector along another vector As seen from Fig 621 the vector x can be expressed in terms of vector y as x cy e However this is not the only way to express x in terms of y From Fig 622 which shows two of the infinite other possibilities we have x c1y e1 c2y e2 In each of these three representations x is represented in terms of y plus another vector called the error vector If we approximate x by cy x cy the error in the approximation is the vector e x cy Similarly the errors in approximations in these drawings are e1 Fig 622a and e2 Fig 622b What is unique about the approximation in Fig 621 is that the error vector is the smallest We can now define mathematically the component of a vector x along vector y to be cy where c is chosen to minimize the length of the error vector e x cy Now the length of the component of x along y is xcosθ But it is also cy as seen from Fig 621 Therefore cy xcosθ Multiplying both sides by y yields cy2 xycos θ x y x cy y e u Figure 621 Component projection of a vector along another vector 06LathiC06 2017925 1554 page 660 68 660 CHAPTER 6 CONTINUOUSTIME SIGNAL ANALYSIS THE FOURIER SERIES this purpose we need samples of xt over one period starting at t 0 In this algorithm it is also preferable although not necessary that N0 be a power of 2 ie N0 2m where m is an integer EXAMPLE 616 Numerical Computation of Fourier Spectra Numerically compute and then plot the exponential Fourier spectra for the periodic signal in Fig 62a Ex 61 The samples of xt start at t 0 and the last N0th sample is at t T0 T At the points of discontinuity the sample value is taken as the average of the values of the function on two sides of the discontinuity Thus the sample at t 0 is not 1 but eπ2 12 0604 To determine N0 we require that Dn for n N02 be negligible Because xt has a jump discontinuity Dn decays rather slowly as 1n Hence a choice of N0 200 is acceptable because the N02nd 100th harmonic is about 1 of the fundamental However we also require N0 to be a power of 2 Hence we shall take N0 256 28 First the basic parameters are established T0 pi N0 256 T T0N0 t 0TTN01 x expt2 x1 exppi212 Next the DFT computed by means of the fft function is used to approximate the exponential Fourier spectra up to n N02 To facilitate comparison with previous plots of Dn we only plot the results over 5 n 5 Dn fftxN0 n N02N021 clf subplot121 stemnabsfftshiftDnk axis5 5 0 6 xlabeln ylabelDn subplot122 stemnanglefftshiftDnk axis5 5 2 2 xlabeln ylabelangle Dn rad As shown in Fig 628 the resulting approximation is visually indistinguishable from the true Fourier series spectra shown in Fig 612 or Fig 613 n 0 02 04 06 Dn 5 5 0 5 0 5 n 2 0 2 Dn rad Figure 628 Numerical approximation of exponential Fourier series spectra using the DFT 06LathiC06 2017925 1554 page 662 70 662 CHAPTER 6 CONTINUOUSTIME SIGNAL ANALYSIS THE FOURIER SERIES t time vector for xN Define FS coefficients for signal xt D n 12pinexp1jnA1nA 1jexp1jnpi Construct truncated FS approximation of xt using N harmonics t linspacepi42pipi410000 Time vector exceeds one period xN 2piA4pionessizet Compute dc term for n 1N Compute N remaining terms xN xNrealDnexp1jnt conjDnexp1jnt end Although theoretically not required the real command ensures that small computer roundoff errors do not cause a complexvalued result Using program CH6MP1 with A π2 and N 20 Fig 629 compares xt and x20t A pi2 x20t CH6MP1A20 plottx20ktxtAk axispi42pipi40111 xlabelt ylabelx20t As expected the falling edge is accompanied by the overshoot that is characteristic of the Gibbs phenomenon Increasing N to 100 as shown in Fig 630 improves the approximation but does not reduce the overshoot x100t CH6MP1A100 plottx100ktxtAk axispi42pipi40111 xlabelt ylabelx100t Reducing A to π64 produces a curious result For N 20 both the rising and falling edges are accompanied by roughly 9 of overshoot as shown in Fig 631 As the number of terms is increased overshoot persists only in the vicinity of jump discontinuities For xNt increasing N decreases the overshoot near the rising edge but not near the falling edge Remember that it is a 0 1 2 3 4 5 6 7 t 0 02 04 06 08 1 x20t Figure 629 Comparison of x20t and xt when A π2 06LathiC06 2017925 1554 page 663 71 67 MATLAB Fourier Series Applications 663 0 1 2 3 4 5 6 7 t 0 02 04 06 08 1 x100 t Figure 630 Comparison of x100t and xt when A π2 0 1 2 3 4 5 6 7 t 0 02 04 06 08 1 x20t Figure 631 Comparison of x20t and xt when A π64 0 1 2 3 4 5 6 7 t 0 02 04 06 08 1 x100 t Figure 632 Comparison of x100t and xt when A π64 true jump discontinuity that causes the Gibbs phenomenon A continuous signal no matter how sharply it rises can always be represented by a Fourier series at every point within any small error by increasing N This is not the case when a true jump discontinuity is present Figure 632 illustrates this behavior using N 100 06LathiC06 2017925 1554 page 665 73 67 MATLAB Fourier Series Applications 665 001 0008 0006 0004 0002 0 0002 0004 0006 0008 001 t sec 5 0 5 10 15 20 mt volts Figure 633 Test signal mt with θn 0 As with any computer MATLAB cannot generate truly random numbers Rather it generates pseudorandom numbers Pseudorandom numbers are deterministic sequences that appear to be random The particular sequence of numbers that is realized depends entirely on the initial state of the pseudorandom number generator Setting the generators initial state to a known value allows a random experiment with reproducible results The command rng0 initializes the state of the pseudorandom number generator to a known condition of zero and the MATLAB command randab generates an abyb matrix of pseudorandom numbers that are uniformly distributed over the interval 01 Radian phases occupy the wider interval 02π so the results from rand need to be appropriately scaled rng0 thetarand0 2pirandN1 Next we recompute and plot mt using the randomly chosen θn mrand0 mthetarand0tomega plottmrand0k axis0010011010 xlabelt sec ylabelmt volts setgcaytickminmrand0maxmrand0 grid on For a vector input the min and max commands return the minimum and maximum values of the vector Using these values to set y axis tick marks makes it easy to identify the extreme values of the mt As seen from Fig 634 the maximum amplitude is now 76307 which is significantly smaller than the maximum of 20 when θn 0 Randomly chosen phases suffer a fatal fault there is little guarantee of optimal performance For example repeating the experiment with rng5 produces a maximum magnitude of 82399 volts as shown in Fig 635 This value is significantly higher than the previous maximum of 76307 volts Clearly it is better to replace a random solution with an optimal solution What constitutes optimal Many choices exist but desired signal criteria naturally suggest that optimal phases minimize the maximum magnitude of mt over all t To find these optimal phases MATLABs fminsearch command is useful First the function to be minimized called the objective function is defined maxmagm thetatomega maxabssumcosomegatthetaonessizet 06LathiC06 2017925 1554 page 666 74 666 CHAPTER 6 CONTINUOUSTIME SIGNAL ANALYSIS THE FOURIER SERIES 001 0008 0006 0004 0002 0 0002 0004 0006 0008 001 t sec 74460 76307 mt volts Figure 634 Test signal mt with random θn found by using rng0 001 0008 0006 0004 0002 0 0002 0004 0006 0008 001 t sec 82399 78268 mt volts Figure 635 Test signal mt with random θn found by using randstate1 The anonymous function argument order is important fminsearch uses the first input argument as the variable of minimization To minimize over θ as desired θ must be the first argument of the objective function maxmagm Next the time vector is shortened to include only one period of mt t linspace0001401 A full period ensures that all values of mt are considered the short length of t helps ensure that functions execute quickly An initial value of θ is randomly chosen to begin the search rng0 thetainit 2pirandN1 thetaopt fminsearchmaxmagmthetainittomega Notice that fminsearch finds the minimizer to maxmagm over θ by using an initial value thetainit Most numerical minimization techniques are capable of finding only local minima and fminsearch is no exception As a result fminsearch does not always produce a unique solution The empty square brackets indicate no special options are requested and the remaining ordered arguments are secondary inputs for the objective function Full format details for fminsearch are available from MATLABs help facilities 06LathiC06 2017925 1554 page 667 75 68 Summary 667 001 0008 0006 0004 0002 0 0002 0004 0006 0008 001 t sec 53632 53414 mt volts Figure 636 Test signal mt with optimized phases Figure 636 shows the phaseoptimized test signal The maximum magnitude is reduced to a value of 53632 volts which is a significant improvement over the original peak of 20 volts Although the signals shown in Figs 633 through 636 look different they all possess the same magnitude spectra The signals differ only in phase spectra It is interesting to investigate the similarities and differences of these signals in ways other than graphs and mathematics For example is there an audible difference between the signals For computers equipped with sound capability the MATLAB sound command can be used to find out Fs 8000 t 01Fs2 Two second records at a sampling rate of 8kHz soundmthetatomega20Fs Play scaled mt constructed using zero phases Since the sound command clips magnitudes that exceed 1 the input vector is scaled by 120 to avoid clipping and the resulting sound distortion The signals using other phase assignments are created and played in a similar fashion How well does the human ear discern the differences in phase spectra If you are like most people you will not be able to discern any differences in how these waveforms sound 68 SUMMARY In this chapter we showed how a periodic signal can be represented as a sum of sinusoids or exponentials If the frequency of a periodic signal is f0 then it can be expressed as a weighted sum of a sinusoid of frequency f0 and its harmonics the trigonometric Fourier series We can reconstruct the periodic signal from a knowledge of the amplitudes and phases of these sinusoidal components amplitude and phase spectra If a periodic signal xt has an even symmetry its Fourier series contains only cosine terms including dc In contrast if xt has an odd symmetry its Fourier series contains only sine terms If xt has neither type of symmetry its Fourier series contains both sine and cosine terms At points of discontinuity the Fourier series for xt converges to the mean of the values of xt on either side of the discontinuity For signals with discontinuities the Fourier series converges in the mean and exhibits Gibbs phenomenon at the points of discontinuity The amplitude spectrum of the Fourier series for a periodic signal xt with jump discontinuities decays slowly as 1n with frequency We need a large number of terms in the Fourier series to approximate xt within 06LathiC06 2017925 1554 page 668 76 668 CHAPTER 6 CONTINUOUSTIME SIGNAL ANALYSIS THE FOURIER SERIES a given error In contrast the amplitude spectrum of a smoother periodic signal decays faster with frequency and we require a smaller number of terms in the series to approximate xt within a given error A sinusoid can be expressed in terms of exponentials Therefore the Fourier series of a periodic signal can also be expressed as a sum of exponentials the exponential Fourier series The exponential form of the Fourier series and the expressions for the series coefficients are more compact than those of the trigonometric Fourier series Also the response of LTIC systems to an exponential input is much simpler than that for a sinusoidal input Moreover the exponential form of representation lends itself better to mathematical manipulations than does the trigonometric form This includes the establishment of useful Fourier series properties that simplify work and help provide a more intuitive understanding of signals For these reasons the exponential form of the series is preferred in modern practice in the areas of signals and systems The plots of amplitudes and angles of various exponential components of the Fourier series as functions of the frequency are the exponential Fourier spectra amplitude and angle spectra of the signal Because a sinusoid cosω0t can be represented as a sum of two exponentials ejω0t and ejω0t the frequencies in the exponential spectra range from ω to By definition frequency of a signal is always a positive quantity Presence of a spectral component of a negative frequency nω0 merely indicates that the Fourier series contains terms of the form ejnω0t The spectra of the trigonometric and exponential Fourier series are closely related and one can be found by the inspection of the other In Sec 65 we discuss a method of representing signals by the generalized Fourier series of which the trigonometric and exponential Fourier series are special cases Signals are vectors in every sense Just as a vector can be represented as a sum of its components in a variety of ways depending on the choice of the coordinate system a signal can be represented as a sum of its components in a variety of ways of which the trigonometric and exponential Fourier series are only two examples Just as we have vector coordinate systems formed by mutually orthogonal vectors we also have signal coordinate systems basis signals formed by mutually orthogonal signals Any signal in this signal space can be represented as a sum of the basis signals Each set of basis signals yields a particular Fourier series representation of the signal The signal is equal to its Fourier series not in the ordinary sense but in the special sense that the energy of the difference between the signal and its Fourier series approaches zero This allows for the signal to differ from its Fourier series at some isolated points REFERENCES 1 Bell E T Men of Mathematics Simon Schuster New York 1937 2 Durant W and Durant A The Age of Napoleon Part XI in The Story of Civilization Series Simon Schuster New York 1975 3 Calinger R Classics of Mathematics 4th ed Moore Publishing Oak Park IL 1982 4 Lanczos C Discourse on Fourier Series Oliver Boyd London 1966 5 Körner T W Fourier Analysis Cambridge University Press Cambridge UK 1989 6 Guillemin E A Theory of Linear Physical Systems Wiley New York 1963 7 Gibbs W J Nature vol 59 p 606 April 1899 8 Bôcher M Annals of Mathematics vol 7 no 2 1906 07LathiC07 2017925 1917 page 680 1 C H A P T E R CONTINUOUSTIME SIGNAL ANALYSIS THE FOURIER TRANSFORM 7 We can analyze linear systems in many different ways by taking advantage of the property of linearity whereby the input is expressed as a sum of simpler components The system response to any complex input can be found by summing the systems response to these simpler components of the input In timedomain analysis we separated the input into impulse components In the frequencydomain analysis in Ch 4 we separated the input into exponentials of the form est the Laplace transform where the complex frequency s σ jω The Laplace transform although very valuable for system analysis proves somewhat awkward for signal analysis where we prefer to represent signals in terms of exponentials ejωt instead of est This is accomplished by the Fourier transform In a sense the Fourier transform may be considered to be a special case of the Laplace transform with s jω Although this view is true most of the time it does not always hold because of the nature of convergence of the Laplace and Fourier integrals In Ch 6 we succeeded in representing periodic signals as a sum of everlasting sinusoids or exponentials of the form ejωt The Fourier integral developed in this chapter extends this spectral representation to aperiodic signals 71 APERIODIC SIGNAL REPRESENTATION BY THE FOURIER INTEGRAL Applying a limiting process we now show that an aperiodic signal can be expressed as a continuous sum integral of everlasting exponentials To represent an aperiodic signal xt such as the one depicted in Fig 71a by everlasting exponentials let us construct a new periodic signal xT0t formed by repeating the signal xt at intervals of T0 seconds as illustrated in Fig 71b The period T0 is made long enough to avoid overlap between the repeating pulses The periodic signal xT0t can be represented by an exponential Fourier series If we let T0 the pulses in the periodic signal repeat after an infinite interval and therefore lim T0xT0t xt 680 07LathiC07 2017125 2004 page 701 22 73 Some Properties of the Fourier Transform 701 The Fourier transform is given by ut 1 jω πδω Clearly Xjω Xω in this case To understand this puzzle consider the fact that we obtain Xjω by setting s jω in Eq 724 This implies that the integral on the righthand side of Eq 724 converges for s jω meaning that s jω the imaginary axis lies in the ROC for Xs The general rule is that only when the ROC for Xs includes the ω axis does setting s jω in Xs yield the Fourier transform Xω that is Xjω Xω This is the case of absolutely integrable xt If the ROC of Xs excludes the ω axis Xjω Xω This is the case for exponentially growing xt and also xt that is constant or is oscillating with constant amplitude The reason for this peculiar behavior has something to do with the nature of convergence of the Laplace and the Fourier integrals when xt is not absolutely integrable This discussion shows that although the Fourier transform may be considered as a special case of the Laplace transform we need to circumscribe such a view This fact can also be confirmed by noting that a periodic signal has the Fourier transform but the Laplace transform does not exist 73 SOME PROPERTIES OF THE FOURIER TRANSFORM We now study some of the important properties of the Fourier transform and their implications as well as applications We have already encountered two important properties linearity Eq 715 and the conjugation property Eq 711 Before embarking on this study we shall explain an important and pervasive aspect of the Fourier transform the timefrequency duality To explain this point consider the unit step function and its transforms Both the Laplace and the Fourier transform synthesize xt using everlasting exponentials of the form est The frequency s can be anywhere in the complex plane for the Laplace transform but it must be restricted to the ω axis in the case of the Fourier transform The unit step function is readily synthesized in the Laplace transform by a relatively simple spectrum Xs 1s in which the frequencies s are chosen in the RHP the region of convergence for ut is Re s 0 In the Fourier transform however we are restricted to values of s on the ω axis only The function ut can still be synthesized by frequencies along the ω axis but the spectrum is more complicated than it is when we are free to choose the frequencies in the RHP In contrast when xt is absolutely integrable the region of convergence for the Laplace transform includes the ω axis and we can synthesize xt by using frequencies along the ω axis in both transforms This leads to Xjω Xω We may explain this concept by an example of two countries X and Y Suppose these countries want to construct similar dams in their respective territories Country X has financial resources but not much manpower In contrast Y has considerable manpower but few financial resources The dams will still be constructed in both countries although the methods used will be different Country X will use expensive but efficient equipment to compensate for its lack of manpower whereas Y will use the cheapest possible equipment in a laborintensive approach to the project Similarly both Fourier and Laplace integrals converge for ut but the makeup of the components used to synthesize ut will be very different for two cases because of the constraints of the Fourier transform which are not present for the Laplace transform 07LathiC07 2017925 1917 page 708 29 708 CHAPTER 7 CONTINUOUSTIME SIGNAL ANALYSIS THE FOURIER TRANSFORM t t0 t Figure 722 Physical explanation of the timeshifting property cosωt delayed by t0 is given by cos ωt t0 cosωt ωt0 Therefore a time delay t0 in a sinusoid of frequency ω manifests as a phase delay of ωt0 This is a linear function of ω meaning that higherfrequency components must undergo proportionately higher phase shifts to achieve the same time delay This effect is depicted in Fig 722 with two sinusoids the frequency of the lower sinusoid being twice that of the upper The same time delay t0 amounts to a phase shift of π2 in the upper sinusoid and a phase shift of π in the lower sinusoid This verifies the fact that to achieve the same time delay higherfrequency sinusoids must undergo proportionately higher phase shifts The principle of linear phase shift is very important and we shall encounter it again in distortionless signal transmission and filtering applications EXAMPLE 713 Fourier Transform TimeShifting Property Use the timeshifting property to find the Fourier transform of eatt0 This function shown in Fig 723a is a timeshifted version of eat depicted in Fig 721a From Eqs 728 and 729 we have eatt0 2a a2 ω2 ejωt0 The spectrum of eatt0 Fig 723b is the same as that of eat Fig 721b except for an added phase shift of ωt0 07LathiC07 2017925 1917 page 714 35 714 CHAPTER 7 CONTINUOUSTIME SIGNAL ANALYSIS THE FOURIER TRANSFORM Old is gold but sometimes it is fools gold by using modulation whereby each radio station is assigned a distinct carrier frequency Each station transmits a modulated signal This procedure shifts the signal spectrum to its allocated band which is not occupied by any other station A radio receiver can pick up any station by tuning to the band of the desired station The receiver must now demodulate the received signal undo the effect of modulation Demodulation therefore consists of another spectral shift required to restore the signal to its original band Note that both modulation and demodulation implement spectral shifting consequently demodulation operation is similar to modulation see Sec 77 This method of transmitting several signals simultaneously over a channel by sharing its frequency band is known as frequencydivision multiplexing FDM 2 For effective radiation of power over a radio link the antenna size must be of the order of the wavelength of the signal to be radiated Audio signal frequencies are so low wavelengths are so large that impracticably large antennas would be required for radiation Here shifting the spectrum to a higher frequency a smaller wavelength by modulation solves the problem CONVOLUTION The timeconvolution property and its dual the frequencyconvolution property state that if x1t X1ω and x2t X2ω then x1tx2t X1ωX2ω time convolution 733 07LathiC07 2017925 1917 page 720 41 720 CHAPTER 7 CONTINUOUSTIME SIGNAL ANALYSIS THE FOURIER TRANSFORM Fourier series to verify spectrum correctness Let us demonstrate the idea for the current example with τ 1 To begin we represent Xω τ 2sinc2ωτ4 using an anonymous function in MATLAB Since MATLAB computes sincx as sinπxπx we must scale the input by 1π to match the notation of sinc in this book tau 1 X omega tau2sincomegatau4pi2 For our periodic replication let us pick T0 2 which is comfortably wide enough to accommodate our τ 1width function without overlap We use Eq 75 to define the needed Fourier series coefficients Dn T0 2 omega0 2piT0 D n Xnomega0T0 Let us use 25 harmonics to synthesize the periodic replication x25t of our triangular signal xt To begin waveform synthesis we set the dc portion of the signal t T0001T0 x25 D0onessizet To add the desired 25 harmonics we enter a loop for 1 n 25 and add in the Dn and Dn terms Although the result should be real small roundoff errors cause the reconstruction to be complex These small imaginary parts are removed by using the real command for n 125 x25 x25realDnexp1jomega0ntDnexp1jomega0nt end Lastly we plot the resulting truncated Fourier series synthesis of xt plottx25k xlabelt ylabelx25t Since the synthesized waveform shown in Fig 728 closely matches a 2periodic replication of the triangle wave in Fig 727a we have high confidence that both the computed Dn and by extension the Fourier spectrum Xω are correct 2 15 1 05 0 05 1 15 2 t 0 05 1 x25t Figure 728 Synthesizing a 2periodic replication of xt using a truncated Fourier series 07LathiC07 2017925 1917 page 721 42 74 Signal Transmission Through LTIC Systems 721 DRILL 79 Fourier Transform TimeDifferentiation Property Use the timedifferentiation property to find the Fourier transform of rect tτ 74 SIGNAL TRANSMISSION THROUGH LTIC SYSTEMS If xt and yt are the input and output of an LTIC system with impulse response ht then as demonstrated in Eq 735 Yω HωXω This equation does not apply to asymptotically unstable systems because ht for such systems is not Fourier transformable It applies to BIBOstable as well as most of the marginally stable systems Similarly this equation does not apply if xt is not Fourier transformable In Ch 4 we saw that the Laplace transform is more versatile and capable of analyzing all kinds of LTIC systems whether stable unstable or marginally stable Laplace transform can also handle exponentially growing inputs In comparison to the Laplace transform the Fourier transform in system analysis is not just clumsier but also very restrictive Hence the Laplace transform is preferable to the Fourier transform in LTIC system analysis We shall not belabor the application of the Fourier transform to LTIC system analysis We consider just one example here EXAMPLE 718 Fourier Transform to Determine the ZeroState Response Use the Fourier transform to find the zerostate response of a stable LTIC system with frequency response Hs 1 s 2 and the input is xt etut Stability implies that the region of convergence of Hs includes the ω axis In this case Xω 1 jω 1 For marginally stable systems if the input xt contains a finiteamplitude sinusoid of the systems natural frequency which leads to resonance the output is not Fourier transformable It does however apply to marginally stable systems if the input does not contain a finiteamplitude sinusoid of the systems natural frequency 07LathiC07 2017925 1917 page 725 46 74 Signal Transmission Through LTIC Systems 725 component is delayed by td seconds This results in the output equal to G0 times the input delayed by td seconds Because each spectral component is attenuated by the same factor G0 and delayed by exactly the same amount td the output signal is an exact replica of the input except for attenuating factor G0 and delay td For distortionless transmission we require a linear phase characteristic The phase is not only a linear function of ω it should also pass through the origin ω 0 In practice many systems have a phase characteristic that may be only approximately linear A convenient way of judging phase linearity is to plot the slope of Hω as a function of frequency This slope which is constant for an ideal linear phase ILP system is a function of ω in the general case and can be expressed as tgω d dω Hω 740 If tgω is constant all the components are delayed by the same time interval tg But if the slope is not constant the time delay tg varies with frequency This variation means that different frequency components undergo different amounts of time delay and consequently the output waveform will not be a replica of the input waveform As we shall see tgω plays an important role in bandpass systems and is called the group delay or envelope delay Observe that constant td Eq 739 implies constant tg Note that Hω φ0 ωtg also has a constant tg Thus constant group delay is a more relaxed condition It is often thought erroneously that flatness of amplitude response Hω alone can guarantee signal quality However a system that has a flat amplitude response may yet distort a signal beyond recognition if the phase response is not linear td not constant THE NATURE OF DISTORTION IN AUDIO AND VIDEO SIGNALS Generally speaking the human ear can readily perceive amplitude distortion but is relatively insensitive to phase distortion For the phase distortion to become noticeable the variation in delay variation in the slope of Hω should be comparable to the signal duration or the physically perceptible duration in case the signal itself is long In the case of audio signals each spoken syllable can be considered to be an individual signal The average duration of a spoken syllable is of a magnitude of the order of 001 to 01 second Audio systems may have nonlinear phases yet no noticeable signal distortion results because in practical audio systems maximum variation in the slope of Hω is only a small fraction of a millisecond This is the real truth underlying the statement that the human ear is relatively insensitive to phase distortion 3 As a result the manufacturers of audio equipment make available only Hω the amplitude response characteristic of their systems For video signals in contrast the situation is exactly the opposite The human eye is sensitive to phase distortion but is relatively insensitive to amplitude distortion Amplitude distortion in television signals manifests itself as a partial destruction of the relative halftone values of the resulting picture but this effect is not readily apparent to the human eye Phase distortion nonlinear phase on the other hand causes different time delays in different picture elements The result is a smeared picture and this effect is readily perceived by the human eye Phase distortion is also very important in digital communication systems because the nonlinear phase characteristic of a channel causes pulse dispersion spreading out which in turn causes pulses to interfere with neighboring pulses Such interference between pulses can cause an error in the pulse amplitude at the receiver a binary 1 may read as 0 and vice versa 07LathiC07 2017925 1917 page 727 48 74 Signal Transmission Through LTIC Systems 727 spectrum ˆYω is given by ˆYω HωˆZω HωXω ωc Recall that the bandwidth of Xω is W so that the bandwidth of Xω ωc is 2W centered at ωc Over this range Hω is given by Eq 741 Hence ˆYω G0Xω ωcejφ0ωtg G0ejφ0Xω ωceωtg Use of Eqs 729 and 730 yields ˆyt as ˆyt G0ejφ0xt tgejωcttg G0xt tgejωcttgφ0 This is the system response to input ˆzt xtejωct which is a complex signal We are really interested in finding the response to the input zt xtcosωct which is the real part of ˆzt xtejωct Hence we use Eq 231 to obtain yt the system response to the input zt xtcosωct as yt G0xt tgcosωct tg φ0 742 where tg the group or envelope delay is the negative slope of Hω at ωc The output yt is basically the delayed input zt tg except that the output carrier acquires an extra phase φ0 The output envelope xt tg is the delayed version of the input envelope xt and is not affected by extra phase φ0 of the carrier In a modulated signal such as xtcosωct the information generally resides in the envelope xt Hence the transmission is considered to be distortionless if the envelope xt remains undistorted Most practical systems satisfy Eq 741 at least over a very small band Figure 730b shows a typical case in which this condition is satisfied for a small band W centered at frequency ωc A system in Eq 741 is said to have a generalized linear phase GLP as illustrated in Fig 730 The ideal linear phase ILP characteristics is shown in Fig 729 For distortionless transmission of bandpass signals the system need satisfy Eq 741 only over the bandwidth of the bandpass signal Caution Recall that the phase response associated with the amplitude response may have jump discontinuities when the amplitude response goes negative Jump discontinuities also arise because of the use of the principal value for phase Under such conditions to compute the group delay Eq 740 we should ignore the jump discontinuities Equation 742 can also be expressed as yt Goxt tgcosωct tph where tph called the phase delay at ωc is given by tphωc ωctg φ0ωc Generally tph varies with ω and we can write tphω ωtg φ0 ω Recall also that tg itself may vary with ω 07LathiC07 2017925 1917 page 728 49 728 CHAPTER 7 CONTINUOUSTIME SIGNAL ANALYSIS THE FOURIER TRANSFORM EXAMPLE 719 Distortionless Bandpass Transmission a A signal zt shown in Fig 731b is given by zt xtcosωct where ωc 2000π The pulse xt Fig 731a is a lowpass pulse of duration 01 second and has a bandwidth of about 10 Hz This signal is passed through a filter whose frequency response is shown in Fig 731c shown only for positive ω Find and sketch the filter output yt b Find the filter response if ωc 4000π a The spectrum Zω is a narrow band of width 20 Hz centered at frequency f0 1 kHz The gain at the center frequency 1 kHz is 2 The group delay which is the negative of the slope of the phase plot can be found by drawing tangents at ωc as shown in Fig 731c The negative of the slope of the tangent represents tg and the intercept along the vertical axis by the tangent represents φ0 at that frequency From the tangents at ωc we find tg the group delay as tg 24π 04π 2000π 103 The vertical axis intercept is φ0 04π Hence by using Eq 742 with gain G0 2 we obtain yt 2xt tgcosωct tg 04π ωc 2000π tg 103 Figure 731d shows the output yt which consists of the modulated pulse envelope xt delayed by 1 ms and the phase of the carrier changed by 04π The output shows no distortion of the envelope xt only the delay The carrier phase change does not affect the shape of envelope Hence the transmission is considered distortionless b Figure 731c shows that when ωc 4000π the slope of Hω is zero so that tg 0 Also the gain G0 15 and the intercept of the tangent with the vertical axis is φ0 31π Hence yt 15xtcosωct 31π This too is a distortionless transmission for the same reasons as for case a 07LathiC07 2017925 1917 page 732 53 732 CHAPTER 7 CONTINUOUSTIME SIGNAL ANALYSIS THE FOURIER TRANSFORM 0 t hˆt td Figure 734 Approximate realization of an ideal lowpass filter by truncation of its impulse response 01 ms would be a reasonable choice The truncation operation cutting the tail of ht to make it causal however creates some unsuspected problems We discuss these problems and their cure in Sec 78 In practice we can realize a variety of filter characteristics that approach the ideal Practical realizable filter characteristics are gradual without jump discontinuities in amplitude response DRILL 711 The Unrealizable Gaussian Response Show that a filter with Gaussian frequency response Hω eαω2 is unrealizable Demonstrate this fact in two ways first by showing that its impulse response is noncausal and then by showing that Hω violates the PaleyWiener criterion Hint Use pair 22 in Table 71 THINKING IN THE TIME AND FREQUENCY DOMAINS A TWODIMENSIONAL VIEW OF SIGNALS AND SYSTEMS Both signals and systems have dual personalities the time domain and the frequency domain For a deeper perspective we should examine and understand both these identities because they offer complementary insights An exponential signal for instance can be specified by its timedomain description such as e2tut or by its Fourier transform its frequencydomain description 1jω 2 The timedomain description depicts the waveform of a signal The frequencydomain description portrays its spectral composition relative amplitudes of its sinusoidal or exponential components and their phases For the signal e2t for instance the timedomain description portrays the exponentially decaying signal with a time constant 05 The frequencydomain description characterizes it as a lowpass signal which can be synthesized by sinusoids with amplitudes decaying with frequency roughly as 1ω An LTIC system can also be described or specified in the time domain by its impulse response ht or in the frequency domain by its frequency response Hω In Sec 26 we studied intuitive insights in the system behavior offered by the impulse response which consists of characteristic modes of the system By purely qualitative reasoning we saw that the system responds well to signals that are similar to the characteristic modes and responds poorly to signals that are very different from those modes We also saw that the shape of the impulse response ht determines the system time constant speed of response and pulse dispersion spreading which in turn determines the rate of pulse transmission The frequency response Hω specifies the system response to exponential or sinusoidal input of various frequencies This is precisely the filtering characteristic of the system 07LathiC07 2017925 1917 page 736 57 736 CHAPTER 7 CONTINUOUSTIME SIGNAL ANALYSIS THE FOURIER TRANSFORM This result indicates that the spectral components of xt in the band from 0 dc to 12706a rads 202a Hz contribute 95 of the total signal energy all the remaining spectral components in the band from 12706a rads to contribute only 5 of the signal energy DRILL 712 Signal Energy and Parsevals Theorem Use Parsevals theorem to show that the energy of the signal xt 2at2 a2 is 2πa Hint Find Xω using pair 3 of Table 71 and the duality property THE ESSENTIAL BANDWIDTH OF A SIGNAL The spectra of all practical signals extend to infinity However because the energy of any practical signal is finite the signal spectrum must approach 0 as ω Most of the signal energy is contained within a certain band of B Hz and the energy contributed by the components beyond B Hz is negligible We can therefore suppress the signal spectrum beyond B Hz with little effect on the signal shape and energy The bandwidth B is called the essential bandwidth of the signal The criterion for selecting B depends on the error tolerance in a particular application We may for example select B to be that band which contains 95 of the signal energy This figure may be higher or lower than 95 depending on the precision needed Using such a criterion we can determine the essential bandwidth of a signal The essential bandwidth B for the signal eatut using 95 energy criterion was determined in Ex 720 to be 202a Hz Suppression of all the spectral components of xt beyond the essential bandwidth results in a signal ˆxt which is a close approximation of xt If we use the 95 criterion for the essential bandwidth the energy of the error the difference xt ˆxt is 5 of Ex 77 APPLICATION TO COMMUNICATIONS AMPLITUDE MODULATION Modulation causes a spectral shift in a signal and is used to gain certain advantages mentioned in our discussion of the frequencyshifting property Broadly speaking there are two classes of modulation amplitude linear modulation and angle nonlinear modulation In this section we shall discuss some practical forms of amplitude modulation For lowpass signals the essential bandwidth may also be defined as a frequency at which the value of the amplitude spectrum is a small fraction about 1 of its peak value In Ex 720 for instance the peak value which occurs at ω 0 is 1a 07LathiC07 2017925 1917 page 738 59 738 CHAPTER 7 CONTINUOUSTIME SIGNAL ANALYSIS THE FOURIER TRANSFORM then mtcos ωct 1 2 Mω ωc Mω ωc 748 Recall that Mω ωc is Mωshifted to the right by ωc and Mω ωc is Mωshifted to the left by ωc Thus the process of modulation shifts the spectrum of the modulating signal to the left and the right by ωc Note also that if the bandwidth of mt is B Hz then as indicated in Fig 736c the bandwidth of the modulated signal is 2B Hz We also observe that the modulated signal spectrum centered at ωc is composed of two parts a portion that lies above ωc known as the upper sideband USB and a portion that lies below ωc known as the lower sideband LSB Similarly the spectrum centered at ωc has upper and lower sidebands This form of modulation is called double sideband DSB modulation for the obvious reason The relationship of B to ωc is of interest Figure 736c shows that ωc 2πB to avoid the overlap of the spectra centered at ωc If ωc 2πB the spectra overlap and the information of mt are lost in the process of modulation a loss that makes it impossible to get back mt from the modulated signal mtcos ωct EXAMPLE 721 DoubleSideband SuppressedCarrier Modulation For a baseband signal mt cos ωmt find the DSBSC signal and sketch its spectrum Identify the upper and lower sidebands We shall work this problem in the frequency domain as well as the time domain to clarify the basic concepts of DSBSC modulation In the frequencydomain approach we work with the signal spectra The spectrum of the baseband signal mt cos ωmt is given by Mω πδω ωm δω ωm The spectrum consists of two impulses located at ωm as depicted in Fig 737a The DSBSC modulated spectrum as indicated by Eq 748 is the baseband spectrum in Fig 737a shifted to the right and the left by ωc times 05 as depicted in Fig 737b This spectrum consists of impulses at ωc ωm and ωc ωm The spectrum beyond ωc is the upper sideband USB and the one below ωc is the lower sideband LSB Observe that the DSBSC spectrum does not have as a component the carrier frequency ωc This is why the term doublesideband suppressed carrier DSBSC is used for this type of modulation Practical factors may impose additional restrictions on ωc For instance in broadcast applications a radiating antenna can radiate only a narrow band without distortion This restriction implies that avoiding distortion caused by the radiating antenna calls for ωc2πB 1 The broadcast band AM radio for instance with B 5 kHz and the band of 5501600 kHz for carrier frequency gives a ratio of ωc2πB roughly in the range of 100300 07LathiC07 2017925 1917 page 742 63 742 CHAPTER 7 CONTINUOUSTIME SIGNAL ANALYSIS THE FOURIER TRANSFORM 772 Amplitude Modulation AM For the suppressedcarrier scheme just discussed a receiver must generate a carrier in frequency and phase synchronism with the carrier at a transmitter that may be located hundreds or thousands of miles away This situation calls for a sophisticated receiver which could be quite costly The other alternative is for the transmitter to transmit a carrier A cosωct along with the modulated signal mt cosωct so that there is no need to generate a carrier at the receiver In this case the transmitter needs to transmit much larger power a rather expensive procedure In pointtopoint communications where there is one transmitter for each receiver substantial complexity in the receiver system can be justified provided there is a large enough saving in expensive highpower transmitting equipment On the other hand for a broadcast system with a multitude of receivers for each transmitter it is more economical to have one expensive highpower transmitter and simpler less expensive receivers The second option transmitting a carrier along with the modulated signal is the obvious choice in this case This is amplitude modulation AM in which the transmitted signal ϕAMt is given by ϕAMt Acos ωct mtcosωct A mtcosωct 750 Recall that the DSBSC signal is mt cos ωct From Eq 750 it follows that the AM signal is identical to the DSBSC signal with Amt as the modulating signal instead of mt Therefore to sketch ϕAMt we sketch A mt and A mt as the envelopes and fill in between with the sinusoid of the carrier frequency Two cases are considered in Fig 739 In the first case A is large enough so that A mt 0 is nonnegative for all values of t In the second case A is not large enough to satisfy this condition In the first case the envelope Fig 739d has the same shape as mt although riding on a dc of magnitude A In the second case the envelope shape is not mt for some parts get rectified Fig 739e Thus we can detect the desired signal mt by detecting the envelope in the first case In the second case such a detection is not possible We shall see that envelope detection is an extremely simple and inexpensive operation which does not require generation of a local carrier for the demodulation But as just noted the envelope of AM has the information about mt only if the AM signal Amtcos ωct satisfies the condition A mt 0 for all t Thus the condition for envelope detection of an AM signal is A mt 0 for allt 751 If mp is the peak amplitude positive or negative of mt then Eq 751 is equivalent to A mp Thus the minimum carrier amplitude required for the viability of envelope detection is mp This point is clearly illustrated in Fig 739 We define the modulation index μ as μ mp A 752 where A is the carrier amplitude Note that mp is a constant of the signal mt Because A mp and because there is no upper bound on A it follows that 0 μ 1 07LathiC07 2017925 1917 page 743 64 77 Application to Communications Amplitude Modulation 743 Figure 739 An AM signal a for two values of A b c and the respective envelopes d e as the required condition for the viability of demodulation of AM by an envelope detector When A mp Eq 752 shows that μ 1 overmodulation shown in Fig 739e In this case the option of envelope detection is no longer viable We then need to use synchronous demodulation Note that synchronous demodulation can be used for any value of μ see Prob 777 The envelope detector which is considerably simpler and less expensive than the synchronous detector can be used only when μ 1 EXAMPLE 723 Amplitude Modulation Sketch ϕAMt for modulation indices of μ 05 50 modulation and μ 1 100 modulation when mt Bcos ωmt This case is referred to as tone modulation because the modulating signal is a pure sinusoid or tone 07LathiC07 2017925 1917 page 747 68 77 Application to Communications Amplitude Modulation 747 as singlesideband SSB transmission which requires only half the bandwidth of the DSB signal Thus we transmit only the upper sidebands Fig 742c or only the lower sidebands Fig 742d An SSB signal can be coherently synchronously demodulated For example multiplication of a USB signal Fig 742c by 2cos ωct shifts its spectrum to the left and to the right by ωc yielding the spectrum in Fig 742e Lowpass filtering of this signal yields the desired baseband signal The case is similar with an LSB signal Hence demodulation of SSB signals is identical to that of DSBSC signals and the synchronous demodulator in Fig 738a can demodulate SSB signals Note that we are talking of SSB signals without an additional carrier Hence they are suppressedcarrier signals SSBSC EXAMPLE 724 SingleSideband Modulation Find the USB upper sideband and LSB lower sideband signals when mt cos ωmt Sketch their spectra and show that these SSB signals can be demodulated using the synchronous demodulator in Fig 738a The DSBSC signal for this case is ϕDSBSCt mtcos ωct cos ωmtcos ωct 1 2cosωc ωmt cosωc ωmt As pointed out in Ex 721 the terms 12cosωc ωmt and 12cosωc ωmt represent the upper and lower sidebands respectively The spectra of the upper and lower sidebands are given in Figs 743a and 743b Observe that these spectra can be obtained from the DSBSC spectrum in Fig 737b by using a proper filter to suppress the undesired sidebands For instance the USB signal in Fig 743a can be obtained by passing the DSBSC signal Fig 737b through a highpass filter of cutoff frequency ωc Similarly the LSB signal in Fig 743b can be obtained by passing the DSBSC signal through a lowpass filter of cutoff frequency ωc If we apply the LSB signal 12cosωc ωmt to the synchronous demodulator in Fig 738a the multiplier output is et 1 2 cosωc ωmtcos ωct 1 4cos ωmt cos2ωc ωmt The term 14cos2ωcωmt is suppressed by the lowpass filter producing the desired output 14cos ωmt which is mt4 The spectrum of this term is πδω ωmδω ωm4 as depicted in Fig 743c In the same way we can show that the USB signal can be demodulated by the synchronous demodulator In the frequency domain demodulation multiplication by cos ωct amounts to shifting the LSB spectrum Fig 743b to the left and the right by ωc times 05 and then suppressing the high frequency as illustrated in Fig 743c The resulting spectrum represents the desired signal 14mt 07LathiC07 2017925 1917 page 749 70 78 Data Truncation Window Functions 749 200 3200 0 fHz Relative power Figure 744 Voice spectrum at low frequencies around ω 0 SSB techniques cause considerable distortion Such is the case with video signals Consequently for video signals instead of SSB we use another technique the vestigial sideband VSB which is a compromise between SSB and DSB It inherits the advantages of SSB and DSB but avoids their disadvantages at a cost of slightly increased bandwidth VSB signals are relatively easy to generate and their bandwidth is only slightly typically 25 greater than that of SSB signals In VSB signals instead of rejecting one sideband completely as in SSB we accept a gradual cutoff from one sideband 4 774 FrequencyDivision Multiplexing Signal multiplexing allows transmission of several signals on the same channel Later in Ch 8 Sec 822 we shall discuss timedivision multiplexing TDM where several signals timeshare the same channel such as a cable or an optical fiber In frequencydivision multiplexing FDM the use of modulation as illustrated in Fig 745 makes several signals share the band of the same channel Each signal is modulated by a different carrier frequency The various carriers are adequately separated to avoid overlap or interference between the spectra of various modulated signals These carriers are referred to as subcarriers Each signal may use a different kind of modulation for example DSBSC AM SSBSC VSBSC or even other forms of modulation not discussed here such as FM frequency modulation or PM phase modulation The modulatedsignal spectra may be separated by a small guard band to avoid interference and to facilitate signal separation at the receiver When all the modulated spectra are added we have a composite signal that may be considered to be a new baseband signal Sometimes this composite baseband signal may be used to further modulate a highfrequency radio frequency or RF carrier for the purpose of transmission At the receiver the incoming signal is first demodulated by the RF carrier to retrieve the composite baseband which is then bandpassfiltered to separate the modulated signals Then each modulated signal is individually demodulated by an appropriate subcarrier to obtain all the basic baseband signals 78 DATA TRUNCATION WINDOW FUNCTIONS We often need to truncate data in diverse situations from numerical computations to filter design For example if we need to compute numerically the Fourier transform of some signal say etut we will have to truncate the signal etut beyond a sufficiently large value of t typically five time constants and above The reason is that in numerical computations we have to deal with 07LathiC07 2017925 1917 page 751 72 78 Data Truncation Window Functions 751 by adding the first n harmonics and truncating all the higher harmonics These examples show that data truncation can occur in both time and frequency domains On the surface truncation appears to be a simple problem of cutting off the data at a point at which values are deemed to be sufficiently small Unfortunately this is not the case Simple truncation can cause some unsuspected problems WINDOW FUNCTIONS Truncation operation may be regarded as multiplying a signal of a large width by a window function of a smaller finite width Simple truncation amounts to using a rectangular window wRt shown later in Fig 748a in which we assign unit weight to all the data within the window width t T2 and assign zero weight to all the data lying outside the window t T2 It is also possible to use a window in which the weight assigned to the data within the window may not be constant In a triangular window wTt for example the weight assigned to data decreases linearly over the window width shown later in Fig 748b Consider a signal xt and a window function wt If xt Xω and wt Wω and if the windowed function xwt Xwω then xwt xtwt and Xwω 1 2π XωWω According to the width property of convolution it follows that the width of Xwω equals the sum of the widths of Xω and Wω Thus truncation of a signal increases its bandwidth by the amount of bandwidth of wt Clearly the truncation of a signal causes its spectrum to spread or smear by the amount of the bandwidth of wt Recall that the signal bandwidth is inversely proportional to the signal duration width Hence the wider the window the smaller its bandwidth and the smaller the spectral spreading This result is predictable because a wider window means that we are accepting more data closer approximation which should cause smaller distortion smaller spectral spreading Smaller window width poorer approximation causes more spectral spreading more distortion In addition since Wω is really not strictly bandlimited and its spectrum 0 only asymptotically the spectrum of Xwω 0 asymptotically also at the same rate as that of Wω even if Xω is in fact strictly bandlimited Thus windowing causes the spectrum of Xω to spread into the band where it is supposed to be zero This effect is called leakage The following example clarifies these twin effects of spectral spreading and leakage Let us consider xt cos ω0t and a rectangular window wRt recttT illustrated in Fig 746b The reason for selecting a sinusoid for xt is that its spectrum consists of spectral lines of zero width Fig 746a Hence this choice will make the effect of spectral spreading and leakage easily discernible The spectrum of the truncated signal xwt is the convolution of the two impulses of Xω with the sinc spectrum of the window function Because the convolution of any function with an impulse is the function itself shifted at the location of the impulse the resulting spectrum of the truncated signal is 12π times the two sinc pulses at ω0 as depicted in Fig 746c also see Fig 726 Comparison of spectra Xω and Xwω reveals the effects of truncation These are 1 The spectral lines of Xω have zero width But the truncated signal is spread out by 2πT about each spectral line The amount of spread is equal to the width of the mainlobe of the window spectrum One effect of this spectral spreading or smearing is that if xt has two spectral components of frequencies differing by less than 4πT rads 2T Hz they 07LathiC07 2017925 1917 page 753 74 78 Data Truncation Window Functions 753 other hand the truncated signal spectrum Xwω is zero nowhere because of the sidelobes These sidelobes decay asymptotically as 1ω Thus the truncation causes spectral leakage in the band where the spectrum of the signal xt is zero The peak sidelobe magnitude is 0217 times the mainlobe magnitude 133 dB below the peak mainlobe magnitude Also the sidelobes decay at a rate 1ω which is 6 dBoctave or 20 dBdecade This is the sidelobes rolloff rate We want smaller sidelobes with a faster rate of decay high rolloff rate Figure 746d which plots WRω as a function of ω clearly shows the mainlobe and sidelobe features with the first sidelobe amplitude 133 dB below the mainlobe amplitude and the sidelobes decaying at a rate of 6 dBoctave or 20 dBdecade So far we have discussed the effect on the signal spectrum of signal truncation truncation in the time domain Because of the timefrequency duality the effect of spectral truncation truncation in frequency domain on the signal shape is similar REMEDIES FOR SIDE EFFECTS OF TRUNCATION For better results we must try to minimize the twin side effects of truncations spectral spreading mainlobe width and leakage sidelobe Let us consider each of these ills 1 The spectral spread mainlobe width of the truncated signal is equal to the bandwidth of the window function wt We know that the signal bandwidth is inversely proportional to the signal width duration Hence to reduce the spectral spread mainlobe width we need to increase the window width 2 To improve the leakage behavior we must search for the cause of the slow decay of sidelobes In Ch 6 we saw that the Fourier spectrum decays as 1ω for a signal with jump discontinuity decays as 1ω2 for a continuous signal whose first derivative is discontinuous and so on Smoothness of a signal is measured by the number of continuous derivatives it possesses The smoother the signal the faster the decay of its spectrum Thus we can achieve a given leakage behavior by selecting a suitably smooth tapered window 3 For a given window width the remedies for the two effects are incompatible If we try to improve one the other deteriorates For instance among all the windows of a given width the rectangular window has the smallest spectral spread mainlobe width but its sidelobes have high level and they decay slowly A tapered smooth window of the same width has smaller and faster decaying sidelobes but it has a wider mainlobe But we can compensate for the increased mainlobe width by widening the window Thus we can remedy both the side effects of truncation by selecting a suitably smooth window of sufficient width There are several wellknown taperedwindow functions such as Bartlett triangular Hanning von Hann Hamming Blackman and Kaiser which truncate the data gradually These This result was demonstrated for periodic signals However it applies to aperiodic signals also This is because we showed in the beginning of this chapter that if xT0t is a periodic signal formed by periodic extension of an aperiodic signal xt then the spectrum of xT0t is 1T0 times the samples of Xω Thus what is true of the decay rate of the spectrum of xT0t is also true of the rate of decay of Xω A tapered window yields a higher mainlobe width because the effective width of a tapered window is smaller than that of the rectangular window see Sec 262 Eq 247 for the definition of effective width Therefore from the reciprocity of the signal width and its bandwidth it follows that the rectangular window mainlobe is narrower than a tapered window 07LathiC07 2017925 1917 page 755 76 79 MATLAB Fourier Transform Topics 755 triangle window also called the Fejer or Cesaro is inferior in all respects to the Hanning window For this reason it is rarely used in practice Hanning is preferred over Hamming in spectral analysis because it has faster sidelobe decay For filtering applications on the other hand the Hamming window is chosen because it has the smallest sidelobe magnitude for a given mainlobe width The Hamming window is the most widely used generalpurpose window The Kaiser window which uses I0α the modified zeroorder Bessel function is more versatile and adjustable Selecting a proper value of α 0 α 10 allows the designer to tailor the window to suit a particular application The parameter α controls the mainlobesidelobe tradeoff When α 0 the Kaiser window is the rectangular window For α 54414 it is the Hamming window and when α 8885 it is the Blackman window As α increases the mainlobe width increases and the sidelobe level decreases 781 Using Windows in Filter Design We shall design an ideal lowpass filter of bandwidth W rads with frequency response Hω as shown in Fig 748e or Fig 748f For this filter the impulse response ht WπsincWt Fig 748c is noncausal and therefore unrealizable Truncation of ht by a suitable window Fig 748a makes it realizable although the resulting filter is now an approximation to the desired ideal filter We shall use a rectangular window wRt and a triangular Bartlett window wTt to truncate ht and then examine the resulting filters The truncated impulse responses hRt htwRt and hTt htwTt are depicted in Fig 748d Hence the windowed filter frequency response is the convolution of Hω with the Fourier transform of the window as illustrated in Figs 748e and 748f We make the following observations 1 The windowed filter spectra show spectral spreading at the edges and instead of a sudden switch there is a gradual transition from the passband to the stopband of the filter The transition band is smaller 2πT rads for the rectangular case than for the triangular case 4πT rads 2 Although Hω is bandlimited the windowed filters are not But the stopband behavior of the triangular case is superior to that of the rectangular case For the rectangular window the leakage in the stopband decreases slowly as 1ω in comparison to that of the triangular window as 1ω2 Moreover the rectangular case has a higher peak sidelobe amplitude than that of the triangular window 79 MATLAB FOURIER TRANSFORM TOPICS MATLAB is useful for investigating a variety of Fourier transform topics In this section a rectangular pulse is used to investigate the scaling property Parsevals theorem essential bandwidth and spectral sampling Kaiser window functions are also investigated In addition to truncation we need to delay the truncated function by T2 to render it causal However the time delay only adds a linear phase to the spectrum without changing the amplitude spectrum Thus to simplify our discussion we shall ignore the delay 07LathiC07 2017925 1917 page 757 78 79 MATLAB Fourier Transform Topics 757 791 The Sinc Function and the Scaling Property As shown in Ex 72 the Fourier transform of xt recttτ is Xω τ sincωτ2 To represent Xω in MATLAB a sinc function is first required As an alternative to the signal processing toolbox function sinc which computes sincx as sinπxπx we create our own function that follows the conventions of this book and defines sincx sinxx function y CH7MP1x CH7MP1m Chapter 7 MATLAB Program 1 Function Mfile computes the sinc function y sinxx yx0 1 yx0 sinxx0xx0 The computational simplicity of sinc x sinxx is somewhat deceptive sin00 results in a dividebyzero error Thus program CH7MP1 assigns sinc 0 1 and computes the remaining values according to the definition Notice that CH7MP1 cannot be directly replaced by an anonymous function Anonymous functions cannot have multiple lines or contain certain commands such as if or for Mfiles however can be used to define an anonymous function For example we can represent Xω as an anonymous function that is defined in terms of CH7MP1 X omegatau tauCH7MP1omegatau2 Once we have defined Xω it is simple to investigate the effects of scaling the pulse width τ Consider the three cases τ 10 τ 05 and τ 20 omega linspace4pi4pi200 plotomegaXomega1komegaXomega05komegaXomega2k grid axis tight xlabelomega ylabelXomega legendBaseline au 1Compressed au 05 Expanded au 20 Figure 749 confirms the reciprocal relationship between signal duration and spectral bandwidth time compression causes spectral expansion and time expansion causes spectral compression Additionally spectral amplitudes are directly related to signal energy As a signal is compressed signal energy and thus spectral magnitude decrease The opposite effect occurs when the signal is expanded 10 5 ω 0 5 10 0 1 2 Xω τ 1 τ 05 τ 20 Figure 749 Spectra Xω τ sincωτ2 for τ 10 τ 05 and τ 20 07LathiC07 2017925 1917 page 759 80 79 MATLAB Fourier Transform Topics 759 W Wstep end EW 12piquadXsquaredWWtau relerr E EWE end Although this guessandcheck method is not the most efficient it is relatively simple to understand CH7MP2 sensibly adjusts W until the relative error is within tolerance The number of iterations needed to converge to a solution depends on a variety of factors and is not known beforehand The while command is ideal for such situations while expression statements end While the expression is true the statements are continually repeated To demonstrate CH7MP2 consider the 90 essential bandwidth W for a pulse of 1 second duration Typing WEWCH7MP21090001 returns an essential bandwidth W 53014 that contains 8997 of the energy Reducing the error tolerance improves the estimate CH7MP2109000005 returns an essential bandwidth W 53321 that contains 9000 of the energy These essential bandwidth calculations are consistent with estimates presented after Ex 72 793 Spectral Sampling Consider a signal with finite duration τ A periodic signal xT0t is constructed by repeating xt every T0 seconds where T0 τ From Eq 75 we can write the Fourier series coefficients of xT0t as Dn 1T0Xn2πT0 Put another way the Fourier series coefficients are obtained by sampling the spectrum Xω By using spectral sampling it is simple to determine the Fourier series coefficients for an arbitrary dutycycle squarepulse periodic signal The square pulse xt recttτ has spectrum Xω τ sincωτ2 Thus the nth Fourier coefficient of the periodic extension xT0t is Dn τT0sincnπτT0 As in Ex 64 τ π and T0 2π provide a squarepulse periodic signal The Fourier coefficients are determined by tau pi T0 2pi n 010 Dn tauT0MS7P1npitauT0 stemnDn xlabeln ylabelDn axis05 105 02 055 The results shown in Fig 750 agree with Fig 66b Doubling the period to T0 4π effectively doubles the density of spectral samples and halves the spectral amplitude as shown in Fig 751 As T0 increases the spectral sampling becomes progressively finer while the amplitude becomes infinitesimal An evolution of the Fourier series toward the Fourier integral is seen by allowing the period T0 to become large Figure 752 shows the result for T0 40π If T0 τ the signal xT0 is a constant and the spectrum should concentrate energy at dc In this case the sinc function is sampled at the zero crossings and Dn 0 for all n not equal to 0 Only the sample corresponding to n 0 is nonzero indicating a dc signal as expected It is a simple matter to modify the previous code to verify this case 07LathiC07 2017925 1917 page 762 83 762 CHAPTER 7 CONTINUOUSTIME SIGNAL ANALYSIS THE FOURIER TRANSFORM 06 04 02 0 02 04 t 0 05 1 wKt Rectangular Hamming Blackman Figure 753 Specialcase unitduration Kaiser windows Figure 753 shows the three specialcase unitduration Kaiser windows generated by t 0600106 T 1 plottCH7MP3tTrktCH7MP3tThamktCH7MP3tTbk axis06 06 1 11 xlabelt ylabelwKt legendRectangularHammingBlackmanLocationEastOutside 710 SUMMARY In Ch 6 we represented periodic signals as a sum of everlasting sinusoids or exponentials Fourier series In this chapter we extended this result to aperiodic signals which are represented by the Fourier integral instead of the Fourier series An aperiodic signal xt may be regarded as a periodic signal with period T0 so that the Fourier integral is basically a Fourier series with a fundamental frequency approaching zero Therefore for aperiodic signals the Fourier spectra are continuous This continuity means that a signal is represented as a sum of sinusoids or exponentials of all frequencies over a continuous frequency interval The Fourier transform Xω therefore is the spectral density per unit bandwidth in hertz An everpresent aspect of the Fourier transform is the duality between time and frequency which also implies duality between the signal xt and its transform Xω This duality arises because of nearsymmetrical equations for direct and inverse Fourier transforms The duality principle has farreaching consequences and yields many valuable insights into signal analysis The scaling property of the Fourier transform leads to the conclusion that the signal bandwidth is inversely proportional to signal duration signal width Time shifting of a signal does not change its amplitude spectrum but it does add a linear phase component to its spectrum Multiplication of a signal by an exponential ejω0t shifts the spectrum to the right by ω0 In practice spectral shifting is achieved by multiplying a signal by a sinusoid such as cosω0t rather than the exponential ejω0t This process is known as amplitude modulation Multiplication of two signals results in convolution of their spectra whereas convolution of two signals results in multiplication of their spectra For an LTIC system with the frequency response Hω the input and output spectra Xω and Yω are related by the equation Yω XωHω This is valid only for asymptotically stable systems It also applies to marginally stable systems if the input does not contain a finiteamplitude 08LathiC08 2017925 1554 page 776 1 C H A P T E R SAMPLING THE BRIDGE FROM CONTINUOUS TO DISCRETE 8 A continuoustime signal can be processed by applying its samples through a discretetime system For this purpose it is important to maintain the signal sampling rate high enough to permit the reconstruction of the original signal from these samples without error or with an error within a given tolerance The necessary quantitative framework for this purpose is provided by the sampling theorem derived in Sec 81 Sampling theory is the bridge between the continuoustime and discretetime worlds The information inherent in a sampled continuoustime signal is equivalent to that of a discretetime signal A sampled continuoustime signal is a sequence of impulses while a discretetime signal presents the same information as a sequence of numbers These are basically two different ways of presenting the same data Clearly all the concepts in the analysis of sampled signals apply to discretetime signals We should not be surprised to see that the Fourier spectra of the two kinds of signal are also the same within a multiplicative constant 81 THE SAMPLING THEOREM We now show that a real signal whose spectrum is bandlimited to B Hz Xω 0 for ω 2πB can be reconstructed exactly without any error from its samples taken uniformly at a rate fs 2B samples per second In other words the minimum sampling frequency is fs 2B Hz To prove the sampling theorem consider a signal xt Fig 81a whose spectrum is bandlimited to B Hz Fig 81b For convenience spectra are shown as functions of ω as well as of f hertz Sampling xt at a rate of fs Hz fs samples per second can be accomplished by multiplying xt by an impulse train δTt Fig 81c consisting of unit impulses repeating periodically every T seconds where T 1fs The schematic of a sampler is shown in Fig 81d The resulting sampled signal xt is shown in Fig 81e The sampled signal consists of impulses The theorem stated here and proved subsequently applies to lowpass signals A bandpass signal whose spectrum exists over a frequency band fc B2 f fc B2 has a bandwidth of B Hz Such a signal is uniquely determined by 2B samples per second In general the sampling scheme is a bit more complex in this case It uses two interlaced sampling trains each at a rate of B samples per second See for example 1 The spectrum Xω in Fig 81b is shown as real for convenience However our arguments are valid for complex Xω as well 776 08LathiC08 2017925 1554 page 781 6 81 The Sampling Theorem 781 from Xω using an ideal lowpass filter of bandwidth 5 Hz Fig 82f Finally in the last case of oversampling sampling rate 20 Hz the spectrum Xω consists of nonoverlapping repetitions of 1TXω repeating every 20 Hz with empty bands between successive cycles Fig 82h Hence Xω can be recovered from Xω by using an ideal lowpass filter or even a practical lowpass filter shown dashed in Fig 82h DRILL 81 Nyquist Sampling Find the Nyquist rate and the Nyquist sampling interval for the signals sinc100πt and sinc100πt sinc50πt ANSWERS The Nyquist sampling interval is 001 s and the Nyquist sampling rate is 100 Hz for both signals FOR SKEPTICS ONLY Rare is the reader who at first encounter is not skeptical of the sampling theorem It seems impossible that Nyquist samples can define the one and the only signal that passes through those sample values We can easily picture infinite number of signals passing through a given set of samples However among all these infinite number of signals only one has the minimum bandwidth B 12T Hz where T is the sampling interval See Prob 8215 To summarize for a given set of samples taken at a rate fs Hz there is only one signal of bandwidth B fs2 that passes through those samples All other signals that pass through those samples have bandwidth higher than fs2 and the samples are subNyquist rate samples for those signals 811 Practical Sampling In proving the sampling theorem we assumed ideal samples obtained by multiplying a signal xt by an impulse train that is physically unrealizable In practice we multiply a signal xt by a train of pulses of finite width depicted in Fig 83c The sampler is shown in Fig 83d The sampled signal xt is illustrated in Fig 83e We wonder whether it is possible to recover or reconstruct xt from this xt Surprisingly the answer is affirmative provided the sampling rate is not below the Nyquist rate The signal xt can be recovered by lowpass filtering xt as if it were sampled by impulse train The filter should have a constant gain between 0 and 5 Hz and zero gain beyond 10 Hz In practice the gain beyond 10 Hz can be made negligibly small but not zero 08LathiC08 2017925 1554 page 788 13 788 CHAPTER 8 SAMPLING THE BRIDGE FROM CONTINUOUS TO DISCRETE where the sampling interval T is the Nyquist interval for xt that is T 12B Because we are given the Nyquist sample values we use the interpolation formula of Eq 86 to construct xt from its samples Since all but one of the Nyquist samples are zero only one term corresponding to n 0 in the summation on the righthand side of Eq 86 survives Thus xt sinc2πBt This signal is illustrated in Fig 86b Observe that this is the only signal that has a bandwidth B Hz and the sample values x0 1 and xnT 0n 0 No other signal satisfies these conditions 821 Practical Difficulties in Signal Reconstruction Consider the signal reconstruction procedure illustrated in Fig 87a If xt is sampled at the Nyquist rate fs 2B Hz the spectrum Xω consists of repetitions of Xω without any gap between successive cycles as depicted in Fig 87b To recover xt from xt we need to pass the sampled signal xt through an ideal lowpass filter shown dotted in Fig 87b As seen in Sec 75 such a filter is unrealizable it can be closely approximated only with infinite time delay in the response In other words we can recover the signal xt from its samples with infinite time delay A practical solution to this problem is to sample the signal at a rate higher than the Nyquist rate fs 2B or ωs 4πB The result is Xω consisting of repetitions of Xω with a finite bandgap between successive cycles as illustrated in Fig 87c Now we can recover Xω from Xω using a lowpass filter with a gradual cutoff characteristic shown dotted in Fig 87c But even in this case if the unwanted spectrum is to be suppressed the filter gain must be zero beyond some frequency see Fig 87c According to the PaleyWiener criterion Eq 743 it is impossible to realize even this filter The only advantage in this case is that the required filter can be closely approximated with a smaller time delay All this means that it is impossible in practice to recover a bandlimited signal xt exactly from its samples even if the sampling rate is higher than the Nyquist rate However as the sampling rate increases the recovered signal approaches the desired signal more closely THE TREACHERY OF ALIASING There is another fundamental practical difficulty in reconstructing a signal from its samples The sampling theorem was proved on the assumption that the signal xt is bandlimited All practical signals are timelimited that is they are of finite duration or width We can demonstrate see Prob 8220 that a signal cannot be timelimited and bandlimited simultaneously If a signal is timelimited it cannot be bandlimited and vice versa but it can be simultaneously nontimelimited and nonbandlimited Clearly all practical signals which are necessarily timelimited are nonbandlimited as shown in Fig 88a they have infinite bandwidth and the spectrum Xω consists of overlapping cycles of Xω repeating every fs Hz the sampling frequency as 08LathiC08 2017925 1554 page 789 14 82 Signal Reconstruction 789 c 2pB vs vs b v v a Ideal lowpass filter cutoff B Hz Sampler dTt xt xt Xv Xv xt Figure 87 a Signal reconstruction from its samples b Spectrum of a signal sampled at the Nyquist rate c Spectrum of a signal sampled above the Nyquist rate illustrated in Fig 88b Because of infinite bandwidth in this case the spectral overlap is unavoidable regardless of the sampling rate Sampling at a higher rate reduces but does not eliminate overlapping between repeating spectral cycles Because of the overlapping tails Xω no longer has complete information about Xω and it is no longer possible even theoretically to recover xt exactly from the sampled signal xt If the sampled signal is passed through an ideal lowpass filter of cutoff frequency fs2 Hz the output is not Xω but Xaω Fig 88c which is a version of Xω distorted as a result of two separate causes 1 The loss of the tail of Xω beyond f fs2 Hz 2 The reappearance of this tail inverted or folded onto the spectrum Note that the spectra cross at frequency fs2 12T Hz This frequency is called the folding frequency Figure 88b shows that from the infinite number of repeating cycles only the neighboring spectral cycles overlap This is a somewhat simplified picture In reality all the cycles overlap and interact with every other cycle because of the infinite width of all practical signal spectra Fortunately all practical spectra also must decay at higher frequencies This results in insignificant amount of interference from cycles other than the immediate neighbors When such an assumption is not justified aliasing computations become little more involved 08LathiC08 2017925 1554 page 791 16 82 Signal Reconstruction 791 The spectrum may be viewed as if the lost tail is folding back onto itself at the folding frequency For instance a component of frequency fs2 fz shows up as or impersonates a component of lower frequency fs2 fz in the reconstructed signal Thus the components of frequencies above fs2 reappear as components of frequencies below fs2 This tail inversion known as spectral folding or aliasing is shown shaded in Fig 88b and also in Fig 88c In the process of aliasing not only are we losing all the components of frequencies above the folding frequency fs2 Hz but these very components reappear aliased as lowerfrequency components as shown in Figs 88b and 88c Such aliasing destroys the integrity of the frequency components below the folding frequency fs2 as depicted in Fig 88c The aliasing problem is analogous to that of an army with a platoon that has secretly defected to the enemy side The platoon is however ostensibly loyal to the army The army is in double jeopardy First the army has lost this platoon as a fighting force In addition during actual fighting the army will have to contend with sabotage by the defectors and will have to find another loyal platoon to neutralize the defectors Thus the army has lost two platoons in nonproductive activity DEFECTORS ELIMINATED THE ANTIALIASING FILTER If you were the commander of the betrayed army the solution to the problem would be obvious As soon as the commander got wind of the defection he would incapacitate by whatever means the defecting platoon before the fighting begins This way he loses only one the defecting platoon This is a partial solution to the double jeopardy of betrayal and sabotage a solution that partly rectifies the problem and cuts the losses to half We follow exactly the same procedure The potential defectors are all the frequency components beyond the folding frequency fs2 12T Hz We should eliminate suppress these components from xt before sampling xt Such suppression of higher frequencies can be accomplished by an ideal lowpass filter of cutoff fs2 Hz as shown in Fig 88d This is called the antialiasing filter Figure 88d also shows that antialiasing filtering is performed before sampling Figure 88e shows the sampled signal spectrum dotted and the reconstructed signal Xaaω when an antialiasing scheme is used An antialiasing filter essentially bandlimits the signal xt to fs2 Hz This way we lose only the components beyond the folding frequency fs2 Hz These suppressed components now cannot reappear to corrupt the components of frequencies below the folding frequency Clearly use of an antialiasing filter results in the reconstructed signal spectrum Xaaω Xω for f fs2 Thus although we lost the spectrum beyond fs2 Hz the spectrum for all the frequencies below fs2 remains intact The effective aliasing distortion is cut in half owing to elimination of folding We stress again that the antialiasing operation must be performed before the signal is sampled An antialiasing filter also helps to reduce noise Noise generally has a wideband spectrum and without antialiasing the aliasing phenomenon itself will cause the noise lying outside the desired band to appear in the signal band Antialiasing suppresses the entire noise spectrum beyond frequency fs2 The antialiasing filter being an ideal filter is unrealizable In practice we use a steep cutoff filter which leaves a sharply attenuated spectrum beyond the folding frequency fs2 08LathiC08 2017925 1554 page 793 18 82 Signal Reconstruction 793 This discussion again shows that sampling a sinusoid of frequency f aliasing can be avoided if the sampling rate fs 2f Hz 0 f fs 2 or 0 ω π T Violating this condition leads to aliasing implying that the samples appear to be those of a lowerfrequency signal Because of this loss of identity it is impossible to reconstruct the signal faithfully from its samples GENERAL CONDITION FOR ALIASING IN SINUSOIDS We can generalize the foregoing result by showing that samples of a sinusoid of frequency f0 are identical to those of a sinusoid of frequency f0 mfs Hz integer m where fs is the sampling frequency The samples of cos2πf0 mfst are cos 2πf0 mfsnT cos2πf0nT 2πmn cos 2πf0nT The result follows because mn is an integer and fsT 1 This result shows that sinusoids of frequencies that differ by an integer multiple of fs result in identical set of samples In other words samples of sinusoids separated by frequency fs Hz are identical This implies that samples of sinusoids in any frequency band of fs Hz are unique that is no two sinusoids in that band have the same samples when sampled at a rate fs Hz For instance frequencies in the band from fs2 to fs2 have unique samples at the sampling rate fs This band is called the fundamental band Recall also that fs2 is the folding frequency From the discussion thus far we conclude that if a continuoustime sinusoid of frequency f Hz is sampled at a rate of fs Hz sampless the resulting samples would appear as samples of a continuoustime sinusoid of frequency fa in the fundamental band where fa f mfs fs 2 fa fs 2 m an integer 87 The frequency fa lies in the fundamental band from fs2 to fs2 Figure 89a shows the plot of fa versus f where f is the actual frequency and fa is the corresponding fundamental band frequency whose samples are identical to those of the sinusoid of frequency f when the sampling rate is fs Hz Recall however that the sign change of a frequency does not alter the actual frequency of the waveform This is because cosωat θ cosωat θ Clearly the apparent frequency of a sinusoid of frequency fa is also fa However its phase undergoes a sign change This means the apparent frequency of any sampled sinusoid lies in the range from 0 to fs2 Hz To summarize if a continuoustime sinusoid of frequency f Hz is sampled at a rate of fs Hz samplessecond the resulting samples would appear as samples of a continuoustime sinusoid of frequency fa that lies in the band from 0 to fs2 According to Eq 87 fa f mfs fa fs 2 m an integer 08LathiC08 2017925 1554 page 796 21 796 CHAPTER 8 SAMPLING THE BRIDGE FROM CONTINUOUS TO DISCRETE d Here f 2400 Hz can be expressed as 2400 400 2 1000 so that fa 400 Hence the aliased frequency is 400 Hz and there is no sign change for the phase The apparent sinusoid is cos2πft θ with f 400 We could have found these answers directly from Fig 89b For example for case b we read fa 400 corresponding to f 600 Moreover f 600 lies in the shaded belt Hence there is a phase sign change DRILL 83 A Case of Identical Sampled Sinusoids Show that samples of 90 Hz and 110 Hz sinusoids of the form cosωt are identical when sampled at a rate 200 Hz DRILL 84 Apparent Frequency of Sampled Sinusoids A sinusoid of frequency f0 Hz is sampled at a rate of 100 Hz Determine the apparent frequency of the samples if f0 is a 40 Hz b 60 Hz c 140 Hz and d 160 Hz ANSWERS All four cases have an apparent frequency of 40 Hz 822 Some Applications of the Sampling Theorem The sampling theorem is very important in signal analysis processing and transmission because it allows us to replace a continuoustime signal with a discrete sequence of numbers Processing a continuoustime signal is therefore equivalent to processing a discrete sequence of numbers Such processing leads us directly into the area of digital filtering In the field of communication the transmission of a continuoustime message reduces to the transmission of a sequence of numbers by means of pulse trains The continuoustime signal xt is sampled and sample values are used to modify certain parameters of a periodic pulse train We may vary the amplitudes Fig 811b widths Fig 811c or positions Fig 811d of the pulses in proportion to the sample values of the signal xt Accordingly we may have pulseamplitude modulation PAM pulsewidth modulation PWM or pulseposition modulation PPM The most important form of pulse modulation today is pulsecode modulation PCM discussed in Sec 83 in connection with Fig 814b In all these cases instead of transmitting xt we transmit the corresponding pulsemodulated signal At the receiver we read the information of the pulsemodulated signal and reconstruct the analog signal xt 08LathiC08 2017925 1554 page 797 22 82 Signal Reconstruction 797 t t t t a b c Pulse locations are the same but their widths change d Pulse widths are the same but their locations change xt Figure 811 Pulsemodulated signals a The signal b The PAM signal c The PWM PDM signal d The PAM signal One advantage of using pulse modulation is that it permits the simultaneous transmission of several signals on a timesharing basistimedivision multiplexing TDM Because a pulsemodulated signal occupies only a part of the channel time we can transmit several pulsemodulated signals on the same channel by interweaving them Figure 812 shows the TDM of two PAM signals In this manner we can multiplex several signals on the same channel by reducing pulse widths Digital signals also offer an advantage in the area of communications where signals must travel over distances Transmission of digital signals is more rugged than that of analog signals because digital signals can withstand channel noise and distortion much better as long as the noise Another method of transmitting several baseband signals simultaneously is frequencydivision multiplexing FDM discussed in Sec 774 In FDM various signals are multiplexed by sharing the channel bandwidth The spectrum of each message is shifted to a specific band not occupied by any other signal The information of various signals is located in nonoverlapping frequency bands of the channel Fig 745 In a way TDM and FDM are duals of each other 08LathiC08 2017925 1554 page 805 30 85 Numerical Computation of the Fourier Transform 805 85 NUMERICAL COMPUTATION OF THE FOURIER TRANSFORM THE DISCRETE FOURIER TRANSFORM Numerical computation of the Fourier transform of xt requires sample values of xt because a digital computer can work only with discrete data sequence of numbers Moreover a computer can compute Xω only at some discrete values of ω samples of Xω We therefore need to relate the samples of Xω to samples of xt This task can be accomplished by using the results of the two sampling theorems developed in Secs 81 and 84 We begin with a timelimited signal xt Fig 816a and its spectrum Xω Fig 816b Since xt is timelimited Xω is nonbandlimited For convenience we shall show all spectra as functions of the frequency variable f in hertz rather than ω According to the sampling theorem the spectrum Xω of the sampled signal xt consists of Xω repeating every fs Hz where fs 1T as depicted in Fig 816d In the next step the sampled signal in Fig 816c is repeated periodically every T0 seconds as illustrated in Fig 816e According to the spectral sampling theorem such an operation results in sampling the spectrum at a rate of T0 samplesHz This sampling rate means that the samples are spaced at f0 1T0 Hz as depicted in Fig 816f The foregoing discussion shows that when a signal xt is sampled and then periodically repeated the corresponding spectrum is also sampled and periodically repeated Our goal is to relate the samples of xt to the samples of Xω NUMBER OF SAMPLES One interesting observation from Figs 816e and 816f is that N0 the number of samples of the signal in Fig 816e in one period T0 is identical to N 0 the number of samples of the spectrum in Fig 816f in one period fs To see this we notice that N0 T0 T N 0 fs f0 fs 1 T and f0 1 T0 810 Using these relations we see that N0 T0 T fs f0 N 0 ALIASING AND LEAKAGE IN NUMERICAL COMPUTATION Figure 816f shows the presence of aliasing in the samples of the spectrum Xω This aliasing error can be reduced as much as desired by increasing the sampling frequency fs decreasing the sampling interval T 1fs The aliasing can never be eliminated for timelimited xt however because its spectrum Xω is nonbandlimited Had we started with a signal having a bandlimited spectrum Xω there would be no aliasing in the spectrum in Fig 816f Unfortunately such a signal is nontimelimited and its repetition in Fig 816e would result in signal overlapping aliasing in the time domain In this case we shall have to contend with errors in signal There is a multiplying constant 1T for the spectrum in Fig 816d see Eq 82 but this is irrelevant to our discussion here 08LathiC08 2017925 1554 page 811 36 85 Numerical Computation of the Fourier Transform 811 ZERO PADDING DOES NOT IMPROVE ACCURACY OR RESOLUTION Actually we are not observing Xω through a picket fence We are observing a distorted version of Xω resulting from the truncation of xt Hence we should keep in mind that even if the fence were transparent we would see a reality distorted by aliasing Seeing through the picket fence just gives us an imperfect view of the imperfectly represented reality Zero padding only allows us to look at more samples of that imperfect reality It can never reduce the imperfection in what is behind the fence The imperfection which is caused by aliasing can be lessened only by reducing the sampling interval T Observe that reducing T also increases N0 the number of samples and is like increasing the number of pickets while reducing their width But in this case the reality behind the fence is also better dressed and we see more of it EXAMPLE 87 Number of Samples and Frequency Resolution A signal xt has a duration of 2 ms and an essential bandwidth of 10 kHz It is desirable to have a frequency resolution of 100 Hz in the DFT f0 100 Determine N0 To have f0 100 Hz the effective signal duration T0 must be T0 1 f0 1 100 10 ms Since the signal duration is only 2 ms we need zero padding over 8 ms Also B 10000 Hence fs 2B 20000 and T 1fs 50 µs Furthermore N0 fs f0 20000 100 200 The fast Fourier transform FFT algorithm discussed later see Sec 86 is used to compute DFT where it proves convenient although not necessary to select N0 as a power of 2 that is N0 2n n integer Let us choose N0 256 Increasing N0 from 200 to 256 can be used to reduce aliasing error by reducing T to improve resolution by increasing T0 using zero padding or a combination of both Reducing Aliasing Error We maintain the same T0 so that f0 100 Hence fs N0f0 256 100 25600 and T 1 fs 39µs Thus increasing N0 from 200 to 256 permits us to reduce the sampling interval T from 50 µs to 39 µs while maintaining the same frequency resolution f0 100 Improving Resolution Here we maintain the same T 50 µs which yields T0 N0T 25650 106 128 ms and f0 1 T0 78125 Hz 08LathiC08 2017925 1554 page 812 37 812 CHAPTER 8 SAMPLING THE BRIDGE FROM CONTINUOUS TO DISCRETE Thus increasing N0 from 200 to 256 can improve the frequency resolution from 100 to 78125 Hz while maintaining the same aliasing error T 50 µs Combination of Reducing Aliasing Error and Improving Resolution To simultaneously reduce alias error and improve resolution we could choose T 45 µs and T0 115 ms so that f0 8696 Hz Many other combinations exist as well EXAMPLE 88 DFT to Compute the Fourier Transform of an Exponential Use the DFT to compute samples of the Fourier transform of e2tut Plot the resulting Fourier spectra We first determine T and T0 The Fourier transform of e2tut is 1jω 2 This lowpass signal is not bandlimited In Sec 76 we used the energy criterion to compute the essential bandwidth of a signal Here we shall present a simpler but workable alternative to the energy criterion The essential bandwidth of a signal will be taken as the frequency at which Xω drops to 1 of its peak value see the footnote on page 736 In this case the peak value occurs at ω 0 where X0 05 Observe that Xω 1 ω2 4 1 ω ω 2 Also 1 of the peak value is 001 05 0005 Hence the essential bandwidth B is at ω 2πB where Xω 1 2πB 0005 B 100 π Hz and from Eq 816 T 1 2B π 200 0015708 Had we used 1 energy criterion to determine the essential bandwidth following the procedure in Ex 720 we would have obtained B 2026 Hz which is somewhat smaller than the value just obtained by using the 1 amplitude criterion The second issue is to determine T0 Because the signal is not timelimited we have to truncate it at T0 such that xT0 1 A reasonable choice would be T0 4 because x4 e8 0000335 1 The result is N0 T0T 2546 which is not a power of 2 Hence we choose T0 4 and T 0015625 164 yielding N0 256 which is a power of 2 Note that there is a great deal of flexibility in determining T and T0 depending on the accuracy desired and the computational capacity available We could just as well have chosen T 003125 yielding N0 128 although this choice would have given a slightly higher aliasing error 08LathiC08 2017925 1554 page 814 39 814 CHAPTER 8 SAMPLING THE BRIDGE FROM CONTINUOUS TO DISCRETE In this example we knew Xω beforehand hence we could make intelligent choices for B or the sampling frequency fs In practice we generally do not know Xω beforehand In fact that is the very thing we are trying to determine In such a case we must make an intelligent guess for B or fs from circumstantial evidence We should then continue reducing the value of T and recomputing the transform until the result stabilizes within the desired number of significant digits USING MATLAB TO COMPUTE AND PLOT THE RESULTS Let us now use MATLAB to confirm the results of this example First parameters are defined and MATLABs fft command is used to compute the DFT T0 4 N0 256 T T0N0 t 0TTN01 x Texp2t x1 x12 Xr fftx r N02N021 omegar r2piT0 The true Fourier transform is also computed for comparison omega linspacepiTpiT5001 X 1jomega2 For clarity we display spectrum over a restricted frequency range subplot121 stemomegarfftshiftabsXrk lineomegaabsXcolor0 0 0 axis001 44 001 051 xlabelomega ylabelXomega subplot122 stemomegarfftshiftangleXrk lineomegaangleXcolor0 0 0 axis001 44 pi2001 001 xlabelomega ylabelangle Xomega The results shown in Fig 818 match the earlier results shown in Fig 817 0 01 02 03 04 05 Xω 0 10 20 30 40 ω ω 0 10 20 30 40 15 1 05 0 Xω Figure 818 MATLABcomputed DFT of an exponential signal e2tut 08LathiC08 2017925 1554 page 815 40 85 Numerical Computation of the Fourier Transform 815 EXAMPLE 89 DFT to Compute the Fourier Transform of a Rectangular Pulse Use the DFT to compute the Fourier transform of 8rectt This gate function and its Fourier transform are illustrated in Figs 819a and 819b To determine the value of the sampling interval T we must first decide on the essential bandwidth B In Fig 819b we see that Xω decays rather slowly with ω Hence the essential bandwidth B is rather large For instance at B 155 Hz 9739 rads Xω 01643 which is about 2 of the peak at X0 Hence the essential bandwidth is well above 16 Hz if we use the 1 of the peak amplitude criterion for computing the essential bandwidth However we shall deliberately take B 4 for two reasons to show the effect of aliasing and because the use of B 4 would give an enormous number of samples which could not be conveniently displayed on the page without losing sight of the essentials Thus we shall intentionally accept approximation to graphically clarify the concepts of the DFT The choice of B 4 results in the sampling interval T 12B 18 Looking again at the spectrum in Fig 819b we see that the choice of the frequency resolution f0 14 Hz is reasonable Such a choice gives us four samples in each lobe of Xω In this case T0 1f0 4 seconds and N0 T0T 32 The duration of xt is only 1 second We must repeat it every 4 seconds T0 4 as depicted in Fig 819c and take samples every 18 second This choice yields 32 samples N0 32 Also xn TxnT 1 8xnT Since xt 8rect t the values of xn are 1 0 or 05 at the points of discontinuity as illustrated in Fig 819c where xn is depicted as a function of t as well as n for convenience In the derivation of the DFT we assumed that xt begins at t 0 Fig 816a and then took N0 samples over the interval 0 T0 In the present case however xt begins at 12 This difficulty is easily resolved when we realize that the DFT obtained by this procedure is actually the DFT of xn repeating periodically every T0 seconds Figure 819c clearly indicates that periodic repeating the segment of xn over the interval from 2 to 2 seconds yields the same signal as the periodic repeating the segment of xn over the interval from 0 to 4 seconds Hence the DFT of the samples taken from 2 to 2 seconds is the same as that of the samples taken from 0 to 4 seconds Therefore regardless of where xt starts we can always take the samples of xt and its periodic extension over the interval from 0 to T0 In the present example the 32 sample values are xn 1 0 n 3 and 29 n 31 0 5 n 27 05 n 428 08LathiC08 2017925 1554 page 816 41 816 CHAPTER 8 SAMPLING THE BRIDGE FROM CONTINUOUS TO DISCRETE Figure 819 Discrete Fourier transform of a gate pulse 08LathiC08 2017925 1554 page 818 43 818 CHAPTER 8 SAMPLING THE BRIDGE FROM CONTINUOUS TO DISCRETE xlabelomega ylabelXomega axis tight The result shown in Fig 820 matches the earlier result shown in Fig 819d The DFT approximation does not perfectly follow the true Fourier transform especially at high frequencies because the parameter B is deliberately set too small 25 20 15 10 5 ω 0 5 10 15 20 25 0 2 4 6 8 Xω Figure 820 MATLABcomputed DFT of a gate pulse 851 Some Properties of the DFT The discrete Fourier transform is basically the Fourier transform of a sampled signal repeated periodically Hence the properties derived earlier for the Fourier transform apply to the DFT as well LINEARITY If xn Xr and gn Gr then a1xn a2gn a1Xr a2Gr The proof is trivial CONJUGATE SYMMETRY From the conjugation property xt Xω we have x n X r From this equation and the timereversal property we obtain x n X r 08LathiC08 2017925 1554 page 823 48 85 Numerical Computation of the Fourier Transform 823 that Hr must be repeated every 8 Hz or 16π rads see Fig 822c The resulting 32 samples of Hr over 0 ω 16π are as follows Hr 1 0 r 7 and 25 r 31 0 9 r 23 05 r 824 We multiply Xr with Hr The desired output signal samples yn are found by taking the inverse DFT of XrHr The resulting output signal is illustrated in Fig 822d It is quite simple to verify the results of this filtering example using MATLAB First parameters are defined and MATLABs fft command is used to compute the DFT of xn T0 4 N0 32 T T0N0 n 0N01 r n xn ones14 05 zeros123 05 ones13 Xr fftxn The DFT of the filters output is just the product of the filter response Hr and the input DFT Xr The output yn is obtained using the ifft command and then plotted Hr ones18 05 zeros115 05 ones17 Yr HrXr yn ifftYr clf stemnrealynk xlabeln ylabelyn axis0 31 1 11 The result shown in Fig 823 matches the earlier result shown in Fig 822d Recall this DFTbased approach shows the samples yn of the filter output yt sampled in this case at a rate T 1 8 over 0 n N0 1 31 when the input pulse xt is periodically replicated to form samples xn see Fig 819c 0 5 10 15 20 25 30 n 0 02 04 06 08 1 yn Figure 823 Using MATLAB and the DFT to determine filter output 08LathiC08 2017925 1554 page 827 52 87 MATLAB The Discrete Fourier Transform 827 Thus an N0point DFT can be computed by combining the two N02point DFTs as in Eq 827 These equations can be represented conveniently by the signal flow graph depicted in Fig 824 This structure is known as a butterfly Figure 825a shows the implementation of Eq 824 for the case of N0 8 The next step is to compute the N02point DFTs Gr and Hr We repeat the same procedure by dividing gn and hn into two N04point sequences corresponding to the even and oddnumbered samples Then we continue this process until we reach the onepoint DFT These steps for the case of N0 8 are shown in Figs 825a 825b and 825c Figure 825c shows that the twopoint DFTs require no multiplication To count the number of computations required in the first step assume that Gr and Hr are known Equation 827 clearly shows that to compute all the N0 points of the Xr we require N0 complex additions and N02 complex multiplications corresponding to Wr N0Hr In the second step to compute the N02point DFT Gr from the N04point DFT we require N02 complex additions and N04 complex multiplications We require an equal number of computations for Hr Hence in the second step there are N0 complex additions and N02 complex multiplications The number of computations required remains the same in each step Since a total of log2 N0 steps is needed to arrive at a onepoint DFT we require conservatively a total of N0 log2 N0 complex additions and N02log2 N0 complex multiplications to compute the N0point DFT Actually as Fig 825c shows many multiplications are multiplications by 1 or 1 which further reduces the number of computations The procedure for obtaining IDFT is identical to that used to obtain the DFT except that WN0 ej2πN0 instead of ej2πN0 in addition to the multiplier 1N0 Another FFT algorithm the decimationinfrequency algorithm is similar to the decimationintime algorithm The only difference is that instead of dividing xn into two sequences of even and oddnumbered samples we divide xn into two sequences formed by the first N02 and the last N02 samples proceeding in the same way until a singlepoint DFT is reached in log2 N0 steps The total number of computations in this algorithm is the same as that in the decimationintime algorithm 87 MATLAB THE DISCRETE FOURIER TRANSFORM As an idea the discrete Fourier transform DFT has been known for hundreds of years Practical computing devices however are responsible for bringing the DFT into common use MATLAB is capable of DFT computations that would have been impractical just a few decades ago 871 Computing the Discrete Fourier Transform The MATLAB command fftx computes the DFT of a vector x that is defined over 0 n N0 1 Problem 871 considers how to scale the DFT to accommodate signals that do not begin at n 0 As its name suggests the function fft uses the computationally more efficient fast Fourier transform algorithm when it is appropriate to do so The inverse DFT is easily computed by using the ifft function Actually N02 is a conservative figure because some multiplications corresponding to the cases of Wr N0 1j and so on are eliminated 08LathiC08 2017925 1554 page 828 53 828 CHAPTER 8 SAMPLING THE BRIDGE FROM CONTINUOUS TO DISCRETE To illustrate MATLABs DFT capabilities consider 50 points of a 10 Hz sinusoid sampled at fs 50 Hz and scaled by T 1fs T 150 N0 50 n 0N01 x Tcos2pi10nT In this case the vector x contains exactly 10 cycles of the sinusoid The fft command computes the DFT X fftx Since the DFT is both discrete and periodic fft needs to return only the N0 discrete values contained in the single period 0 f fs While Xr can be plotted as a function of r it is more convenient to plot the DFT as a function of frequency f A frequency vector in hertz is created by using N0 and T f 0N01TN0 stemfabsXk axis0 50 005 055 xlabelf Hz ylabelXf As expected Fig 826 shows content at a frequency of 10 Hz Since the timedomain signal is real Xf is conjugate symmetric Thus content at 10 Hz implies equal content at 10 Hz The content visible at 40 Hz is an alias of the 10 Hz content Often it is preferred to plot a DFT over the principal frequency range fs2 f fs2 The MATLAB function fftshift properly rearranges the output of fft to accomplish this task stemf1T2fftshiftabsXk axis25 25 005 055 xlabelf Hz ylabelXf When we use fftshift the conjugate symmetry that accompanies the DFT of a real signal becomes apparent as shown in Fig 827 Since DFTs are generally complexvalued the magnitude plots of Figs 826 and 827 offer only half the picture the signals phase spectrum shown in Fig 828 completes it stemf1T2fftshiftangleXk axis25 25 11pi 11pi xlabelf Hz ylabelangle Xf 0 5 10 15 20 25 30 35 40 45 50 f Hz 0 02 04 Xf Figure 826 Xf computed over 0 f 50 by using fft 08LathiC08 2017925 1554 page 830 55 830 CHAPTER 8 SAMPLING THE BRIDGE FROM CONTINUOUS TO DISCRETE 25 20 15 10 5 0 5 10 15 20 25 f Hz 0 05 1 Yf Figure 829 Yf using 50 data points 5 6 7 8 9 10 11 12 13 14 15 f Hz 0 05 1 Yzpf Figure 830 Yzpf over 5 f 15 using 50 data points padded with 550 zeros In this case the vector y contains a noninteger number of cycles Figure 829 shows the significant frequency leakage that results Also notice that since yn is not real the DFT is not conjugate symmetric In this example the discrete DFT frequencies do not include the actual 10 1 3 Hz frequency of the signal Thus it is difficult to determine the signals frequency from Fig 829 To improve the picture the signal is zeropadded to 12 times its original length yzp yzeros111lengthy Yzp fftyzp fzp 012N01T12N0 stemfzp25fftshiftabsYzpk axis25 25 005 105 xlabelf Hz ylabelYzpf Figure 830 zoomed in to 5 f 15 correctly shows the peak frequency at 10 1 3 Hz and better represents the signals spectrum It is important to keep in mind that zero padding does not increase the resolution or accuracy of the DFT To return to the picket fence analogy zero padding increases the number of pickets in our fence but cannot change what is behind the fence More formally the characteristics of the sinc function such as main beam width and sidelobe levels depend on the fixed width of the pulse not on the number of zeros that follow Adding zeros cannot change the characteristics of the sinc function and thus cannot change the resolution or accuracy of the DFT Adding zeros simply allows the sinc function to be sampled more finely 08LathiC08 2017925 1554 page 832 57 832 CHAPTER 8 SAMPLING THE BRIDGE FROM CONTINUOUS TO DISCRETE otherwise dispUnrecognized quantization method return end Several MATLAB commands require discussion First the nargin function returns the number of input arguments In this program nargin is used to ensure that a correct number of inputs is supplied If the number of inputs supplied is incorrect an error message is displayed and the function terminates If only three input arguments are detected the quantization type is not explicitly specified and the program assigns the default symmetric method As with many highlevel languages such as C MATLAB supports general switchcase structures switch switchexpr case caseexpr statements otherwise statements end CH8MP1 switches among cases of the string method In this way methodspecific parameters are easily set The command lower is used to convert a string to all lowercase characters In this way strings such as SYM Sym and sym are all indistinguishable Similar to lower the MATLAB command upper converts a string to all uppercase The floor command rounds input values to the nearest integer toward minus infinity Mathematically it computes To accommodate different types of rounding MATLAB supplies three other rounding commands ceil round and fix The ceil command rounds input values to the nearest integers toward infinity the round command rounds input values toward the nearest integer the fix command rounds input values to the nearest integer toward zero For example if x 05 05 floorx yields 1 0 ceilx yields 0 1 roundx yields 1 1 and fixx yields 0 0 Finally CH8MP1 checks and if necessary corrects large values of xq that may be outside the allowable 2B levels To verify operation CH8MP1 is used to determine the transfer characteristics of a symmetric 3bit quantizer operating over 1010 x 10000110 xsq CH8MP1x103sym plotxxsqk axis10 10 105 105 grid on xlabelQuantizer input ylabelQuantizer output Figure 831 shows the results Clearly the quantized output is limited to 2B 8 levels Zero is not a quantization level for symmetric quantizers so half of the levels occur above zero and half of the levels occur below zero In fact symmetric quantizers get their name from the symmetry in quantization levels above and below zero By changing the method in CH8MP1 from sym to asym we obtain the transfer characteristics of an asymmetric 3bit quantizer as shown in Fig 832 Again the quantized output is limited to 2B 8 levels and zero is now one of the included levels With zero as a quantization A functionally equivalent structure can be written by using if elseif and else statements 08LathiC08 2017925 1554 page 833 58 87 MATLAB The Discrete Fourier Transform 833 10 5 0 5 10 Quantizer input 10 5 0 5 10 Quantizer output Figure 831 Transfer characteristics of a symmetric 3bit quantizer 10 5 0 5 10 Quantizer input 10 5 0 5 10 Quantizer output Figure 832 Transfer characteristics of an asymmetric 3bit quantizer level we need one fewer quantization level above zero than there are levels below Not surprisingly asymmetric quantizers get their name from the asymmetry in quantization levels above and below zero There is no doubt that quantization can change a signal It follows that the spectrum of a quantized signal can also change While these changes are difficult to characterize mathematically they are easy to investigate by using MATLAB Consider a 1 Hz cosine sampled at fs 50 Hz over 1 second x cos2pinT X fftx T 150 N0 50 n 0N01 Upon quantizing by means of a 2bit asymmetric rounding quantizer both the signal and spectrum are substantially changed xaq CH8MP1x12asym Xaq fftxaq subplot221 stemnxk axis0 49 11 11 xlabelnylabelxn subplot222 stemf25fftshiftabsXk axis2525 1 26 xlabelfylabelXf subplot223 stemnxaqkaxis0 49 11 11 08LathiC08 2017925 1554 page 834 59 834 CHAPTER 8 SAMPLING THE BRIDGE FROM CONTINUOUS TO DISCRETE 0 10 20 30 40 n 1 0 1 xn 20 10 0 10 20 f 0 10 20 Xf 0 10 20 30 40 n 1 0 1 xaqn 20 10 0 10 20 f 0 10 20 Xaqf Figure 833 Signal and spectrum effects of quantization xlabelnylabelxaqn subplot224 stemf25fftshiftabsfftxaqk axis2525 1 26 xlabelfylabelXaqf The results are shown in Fig 833 The original signal xn appears sinusoidal and has pure spectral content at 1 Hz The asymmetrically quantized signal xaqn is significantly distorted The corresponding magnitude spectrum Xaqf is spread over a broad range of frequencies 88 SUMMARY A signal bandlimited to B Hz can be reconstructed exactly from its samples if the sampling rate fs 2B Hz the sampling theorem Such a reconstruction although possible theoretically poses practical problems such as the need for ideal filters which are unrealizable or are realizable only with infinite delay Therefore in practice there is always an error in reconstructing a signal from its samples Moreover practical signals are not bandlimited which causes an additional error aliasing error in signal reconstruction from its samples When a signal is sampled at a frequency fs Hz samples of a sinusoid of frequency fs2 x Hz appear as samples of a lower frequency fs2 x Hz This phenomenon in which higher frequencies appear as lower frequencies is known as aliasing Aliasing error can be reduced by bandlimiting a signal to fs2 Hz half the sampling frequency Such bandlimiting done prior to sampling is accomplished by an antialiasing filter that is an ideal lowpass filter of cutoff frequency fs2 Hz The sampling theorem is very important in signal analysis processing and transmission because it allows us to replace a continuoustime signal with a discrete sequence of numbers Processing a continuoustime signal is therefore equivalent to processing a discrete sequence of numbers This leads us directly into the area of digital filtering discretetime systems In the field 08LathiC08 2017925 1554 page 843 68 Problems 843 1 1 t t xt gt 1 1 2 a b 0 Figure P856 been computed derive a method to correct X to reflect an arbitrary starting time n n0 872 Consider a complex signal composed of two closely spaced complex exponentials x1n ej2πn30100 ej2πn33100 For each of the follow ing cases plot the lengthN DFT magnitude as a function of frequency fr where fr rN a Compute and plot the DFT of x1n using 10 samples 0 n 9 From the plot can both exponentials be identified Explain b Zeropad the signal from part a with 490 zeros and then compute and plot the 500point DFT Does this improve the pic ture of the DFT Explain c Compute and plot the DFT of x1n using 100 samples 0 n 99 From the plot can both exponentials be identified Explain d Zeropad the signal from part c with 400 zeros and then compute and plot the 500point DFT Does this improve the pic ture of the DFT Explain 873 Repeat Prob 872 using the complex signal x2n ej2πn30100 ej2πn315100 874 Consider a complex signal composed of a dc term and two complex exponentials y1n 1 ej2πn30100 05 ej2πn43100 For each of the following cases plot the lengthN DFT magnitude as a function of frequency fr where fr rN a Use MATLAB to compute and plot the DFT of y1n with 20 samples 0 n19 From the plot can the two nondc exponentials be identified Given the amplitude rela tion between the two the lowerfrequency peak should be twice as large as the higherfrequency peak Is this the case Explain b Zeropad the signal from part a to a total length of 500 Does this improve locating the two nondc exponential components Is the lowerfrequency peak twice as large as the higherfrequency peak Explain c MATLABs signalprocessing toolbox func tion window allows window functions to be easily generated Generate a length20 Han ning window and apply it to y1n Using this windowed function repeat parts a and b Comment on whether the window function helps or hinders the analysis 875 Repeat Prob 874 using the complex signal y2n 1 ej2πn30100 05ej2πn38100 876 This problem investigates the idea of zero padding applied in the frequency domain When asked plot the lengthN DFT magnitude as a function of frequency fr where fr rN a In MATLAB create a vector x that con tains one period of the sinusoid xn cosπ2n Plot the result How sinu soidal does the signal appear to be b Use the fft command to compute the DFT X of vector x Plot the magnitude of the DFT coefficients Do they make sense c Zeropad the DFT vector to a total length of 100 by inserting the appropriate number of zeros in the middle of the vector X Call this zeropadded DFT sequence Y Why are zeros inserted in the middle rather than the end Take the inverse DFT of Y and plot the result What similarities exist between the new signal y and the original signal x What are the differences between x and y What is the effect of zero padding in the frequency domain How is this type of zero padding similar to zero padding in the time domain d Derive a general modification to the pro cedure of zero padding in the frequency domain to ensure that the amplitude of the resulting timedomain signal is left unchanged 09LathiC09 2017925 1555 page 855 11 92 Aperiodic Signal Representation by Fourier Integral 855 0 5 10 15 20 25 30 r 01 0 01 02 Xr Figure 93 MATLABcomputed DTFS spectra for periodic sampled gate pulse of Ex 92 92 APERIODIC SIGNAL REPRESENTATION BY FOURIER INTEGRAL In Sec 91 we succeeded in representing periodic signals as a sum of everlasting exponentials In this section we extend this representation to aperiodic signals The procedure is identical conceptually to that used in Ch 7 for continuoustime signals Applying a limiting process we now show that an aperiodic signal xn can be expressed as a continuous sum integral of everlasting exponentials To represent an aperiodic signal xn such as the one illustrated in Fig 94a by everlasting exponential signals let us construct a new periodic signal xN0n formed by repeating the signal xn every N0 units as shown in Fig 94b The period N0 is made large enough to avoid overlap between the repeating cycles N0 2N 1 The periodic signal xN0n can be represented by an exponential Fourier series If we let N0 the signal Figure 94 Generation of a periodic signal by periodic extension of a signal xn 09LathiC09 2017925 1555 page 889 45 97 MATLAB Working with the DTFS and the DTFT 889 97 MATLAB WORKING WITH THE DTFS AND THE DTFT This section investigates various methods to compute the discretetime Fourier series DTFS Performance of these methods is assessed by using MATLABs stopwatch and profiling functions Additionally the discretetime Fourier transform DTFT is applied to the important topic of finite impulse response FIR filter design 971 Computing the DiscreteTime Fourier Series Within a scale factor the DTFS is identical to the DFT Thus methods to compute the DFT can be readily used to compute the DTFS Specifically the DTFS is the DFT scaled by 1N0 As an example consider a 50 Hz sinusoid sampled at 1000 Hz over onetenth of a second T 11000 N0 100 n 0N01 x cos2pi50nT The DTFS is obtained by scaling the DFT X fftxN0 f 0N01TN0 stemf12TfftshiftabsXk axis500 500 005 055 xlabelf Hz ylabelXf Figure 919 shows a peak magnitude of 05 at 50 Hz This result is consistent with Eulers representation cos2π50nT 1 2ej2π50nT 1 2ej2π50nT Lacking the 1N0 scale factor the DFT would have a peak amplitude 100 times larger The inverse DTFS is obtained by scaling the inverse DFT by N0 x realifftXN0 stemnxk axis0 99 11 11 xlabeln ylabelxn Figure 920 confirms that the sinusoid xn is properly recovered Although the result is theoretically real computer roundoff errors produce a small imaginary component which the real command removes 500 400 300 200 100 0 100 200 300 400 500 f Hz 0 02 04 Xf Figure 919 DTFS computed by scaling the DFT 09LathiC09 2017925 1555 page 891 47 97 MATLAB Working with the DTFS and the DTFT 891 Let us create an anonymous function to compute the N0byN0 DFT matrix WN0 Although not used here the signalprocessing toolbox function dftmtx computes the same DFT matrix although in a less obvious but more efficient fashion W N0 expj2piN00N010N01 While less efficient than FFTbased methods the matrix approach correctly computes the DTFS X WN0xN0 stemf12TfftshiftabsXk axis500 500 005 055 xlabelf Hz ylabelXf The resulting plot is indistinguishable from Fig 919 Problem 971 investigates a matrixbased approach to compute Eq 93 the inverse DTFS 972 Measuring Code Performance Writing efficient code is important particularly if the code is frequently used requires complicated operations involves large data sets or operates in real time MATLAB provides several tools for assessing code performance When properly used the profile function provides detailed statistics that help assess code performance MATLAB help thoroughly describes the use of the sophisticated profile command A simpler method of assessing code efficiency is to measure execution time and compare it with a reference The MATLAB command tic starts a stopwatch timer The toc command reads the timer Sandwiching instructions between tic and toc returns the elapsed time For example the execution time of the 100point matrixbased DTFS computation is tic WN0xN0 toc Elapsed time is 0004417 seconds Different machines operate at different speeds with different operating systems and with different background tasks Therefore elapsedtime measurements can vary considerably from machine to machine and from execution to execution For relatively simple and short events like the present case execution times can be so brief that MATLAB may report unreliable times or fail to register an elapsed time at all To increase the elapsed time and therefore the accuracy of the time measurement a loop is used to repeat the calculation tic for i1100 WN0xN0 end toc Elapsed time is 0173388 seconds This elapsed time suggests that each 100point DTFS calculation takes a little under 2 milliseconds What exactly does this mean however Elapsed time is only meaningful relative to some reference Let us see what difference occurs by precomputing the DFT matrix rather than repeatedly using our anonymous function W100 W100 tic for i1100 W100xN0 end toc Elapsed time is 0001199 seconds 09LathiC09 2017925 1555 page 897 53 97 MATLAB Working with the DTFS and the DTFT 897 0 5 10 15 20 Ω 25 30 35 40 n 02 0 02 04 hn 0 1 2 3 4 5 6 0 05 1 15 HΩ Samples Desired Actual Figure 924 Length41 FIR lowpass filter using linear phase 0 5 10 15 20 25 30 35 40 45 50 n Ω 02 0 02 hn 0 1 2 3 4 5 6 0 05 1 15 HΩ Samples Desired Actual Figure 925 Length50 FIR bandpass filter using linear phase 10LathiC10 2017925 1555 page 908 1 C H A P T E R STATESPACE ANALYSIS 10 In Sec 110 basic notions of state variables were introduced In this chapter we shall discuss state variables in more depth Most of this book deals with an external inputoutput description of systems As noted in Ch 1 such a description may be inadequate in some cases and we need a systematic way of finding a systems internal description Statespace analysis of systems meets this need In this method we first select a set of key variables called the state variables in the system Every possible signal or variable in the system at any instant t can be expressed in terms of the state variables and the inputs at that instant t If we know all the state variables as a function of t we can determine every possible signal or variable in the system at any instant with a relatively simple relationship The system description in this method consists of two parts 1 A set of equations relating the state variables to the inputs the state equation 2 A set of equations relating outputs to the state variables and the inputs the output equation The analysis procedure therefore consists of solving the state equation first and then solving the output equation The statespace description is capable of determining every possible system variable or output from knowledge of the input and the initial state conditions of the system For this reason it is an internal description of the system By its nature state variable analysis is eminently suited for multipleinput multipleoutput MIMO systems A singleinput single output SISO system is a special case of MIMO systems In addition the statespace techniques are useful for several other reasons mentioned in Sec 110 and repeated here 1 The state equations of a system provide a mathematical model of great generality that can describe not just linear systems but also nonlinear systems not just timeinvariant systems but also timevarying parameter systems not just SISO systems but also MIMO systems Indeed state equations are ideally suited for analysis synthesis and optimization of MIMO systems 2 Compact matrix notation along with powerful techniques of linear algebra greatly facilitates complex manipulations Without such features many important results of 908 10LathiC10 2017925 1555 page 915 8 102 Introduction to State Space 915 or q2 q1 2q2 Thus the two state equations are q1 25q1 5q2 10x q2 q1 2q2 Every possible output can now be expressed as a linear combination of q1 q2 and x From Fig 101 we have v1 x q1 i1 2x q1 v2 q1 i2 3q1 i3 i1 i2 q2 2x q1 3q1 q2 5q1 q2 2x i4 q2 v4 2i4 2q2 v3 q1 v4 q1 2q2 This set of equations is known as the output equation of the system It is clear from this set that every possible output at some instant t can be determined from knowledge of q1t q2t and xt the system state and the input at the instant t Once we have solved the state equations to obtain q1t and q2t we can determine every possible output for any given input xt For continuoustime systems the state equations are N simultaneous firstorder differential equations in N state variables q1 q2 qN of the form qi giq1q2 qNx1x2 xj i 12 N where x1 x2 xj are the j system inputs For a linear system these equations reduce to a simpler linear form qi ai1q1 ai2q2 aiNqN bi1x1 bi2x2 bijxj i 12 N 1014 If there are k outputs y1y2 yk the k output equations are of the form ym cm1q1 cm2q2 cmNqN dm1x1 dm2x2 dmjxj m 12 k 1015 The N simultaneous firstorder state equations are also known as the normalform equations 10LathiC10 2017925 1555 page 952 45 952 CHAPTER 10 STATESPACE ANALYSIS Using our previous calculations we have z1 z1 z2 z2 x and thus y z1 z2 Figure 1010b shows a realization of these equations Clearly each of the two modes is observable at the output but the mode corresponding to λ1 1 is not controllable USING MATLAB TO DETERMINE CONTROLLABILITY AND OBSERVABILITY As demonstrated in Ex 1011 we can use MATLABs eig function to determine the matrix P that will diagonalize A We can then use P to determine ˆB and ˆC from which we can determine the controllability and observability of a system Let us demonstrate the process for the two present systems First let us use MATLAB to compute ˆB and ˆC for the system in Fig 109a A 1 01 1 B 1 0 C 1 2 V Lambda eigA PinvV Bhat PB Chat CinvP Bhat 05000 11180 Chat 2 0 Since all the rows of ˆB are nonzero the system is controllable However one column of ˆC is zero so one mode is unobservable Next let us use MATLAB to compute ˆB and ˆC for the system in Fig 109b A 1 02 1 B 1 1 C 0 1 V Lambda eigA PinvV Bhat PB Chat CinvP Bhat 0 14142 Chat 10000 07071 One of the rows of ˆB is zero so one mode is uncontrollable Since all of the columns of ˆC are nonzero the system is observable As expected the MATLAB results confirm our earlier conclusions regarding the controllability and observability of the systems of Fig 109 10LathiC10 2017925 1555 page 953 46 107 StateSpace Analysis of DiscreteTime Systems 953 1061 Inadequacy of the Transfer Function Description of a System Example 1012 demonstrates the inadequacy of the transfer function to describe an LTI system in general The systems in Figs 109a and 109b both have the same transfer function Hs 1 s 1 Yet the two systems are very different Their true nature is revealed in Figs 1010a and 1010b respectively Both the systems are unstable but their transfer function Hs 1s 1 does not give any hint of it Moreover the systems are very different from the viewpoint of controllability and observability The system in Fig 109a is controllable but not observable whereas the system in Fig 109b is observable but not controllable The transfer function description of a system looks at a system only from the input and output terminals Consequently the transfer function description can specify only the part of the system that is coupled to the input and the output terminals From Figs 1010a and 1010b we see that in both cases only a part of the system that has a transfer function Hs 1s 1 is coupled to the input and the output terminals This is why both systems have the same transfer function Hs 1s 1 The state variable description Eqs 1058 and 1059 on the other hand contains all the information about these systems to describe them completely The reason is that the state variable description is an internal description not the external description obtained from the system behavior at external terminals Apparently the transfer function fails to describe these systems completely because the transfer functions of these systems have a common factor s1 in the numerator and denominator this common factor is canceled out in the systems in Fig 109 with a consequent loss of the information Such a situation occurs when a system is uncontrollable andor unobservable If a system is both controllable and observable which is the case with most of the practical systems the transfer function describes the system completely In such a case the internal and external descriptions are equivalent 107 STATESPACE ANALYSIS OF DISCRETETIME SYSTEMS We have shown that an Nthorder differential equation can be expressed in terms of N firstorder differential equations In the following analogous procedure we show that a general Nthorder difference equation can be expressed in terms of N firstorder difference equations Consider the ztransfer function Hz b0zN b1zN1 bN1z bN zN a1zN1 aN1z aN The input xn and the output yn of this system are related by the difference equation EN a1EN1 aN1E aNyn b0EN b1EN1 bN1E bNxn The DFII realization of this equation is illustrated in Fig 1011 10LathiC10 2017925 1555 page 963 56 108 MATLAB Toolboxes and StateSpace Analysis 963 Q simplifyQ Q 2z 6z2 2z 16z3 11z2 6z 1 2z9z2 7z 16z3 11z2 6z 1 The resulting expression is mathematically equivalent to the original but notationally more compact Since D 0 the output Yz is given by Yz CQz Y simplifyCQ Y 6z13z2 11z 26z3 11z2 6z 1 The corresponding timedomain expression is obtained by using the inverse ztransform command iztrans y iztransY y 312n 213n 12 Like ztrans the iztrans command assumes a causal signal so the result implies multiplication by a unit step That is the system output is yn 312n213n12un which is equivalent to Eq 1069 derived in Ex 1013 Continuoustime systems use inverse Laplace transforms rather than inverse ztransforms In such cases the ilaplace command therefore replaces the iztrans command Following a similar procedure it is a simple matter to compute the zeroinput response yzirn yzir iztranssimplifyCinveye2z1Aq0 yzir 2112n 813n The zerostate response is given by yzsr y yzir yzsr 613n 1812n 12 Typing iztranssimplifyCinvzeye2ABX produces the same result MATLAB plotting functions such as plot and stem do not directly support symbolic expressions By using the subs command however it is easy to replace a symbolic variable with a vector of desired values 0 5 10 15 20 25 n 115 12 125 13 135 yn Figure 1014 Output yn computed by using the symbolic math toolbox 10LathiC10 2017925 1555 page 964 57 964 CHAPTER 10 STATESPACE ANALYSIS n 025 stemnsubsynk xlabeln ylabelyn axis5 255 115 135 Figure 1014 shows the results which are equivalent to the results obtained in Ex 1013 Although there are plotting commands in the symbolic math toolbox such as ezplot that plot symbolic expression these plotting routines lack the flexibility needed to satisfactorily plot discretetime functions 1082 Transfer Functions from StateSpace Representations A systems transfer function provides a wealth of useful information From Eq 1073 the transfer function for the system described in Ex 1013 is H collectsimplifyCinvzeye2ABD H 30z 66z2 5z 1 It is also possible to determine the numerator and denominator transfer function coefficients from a statespace model by using the signalprocessing toolbox function ss2tf numden ss2tfABCD num 0 50000 10000 den 10000 08333 01667 The denominator of Hz provides the characteristic polynomial γ 2 5 6γ 1 6 Equivalently the characteristic polynomial is the determinant of zI A syms gamma charpoly subsdetzeye2Azgamma charpoly gamma2 5gamma6 16 Here the subs command replaces the symbolic variable z with the desired symbolic variable gamma The roots command does not accommodate symbolic expressions Thus the sym2poly command converts the symbolic expression into a polynomial coefficient vector suitable for the roots command rootssym2polycharpoly ans 05000 03333 Taking the inverse ztransform of Hz yields the impulse response hn h iztransH h 1812n 1213n 6kroneckerDeltan 0 As suggested by the characteristic roots the characteristic modes of the system are 12n and 13n Notice that the symbolic math toolbox represents δn as kroneckerDeltan 0 In general δn a is represented as kroneckerDeltana 0 This notation is frequently 10LathiC10 2017925 1555 page 969 62 109 Summary 969 doublesubsAnn3 ans 01389 05278 00880 03009 For continuoustime systems the matrix exponential eAt is commonly encountered The expm command can compute the matrix exponential symbolically Using the system from Ex 108 yields syms t A 12 2336 1 eAt simplifyexpmAt eAt exp9t3exp5t 85 2exp9texp5t 115 36exp9texp5t 15 exp9t8exp5t 35 This result is identical to the result computed in Ex 108 Similar to the discretetime case an identical result is obtained by typing syms s simplifyilaplaceinvseye2A For a specific t the matrix exponential is also easy to compute either through substitution or direct computation Consider the case t 3 doublesubseAtt3 ans 10e004 00369 00082 04424 00983 The command expmA3 produces the same result 109 SUMMARY An Nthorder system can be described in terms of N key variablesthe state variables of the system The state variables are not unique rather they can be selected in a variety of ways Every possible system output can be expressed as a linear combination of the state variables and the inputs Therefore the state variables describe the entire system not merely the relationship between certain inputs and outputs For this reason the state variable description is an internal description of the system Such a description is therefore the most general system description and it contains the information of the external descriptions such as the impulse response and the transfer function The state variable description can also be extended to timevarying parameter systems and nonlinear systems An external description of a system may not characterize the system completely The state equations of a system can be written directly from knowledge of the system structure from the system equations or from the block diagram representation of the system State equations consist of a set of N firstorder differential equations and can be solved by timedomain or frequencydomain transform methods Suitable procedures exist to transform one given set of state variables into another Because a set of state variables is not unique we can have an infinite variety of statespace descriptions of the same system The use of an appropriate transformation allows us to see clearly which of the system states are controllable and which are observable 10LathiC10 2017925 1555 page 974 67 11LathiIndex 2017925 1929 page 975 1 INDEX Abscissa of convergence 336 Accumulator systems 259 295 519 discretetime Fourier transform of 87576 Active circuits 38285 Adders 388 396 399 403 Addition of complex numbers 1112 of matrices 38 of sinusoids 1820 Additivity 9798 Algebra of complex numbers 515 matrix 3842 Aliasing 53638 78895 805 81112 817 834 defined 536 general condition for in sinusoids 79396 treachery of 78891 verification of in sinusoids 79293 Aliasing error 659 811 Amplitude 16 Amplitude modulation 71113 73649 762 Amplitude response 413 41617 421 424 435 43738 44042 Amplitude spectrum 598 607 61525 667 668 707 848 870 878 Analog filters 261 Analog signals 133 defined 78 digital processing of 54754 properties of 78 Analog systems 109 135 261 Analogtodigital AD conversion 799802 831 Analogtodigital converters ADC bit number 8012 bit rate 8012 Angle modulation 736 763 Angles electronic calculators in computing 811 principal value of 9 Angular acceleration 116 Angular position 116 Angular velocity 116 Antialiasing filters 537 791 834 Anticausal exponentials 86263 Anticausal signals 81 Aperiodic signals 133 discretetime Fourier integral and 85567 Fourier integral and 68089 762 properties of 7882 Apparent frequency 53436 79296 Ars Magna Cardano 25 Associative property 171 283 Asymptotic stability See Internal stability Audio signals 71314 725 746 Automatic position control system 4068 Auxiliary conditions 153 differential equation solution and 161 Backward difference system 258 295 519 56869 Bandlimited signals 533 788 792 802 Bandpass filters 44143 54244 749 88283 896 group delay and 72627 ideal 73031 88283 poles and zeros of Hs and 443 Bandstop filters 44142 445 54546 Bandwidth 628 continuoustime systems and 20810 data truncation and 75153 essential 736 75859 Fourier transform and 692 706 76263 Bartlett window 75355 Baseband signals 73740 74647 749 Basis signals 651 655 668 Basis vectors 648 Beat effect 741 Bhaskar 2 Bilateral Laplace transform 330 33537 44555 467 properties of 45155 Bilateral ztransform 431 490 55463 in discretetime system analysis 563 properties of 55960 Bilinear transformation 56970 Binary digital signals 799 Black box 95 119 120 975 11LathiIndex 2017925 1929 page 976 2 976 Index Blackman window 755 761 Block diagrams 38688 405 407 408 519 Bôcher M 62021 Bode plots 41935 constant of 421 firstorder pole and 42427 pole at the origin and 42223 secondorder pole and 42635 Bombelli Raphael 3 Bonaparte Napoleon 347 61011 Boundedinputboundedoutput BIBO stability 110 135 263 of continuoustime systems 19697 199203 22223 of discretetime systems 29899 3014 314 526 527 frequency response and 41213 internal stability relationship to 199203 3014 of the Laplace transform 37173 signal transmission and 721 steadystate response and 418 of the ztransform 518 Butterfly signal flow graph 825 Butterworth filters 440 55154 cascaded secondorder sections for Butterworth filter realization 46163 MATLAB on 45963 transformation of 57172 Canonic direct realization See Direct form II realization Cardano Gerolamo 25 Cartesian form 815 Cascade realization 394 526 920 923 Cascade systems 190 192 37273 Cascaded RC filters 46162 Causal exponentials 86162 Causal signals 81 83 134 Causal sinusoidal input in continuoustime systems 41819 in discretetime systems 527 Causal systems 1046 135 263 properties of 1046 zerostate response and 172 283 CayleyHamilton theorem 91012 Characteristic equations of continuoustime systems 15355 of discretetime systems 271 273 309 of a matrix 91012 933 Characteristic functions 192 Characteristic modes of continuoustime systems 15355 16265 167 170 196 19899 2036 of discretetime systems 27174 27879 297301 305 313 Characteristic polynomials of continuoustime systems 15356 164 166 2023 220 of discretetime systems 271 27475 279 3034 of the Laplace transform 37172 of the ztransform 518 Characteristic roots of continuoustime systems 15356 162 166 198203 206 209 21112 21417 22224 of discretetime systems 271 27375 297 299301 3035 309 314 invariance of 94243 of a matrix 911 93233 Characteristic values See Characteristic roots Characteristic vectors 910 Chebyshev filters 440 46366 Circular convolution 81920 821 Clearing fractions 2627 3233 34243 Closed Loop systems See Feedback systems Coherent demodulation See Synchronous demodulation Coefficients of Fourier series computation 59598 Column vectors 36 Commutative property of the convolution integral 170 173 181 19192 of the convolution sum 283 Compact disc CD 801 Compact form of Fourier series 59798 599 600 6047 Complex factors of Qx 29 Complex frequency 8991 Complex inputs 177 297 Complex numbers 115 54 algebra of 515 arithmetical operations for 1215 conjugates of 67 historical note 15 logarithms of 15 origins of 25 standard forms of 1415 useful identities 78 working with 1314 Complex poles 395 432 497 542 Complex roots 15456 27476 Complex signals 9495 Conjugate symmetry of the discrete Fourier transform 81819 of the discretetime Fourier transform 85859 86768 of the Fourier transform 684 703 Conjugation 684 703 Constants 54 98 100 103 130 422 Constantparameter systems See Timeinvariant systems Continuous functions 858 Continuoustime filters 45563 Continuoustime Fourier transform CTFT 867 88485 Continuoustime signals 1078 135 defined 78 discretetime systems and 238 Fourier series and 593679 Fourier transform and 678769 680775 Continuoustime systems 135 150236 analog systems compared with 261 differential equations of 161 196 213 11LathiIndex 2017925 1929 page 977 3 Index 977 discretetime systems compared with 261 external input response to 16896 frequency response of 41218 73233 internal conditions response to 15163 intuitive insights into 18990 2035 Laplace transform 330487 Periodic inputs and 637641 properties of 1078 signal transmission through 72129 stability of 196203 22223 state equations for 91516 Control systems 40412 analysis of 40612 design specifications 411 step input and 4079 Controllabilityobservability 12324 of continuoustime systems 197 2002 223 of discretetime systems 303 96568 in statespace analysis 94753 961 Convergence abscissa of 336 of Fourier series 61314 to the mean 613 614 region of See region of convergence Convolution 5079 with an impulse 283 of the bilateral ztransform 560 circular 81920 821 discretetime 31112 fast 821 886 frequency See Frequency convolution of the Fourier transform 71416 linear 821 periodic 886 time See Time convolution Convolution integral 17093 222 282 288 313 722 explanation for use 18990 graphical understanding of 17890 21720 properties of 17072 Convolution sum 28286 313 graphical procedure for 28893 properties of 28283 from a table 28586 Convolution table 17576 Cooley J W 824 Corner frequency 424 Cramers rule 2325 40 51 379 385 Critically damped systems 409 410 Cubic equations 23 58 Custom filter function 31011 Cutoff frequency 208 209 Damping coefficient 11518 Dashpots linear 115 torsional 116 Data truncations 74955 763 Decades 422 Decibels 421 Decimationinfrequency algorithm 824 827 Decimationintime algorithm 82527 Decomposition 99100 151 Delayed impulse 168 Demodulation 714 of amplitude modulation 74446 of DSBSC signals 73941 synchronous 74344 Depressed cubic equation 58 Derivative formulas 56 Descartes René 2 Detection See Demodulation Deterministic signals 82 134 Diagonal matrices 37 Difference equations 25960 26570 causality condition in 26566 classical solution of 298 differential equation kinship with 260 frequency response 532 order of 260 recursive and nonrecursive forms of 259 recursive solution of 26670 sinusoidal response of difference equation systems 528 ztransform solution of 488 51019 574 Differential equations 161 classical solution of 196 difference equation kinship with 260 Laplace transform solution of 34648 36073 Differentiators digital 25658 ideal 36971 373 41617 Digital differentiator example 25859 Digital filters 108 238 26162 Digital integrators 25859 Digital processing of analog signals 54753 Digital signals 135 79799 advantages of 26162 binary 799801 defined 78 Lary 799 properties of 78 See also Analogtodigital conversion Digital systems 109 135 261 Dirac definition of an impulse 88 134 Dirac delta train 69697 Dirac PAM 86 Direct discrete Fourier transform DFT 808 857 Direct form I DFI realization Laplace transform and 39091 394 ztransform and 521 See also Transposed direct form II realization 11LathiIndex 2017925 1929 page 978 4 978 Index Direct form II DFII realization 92025 954 965 967 Laplace transform and 391 398 ztransform and 52022 525 Direct Fourier transform 683 7023 762 Direct ztransform 488592 Dirichlet conditions 612 614 686 Discrete Fourier transform DFT 659 80523 82734 835 aliasing and leakage and 8056 applications of 82023 computing Fourier transform 81218 derivation of 80710 determining filter output 82223 direct 808 857 discretetime Fourier transform and 88586 898 inverse 808 835 857 MATLAB on 82734 picket fence effect and 807 points of discontinuity 807 properties of 81820 zero padding and 81011 82930 Discretetime complex exponentials 252 Discretetime convolution 31112 Discretetime exponentials 24749 Discretetime Fourier integral 85567 Discretetime Fourier series DTFS 84555 computation of 88586 MATLAB on 88997 of periodic gate function 85355 periodic signals and 84647 898 of sinusoids 84952 Discretetime Fourier transform DTFT 85788 of accumulator systems 87576 of anticausal exponentials 86263 of causal exponentials 86162 continuoustime Fourier transform and 88386 existence of 859 886 inverse 886 linear timeinvariant discretetime system analysis by 87980 MATLAB on 88997 physical appreciation of 859 properties of 86778 of rectangular pulses 86365 table of 860 ztransform connection with 86667 88688 898 Discretetime signals 78 79 1078 133 23753 defined 78 Fourier analysis of 845907 inherently bandlimited 533 size of 23840 useful models 24553 useful operations 24045 Discretetime systems 135 237329 classification of 26264 controllabilityobservability of 303 96568 difference equations of 25960 26570 298 discretetime Fourier transform analysis of 87883 examples of 25365 external input response to 28098 frequency response of 52638 internal conditions response to 27076 intuitive insights into 3056 properties of 1078 26465 stability of 263 298305 314 statespace analysis of 95364 ztransform analysis of 488592 Distinct factors of Qx 27 Distortionless transmission 72428 730 763 88082 bandpass systems and 72627 88182 measure of delay variation 881 Distributive property 171 283 Division of complex numbers 1214 Doublesideband suppressedcarrier DSBSC modulation 73741 742 74649 Downsampling 24344 Duality 7034 Dynamic systems 1034 13435 263 Eigenfunctions 193 Eigenvalues See Characteristic roots Eigenvectors 910 Einstein Albert 348 Electrical systems 9596 11114 Laplace transform analysis of 37385 467 state equations for 91619 Electromechanical systems 11819 Electronic calculators 811 Energy signals 67 82 134 23940 Energy spectral density 734 763 Envelope delay See Group delay Envelope detector 74345 Equilibrium states 196 198 Error signals 65051 Error vectors 642 Essential bandwidth 736 75859 Euler Leonhard 2 3 Eulers formula 56 45 252 Even component of a signal 9395 Even functions 9293 134 Everlasting exponentials continuoustime systems and 189 19395 222 discretetime systems and 29697 313 Fourier series and 637 638 641 Fourier transform and 687 Laplace transform and 36768 412 419 Everlasting signals 81 134 Exponential Fourier series 62137 661 803 periodic inputs and 63741 reasons for using 640 symmetry effect on 63032 11LathiIndex 2017925 1929 page 979 5 Index 979 Exponential Fourier spectra 62432 664 667 668 Exponential functions 8991 134 Exponential input 193 296 Exponentials computation of matrix 922913 discretetime 24749 discretetime complex 252 everlasting See Everlasting exponentials matrix 96869 monotonic 2022 90 91 134 sinusoid varying 2223 90 134 sinusoids expressed in 20 Exposition du système du monde Laplace 346 External description of a system 11920 135 External input continuoustime system response to 16896 discretetime system response to 28098 External stability See Boundedinputboundedoutput stability Fast convolution 821 886 Fast Fourier transform FFT 659 811 821 82427 835 computations reduced by 824 discretetime Fourier series and 847 discretetime Fourier transform and 88586 898 Feedback systems Laplace transform and 38688 39295 399 40412 ztransform and 521 Feedforward connections 39294 403 Filtering discrete Fourier transform and 82123 MATLAB on 30810 selective 74849 time constant and 2078 Filters analog 261 antialiasing 537 791 834 bandpass 44143 bandstop 44142 445 54546 Butterworth See Butterworth Filters cascaded RC 46162 Chebyshev 440 46366 continuoustime 45563 custom function 31011 digital 108 238 26162 finite impulse response 524 89297 firstorder hold 785 frequency response of 41218 highpass 443 445 542 73031 88283 Ideal See Ideal filters impulse invariance criterion of 548 infinite impulse response 524 56574 lowpass 43941 lowpass See Lowpass filters notch 44143 540 54546 poles and zeros of Hs and 43645 practical 44445 88283 sharp cutoff 748 windows in design of 755 zeroorder hold 785 Final value theorem 35961 508 Finite impulse response FIR filters 524 89297 Finiteduration signals 333 Finitememory systems 104 Firstorder factors method of 497 Firstorder hold filters 785 Folding frequency 78991 793 795 817 Forloops 21618 Forced response difference equations and 298 differential equations and 198 Forward amplifiers 4056 Fourier integral 722 aperiodic signal and 68089 762 discretetime 85567 Fourier series 593679 compact form of 59798 599 600 6047 computing the coefficients of 59598 discrete time See Discretetime Fourier series existence of 61213 exponential See Exponential Fourier series generalized 64159 668 Legendre 65657 limitations of analysis method 641 trigonometric See Trigonometric Fourier series waveshaping in 61517 Fourier spectrum 598607 777 exponential 62432 664 667 668 nature of 85859 of a periodic signal 84855 Fourier transform 680755 778 8023 continuoustime 867 88386 discrete See Discrete Fourier transform discretetime See Discretetime Fourier transform direct 683 7023 762 existence of 68586 fast See fast Fourier transform interpolation and 785 inverse 683 69395 699 762 78687 physical appreciation of 68789 properties of 70121 useful functions of 689701 Fourier transform pairs 683 700 Fourier Baron JeanBaptisteJoseph 61012 Fractions 12 clearing 2627 3234 34243 partial See Partial fractions Frequency apparent 53436 79394 complex 8991 11LathiIndex 2017925 1929 page 980 6 980 Index Frequency continued corner 424 cutoff 208 209 folding 78991 793 795 817 fundamental 594 60910 846 negative 62628 neper 91 radian 16 91 594 reduction in range 535 of sinusoids 16 time delay variation with 72425 Frequency convolution of the bilateral Laplace transform 452 of the discretetime Fourier transform 87576 of the Fourier transform 71416 of the Laplace transform 357 Frequency differentiation 869 Frequency domain analysis 368 72223 848 of electrical networks 37478 of the Fourier series 598 601 twodimensional view and 73233 See also Laplace transform Frequency inversion 706 Frequency resolution 807 81012 815 817 Frequency response 724 Bode plots and 41922 of continuoustime systems 41218 73233 of discretetime systems 52638 MATLAB on 45657 53132 periodic nature of 53236 from polezero location 53847 polezero plots and 56668 poles and zeros of Hs and 43639 transfer function from 435 Frequency reversal 86869 Frequency shifting of the bilateral Laplace transform 451 of the discrete Fourier transform 819 of the discretetime Fourier transform 87174 of the Fourier transform 71113 of the Laplace transform 35354 Frequency spectra 598 601 Frequencydivision multiplexing FDM 714 74950 Function Mfiles 21415 Functions characteristic 193 continuous 858 even 9293 134 exponential 8991 134 improper 2526 34 interpolation 690 MATLAB on 12633 odd 9295 134 proper 2527 rational 2529 338 singularity 89 Fundamental band 533 534 537 793 Fundamental frequency 594 60910 846 Fundamental period 79 133 23940 593 595 846 Gain enhancement by poles 43738 Gauss Karl Friedrich 34 Generalized Fourier series 64159 668 Generalized linear phase GLP 72627 Gibbs phenomenon 61921 66163 Gibbs Josiah Willard 62021 Graphical interpretation of convolution integral 17890 21720 of convolution sum 28893 Greatest common factor of frequencies 60910 Group delay 72528 881 Hs filter design and 43645 realization of 54849 See also Transfer functions Halfwave symmetry 608 Hamming window 75455 761 Hanning window 75455 761 Hardware realization 64 95 133 Harmonic distortion 634 Harmonically related frequencies 609 Heaviside coverup method 2730 3335 341 34243 497 Heaviside Oliver 34748 612 Highpass filters 443 445 542 745 747 88283 Homogeneity 9798 Ideal delay 369 416 Ideal differentiators 36971 373 41617 Ideal filters 73033 763 785 791 834 88283 Ideal integrators 369 370 373 400 41618 Ideal interpolation 78687 Ideal linear phase ILP 725 727 Ideal masses 114 Identity matrices 37 Identity systems 109 192 263 Imaginary numbers 15 Impedance 37477 379 380 382 384 387 399 Improper functions 2526 34 Impulse invariance criterion of filter design 548 Impulse matching 16466 Impulse response matrix 938 Indefinite integrals 57 Indicator function See Relational operators Inertia moment of 11618 Infinite impulse response IIR filters 524 56574 Information transmission rate 20910 11LathiIndex 2017925 1929 page 981 7 Index 981 Initial conditions 97100 102 122 134 335 at 0 and 0 36364 continuoustime systems and 15861 generators of 37683 Initial value theorem 35961 508 Input 64 complex 177 297 exponential 193 296 external See External input in linear systems 97 multiple 178 28788 ramp 41011 sinusoidal See Sinusoidal input step 40710 Inputoutput description 11119 Instantaneous systems 1034 134 263 Integrals convolution See Convolutional integral discretetime Fourier 85567 Fourier See Fourier integral indefinite 57 of matrices 90910 Integrators digital 25859 ideal 369 370 373 400 41618 system realization and 400 Integrodifferential equations 36073 466 488 Interconnected systems continuoustime 19093 discretetime 29497 Internal conditions continuoustime system response to 15163 discretetime system response to 27076 Internal description of a system 11921 135 908 See also Statespace description of a system Internal stability 110 135 263 BIBO relationship to 199203 3014 of continuoustime systems 196203 22223 of discretetime systems 298302 305 314 526 527 of the Laplace transform 372 of the ztransform 518 Interpolation 78588 of discretetime signals 24344 ideal 78687 simple 78586 spectral 804 Interpolation formula 779 787 Interpolation function 690 Intuitive insights into continuoustime systems 18990 20312 into discretetime systems 3056 into the Laplace transform 36768 Inverse continuoustime systems 19293 Inverse discrete Fourier transform IDFT 808 827 857 Inverse discretetime Fourier transform IDTFT 886 of rectangular spectrum 86566 Inverse discretetime systems 29495 Inverse Fourier transform 683 69395 699 762 78687 Inverse Laplace transform 333 335 445 549 finding 33846 Inverse ztransform 48889 491 499 500 501 510 554 555 559 finding 495 Inversion frequency 706 matrix 4042 Invertible systems 10910 135 263 Irrational numbers 12 Kaiser window 755 76062 Kelvin Lord 348 KennellyHeaviside atmosphere layer 348 Kirchhoffs laws 95 current KCL 111 213 374 voltage KVL 111 374 Kronecker delta functions 245 bandlimited interpolation of 78788 Lary digital signals 799 LHôpitals rule 58 211 690 Lagrange Louis de 347 612 613 Laplace transform 167 330487 721 bilateral See Bilateral Laplace transform differential equation solutions and 34648 36073 electrical network analysis and 37385 467 existence of 33637 Fourier transform connection with 699701 866 intuitive interpretation of 36769 inverse 549 93839 properties of 34962 stability of 37174 state equation solutions by 92733 system realization and 388404 unilateral 33336 337 338 345 360 445 467 ztransform connection with 488 489 491 56365 Laplace transform pairs 333 Laplace Marquis PierreSimon de 34647 611 612 613 Leakage 751 75355 763 8056 Left half plane LHP 91 19899 202 211 223 435 Left shift 71 73 130 134 503 509 510 512 Leftsided sequences 55556 Legendre Fourier series 65657 Leibniz Gottfried Wilhelm 801 Linear convolution 821 Linear dashpots 115 Linear phase distortionless transmission and 725 881 generalized 72627 ideal 725 727 11LathiIndex 2017925 1929 page 982 8 982 Index Linear phase continued physical description of 7079 physical explanation of 87071 Linear springs 114 Linear systems 97101 134 heuristic understanding of 72223 response of 98100 Linear timeinvariant continuoustime LTIC systems See Continuoustime systems Linear timeinvariant discretetime LTID systems See Discretetime systems Linear timeinvariant LTI systems 103 19495 Linear timeinvariant discretetime LTID systems 87980 Linear timevarying systems 103 Linear transformation of vectors 36 93947 961 Linearity of the bilateral Laplace transform 451 of the bilateral ztransform 559 concept of 9798 of the discrete Fourier transform 818 824 of the discretetime Fourier transform 867 of discretetime systems 262 of the Fourier transform 68687 824 of the Laplace transform 33132 of the ztransform 489 Log magnitude 27 42224 Loop currents continuoustime systems and 15963 175 Laplace transform and 375 Lower sideband LSB 73839 747 Lowpass filters 43941 54042 ideal 730 78485 78889 88283 poles and zeros of Hs and 43645 Mfiles 21220 function 21415 script 21314 218 Maclaurin series 6 55 Magnitude response See Amplitude response Marginally stable systems continuoustime 198200 203 211 22224 discretetime 3012 304 314 Laplace transform 373 signal transmission and 721 ztransform 519 Mathematical models of systems 9596 125 MATLAB on Butterworth filters 45963 calculator operations in 4345 on continuoustime filters 45563 on discrete Fourier transform 82734 on discretetime Fourier series and transform 88997 on discretetime systemssignals 30612 elementary operations in 4253 on filtering 30810 Fourier series applications in 66167 Fourier transform topics in 75562 frequency response plots 53132 on functions 12633 impulse invariance 553 impulse response and 167 on infiniteimpulse response filters 56574 Mfiles in 21220 matrix operations in 4953 multiple magnitude response curves 544 partial fraction expansion in 53 periodic functions 66163 phase spectrum 66467 polynomial roots and 157 simple plotting in 4648 statespace analysis in 96169 vector operations in 4546 zeroinput response and 15758 Matrices 3642 algebra of 3842 characteristic equation of 90910 933 characteristic roots of 93233 computing exponential of 91213 definitions and properties of 3738 derivatives of 90910 diagonal 37 diagonalization of 94344 equal 37 functions of 91112 identity 37 impulse response 938 integrals of 90910 inversion of 4042 MATLAB operations 4953 nonsingular 41 square 36 37 41 state transition 936 symmetric 37 transpose of 3738 zero 37 Matrix exponentials 96869 Matrix exponentiation 96869 Mechanical systems 11418 Memory systems and 104 263 Memoryless systems See Instantaneous systems Method of residues 27 Michelson Albert 62021 Minimum phase systems 435 436 Modified partial fractions 35 496 Modulation 71314 73649 amplitude 71113 736 74246 762 angle 736 763 of the discretetime Fourier transform 872 11LathiIndex 2017925 1929 page 983 9 Index 983 doublesideband suppressedcarrier 73741 742 74649 pulseamplitude 796 pulsecode 796 799 pulseposition 796 pulsewidth 796 singlesideband 74649 Moment of inertia 11618 Monotonic exponentials 2022 90 91 134 Multiple inputs 178 28788 Multipleinput multipleoutput MIMO systems 98 125 908 Multiplication bilateral ztransform and 560 of complex numbers 1214 discretetime Fourier transform and 869 of a function by an impulse 87 matrix 3840 scalar 38 4001 505 ztransform and 5067 Natural binary code NBC 799 Natural modes See Characteristic modes Natural numbers 1 Natural response difference equations and 298 differential equations and 196 Negative feedback 406 Negative frequency 62628 Negative numbers 13 45 Neper frequency 91 Neutral equilibrium 197 198 Newton Sir Isaac 2 34647 Noise 66 151 371 417 791 79799 Nonanticipative systems See Causal systems Nonbandlimited signals 792 Noncausal signals 81 Noncausal systems 1047 135 263 properties of 1046 reasons for studying 1067 Noninvertible systems 10910 135 263 Noninverting amplifiers 382 Nonlinear systems 97101 134 Nonsingular matrices 41 Nonuniqueness 533 Normalform equations 915 Norton theorem 375 Notch filters 44143 540 54546 See also Bandstop filters Numerical integration 13133 Nyquist interval 778 779 Nyquist rate 77881 78889 792 795 821 Nyquist samples 778 781 782 788 792 Observability See controllabilityobservability Octave 422 Odd component of a signals 9395 Odd functions 9295 134 Operational amplifiers 38283 399 467 Ordinary numbers 15 Orthogonal signal space 64950 Orthogonal signals 668 energy of the sum of 647 signal representation by set 64759 Orthogonal vector space 64748 Orthogonality 622 Orthonormal sets 649 Oscillators 203 Output 64 97 Output equations 122 124 908 930 941 Overdamped systems 40910 PaleyWiener criterion 444 73132 788 Parallel realization 39394 52526 921 92425 Parallel systems 190 387 Parsevals theorem 632 65152 73435 755 75859 87678 Partial fractions expansion of 2535 53 inverse transform by partial fraction expansion and tables 49598 Laplace transform and 33839 341 344 362 394 395 419 454 modified 35 ztransform 499 Passbands 441 44445 748 755 Peak time 40910 Percent overshoot PO 40910 Periodic circular convolution 81920 of the discretetime Fourier transform 875 Periodic extension of the Fourier spectrum 84855 properties of 8081 Periodic functions Fourier spectra as 858 MATLAB on 66163 Periodic gate function 85355 Periodic signals 133 63740 discretetime Fourier series and 84647 Fourier spectra of 84855 Fourier transform of 69596 properties of 7882 and trigonometric Fourier series 593612 661 Periods fundamental 79 133 23940 593 595 846 sinusoid 16 Phase response 41325 42735 439 467 Phase spectrum 598 607 61718 707 848 MATLAB on 66467 using principal values 70910 11LathiIndex 2017925 1929 page 984 10 984 Index Phaseplane analysis 125 909 Phasors 1820 Physical systems See Causal systems Picket fence effect 807 Pickoff nodes 190 25455 396 Pingala 801 Pointwise convergent series 613 Polar coordinates 56 Polar form 815 arithmetical operations in 1215 sinusoids and 18 Polezero location 53847 Polezero plots 56668 Poles complex 395 432 497 542 controlling gain by 540 firstorder 42427 gain enhancement by 43738 Hs filter design and 43645 at the origin 42223 repeated 395 525 926 in the right half plane 371 43536 secondorder 42635 wall of 43941 542 Polynomial expansion 45859 Polynomial roots 157 572 Positive feedback 406 Power series 55 Power signals 67 82 134 23940 See also Signal power Power determining 6869 matrix 91213 Powers of complex numbers 1316 Practical filters 73033 88283 Preece Sir William 349 Prewarping 57071 Principal values of the angle 9 phase spectrum using 70910 Proper functions 2527 Pulseamplitude modulation PAM 796 Pulsecode modulation PCM 796 799 Pulse dispersion 209 Pulseposition modulation PPM 796 Pulsewidth modulation PWM 796 Pupin M 348 Pythagoras 2 Quadratic equations 58 Quadratic factors 2930 for the Laplace transform 34142 for the ztransform 497 Quantization 799 83134 Quantized levels 799 Radian frequency 16 91 594 Random signals 82 134 Rational functions 2529 338 Real numbers 27 43 Real time 1056 Rectangular pulses 86365 Rectangular spectrum 86566 Rectangular windows 751 75355 763 Reflection property 86869 Region of convergence ROC for continuoustime systems 193 for finiteduration signals 333 for the Laplace transform 33133 337 347 448 449 45455 467 for the ztransform 48991 55558 561 Relational operators 12829 Repeated factors of Qx 3132 Repeated poles 395 525 926 Repeated roots of continuoustime systems 15456 195 198 202 223 of discretetime systems 270 27374 297 301 31314 Resonance phenomenon 163 204 205 21012 305 Right half plane RHP 91 198 2003 223 371 43536 Right shift 7172 131 134 5014 509 510 Rightsided sequences 55556 Rise time 2067 405 40910 411 RLC networks 914 91618 RMS value 6869 70 Rolloff rate 753 754 Roots complex 15456 27476 of complex numbers 1115 polynomial 157 572 repeated See Repeated roots unrepeated 198 202 223 301 314 Rotational systems 11619 Rotational mass See Moment of inertia Row vectors 36 45 4850 Sales estimate example 25556 SallenKey circuit 383 384 46162 463 466 Sampled continuoustime sinusoids 52731 Sampling 776844 practical 78184 properties of 8788 134 signal reconstruction and 78599 spectral 75960 8024 See also Discrete Fourier transform FastFourier transform Sampling interval 55054 Sampling rate 24344 53637 Sampling theorem 537 77684 83435 applications of 79699 spectral 802 Savings account example 25355 11LathiIndex 2017925 1929 page 985 11 Index 985 Scalar multiplication 38 4001 505 509 520 Scaling 9798 130 of the Fourier transform 7056 755 757 762 of the Laplace transform 357 See also Time scaling Script Mfiles 21314 216 218 Selectivefiltering method 74849 Sharp cutoff filters 748 Shifting of the bilateral ztransform 559 of the convolution integral 17172 of the convolution sum 283 of discretetime signals 240 See also Frequency shifting Time shifting Sideband 74649 sifting See Sampling Signal distortion 72325 Signal energy 6566 70 13133 73336 757 87778 See also Energy signals Signal power 6567 133 See also Power signals Signal reconstruction 78599 See also Interpolation Signaltonoise power ratio 66 Signal transmission 72129 Signals 6491 13334 analog See Analog signals anticausal 81 aperiodic See Aperiodic signals audio 71314 725 746 bandlimited 533 788 792 802 baseband 73740 74647 749 basis 651 655 668 causal 81 83 134 classification of 7882 13334 comparison and components of 64345 complex 9495 continuous time See continuoustime signals defined 65 deterministic 83 134 digital See Digital signals discrete time See Discretetime signals energy 82 134 23940 error 65051 even components of 9395 everlasting 81 134 finiteduration 333 modulating 711 73739 nonbandlimited 792 noncausal 81 odd components of 9395 orthogonal See Orthogonal signals periodic See Periodic signals phantoms of 189 power 82 134 23940 random 82 134 size of 6470 133 sketching 2023 time reversal of 77 time limited 802 805 807 twodimensional view of 73233 useful models 8291 useful operations 7178 as vectors 64159 video 725 749 Sinc function 757 Singleinput singleoutput SISO systems 98 125 908 Singlesideband SSB modulation 74649 Singularity functions 89 Sinusoidal input causal See Causal sinusoidal input continuoustime systems and 208 discretetime systems and 309 frequency response and 41317 steadystate response to causal sinusoidal input 41819 Sinusoids 1620 8991 134 addition of 1820 apparent frequency of sampled 79596 compression and expansion 76 continuoustime 25152 53337 discretetime 251 527 528 53337 discretetime Fourier series of 84952 in exponential terms 20 exponentially varying 2223 80 134 general condition for aliasing in 79396 power of a sum of two equalfrequency 70 sampled continuoustime 52731 verification of aliasing in 79293 Sketching signals 2023 Slidingtape method 29093 Software realization 64 95 133 Spectral density 688 Spectral folding See Aliasing Spectral interpolation 804 Spectral resolution 807 Spectral sampling 75960 802 Spectral sampling theorem 802 Spectral spreading 75153 755 763 807 Springs linear 114 torsional 11617 Square matrices 36 37 41 Square roots of negative numbers 24 Stability BIBO See Boundedinputboundedoutput stability of continuoustime systems 196203 22223 of discretetime systems 263 298305 314 of the Laplace transform 37174 Internal See Internal stability of the ztransform 51819 marginal See marginally stable systems 11LathiIndex 2017925 1929 page 986 12 986 Index Stable equilibrium 19697 Stable systems 110 263 State equations 12225 135 9089 969 alternative procedure to determine 91819 diagonal form of 94447 solution of 92639 for the state vector 94142 systematic procedure for determining 91326 timedomain method to solve 93637 State transition matrix STM 936 State variables 12125 135 908 969 State vectors 92730 961 linear transformation of 94142 Statespace analysis 90873 controllabilityobservability in 94753 961 of discretetime systems 95364 in MATLAB 96169 transfer function and 92024 transfer function matrix 93132 Statespace description of a system 12125 Steadystate error 40911 Steadystate response in continuoustime systems 41819 in discretetime systems 527 Stem plots 3068 Step input 40710 Stiffness of linear springs 114 of torsional springs 11617 Stopbands 441 444 445 456 457 459 460 463 755 Subcarriers 749 Subtraction of complex numbers 1112 Superposition 98 99 100 123 134 continuoustime systems and 168 170 178 discretetime systems and 287 Symmetric matrices 37 Symmetry conjugate See Conjugate symmetry exponential Fourier series and 63032 trigonometric Fourier series and 6078 Synchronous demodulation 74344 747 System realization 388404 51925 567 cascade 394 52526 91920 923 of complex conjugate poles 395 direct See Direct form I realization Direct form II realization differences in performance 52526 hardware 64 95 133 parallel See Parallel realization software 64 95 129 Systems 95133 13435 accumulator 259 295 519 analog 109 135 261 backward difference 258 295 519 56869 BIBO stability assessing 110 cascade 190 192 372 373 causal See causal systems causality assessing 105 classification of 97110 13435 continuous time See Continuoustime systems control See control systems critically damped 409 410 data for computing response 9697 defined 64 digital 78 135 261 discrete time See discrete time systems dynamic 1034 13435 263 electrical 9596 11114 electrical See Electrical systems electromechanical 11819 feedback See feedback systems finitememory 104 identity 109 192 263 inputoutput description 11119 instantaneous 1034 263 interconnected See interconnected systems invertible 10910 135 263 linear See Linear systems mathematical models of 9596 125 mechanical 11418 memory and 104 263 minimum phase 435 436 multipleinput multipleoutput 98 125 908 noncausal 1047 263 noninvertible 10910 135 nonlinear 97101 134 overdamped 40910 parallel 190 387 phantoms of 189 properties of 26465 rotational 11619 singleinput singleoutput 98 125 908 stable 110 263 translational 11416 time invariant See Timeinvariant systems time varying See Timevarying systems twodimensional view of 73233 underdamped 409 unstable 110 263 Tacoma Narrows Bridge failure 212 Tapered windows 75354 763 807 Taylor series 55 Théorie analytique de la chaleur Fourier 612 Thévenins theorem 375 378 379 Time constant of continuoustime systems 20510 223 of the exponential 2122 filtering and 2079 information transmission rate and 20910 11LathiIndex 2017925 1929 page 987 13 Index 987 pulse dispersion and 209 rise time and 2067 Time convolution of the bilateral Laplace transform 452 of the discretetime Fourier transform 87576 of the Fourier transform 71416 of the Laplace transform 357 of the ztransform 5078 Time delay variation with frequency 72425 Time differentiation of the bilateral Laplace transform 451 of the Fourier transform 71618 of the Laplace transform 35456 Time integration of the bilateral Laplace transform 451 of the Fourier transform 71618 of the Laplace transform 35657 Time inversion 706 Time reversal 134 of the bilateral Laplace transform 452 of the bilateral ztransform 560 of the convolution integral 178 181 described 7677 of the discretetime Fourier transform 86869 of discretetime signals 242 of the ztransform 5067 Time scaling 77 of the bilateral Laplace transform 452 described 7374 Time shifting 77 79 of the bilateral Laplace transform 451 of the convolution integral 178 described 7173 of the discrete Fourier transform 819 of the discretetime Fourier transform 870 of the Fourier transform 707 of the Laplace transform 34951 of the ztransform 5015 510 Timedivision multiplexing TDM 749 797 Timedomain analysis 723 of continuoustime systems 150236 of discretetime systems 237329 of the Fourier series 598 601 of interpolation 78588 state equation solution in 93339 twodimensional view and 73233 Timefrequency duality 7023 723 753 Time invariant systems 134 discretetime 262 linear See Linear timeinvariant systems properties of 1023 Timevarying systems 134 discretetime 262 linear 103 properties of 1023 Timelimited signals 802 805 807 Torque 11618 Torsional dashpots 116 Torsional springs 116 117 Total response of continuoustime systems 19596 of discretetime systems 29798 Traité de mécanique céleste Laplace 346 Transfer functions 522 analog filter realization with 54849 block diagrams and 38688 of continuoustime systems 19394 222 of discretetime systems 29697 314 51415 56768 from the frequency response 435 inadequacy for system description 953 realization of 38999 401 52425 state equations from 916 91926 from statespace representations 96465 Translational systems 11416 Transpose of a matrix 3738 Transposed direct form II TDFII realization 398 96769 state equations and 92024 ztransform and 52022 52526 Triangular windows 751 Trigonometric Fourier series 640 652 65758 667 668 exponential 62137 661 periodic signals and 593612 661 sampling and 777 782 symmetry effect on 6078 Trigonometric identities 5556 Tukey J W 824 Underdamped systems 409 Uniformly convergent series 613 Unilateral Laplace transform 33336 337 338 345 360 445 467 Unilateral ztransform 489 491 492 495 55455 559 Uniqueness 335 Unit delay 517 520 521 Unitgate function 689 Unitimpulse function 133 of discretetime systems 24647 280 313 as a generalized function 8889 properties of 8689 Unitimpulse response of continuoustime systems 16368 170 18993 22021 222 731 convolution with 171 determining 221 of discretetime systems 27780 286 295 313 Unit matrices 37 Unitstep function 8486 8889 of discretetime systems 24647 relational operators and 12830 11LathiIndex 2017925 1929 page 988 14 988 Index Unittriangle function 68990 Unrepeated roots 198 202 223 301 314 Unstable equilibrium 19697 Unstable systems 110 263 Upper sideband USB 73739 74648 Upsampling 24344 Vectors 3637 64159 basis 648 characteristic 910 column 36 components of 64243 error 642 MATLAB operations 4546 matrix multiplication by 40 orthogonal space 64748 row 36 45 4850 signals as 64159 state 92730 961 Vestigial sideband VSB 749 Video signals 725 749 Waveshaping 61517 WeberFechner law 421 Width of the convolution integral 172 187 of the convolution sum 283 Window functions 74955 76062 ztransform 488592 bilateral See Bilateral ztransform difference equation solutions of 488 51019 574 direct 488592 discretetime Fourier transform and 86667 88688 898 existence of 49195 inverse See inverse ztransform properties of 5019 stability of 51819 statespace analysis and 956 95965 system realization and 51925 567 timereversal property 5067 timeshifting properties 5015 unilateral 489 491 492 495 55455 559 zdomain differentiation property 506 zdomain scaling property 505 Zero matrices 37 Zero padding 81011 82930 Zeroinput response 119 123 of continuoustime systems 15163 19596 203 22022 described 98100 of discretetime systems 27076 297301 30911 insights into behavior of 16163 of the Laplace transform 363 368 in oscillators 203 of the ztransform 51213 zerostate response independence from 161 Zeroorder hold ZOH filters 785 Zerostate response 119 123 alternate interpretation 51518 causality and 17273 of continuoustime systems 151 161 16896 22122 51216 described 98101 of discretetime systems 28098 3089 311 312 313 of the Laplace transform 358 363 36667 369 370 zeroinput response independence from 161 Zeros controlling gain by 540 filter design 43645 firstorder 42427 gain suppression by 43940 at the origin 42223 secondorder 42635 11LathiIndex 2017925 1929 page 989 15 11LathiIndex 2017925 1929 page 990 16